Advancing Content Recommendation in Social Network Analysis: Leveraging ChatGPT for Enhanced Personalization
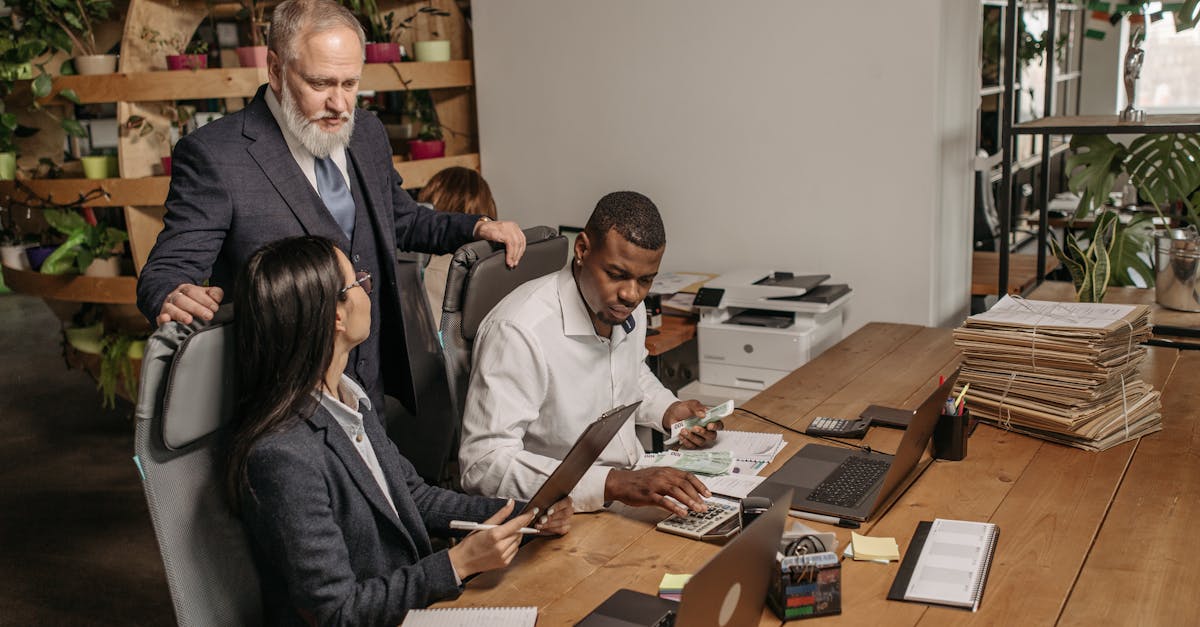
With the rapid growth of social media platforms and the ever-increasing amount of digital content available, it has become challenging for users to discover relevant and personalized information. To address this issue, social network analysis (SNA) has emerged as a powerful technology in the field of content recommendation.
Understanding Social Network Analysis
Social network analysis is a technique that aims to analyze and understand social structures and relationships between individuals or entities within a network. It involves studying the patterns of interactions and communication between network nodes, such as users on a social networking platform.
While SNA was initially developed for sociological research, it has found practical applications in various fields, including content recommendation systems. By leveraging the rich data generated by user behavior and interactions on social networks, SNA can provide valuable insights for personalized content recommendations.
Delivering Personalized Recommendations
Traditional content recommendation systems primarily rely on collaborative filtering or content-based algorithms. However, these approaches often fail to capture the specific interests and preferences of individual users, leading to less accurate recommendations.
By incorporating social network analysis into the recommendation process, algorithms can utilize additional information about a user's social connections, their online activities, and the content they engage with. This enables the system to identify patterns and similarities between users, enhancing the accuracy and relevancy of content recommendations.
For example, suppose User A is connected to Users B, C, and D on a social networking platform. User A frequently interacts with and shares posts from User C, indicating a strong interest in the content produced by User C. By leveraging SNA techniques, the content recommendation system can identify this relationship and suggest similar content to User A based on the preferences of User C.
Improving User Experience
Implementing social network analysis in content recommendation systems can significantly improve the overall user experience by reducing the information overload and helping users discover relevant content. When users are presented with personalized recommendations that align with their interests and preferences, they are more likely to engage with the content, fostering a positive user experience and increasing user satisfaction.
By combining SNA with other machine learning and artificial intelligence techniques, content recommendation systems can continuously learn from user feedback and adapt to evolving user interests. This dynamic approach ensures that the recommendations remain relevant and up-to-date as user preferences shift.
The Future of Content Recommendation
Social network analysis has revolutionized content recommendation systems by providing a more personalized and accurate approach to content discovery. As the amount of digital content continues to grow, the need for sophisticated recommendation systems becomes even more crucial.
With advancements in technology and the constant evolution of social networks, we can expect social network analysis to play an increasingly prominent role in content recommendation systems. By leveraging the power of social connections and user behavior, we can ensure that users are presented with the most relevant and engaging content, ultimately enhancing their overall online experience.
Conclusion
Social network analysis has proven to be a game-changer in the field of content recommendation. By leveraging the rich data generated by user interactions on social networks, SNA techniques enable personalized and relevant content suggestions. As technology continues to advance, we can expect social network analysis to shape the future of content recommendation, providing users with a more tailored online experience.
Comments:
Thank you all for your comments on my article. I appreciate your insights!
Great article, Jeff! I found your exploration of leveraging ChatGPT for enhanced personalization in social network analysis quite fascinating.
Thank you, Mark! I'm glad you found it interesting. How do you think the use of ChatGPT can impact content recommendation?
Hi Jeff, your article provided a clear overview of leveraging ChatGPT. I believe it has the potential to greatly improve personalized content recommendations on social networks.
Thank you, Emily! Indeed, leveraging ChatGPT's capabilities can enhance the accuracy and relevance of content recommendations. It opens up new possibilities for personalized user experiences.
ChatGPT seems like a powerful tool for personalization. However, do you think there are any ethical concerns around data privacy and manipulation?
That's a great point, Sarah. Ethical concerns are definitely important when it comes to leveraging user data. Proper safeguards and transparency should be in place to protect privacy and ensure responsible use of technology.
I agree, Jeff. While the potential benefits are exciting, we must ensure that users' privacy and autonomy are respected throughout the process.
Absolutely, Alex. Striking the right balance between personalization and user privacy is crucial. It requires a thoughtful approach and adherence to ethical guidelines.
Jeff, I enjoyed reading your article. The use of ChatGPT for enhanced personalization could revolutionize how users engage with content on social networks.
Thank you, Olivia! It's exciting to explore the potential impact of ChatGPT in improving user engagement and satisfaction on social networks. The future holds interesting possibilities.
Hey Jeff, thanks for sharing your insights. I believe leveraging ChatGPT for enhanced personalization can help overcome the information overload we often face on social networks.
You're welcome, David! I agree with you. By utilizing ChatGPT, we can alleviate the overwhelming amount of content and provide users with relevant recommendations tailored to their preferences.
Great article, Jeff! I'm curious how the performance of ChatGPT compares to other recommendation algorithms in social network analysis?
Thank you, Laura! ChatGPT performs well in terms of its natural language processing capabilities. However, it's important to note that its effectiveness as a recommendation algorithm may vary depending on the specific use case and dataset.
Jeff, do you think ChatGPT can be utilized beyond content recommendation in social network analysis? Any other potential applications?
Absolutely, Robert! ChatGPT has applications beyond content recommendation. It can be used for chatbots, virtual assistants, customer support, and even creative writing. Its versatility makes it a powerful tool in various domains.
Hi Jeff! I have concerns about potential biases ChatGPT might introduce into content recommendations. How can we address this issue?
Hi Sophia! Bias is indeed a critical concern. By adopting fairness-aware algorithms and diverse training data, we can mitigate biases and strive for more inclusive and balanced content recommendations.
Jeff, your article shed light on the significant impact ChatGPT could have on enhancing personalized user experiences. I'm excited to see its integration in social networks.
Thank you, Daniel! It's encouraging to see the potential future advancements in personalized user experiences with the integration of ChatGPT. The possibilities are indeed exciting!
Great read, Jeff! Leveraging ChatGPT in social network analysis can provide a more tailored and engaging user experience. Looking forward to its implementation.
Thank you, Lily! I'm glad you found it valuable. The implementation of ChatGPT can indeed revolutionize the user experience on social networks by providing personalized and engaging content.
Jeff, I'm curious about the challenges in implementing ChatGPT for content recommendation. Could you elaborate on that?
Sure, Steven! Implementing ChatGPT for content recommendation entails challenges such as managing large-scale data, training models, addressing biases, and ensuring real-time responses. These are areas that require careful consideration and optimization.
Hi Jeff! I think leveraging ChatGPT for enhanced personalization is exciting. How do you envision its integration into existing social media platforms?
Hi Melissa! Integrating ChatGPT into existing social media platforms would involve developing interfaces and APIs that enable seamless interaction with the models. This integration can enhance the user experience and provide more relevant recommendations within the platform.
Jeff, what are the potential limitations of leveraging ChatGPT for content recommendation, if any?
Good question, William. ChatGPT's limitations include potential biases, sensitivity to input phrasing, generating plausible but incorrect responses, and dependence on training data. These limitations need to be addressed for optimal utilization.
Jeff, I think leveraging ChatGPT can significantly improve user engagement. How do you foresee its impact on content creators?
Hi Chloe! ChatGPT can indeed enhance user engagement, which can have a positive impact on content creators. It can promote better discovery of their work, increase reach, and provide more avenues for user interaction and feedback.
Jeff, your article is thought-provoking. What kind of advancements do you envision in ChatGPT or similar models for social network analysis?
Thank you, Grace! I believe we'll see advancements in leveraging even larger pre-trained models, enhanced handling of context, and improved understanding of nuanced language interactions. These developments will lead to more accurate and context-aware content recommendations.
Jeff, your article made me reflect on the potential impact of ChatGPT on the echo chamber effect in social networks. How can we ensure diversity and avoid reinforcing existing biases?
Hi Ethan! Ensuring diversity in recommendations is crucial for avoiding echo chambers. By considering diverse training data, promoting inclusive content, and giving users control over their preferences, we can mitigate the reinforcement of biases and foster a more balanced information ecosystem.
Jeff, I appreciate your insights. Can you briefly explain how ChatGPT learns from user interactions and adapts to improve recommendations?
Certainly, Nathan! ChatGPT can learn from user interactions through reinforcement learning, receiving feedback on generated responses. This feedback helps it adapt over time, improving the quality and relevance of recommendations based on user preferences and engagement patterns.
Hi Jeff! I'm curious about the scalability of leveraging ChatGPT for content recommendation. How does it handle large user bases and high request volumes?
Hi Emma! ChatGPT's scalability can be achieved through efficient distributed systems and infrastructure. By parallelizing and optimizing computations, it can handle large user bases and high request volumes while maintaining real-time responsiveness.
Jeff, as content recommendation becomes more personalized, how can we strike a balance between novelty and preserving users' interests?
Finding the balance between novelty and preservation of users' interests is a challenge. By combining serendipity with user feedback and preferences, we can provide recommendations that introduce new content while still aligning with users' interests, ensuring a rewarding and engaging experience.
Jeff, your article highlights the promise of leveraging ChatGPT for improved social network analysis. What are the potential implications for targeted advertising?
Hi Henry! Leveraging ChatGPT can indeed have implications for targeted advertising. By understanding users' preferences and interests more accurately, it can enable more relevant and personalized advertisements. However, striking the right balance is crucial to avoid intrusiveness and respect user privacy preferences.
Jeff, I enjoyed your article. How do you foresee the integration of ChatGPT affecting the role of human moderators in social networks?
Thank you, Liam! The integration of ChatGPT may impact the role of human moderators. They can focus on higher-level judgment, policy enforcement, and ensuring ethical considerations. ChatGPT can assist by filtering out less relevant content, but human moderation remains crucial for maintaining a safe and inclusive environment.
Jeff, what are the potential challenges in implementing the feedback loop for learning and optimizing recommendations with ChatGPT in social network analysis?
Good question, Isabella. Implementing the feedback loop can have challenges such as managing the volume of feedback, developing effective reward models, and addressing potential biases in user interactions. It requires a continuous learning and improvement process to optimize recommendations while maintaining user satisfaction.
Jeff, how do you envision the future of social network analysis with the integration of machine learning techniques like ChatGPT?
Hi Aiden! The integration of machine learning techniques like ChatGPT holds great potential for the future of social network analysis. We can expect more personalized, engaging, and relevant user experiences, as well as improved content discovery and information sharing. The combination of human intelligence and AI capabilities will shape the landscape of social networks.
Jeff, your article emphasized the importance of content personalization. How can we ensure transparency and user understanding of the personalization algorithms used?
Transparency is key, Emma. Social networks should provide clear explanations of the personalization algorithms used, empowering users with an understanding of how their content recommendations are generated. By enhancing transparency and providing control, we can foster trust and enable users to make informed choices about their online experiences.