Achieving Accurate Data Traffic Forecasting in Optical Communications with ChatGPT
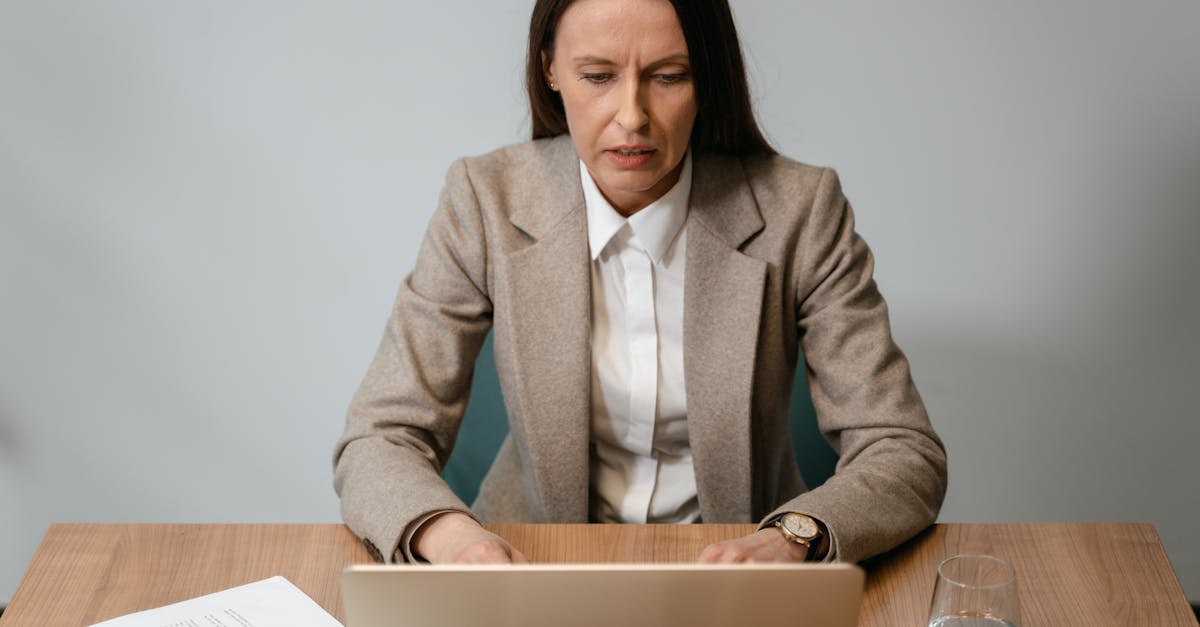
In today's digital era, where data consumption is increasing exponentially, efficient management and optimization of network resources have become paramount for telecommunication service providers. Optical communications, a technology that utilizes light as a means of transferring data, has played a crucial role in ensuring high-speed and reliable data transmission. However, with the ever-growing demand for data, it is essential to forecast network traffic accurately to optimize resource allocation.
Data traffic forecasting refers to the process of predicting the future data demand on a network. Traditionally, such forecasting has relied on statistical methods and historical data analysis. While these approaches have been useful, the complexity and unpredictability of modern data traffic patterns call for more advanced techniques. This is where artificial intelligence (AI) comes into play.
AI, specifically machine learning algorithms, can analyze vast amounts of data and learn patterns and trends. By training on historical data and considering various influencing factors, such as time of day, day of the week, seasonal trends, and user behaviors, AI models can generate accurate forecasts for data traffic. This enables telecom operators to anticipate network congestion, plan network expansions, and allocate resources efficiently.
One of the significant advantages of using AI for data traffic forecasting in optical communications is its ability to adapt and improve over time. As it analyzes new data, AI algorithms fine-tune their models, enhancing the accuracy of future predictions. This iterative learning process ensures that forecasting models stay up-to-date with changing network dynamics and user behaviors.
Optimizing resource allocation based on accurate data traffic forecasts leads to several benefits. Firstly, it allows telecom operators to allocate network bandwidth and capacity more effectively, ensuring a smoother user experience and reducing the likelihood of network congestion. Secondly, it enables proactive maintenance and upgrades, reducing downtime and improving overall network reliability. Thirdly, it helps minimize operational costs by avoiding unnecessary overprovisioning of network resources.
Another crucial aspect of data traffic forecasting in optical communications is the ability to identify and predict emerging trends and patterns. AI models can detect anomalies and deviations from normal traffic patterns, providing early warnings for potential network issues or security threats. This proactive approach enables telecom operators to take preventive actions and maintain network integrity.
In conclusion, the combination of optical communications and AI-powered data traffic forecasting provides telecom operators with a powerful tool to optimize network resource allocation. By accurately predicting future data traffic demand, telecom operators can ensure a reliable and efficient network infrastructure. The iterative learning capabilities of AI algorithms enable continuous improvement and adaptation to changing network dynamics. As data consumption continues to grow, the integration of AI into optical communications will play an increasingly significant role in meeting the demands of the digital age.
Comments:
Thank you all for reading my article on achieving accurate data traffic forecasting in optical communications with ChatGPT. I'm excited to discuss this topic with you.
Great article, Mark! I've been following advancements in optical communications, and utilizing ChatGPT for data traffic forecasting seems like a promising approach.
Thank you, Sarah! Optical communications indeed hold tremendous potential, and ChatGPT can be a valuable tool in optimizing data traffic management.
Mark, I'm impressed with the potential of using ChatGPT for accurate data traffic forecasting in optical communications. The ability to predict network traffic patterns can greatly improve network efficiency.
Very informative article, Mark! Is ChatGPT based on machine learning algorithms? I'd love to learn more about the technical aspects of this technology.
Emily, thank you for your kind words! Yes, ChatGPT is built on powerful machine learning algorithms, specifically employing transformers for natural language processing.
Mark, how accurate are the data traffic predictions using ChatGPT? Are there any limitations or challenges to consider?
Liam, the accuracy of ChatGPT's data traffic predictions depends on various factors, including the quality and quantity of training data. It's important to understand that although it's generally accurate, occasional outliers and unforeseen scenarios can impact accuracy.
Hi Mark! I'm curious to know if ChatGPT can adapt to changing network conditions and adjust its predictions accordingly.
Megan, great question! ChatGPT can adapt to changing network conditions up to a certain extent. However, significant changes and network-wide disruptions may require periodic retraining to ensure accurate predictions.
Hey Mark, could you elaborate on how ChatGPT handles real-time data traffic forecasting? Does it require substantial computing resources?
Nathan, ChatGPT can handle real-time data traffic forecasting with reasonable computational resources. However, for extremely large-scale networks, distributed computing or specialized hardware may be required to ensure efficient processing.
Mark, can ChatGPT be used to forecast traffic in different regions simultaneously? Can it adapt to different network infrastructures and configurations?
Benjamin, ChatGPT can be used to forecast traffic in different regions, assuming appropriate training and relevant historical data are available. It has the flexibility to adapt to different network infrastructures and configurations through continuous learning and fine-tuning.
Hi Mark! How scalable is ChatGPT for large-scale optical communication networks? Can it handle enormous amounts of data and predict accurately in such scenarios?
Olivia, ChatGPT's scalability is one of its strengths. It can handle large-scale optical communication networks while maintaining reasonable prediction accuracy. Plus, it has the ability to process enormous amounts of data efficiently.
Mark, can ChatGPT detect and predict anomalous network activity or potential security threats based on data traffic patterns?
Sophia, while ChatGPT can analyze data traffic patterns, it's primarily focused on accurate forecasting rather than anomaly detection or security threat identification. Dedicated security solutions may be more appropriate for such concerns.
Mark, it would be interesting to know how ChatGPT compares to traditional methods of data traffic forecasting in optical communications. Are there any notable advantages or limitations?
Oliver, compared to traditional methods, ChatGPT has the advantage of leveraging deep learning techniques to capture complex patterns in data traffic. It can adapt and learn from new data more effectively. However, ensuring a diverse and representative training dataset is crucial to overcome limitations and biases.
Hi Mark! How does ChatGPT handle unexpected or sudden spikes in data traffic? Can it provide real-time alerts or recommendations to avoid network congestion?
Isabella, ChatGPT can handle unexpected spikes in data traffic to some extent. It's capable of providing real-time recommendations to avoid network congestion, but it's essential to have appropriate mechanisms and network resources in place to implement those recommendations.
Mark, are there any potential limitations or risks associated with relying solely on ChatGPT for data traffic forecasting? What are some best practices to ensure reliable predictions?
Mia, like any forecasting tool, ChatGPT has limitations. It's essential to keep monitoring the predictions, compare them to actual network conditions, and make necessary adjustments based on domain expertise. Combining ChatGPT with other forecasting methods and validation techniques can help enhance reliability.
Mark, does ChatGPT's accuracy improve over time with continued usage and feedback? Can it learn from its own predictions and user interventions to enhance its forecasting capabilities?
Ethan, ChatGPT's accuracy can improve over time through continued usage and feedback. It can learn from its own predictions and user interventions, allowing it to refine its forecasting capabilities and adapt to specific network contexts.
Mark, are there specific criteria to evaluate the accuracy of ChatGPT's predictions? How can we measure its performance against ground truth or other benchmarks?
Lucas, evaluating the accuracy of ChatGPT's predictions can be done by comparing the forecasted network traffic with the actual traffic data, along with considering various metrics like mean absolute error or root mean square error. It's crucial to establish suitable benchmarks and ground truth data for comparison.
Hi Mark! Are there any privacy concerns to consider when deploying ChatGPT for data traffic forecasting in optical communications? How is user data handled?
Julia, privacy concerns are significant. When deploying ChatGPT, it's crucial to ensure compliance with relevant data protection regulations. User data should be anonymized and only used for the intended purposes, with appropriate security measures in place.
Mark, can ChatGPT handle intermittently changing traffic patterns or sudden shifts? For example, during peak hours or significant events that cause temporary surges in data traffic.
Mark, what are the main differences between using ChatGPT and traditional statistical or mathematical models for data traffic forecasting?
Hi Mark! Has ChatGPT been applied in real-world scenarios for data traffic forecasting? Can you share any success stories or use cases?