Accelerating Data Analysis with ChatGPT: A Solution-Focused Approach
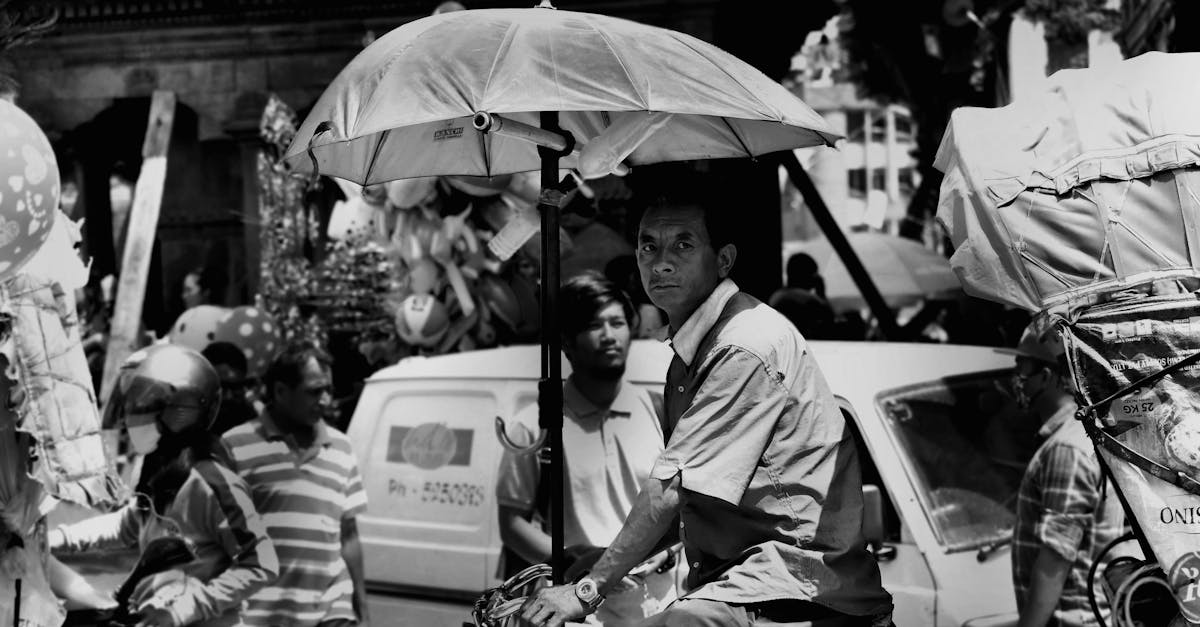
Data analysis plays a crucial role in extracting insights and valuable information from large datasets. As the volume and complexity of data continue to grow exponentially, there is a pressing need for advanced technologies that can aid data analysts in analyzing and summarizing data efficiently. GPT-4, a state-of-the-art natural language processing technology, is the perfect solution that meets these requirements.
Technology Overview
GPT-4, developed by OpenAI, stands for "Generative Pre-trained Transformer 4". It is an advanced language model that has been trained on vast amounts of data and possesses the ability to generate human-like text. GPT-4 leverages deep learning techniques, neural networks, and transformer architectures to process and analyze large datasets in a highly efficient manner.
Area of Application
Data analysis is a broad field with applications in various industries, including finance, healthcare, marketing, and more. GPT-4 can be utilized in any domain that requires analysis of substantial amounts of data to extract meaningful insights. It can handle structured, unstructured, and semi-structured data, making it versatile for different use cases.
Usage Benefits
- Efficiency: GPT-4 significantly reduces the time taken for data analysis by automating the process of summarizing insights. It can analyze large datasets and present the findings in a concise and understandable language, saving valuable time for data analysts.
- Accuracy: With GPT-4's advanced natural language processing capabilities, the generated summaries are highly accurate and coherent. It can identify patterns, trends, and correlations within the data, enabling accurate interpretation of complex information.
- Language Flexibility: GPT-4 supports multiple languages, allowing data analysts to work with datasets written in different languages effortlessly. This feature makes it an invaluable tool for global organizations working with diverse datasets.
- Scalability: As the volume of data continues to grow, GPT-4's scalability ensures that it can handle and process large datasets without compromising performance. It can analyze vast amounts of data efficiently, making it suitable for enterprise-level data analysis tasks.
- Ease of Use: GPT-4's user-friendly interface and intuitive functionalities make it accessible to both technical and non-technical users. Data analysts can utilize its power without extensive programming knowledge, fostering collaboration and enhancing productivity.
Conclusion
GPT-4 is revolutionizing the field of data analysis by providing a solution-focused approach. Its ability to analyze vast datasets and summarize valuable insights in a more understandable language saves significant time for data analysts, allowing them to focus on critical decision-making tasks. With enhanced efficiency, accuracy, language flexibility, scalability, and ease of use, GPT-4 is an indispensable technology for unlocking the true potential of data analysis. Embrace the power of GPT-4 and elevate your data analysis capabilities to new heights!
Comments:
Thank you all for reading my article on accelerating data analysis with ChatGPT! I'm excited to hear your thoughts and discuss further.
Great article, Hank! I found the use of ChatGPT in data analysis very intriguing. It seems like a powerful tool for speeding up the process. Can you share any specific examples where you've applied it?
Hi Laura, thanks for your comment! One example where I have used ChatGPT in data analysis is for anomaly detection in large datasets. By training the model on normal patterns, it can aid in identifying outliers or anomalies more effectively.
Thanks for the example, Hank! It's impressive how ChatGPT can enhance anomaly detection. I can see the potential for various applications across different industries.
I agree with you, Laura. The versatility of ChatGPT in anomaly detection can be groundbreaking. It's exciting to think about the potential impact it can have on various industries.
Hi Hank, thanks for explaining the benefits of using ChatGPT in data analysis. I wonder, are there any limitations or challenges associated with this approach that we should be aware of?
Hi Mark, great question! While ChatGPT is a powerful tool, it does have some limitations. One challenge is the need for a large amount of training data to ensure accurate results. Additionally, it may struggle with handling complex or ambiguous queries that require domain-specific knowledge. However, continuous improvements are being made to address these challenges.
I enjoyed reading your article, Hank. ChatGPT seems like a breakthrough in data analysis. Have you encountered any privacy concerns when working with sensitive data?
Hi Emily, I appreciate your positive feedback! When working with sensitive data, ensuring privacy is indeed crucial. It's important to follow best practices such as data anonymization, encryption, and access controls to protect sensitive information when using ChatGPT or any other data analysis tool.
Hi Hank, great article! I'm curious, how does ChatGPT compare to traditional data analysis methods in terms of accuracy and efficiency? Is it suitable for real-time analysis?
Hank, your article has opened up new possibilities for data analysis. However, I wonder if there are specific use cases where ChatGPT may not be the ideal approach?
Hi Sophia, you bring up an excellent point! While ChatGPT offers many benefits, it might not be the ideal approach for use cases where real-time analysis is required. It may have limitations in terms of response time, especially with large datasets or complex queries. For time-critical applications, traditional data analysis methods might still be more suitable.
Thanks for your response, Hank. That clarifies the scenario where ChatGPT might not be the best fit. It's important to consider the specific requirements and constraints of each use case.
Hank, your article highlights the potential of ChatGPT in data analysis. Have you observed any specific challenges in implementing and fine-tuning the model for different types of analytical tasks?
Hi Benjamin, thanks for your question! Implementing ChatGPT for different analytical tasks can sometimes be challenging due to the need for domain expertise in fine-tuning the model. Designing the conversational setup and training data can be time-consuming, but it's essential for achieving accurate results in specific use cases.
Great article, Hank! I'm curious about the computational resources required for training and deploying ChatGPT for data analysis. Can you provide any insights?
Hi Natalie, computational resources are an essential consideration when working with ChatGPT. Training the model with large datasets can require significant computational power, including GPUs or TPUs. However, deploying ChatGPT for data analysis tasks can be done on less resource-intensive hardware, depending on the specific requirements and scale of the analysis.
Hank, your article sheds light on an exciting approach to data analysis. In your experience, how does the interpretability of ChatGPT compare to traditional analysis methods? Can we trust the recommendations made by the model?
Hi Jason, interpretability is indeed an important aspect. While traditional analysis methods often provide clearer insights into the reasoning behind recommendations, ChatGPT can sometimes lack interpretability. This is because the model is based on statistical patterns learned during training, making it challenging to fully understand the decision-making process. Trusting the recommendations made by ChatGPT requires careful validation and domain expertise to ensure the results align with expectations.
Great article, Hank! I'm curious, how do you see the future of ChatGPT evolving in the field of data analysis? Do you anticipate any major advancements or challenges?
Hi Alyssa, great question! The future of ChatGPT in data analysis holds significant potential. Advancements in fine-tuning techniques and training methodologies may lead to even higher accuracy and efficiency. However, challenges such as ethical considerations, bias detection, and overcoming limitations in understanding complex queries will require ongoing research and development. Overall, I believe ChatGPT will continue to play a valuable role in data analysis, complementing traditional methods.
Hank, your article was enlightening! Are there any known security risks associated with using ChatGPT for analyzing sensitive data? How can such risks be mitigated?
Hi Emma, security risks are an important consideration when working with sensitive data. While ChatGPT itself is a language model, precautions must be taken during implementation and deployment. Data encryption, secure access controls, and auditing practices can help mitigate security risks associated with using ChatGPT or any other data analysis tool.
Great article, Hank! I'm wondering if ChatGPT can be integrated with existing data analysis tools and workflows.
Hi Robert, integrating ChatGPT with existing data analysis tools and workflows is definitely possible. RESTful APIs or SDKs can enable seamless integration, allowing organizations to leverage the power of ChatGPT while maintaining the efficiency of their established analysis processes.
Hi Hank, excellent article! How do you ensure that ChatGPT provides accurate and reliable analysis results, especially when dealing with noisy or incomplete datasets?
Hi Kelly, ensuring accurate analysis results with noisy or incomplete datasets can be challenging. It's important to preprocess and clean the data as much as possible before utilizing ChatGPT. Data cleaning techniques such as handling missing values, outlier detection, and noise reduction can improve the quality of input data, thereby enhancing the reliability and accuracy of ChatGPT's analysis results.
Great read, Hank! Can ChatGPT assist in data cleaning and preprocessing tasks as well, or is it primarily focused on analysis itself?
Hi Luis, ChatGPT can assist in data cleaning and preprocessing tasks to some extent. It can help in identifying data patterns, suggesting potential preprocessing steps, and guiding users through the data preparation phase. However, incorporating domain-specific preprocessing techniques and expert knowledge alongside ChatGPT is essential for optimal data cleaning and preprocessing.
Hank, ChatGPT seems promising. How do you handle cases where the model produces incorrect or biased analysis results?
Hi Sophie, addressing incorrect or biased analysis results is crucial. Ensuring a diverse and representative training dataset, regularly monitoring model performance, and validating results against ground truth are important steps. If biases are detected, refining the training data and considering alternative fine-tuning techniques can help mitigate such issues and enhance the overall accuracy and fairness of ChatGPT's analysis results.
Great article, Hank! How do you tackle the challenge of explainability when using ChatGPT for data analysis? Can it provide insights into how it reached certain conclusions?
Hi Oliver, explainability is a challenge with ChatGPT, as it's harder to interpret its decision-making process. While it doesn't provide direct insights into how it reached conclusions, techniques like attention mapping and interpretability methods can offer some level of explanation. Additionally, external auditing, running sensitivity analyses, and comparing against established analytical methods can help increase confidence in the model's conclusions.
Hi Hank, great article! With the rapid advancements in AI, how do you envision ChatGPT impacting the role of data analysts and scientists in the future?
Hi Sophie, ChatGPT is likely to impact the role of data analysts and scientists significantly. While there will be an increased reliance on AI models like ChatGPT for data analysis tasks, the role of analysts will evolve to focus more on the interpretation, validation, and integration of the outputs. Analysts will play a crucial role in understanding the context, refining the models, and ensuring alignment with business goals and human expertise.
Interesting read, Hank! How do you handle cases where ChatGPT may provide analysis results that are contradictory or conflicting with known ground truth?
Hi Rachel, handling contradictory or conflicting analysis results requires careful investigation. It's important to assess the quality and diversity of the training data, fine-tuning process, and evaluate if the issue arises from specific input scenarios. Conducting thorough analysis validation, collecting additional ground truth data, and refining the model or training techniques can help address such cases and improve the overall accuracy of ChatGPT's results.
Hi Hank! Your article was thought-provoking. What are the potential applications of ChatGPT in sectors beyond data analysis?
Hi Sophia, beyond data analysis, ChatGPT has potential applications in customer support, virtual assistants, content creation, and even creative writing. It can provide human-like interactions and generate contextually relevant responses, making it valuable in various sectors where natural language understanding and generation are essential.
Great article, Hank! How can organizations ensure the responsible and ethical use of ChatGPT in data analysis?
Hi Thomas, ensuring responsible and ethical use of ChatGPT is crucial. Organizations should establish clear guidelines for data usage, implement bias detection systems, and continuously monitor and evaluate the model's performance. It's crucial to train the model on diverse and inclusive datasets and involve domain experts in the fine-tuning process to avoid biased or harmful results. Regular audits and transparent disclosure of the model's limitations also contribute to responsible and ethical use.
Hank, fascinating article! Are there any alternative solutions to ChatGPT for accelerating data analysis, or is it currently the most effective approach?
Hi Marco, while ChatGPT is a powerful approach, there are alternative solutions for accelerating data analysis. Traditional methods like data mining, machine learning algorithms, and more targeted task-specific models can also be effective depending on the use case. ChatGPT offers a more conversational and interactive analysis experience, but evaluating the specific requirements and constraints will help determine the most effective approach.
Great insights, Hank! Is ChatGPT suitable for small-scale data analysis, or is it primarily beneficial for larger datasets?
Hi Sara, ChatGPT can be beneficial for both small-scale and larger datasets. However, the scale and complexity of the dataset may influence the model's performance. While ChatGPT excels in providing conversational insights, for extremely small datasets or analysis tasks where fine-grained control over analytical steps is crucial, traditional methods might be a better fit. The suitability of ChatGPT depends on the specific use case and available training data.
Hank, your article presents an exciting approach for data analysis. Can ChatGPT be used to automate the entire data analysis process, or is it more of an assistance tool for analysts?
Hi Ethan, ChatGPT can be used as an assistance tool throughout the data analysis process, but automating the entire process solely based on ChatGPT might not be ideal. While it can aid in tasks like data exploration, visualization suggestions, initial insights, and even specific analysis steps, the interpretability and control provided by traditional methods make them necessary for a robust analysis process. A combination of ChatGPT and traditional methods is often more effective.
Interesting perspective, Hank! How does ChatGPT deal with noisy or unstructured data, where conventional methods might struggle?
Hi Julia, dealing with noisy or unstructured data is a challenge for many conventional methods. ChatGPT has shown promise in understanding and making sense of such data, as it can learn patterns and potentially uncover hidden insights. Its ability to process and generate language-based responses makes it well-suited to handle unstructured or semi-structured data, where traditional methods might struggle due to the lack of clear rules or well-defined structures.