Accelerating Clinical Trials in Pharmaceuticals: The Power of ChatGPT
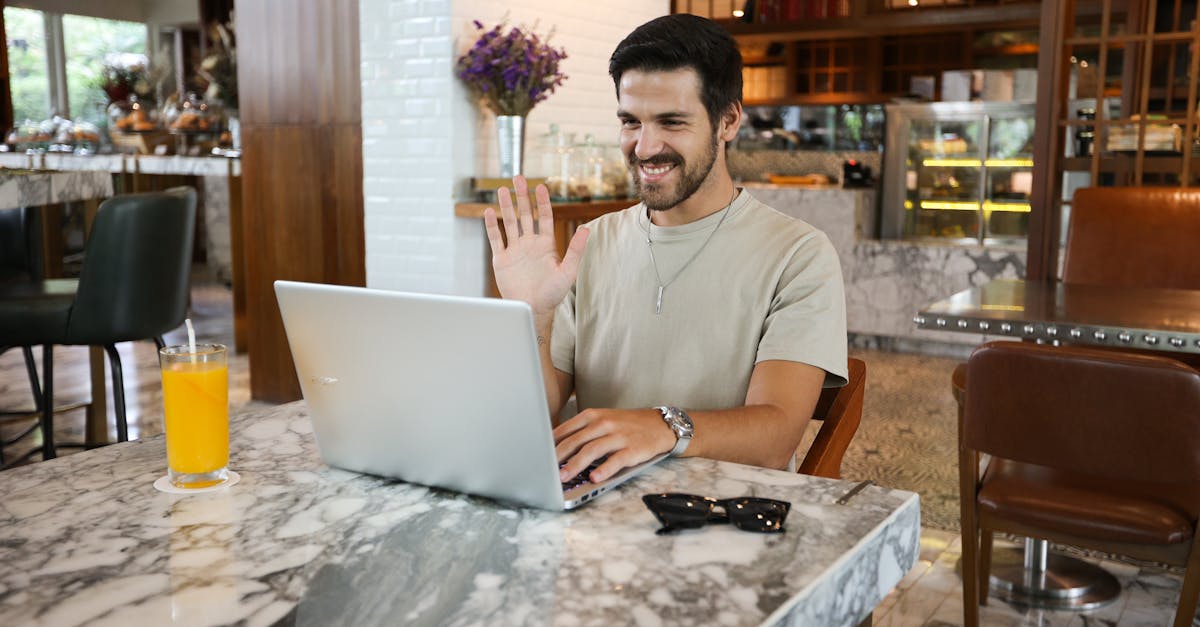
Clinical trials play a crucial role in the development and evaluation of new pharmaceutical drugs and medical treatments. These trials collect large amounts of data, ranging from patient demographics to treatment outcomes. Analyzing this data effectively and efficiently is essential for a successful clinical trial.
Machine learning, a branch of artificial intelligence, has emerged as a valuable tool in various industries, and it is now making significant contributions to the pharmaceutical field. Machine learning models can help streamline the data analysis process, providing researchers and professionals with deeper insights and more accurate predictions.
Utilizing Machine Learning Models
Machine learning models can be used in multiple stages of clinical trials, from patient selection to data interpretation. Here are some areas where machine learning models can be applied:
Patient Selection:
Identifying the right participants for clinical trials is crucial. Machine learning models can assist in screening patient records and identifying individuals who meet specific criteria. By analyzing large datasets, these models can identify patterns and characteristics that make someone an ideal candidate for a particular trial.
Drug Dosage Optimization:
Determining the optimal dosage for a drug is a complex process. Machine learning models can analyze patient responses and clinical data to predict the most effective dosage for different demographics, such as age, gender, or specific medical conditions.
Side Effects Prediction:
Machine learning models can help predict potential side effects of a drug based on data collected during clinical trials. By analyzing patient characteristics and treatment outcomes, these models can provide insights into the likelihood and severity of adverse reactions, thereby assisting in risk assessment and patient safety.
Data Analysis and Interpretation:
Clinical trial data can be vast and complex. Machine learning models can aid in comprehensively analyzing this data to identify correlations, trends, and patterns that may not be immediately apparent. These models can help uncover valuable insights that can guide decision-making and improve overall trial outcomes.
Benefits of Machine Learning Models
The utilization of machine learning models in clinical trials offers several benefits:
Efficiency:
Machine learning models can process large amounts of data quickly, saving time and resources for researchers. This efficiency allows for more extensive data analysis and reduces the chances of missing critical insights.
Precision:
Machine learning models can detect subtle patterns and trends in the data that human analysis may overlook. This level of precision can contribute to more accurate predictions, reducing errors and enhancing the overall quality of clinical trial outcomes.
Personalized Medicine:
With machine learning, pharmaceutical companies can potentially develop personalized therapies and treatment plans. By understanding how different patient characteristics influence treatment outcomes, individualized approaches can be tailored to maximize effectiveness and minimize adverse effects.
Cost-Effectiveness:
Machine learning models can aid in streamlining the clinical trial process, identifying inefficiencies, and reducing costs associated with unnecessary treatments or failed trials. By optimizing various aspects of clinical trials, machine learning models can help make the pharmaceutical industry more cost-effective.
Conclusion
Machine learning models have the potential to revolutionize the field of pharmaceuticals by improving the efficiency and accuracy of data analysis in clinical trials. Through their ability to process vast amounts of data and detect hidden patterns, these models can provide valuable insights that can lead to better treatment outcomes and improved patient safety. As technology continues to advance, the integration of machine learning in clinical trials is likely to become even more prevalent, ultimately benefiting both researchers and patients.
Comments:
Thank you all for taking the time to read my article on accelerating clinical trials with ChatGPT. I'm excited to hear your thoughts and answer any questions you may have!
Great article, Mark! The potential of ChatGPT in expediting pharmaceutical clinical trials is fascinating. I can see how it would greatly help with streamlining the recruitment process. Do you think there are any downsides or challenges to consider?
Hi Sarah, thank you for your comment! While ChatGPT offers significant benefits, there are a few challenges. One is ensuring the system accurately understands and responds to complex medical terminology. Another challenge is maintaining patient privacy and security during data interactions. These are areas where further research and development are necessary to overcome any potential downsides.
I'm impressed by the idea of using AI to speed up clinical trials. It can potentially save lives by getting new treatments to patients faster. However, I wonder if there could be any ethical concerns in relying too heavily on AI decisions. What's your take on this, Mark?
Hi David, that's an important consideration. While AI can assist in decision-making, it should never fully replace human judgment in clinical trials. Ethical concerns arise when AI is used without proper supervision or when its decisions are not thoroughly validated. Human oversight and maintaining a balance between AI and human decision-making are vital to ensure ethical practices in clinical trials.
This article highlights the potential of AI in revolutionizing the pharmaceutical industry. ChatGPT can play a significant role in accelerating the development of life-saving drugs. I'm curious, Mark, what other areas within the pharmaceutical industry do you think AI could have a major impact on?
Hi Emma, thanks for your question! AI can have a major impact on various areas in the pharmaceutical industry. It can assist in improving drug discovery by analyzing vast amounts of data, enhancing precision medicine, and optimizing manufacturing processes. Additionally, AI can contribute to personalized healthcare by providing tailored treatment options based on individual patient characteristics.
While AI holds promise, it's crucial to address concerns about bias in AI algorithms. We need to ensure that data used to train models like ChatGPT are diverse and representative. Mark, how do you think we can tackle this issue?
That's an excellent point, Lisa. Addressing bias requires careful data curation and the inclusion of diverse datasets representing different demographics. Additionally, continual monitoring, evaluation, and feedback loops can help identify and mitigate biases in AI algorithms. Collaborative efforts among researchers, regulators, and pharmaceutical companies are key to addressing this issue.
Hi Mark, I found your article very enlightening! I believe AI technology has the potential to reduce costs associated with clinical trials. By streamlining the process, we can expect reduced expenses for pharmaceutical companies. How do you think ChatGPT can specifically contribute to cost reduction?
Hi Robert, thank you for your comment. ChatGPT can contribute to cost reduction in several ways. By automating certain tasks, such as participant screening and data analysis, it can save both time and money. Additionally, faster recruitment and improved trial efficiency can shorten the overall duration of clinical trials, leading to cost savings for pharmaceutical companies.
Mark, in your article, you mentioned the power of ChatGPT in patient engagement during clinical trials. Could you elaborate on how this technology can improve patient involvement and experience?
Certainly, Sarah! ChatGPT can enhance patient involvement by providing personalized support and education throughout the clinical trial process. It can answer common questions, address concerns, and ensure timely communication between patients and researchers. This technology creates a more engaging and informed patient experience, ultimately leading to better overall trial outcomes.
I'm excited about the potential of ChatGPT in expediting drug approvals. With many patients waiting for life-saving treatments, speeding up the regulatory process is crucial. Mark, do you think regulatory agencies will embrace AI technologies like ChatGPT in the near future?
Hi Jennifer, indeed, expediting drug approvals is crucial. Regulatory agencies are becoming increasingly aware of the potential of AI technologies in improving clinical trials and decision-making. However, the adoption of AI in regulatory processes will require careful evaluation, validation, and clear guidelines to ensure safety, transparency, and effectiveness. I believe we will see gradual but steady progress in embracing AI technologies like ChatGPT.
One concern I have is the potential for job displacement due to increased automation in clinical trials. What impact do you think AI adoption, particularly ChatGPT, will have on job roles within the pharmaceutical industry?
Hi David, that's an important consideration. While there may be some automation of certain tasks in clinical trials through AI adoption, it's crucial to remember that AI is a tool meant to complement human expertise, not replace it entirely. The role of human researchers, scientists, and clinicians will continue to be essential for decision-making, data interpretation, and providing necessary context. AI adoption should be seen as an opportunity to augment existing job roles rather than render them obsolete.
ChatGPT seems like a powerful tool for researchers. I'm curious, Mark, what are the key factors to consider when implementing ChatGPT in clinical trial settings?
Hi Emma, great question! When implementing ChatGPT in clinical trials, it's crucial to ensure proper data handling and privacy protection. The system should be thoroughly trained using relevant datasets and validated to ensure accuracy. Additionally, adequate user support and training should be provided to researchers to maximize the benefits of ChatGPT. Ongoing monitoring and iterative improvements are also important to maintain and enhance its performance.
Mark, you've highlighted the potential of ChatGPT in clinical trials. How do you see the future of AI in the pharmaceutical industry? What advancements can we expect in the coming years?
Hi Robert, the future of AI in the pharmaceutical industry looks promising. We can expect advancements in areas such as drug discovery, personalized medicine, predictive analytics, and clinical trial optimization. AI algorithms will become more robust and accurate, enabling better decision-making throughout the drug development process. Additionally, AI can contribute to the identification of new therapeutic targets, leading to innovative treatments for various diseases.
The potential of ChatGPT in clinical trials is exciting, but I'm curious about any limitations the technology might have. Mark, what are some challenges that need to be addressed for the wider adoption of ChatGPT in the pharmaceutical industry?
Hi Lisa, excellent question! One challenge is making ChatGPT more interpretable and explainable. Ensuring transparency in its decision-making process is crucial, especially when dealing with sensitive medical data. Additionally, continued research, evaluation, and addressing potential biases are essential for the wider adoption of ChatGPT. Regulatory guidelines and standards specific to AI applications in the pharmaceutical industry would also greatly facilitate its adoption.
Kudos on the article, Mark. I believe AI technologies like ChatGPT can also aid in detecting adverse events during clinical trials more efficiently. What are your thoughts on this potential application?
Thank you, Sarah. You're absolutely right! AI technologies like ChatGPT can contribute to early detection and monitoring of adverse events during clinical trials. By analyzing real-time patient data, the system can proactively identify potential risks or adverse reactions, enabling timely intervention and more accurate safety assessments. This application has the potential to greatly enhance patient safety and improve the overall trial monitoring process.
Mark, you've discussed the benefits of ChatGPT in clinical trials. Are there any current success stories where AI technologies have already made a significant impact on accelerating the pharmaceutical research process?
Hi Jennifer, there are indeed success stories where AI technologies have made significant impacts. For example, AI algorithms have assisted in identifying potential drug candidates from vast libraries of compounds, helping to accelerate the discovery phase. AI-based predictive models have also been developed to optimize clinical trial designs, matching specific patient populations with trial protocols more efficiently. These advancements showcase the potential of AI in pharmaceutical research.
It's great to see the potential of AI in accelerating clinical trials. However, I'm curious about the level of acceptance among stakeholders like physicians and patients. Mark, have you seen any resistance or hesitation toward adopting AI technologies in the clinical trial process?
Hi David, adoption of AI technologies in the clinical trial process is not without challenges. While some stakeholders are receptive to the potential benefits, there might be resistance due to concerns around data privacy, trust in AI systems, or simply adjusting to new technological approaches. Overcoming these challenges requires clear communication, education, and demonstrating the value and real-world impact of AI in the context of clinical trials.
Mark, I appreciate the insights shared in your article. I'm curious about the scalability of ChatGPT in handling large-scale clinical trials. Can you elaborate on its capacity to accommodate studies with high recruitment numbers?
Thank you, Emma. ChatGPT has the potential for scalability in handling large-scale clinical trials. By automating aspects of participant screening and providing real-time support, it can help manage higher recruitment numbers effectively. However, it's crucial to ensure the system's performance and responsiveness scale accordingly to maintain optimal engagement and support for all participants.
The use of AI in clinical trials looks promising, but I wonder about potential security risks. How can we ensure that patient data shared with a system like ChatGPT remains secure and protected from unauthorized access?
Hi Robert, safeguarding patient data is of utmost importance. When implementing ChatGPT or any AI system, pharmaceutical companies must adhere to strict data protection protocols. This involves using encryption techniques, secure communication channels, and ensuring compliance with relevant privacy regulations, such as HIPAA. Proactive measures and continuous monitoring can help mitigate security risks and protect patient information from unauthorized access.
I'm curious about the user experience with ChatGPT during clinical trials. Mark, have there been any user studies or feedback from participants that shed light on how they perceive and interact with the system?
Great question, Lisa. User experience is key to the successful adoption of ChatGPT. While user studies and feedback specifically related to clinical trials are limited at this early stage, preliminary studies involving other AI chatbot systems have shown positive perceptions from participants. Participants often appreciate the accessibility, prompt responses, and personalized support provided by chatbot systems. Ensuring continuous user feedback and iterative improvements are vital for enhancing the user experience in clinical trial settings.
Mark, you've covered many potential benefits of ChatGPT. I'm curious to know if there are any limitations or situations where this technology might not be as effective in clinical trials. Can you shed some light on this?
Certainly, Sarah. While ChatGPT has great potential, it does have limitations. The system heavily relies on training data, and if that data is not comprehensive or accurate, it may result in biased or incorrect responses. Additionally, ChatGPT may struggle with rare or complex scenarios where the data it was trained on is limited. Balancing AI capabilities with human expertise is crucial to overcome these limitations and ensure the technology's effectiveness across various clinical trial situations.
It's fascinating to see how AI is transforming the pharmaceutical industry. Mark, what are your thoughts on the integration of ChatGPT with other emerging technologies like blockchain for improved data security and transparency in clinical trials?
Hi Jennifer, the integration of ChatGPT with blockchain technology holds great promise in ensuring data security and transparency in clinical trials. By leveraging blockchain's decentralized nature and immutability, we can enhance data privacy, traceability, and eliminate single points of failure. Combining ChatGPT with blockchain can enable secure patient data exchange, auditability, and reinforce trust among all stakeholders involved in the clinical trial process.
Mark, thank you for bringing attention to the potential of ChatGPT in pharmaceutical clinical trials. How long do you think it will take for widespread adoption of AI technologies like this in the industry?
Hi David, predicting the exact timeline for widespread adoption is challenging, as it depends on various factors such as further advancements in AI, regulatory frameworks, and the pace of technology integration within the industry. However, given the momentum and increasing recognition of AI's benefits, I believe we will see progressive adoption over the next decade, with early adopters leading the way and setting precedents for wider implementation.
AI-powered ChatGPT certainly opens up exciting possibilities in the world of clinical trials. To fully realize its potential, what are the key areas you believe need further research, Mark?
Excellent question, Emma. Further research is crucial to harness the full potential of ChatGPT and AI in clinical trials. Some key areas include addressing biases and ensuring fairness in AI decisions, improving interpretability and explainability of AI models, expanding the model's domain expertise, exploring multi-modal interactions, and conducting rigorous validation studies to evaluate system performance on a broader scale. Continuous improvements and advancements in these areas will enhance the reliability and effectiveness of ChatGPT.
Mark, your article is insightful and thought-provoking. In terms of collaboration, do you think there is a need for the industry to come together to establish standardized protocols or guidelines for AI adoption in clinical trials?
Hi Robert, absolutely! Collaboration and standardization are critical for responsible AI adoption in clinical trials. Establishing standardized protocols, guidelines, and best practices will ensure consistency, transparency, and ethical use of AI across the industry. Collaborative efforts between pharmaceutical companies, regulatory agencies, researchers, and other stakeholders can collectively shape the future of AI integration, while maintaining the highest standards of safety, efficacy, and patient privacy.
As AI technologies continue to evolve, how do you see the role of human researchers and clinicians evolving? Is there a concern that AI might replace or diminish their roles in the clinical trial process?
Hi Lisa, AI should never replace or diminish the roles of human researchers and clinicians in the clinical trial process. Rather, AI should be viewed as a complementary tool that streamlines workflows, enhances decision-making, and aids in data analysis. The role of human researchers and clinicians will evolve to incorporate and leverage AI technologies, allowing them to focus more on complex tasks, interpretation of results, and demonstrating expertise through informed judgments. This collaboration can lead to improved outcomes and better patient care.
Thank you, Mark, for sharing your insights. Do you think ChatGPT has the potential to go beyond clinical trials and impact other areas within the healthcare industry as well?
Certainly, Sarah. While the focus of this article is on clinical trials, ChatGPT and similar AI technologies have applications beyond that scope. In healthcare, they can assist in remote patient monitoring, triage, and diagnosis, telemedicine consultations, personalized healthcare recommendations, and even empowering patients with accurate medical information. AI has the potential to transform many aspects of healthcare delivery, making it more efficient, accessible, and patient-centered.
Thank you, Mark, for shedding light on the potential of ChatGPT in clinical trials. Your article has sparked interesting discussions! I look forward to witnessing how AI continues to shape the future of pharmaceutical research and patient care.
Thank you all for the engaging discussions and insightful questions. It has been a pleasure sharing my thoughts on the power of ChatGPT in accelerating clinical trials. I share your excitement about AI's transformative potential in the pharmaceutical industry. Let's continue to explore and innovate together!