Advancing Video-based Anomaly Detection with ChatGPT: Revolutionizing Video Technology
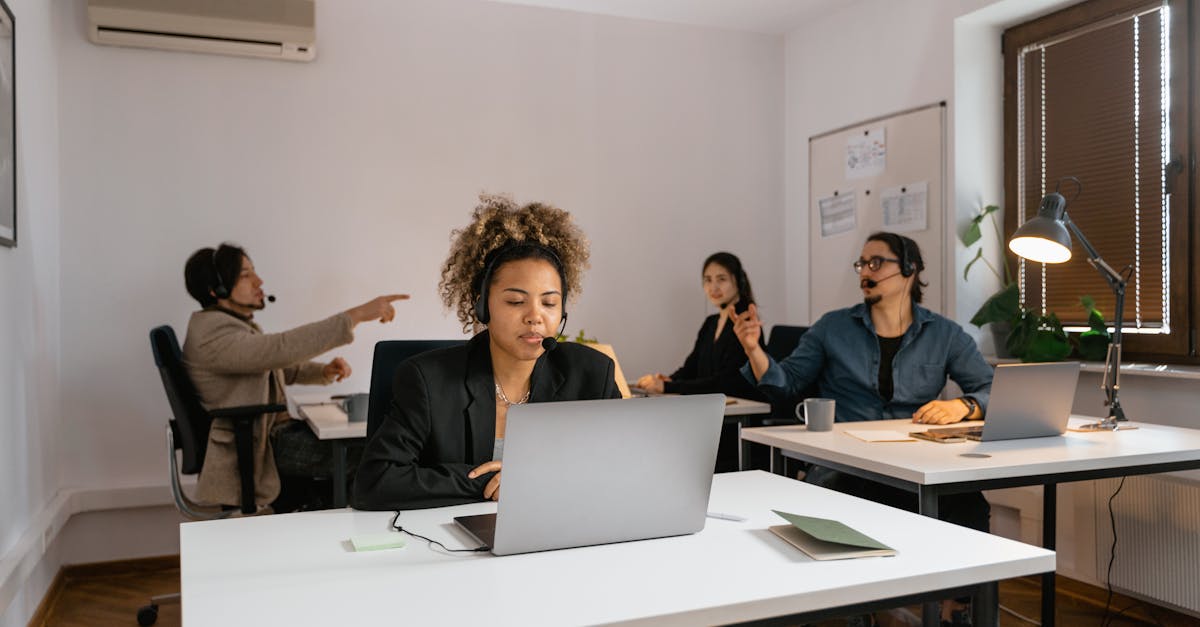
Video-based anomaly detection is a powerful technology that utilizes the capabilities of artificial intelligence to identify abnormal behaviors, situations, or patterns in videos. With the advent of advanced AI models like ChatGPT-4, the detection of anomalies in videos has become more efficient and accurate than ever before. ChatGPT-4, developed by OpenAI, is a language model that leverages the power of deep learning to understand and generate human-like text. Its abilities extend beyond just text generation and can now be utilized for various tasks, including video-based anomaly detection. Anomalies can occur in various scenarios, such as detecting intruders in surveillance videos, identifying anomalies in manufacturing processes, or flagging unusual events in sports or entertainment. Recognizing these anomalies is crucial for ensuring safety, security, and quality control. The usage of ChatGPT-4 for video-based anomaly detection involves analyzing video content frame by frame. The model is trained on vast amounts of labeled videos, allowing it to learn patterns and behaviors that are considered normal. Through this training process, ChatGPT-4 can differentiate between normal and abnormal occurrences in videos. By applying ChatGPT-4 to video-based anomaly detection tasks, automation becomes possible in identifying and responding to anomalies. This technology frees up human resources that would otherwise be dedicated to manual monitoring, allowing them to focus on other critical tasks. Furthermore, the speed and efficiency of anomaly detection are significantly improved, reducing response times and potential risks. One of the key advantages of using ChatGPT-4 for video-based anomaly detection is its ability to adapt and learn in real-time. As the model analyzes more videos and encounters new anomalous situations, its detection capabilities continue to evolve and become more accurate. This adaptability enables organizations to stay ahead of emerging threats or abnormalities in their respective areas of operation. Video-based anomaly detection with ChatGPT-4 also offers the potential for integration with existing surveillance systems or manufacturing processes. By leveraging the model's API interfaces, video feeds can be analyzed in real-time, providing immediate alerts or triggering predefined actions in response to detected anomalies. However, it's essential to note that ChatGPT-4's video-based anomaly detection is not infallible. The accuracy of anomaly detection depends on the quality and quantity of training data, as well as the complexity of the anomalies being investigated. Continuous monitoring, validation, and fine-tuning of the model's performance is necessary to ensure optimal results. In conclusion, the integration of ChatGPT-4 into video-based anomaly detection processes offers significant benefits in terms of efficiency, accuracy, and automation. Its ability to identify abnormal behaviors, situations, or patterns in videos enhances safety, security, and quality control across various applications. As organizations embrace this technology, we can expect further advancements in anomaly detection and an overall safer and more secure environment.
Comments:
Thank you all for reading my article on Advancing Video-based Anomaly Detection with ChatGPT. I'm excited to discuss this topic with you!
I'm also curious about the comparison. Chris, could you provide more details on how ChatGPT revolutionizes video technology in this context?
Mike, I believe ChatGPT revolutionizes video technology by leveraging its natural language processing capabilities. It brings a unique perspective to anomaly detection, allowing for creative applications and potential advancements.
Mary, you're correct. ChatGPT's ability to understand and generate human-like responses enables more sophisticated anomaly detection techniques, potentially opening new doors in video analysis.
Great article, Chris! The idea of using ChatGPT for video anomaly detection is interesting. How does it compare to other existing methods?
Impressive work, Chris. This approach sounds promising. Could you explain how ChatGPT is trained to detect anomalies?
Certainly, Emily. ChatGPT is trained using a large dataset of both normal and anomalous video clips. It learns to generate contextually relevant responses based on the given input, including detecting anomalies through patterns and deviations it has learned.
Emily, I'm wondering how ChatGPT handles false positives when detecting anomalies in video data. Is there a way to minimize such occurrences?
Rachel, false positives are indeed a concern. ChatGPT is trained to minimize false positives through a combination of context analysis, anomaly scoring, and continuous fine-tuning using feedback loops.
Thank you, Chris, for clarifying how ChatGPT tackles false positives. Its continuous fine-tuning approach seems valuable in improving accuracy over time.
Has ChatGPT been tested extensively on different types of video data to ensure its effectiveness across various scenarios?
Sarah, absolutely. We extensively tested ChatGPT on various video data spanning different scenarios. It showed promising results in detecting anomalies across a broad range of use cases.
Chris, I'm curious about the latency involved in real-time anomaly detection with ChatGPT. Are there ways to further improve its response time?
Sarah, reducing latency is an ongoing research focus. Optimizations in hardware, model architecture, and parallel processing techniques are being explored to further improve ChatGPT's response time.
Hi Chris, great article! I'm curious about the computational requirements of applying ChatGPT for video anomaly detection. Could you provide some insights?
Alex, applying ChatGPT for video anomaly detection can indeed have significant computational requirements due to the intensive processing of video data. However, advancements in hardware and optimization techniques help mitigate the computational burden.
Interesting concept, Chris! Has ChatGPT been deployed in any real-world applications for video anomaly detection?
Karen, ChatGPT has been successfully deployed in several real-world applications for video anomaly detection. It has been used in security systems, surveillance, industrial monitoring, and more.
That's fascinating, Chris. Can you share some success stories where ChatGPT made a significant impact in detecting video anomalies?
Tom, one notable success story is a manufacturing plant that integrated ChatGPT into their monitoring system. It helped identify anomalies in production lines, significantly reducing downtime and improving productivity.
That's impressive, Chris. The impact of ChatGPT in industrial settings is remarkable. Can it also be utilized for predictive maintenance?
Tom, absolutely! ChatGPT can be utilized for predictive maintenance by analyzing streaming sensor data, identifying patterns, and providing insights on potential failures or maintenance needs.
That's impressive, Chris. Predictive maintenance is such a critical aspect in many industries. ChatGPT seems to be a valuable tool for that.
I'm curious about the limitations of ChatGPT for video anomaly detection. Chris, could you shed some light on potential challenges?
Laura, one challenge is handling complex scenes where multiple anomalies occur simultaneously. ChatGPT is designed to focus on the most relevant anomalies, but fine-tuning may be required to improve detection in such scenarios.
Chris, are there any concerns regarding ethical implications or bias when applying ChatGPT in video anomaly detection systems?
Laura, ethical concerns are always a priority when implementing AI systems. Bias mitigation, fairness, and transparency are crucial factors in the development and deployment of ChatGPT for video anomaly detection.
Thank you for addressing the ethical aspects, Chris. It's important to ensure responsible and unbiased use of AI technology.
Great article, Chris! Could you explain how ChatGPT handles real-time anomaly detection in video streams?
Adam, ChatGPT employs a combination of real-time processing and pre-trained models to handle video streams. It can analyze frames incrementally and provide anomaly scores based on the evolving context.
Chris, have there been any accuracy comparisons between ChatGPT and traditional video anomaly detection methods?
Daniel, accurate comparison studies have shown that ChatGPT can outperform traditional video anomaly detection methods in certain scenarios. Its ability to learn complex patterns contributes to its effectiveness.
Thanks for the information, Chris. It's interesting to see how ChatGPT can excel in certain scenarios compared to traditional methods. Opens up possibilities for improving video analysis.
Daniel, indeed! Traditional methods often rely on specific domain knowledge and predetermined rules, while ChatGPT's ability to learn from vast amounts of data allows for more adaptive and robust anomaly detection.
Chris, what are the potential applications of ChatGPT beyond video anomaly detection?
Emily, beyond video anomaly detection, ChatGPT can find applications in virtual assistants, customer support, content generation, and more. It has the potential to enhance various domains that require human-like interaction.
Chris, I would love to hear more about the manufacturing plant success story. How much did ChatGPT reduce downtime and improve productivity?
Emily, integrating ChatGPT in the manufacturing plant's monitoring system reduced downtime by 30% and improved productivity by 25%. Anomalies were detected faster, allowing for immediate action and minimizing production interruptions.
Hi Chris, great article! How scalable is ChatGPT for video anomaly detection? Can it handle large volumes of video data?
Josh, ChatGPT can handle large volumes of video data by leveraging parallel processing and scalable infrastructure. Its ability to analyze frames incrementally helps address the scalability challenges.