Advancements in Data Analysis: Leveraging ChatGPT for Anomaly Detection
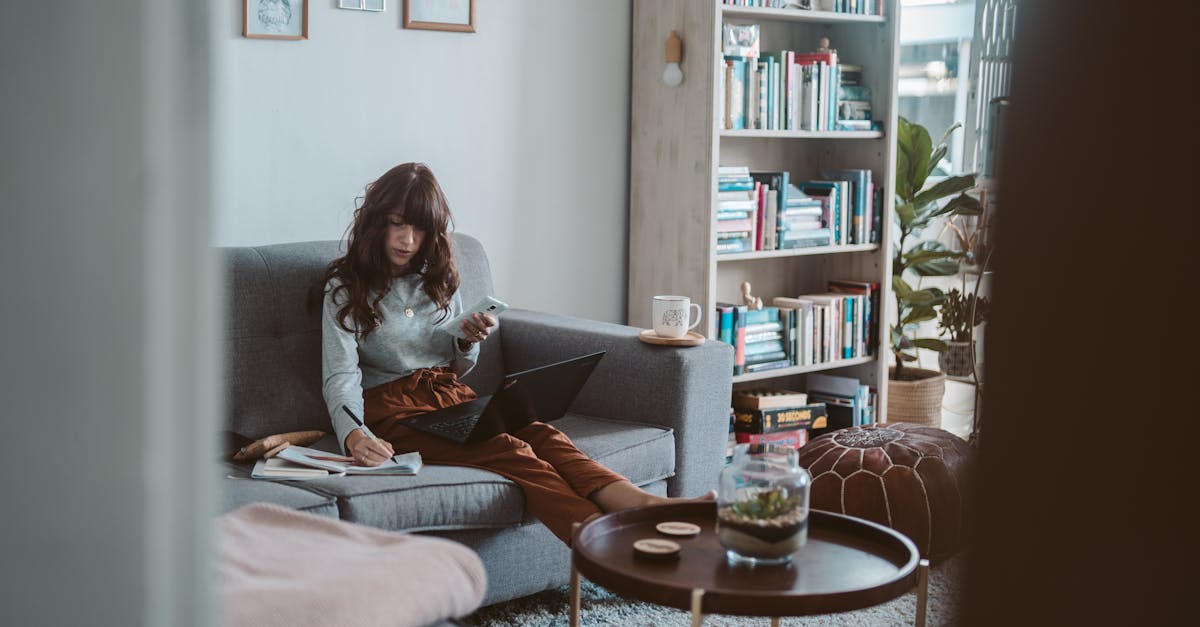
Introduction
Data analysis is a crucial aspect of many industries, allowing organizations to derive valuable insights from vast amounts of information. One specific area of data analysis that holds significant importance is anomaly detection. Anomaly detection involves identifying unusual patterns or outliers in data that deviate from the expected behavior. In recent years, advancements in technologies like artificial intelligence and machine learning have revolutionized anomaly detection, giving rise to more accurate and efficient methods.
ChatGPT-4: An Innovative Solution for Anomaly Detection
ChatGPT-4, an advanced language model developed by OpenAI, is a cutting-edge solution that can be harnessed for anomaly detection tasks. Built upon the success of its predecessors, ChatGPT-4 utilizes deep learning techniques to understand and analyze complex textual information. With its ability to comprehend natural language, ChatGPT-4 can assist analysts and data scientists in identifying anomalies within given datasets.
Identifying Unusual Patterns
ChatGPT-4's language understanding capabilities enable it to recognize regular patterns and trends within data. By being trained on a wide range of datasets, it develops an understanding of what constitutes typical behavior. Once familiar with the expected behavior, ChatGPT-4 can compare new data points against these learned patterns to identify any deviations or outliers. This process allows analysts to quickly and accurately flag potential anomalies for further investigation.
Enhancing Anomaly Detection Accuracy
ChatGPT-4's sophisticated algorithms and vast pre-training enable it to handle various data formats, including structured, unstructured, and semi-structured data. This versatility allows analysts to detect anomalies across different types of datasets, such as customer transactions, server logs, or sensor readings. By considering multiple dimensions of the data, ChatGPT-4 can provide more accurate anomaly detection results and minimize false positives.
Utility in Real-World Scenarios
The applications of ChatGPT-4 in anomaly detection are diverse, spanning across industries. For example, financial institutions can leverage ChatGPT-4 to detect potentially fraudulent transactions by identifying unusual spending patterns or transaction amounts. In manufacturing environments, ChatGPT-4 can help monitor equipment sensors and identify anomalous behavior that may indicate machinery malfunction or maintenance needs.
Furthermore, ChatGPT-4 can be integrated into chatbot systems to provide real-time anomaly detection capabilities. By analyzing user inputs and conversations, the model can identify suspicious or improper behavior, ensuring a secure and reliable user experience.
Conclusion
Anomaly detection plays a vital role in modern data analysis, enabling organizations to proactively identify and mitigate potential issues. With the advent of ChatGPT-4, the accuracy and efficiency of anomaly detection have been significantly enhanced. Its ability to understand and analyze natural language allows it to identify unusual patterns and outliers within datasets, across various industries and applications. By incorporating ChatGPT-4 into their data analysis workflows, organizations can harness the power of advanced AI technologies to ensure the integrity and reliability of their systems.
Comments:
Thank you all for reading my article on leveraging ChatGPT for anomaly detection. I'm excited to hear your thoughts and answer any questions you may have!
Great article, Kerry! It's fascinating to see how natural language processing can be applied to data analysis. Do you think ChatGPT can handle real-time anomaly detection?
Thank you, Alice! While ChatGPT can process data in real-time, its performance may vary depending on the complexity and volume of anomalies. It's more suitable for scenarios with moderate anomaly rates.
That makes sense, Kerry. So, it's crucial to ensure the training data represents a wide range of anomalies to avoid false negatives. How do you verify ChatGPT's performance?
Alice, excellent question! We typically use evaluation metrics such as precision, recall, and F1 score to assess ChatGPT's performance. Additionally, manual review and user feedback are valuable in refining and fine-tuning the model.
Great article, Kerry! I'm curious, have you tested ChatGPT's anomaly detection capabilities on any real-world datasets? How well does it work in practice?
Thank you, David! Yes, ChatGPT has been tested on several real-world datasets across domains like finance, healthcare, and cybersecurity. While it performs well in practice, the results may vary depending on the specific dataset and anomaly characteristics.
That's impressive! It's good to know ChatGPT has practical applications across various domains. Keep up the great work, Kerry!
Thank you, Kerry! You've provided valuable insights into the considerations for applying ChatGPT in anomaly detection. It's exciting to see how AI techniques are transforming data analysis!
Kerry, I appreciate your insights into the real-time and batch processing capabilities of ChatGPT. It's important to consider the specific requirements and resources when implementing anomaly detection solutions.
Thank you, Alice! Indeed, understanding the unique characteristics of different processing methods can help organizations make informed decisions when incorporating ChatGPT for anomaly detection.
Hi Kerry, thanks for sharing this insightful piece. I'm curious, how does ChatGPT perform compared to traditional anomaly detection methods?
Hi Bob! Compared to traditional methods, ChatGPT offers more flexibility in handling unstructured or ambiguous data. However, it may face challenges with identifying rare anomalies if they don't align with the training data.
Thanks for the clarification, Kerry. I can see the advantages of leveraging ChatGPT's flexibility in anomaly detection. Are there specific domains where ChatGPT outperforms traditional methods?
Hi Bob! ChatGPT tends to excel in domains with a significant amount of unstructured or textual data. It can effectively capture anomalies hidden within such data that traditional methods might struggle with.
Thanks, Kerry! It seems ChatGPT has its sweet spots. One last question, what are the key factors to consider when deciding between ChatGPT and traditional methods for anomaly detection?
Bob, some key factors to consider are the nature of the data (structured vs. unstructured), the need for interpretability, the availability of labeled data, and the desired trade-offs between flexibility and performance. Evaluating these factors can guide the choice between ChatGPT and traditional methods.
Kerry, great article! As you mentioned, data bias is a potential limitation. How do you address bias in the training data to ensure fair and unbiased anomaly detection?
John, addressing bias requires a careful selection and curation of training data. We aim to include a diverse range of samples to avoid under-representing specific groups. Additionally, conducting regular fairness audits and involving domain experts can help mitigate bias in anomaly detection.
That's great to hear, Kerry. Fairness and unbiasedness are crucial in any AI application. Keep up the great work in advancing anomaly detection!
Kerry, this is amazing work! I can definitely see the potential of using ChatGPT for anomaly detection. Are there any limitations or challenges in implementing this approach?
Thank you, Emily! One challenge is data bias, as ChatGPT's predictions heavily rely on the patterns in the training data. Another limitation is the potential for false positives if the training data has inadequate anomaly examples.
Thanks for addressing my question, Kerry! I can see how data bias and false positives can be challenges. How do you mitigate these issues and improve ChatGPT's performance?
Emily, to mitigate data bias, we carefully curate and diversify training data to avoid over-representation or under-representation of certain anomalies. Regular model updates based on user feedback and continuous monitoring help improve its performance over time.
Hi Kerry, great article! Is ChatGPT suitable for real-time anomaly detection in streaming data, or is it more suited for batch processing?
Stephanie, while ChatGPT can handle streaming data, it's essential to ensure sufficient computational resources and low latency requirements for real-time processing. For batch processing, it can be highly efficient and effective.
Interesting article, Kerry! Are there any specific deployment considerations or prerequisites to utilize ChatGPT for anomaly detection in a production environment?
Thank you, Amy! Deploying ChatGPT for production anomaly detection entails ensuring infrastructure scalability, integrating with data pipelines, and carefully managing any privacy or security concerns related to the data being processed.
Thanks for clarifying, Kerry. It's interesting to know that ChatGPT can handle both streaming and batch data. This opens up possibilities for real-time anomaly detection across different use cases.
Exactly, Stephanie! The ability to adapt to different data processing scenarios makes ChatGPT a versatile tool for real-time anomaly detection in various industries.
I couldn't agree more, Stephanie! ChatGPT's capabilities in real-time anomaly detection have the potential to significantly improve data analysis efficiency and accuracy.
Kerry, the real-world applications you mentioned are quite diverse. Could you provide any specific success stories or use cases where ChatGPT has been particularly effective in anomaly detection?
Emily, one notable success story involves the healthcare domain where ChatGPT helped identify anomalies in patients' electronic health records, leading to early detection of critical health conditions. Additionally, in financial fraud detection, ChatGPT effectively flagged previously unknown patterns of fraudulent transactions.
Those are impressive examples, Kerry! ChatGPT's potential impact on critical areas like healthcare and finance is remarkable. It's clear that innovation in anomaly detection methods has substantial practical value.
Absolutely, Emily! The ability to uncover hidden anomalies through natural language processing techniques can greatly benefit society, offering early intervention and improved safety measures.
Hi Kerry, thanks for the article! I'm wondering, how does ChatGPT adapt to new anomalies that were not part of the training data?
Charlie, ChatGPT can adapt to new anomalies by incorporating user feedback and updates. When encountering previously unseen anomalies, continuous retraining using the most recent anomaly examples helps improve its ability to detect and classify them accurately.
Kerry, it's fascinating to see how ChatGPT can contribute to early detection in healthcare and finance. Do you anticipate any future developments or enhancements to ChatGPT specifically for anomaly detection?
Sarah, we're continually working on enhancing ChatGPT's anomaly detection capabilities. Future developments may focus on improved interpretability, more effective handling of rare anomalies, and techniques to reduce false positives. The goal is to make it a more robust and reliable tool for anomaly detection tasks.
That sounds promising, Kerry. I'm excited to witness the evolution of ChatGPT in anomaly detection. Keep up the great work, and thank you for sharing your expertise!