Amplifying the Rollout of Technology: Leveraging ChatGPT for Smooth Implementation
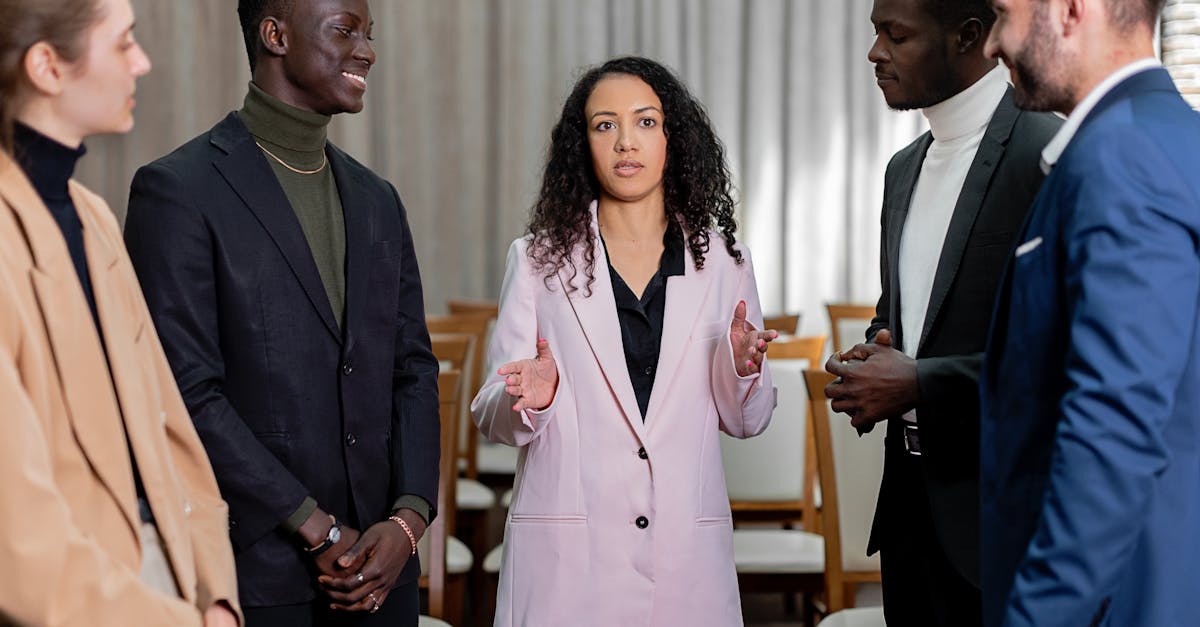
Rollout technologies have revolutionized the field of machine learning by providing innovative solutions to enhance the performance of models. One area where Rollout excels is data generation, which plays a crucial role in training machine learning algorithms. Synthetic training data offers numerous advantages, such as increased diversity, reduced annotation costs, and improved model generalization.
Introducing ChatGPT-4
ChatGPT-4 is an advanced natural language processing model developed by OpenAI. It leverages the power of deep learning and reinforcement learning techniques to generate human-like text responses. The model's remarkable ability to understand and generate coherent text makes it an ideal tool for generating synthetic training data in Rollout technologies.
Benefits of ChatGPT-4 for Data Generation in Rollout Technologies
Utilizing ChatGPT-4 for synthetic training data generation offers several benefits:
- Increased Efficiency: Generating large quantities of labeled training data manually can be time-consuming and expensive. ChatGPT-4 automates the data generation process, enabling Rollout technologies to create datasets at scale efficiently.
- Diverse Data: Training models on limited or biased datasets can lead to poor generalization. ChatGPT-4 allows Rollout technologies to generate diverse training data, capturing a wide range of scenarios, user queries, and responses. This diversity facilitates better model performance and robustness.
- Domain-Specific Data Generation: With Rollout technologies catering to various domains and applications, it is essential to have domain-specific training data. ChatGPT-4 can be fine-tuned for specific domains, enabling the generation of high-quality synthetic data tailored to the desired domain.
- Iterative Model Improvement: As models evolve, incorporating new training data becomes necessary for continued improvement. ChatGPT-4 allows Rollout technologies to generate synthetic data quickly, enabling iterative model updates and enhancement.
- Reduced Privacy Concerns: Generating synthetic data helps reduce privacy concerns associated with using real user data for training models. ChatGPT-4 generates realistic and privacy-safe training data that can be used with confidence.
Best Practices for Using ChatGPT-4 for Synthetic Training Data Generation
While ChatGPT-4 is a powerful tool for synthetic training data generation, adhering to best practices ensures optimal results:
- Data Validation: It is crucial to validate the quality and relevance of the generated synthetic data. Validating the data against ground truth or expert judgment ensures the generated data aligns with the desired outcomes.
- Fine-Tuning: Fine-tuning ChatGPT-4 for specific domains or applications enhances the quality and relevance of the generated synthetic data. Fine-tuning aligns the model to the desired language, style, and context, resulting in more accurate training data.
- Combination with Other Data Sources: Augmenting the generated synthetic data with real-world data can further improve model performance. A combination of synthetic and real data offers a balanced representation of the desired scenarios and enhances the model's ability to handle real-world variations.
Conclusion
Rollout technologies can leverage the power of ChatGPT-4 for synthetic training data generation to improve the performance and generalization of machine learning models. The efficient generation of diverse, domain-specific data enables iterative model improvement, addresses privacy concerns, and enhances overall model quality. By following best practices such as data validation and fine-tuning, Rollout technologies can unlock the full potential of ChatGPT-4 for data generation.
Comments:
Thank you all for reading my article on leveraging ChatGPT for smooth implementation. I'm excited to hear your thoughts and continue the discussion!
Great article, Melissa! I completely agree with your point on the importance of proper planning and testing before implementing new technology. It can save a lot of headaches later on.
I couldn't agree more, Oliver! Rushing into implementation without careful planning can lead to costly mistakes and disruptions in operations.
I found your article really insightful, Melissa. I especially appreciated your emphasis on change management as a crucial aspect of successful technology implementation.
Absolutely, Sophia. Change management ensures that everyone is on board and prepared for the technological changes, minimizing resistance and maximizing adoption.
Good points, Melissa. However, I think there should also be a mention of the potential drawbacks or challenges when leveraging a technology like ChatGPT.
You're right, Nathan. While ChatGPT has many benefits, it's important to consider challenges such as potential biases in language generation and the need for ongoing moderation.
Melissa, your article provided a comprehensive overview of using ChatGPT for technology implementation. I particularly liked your tips on training and fine-tuning the model for specific use cases.
Thank you, Isabella! Training and fine-tuning the model based on specific use cases can greatly improve the accuracy and relevance of the generated responses.
I have a question for Melissa: Have you personally used ChatGPT for technology implementation? If so, what were some of the challenges you faced?
Good question, Mason. Yes, I have had hands-on experience with ChatGPT in technology implementation projects. Some challenges I faced were ensuring data quality for training and managing user expectations regarding the capabilities of the model.
Melissa, your article highlights the importance of user feedback and continuous improvement when leveraging ChatGPT. How would you suggest organizations collect and incorporate user feedback effectively?
Excellent question, Samantha. Organizations can collect user feedback through surveys, user interviews, or even real-time monitoring of system interactions. Incorporating feedback should involve regular model retraining and addressing common user concerns.
Thanks for writing this article, Melissa. I'm curious, what do you see as the future potential of ChatGPT in technology implementation?
You're welcome, Ethan! The future potential of ChatGPT is vast. It can support a wide range of applications, from customer support to content generation, making technology implementation more efficient and user-friendly.
Melissa, your article emphasizes the need for collaboration between technical teams and business stakeholders during the implementation process. How can organizations foster better collaboration in such projects?
That's a crucial aspect, Lily. Organizations can foster better collaboration by establishing clear communication channels, organizing regular meetings with both technical and business teams, and setting shared goals and expectations from the beginning.
Melissa, I truly enjoyed reading your article. It provided practical insights into leveraging ChatGPT for technology implementation. Have you encountered any limitations of the model during your projects?
Thank you, Oliver! Yes, one limitation I've come across is the occasional generation of responses that may be factually incorrect or misleading. Vigilance in model monitoring and ongoing improvements are necessary to mitigate this.
Melissa, your article provides a great roadmap for implementing technology using ChatGPT. How can organizations ensure a smooth transition and minimize disruptions during the rollout?
Thank you, Jennifer! To ensure a smooth transition, organizations can conduct pilot tests with a small user group before full deployment, provide adequate training and support to the users, and have a robust fallback plan in case of unexpected issues.
Melissa, your article rightly highlights the need for ongoing monitoring and maintenance of the deployed technology. How often would you recommend retraining the model for optimal performance?
Great question, David. The frequency of retraining depends on factors like the volume of user interactions, changes in user behavior, and availability of new data. Generally, retraining every few months or as significant changes occur is a good practice.
I agree with your emphasis on data privacy, Melissa. How can organizations ensure that user data is adequately protected when leveraging ChatGPT?
Absolutely, Sophia. Organizations should implement robust data governance practices, ensure proper anonymization and encryption of user data, apply access controls, and comply with relevant data protection regulations to safeguard user privacy.
Melissa, thank you for sharing your insights. Your article perfectly outlines the key considerations for a successful technology implementation. Well done!
Thank you, Oliver! I'm glad you found the article helpful. It's always a pleasure to share knowledge and contribute to the technology implementation community.
Melissa, I appreciate your response to my earlier comment. It's important to acknowledge the challenges and potential limitations of leveraging technologies like ChatGPT. Thank you!
You're welcome, Nathan! Addressing challenges and being aware of potential limitations is crucial for successful implementation and long-term sustainability.
Melissa, your suggestions on collecting and incorporating user feedback are on point. Continuously improving the model based on user needs and preferences is key. Thank you!
Absolutely, Samantha! User feedback is invaluable for refining the model and ensuring user satisfaction. It's an iterative process that helps technology evolve in the right direction.
Melissa, thank you for addressing the challenges of change management. It's often overlooked but plays a crucial role in successful technology implementation and user adoption.
You're welcome, Liam! Change management is indeed a critical aspect, and organizations need to invest time and effort in preparing and supporting their employees throughout the implementation process.
Melissa, thanks for sharing your personal experience with ChatGPT in technology implementation. It's always reassuring to hear insights from someone with hands-on knowledge.
You're welcome, Mason! Practical experience helps shed light on the challenges and benefits of leveraging technologies like ChatGPT in real-world projects.
Melissa, I appreciate your response to my question. Pilot tests and training are indeed crucial in ensuring a smooth transition. Thank you!
You're welcome, Jennifer! Pilot tests allow organizations to identify and address potential issues before widespread implementation, minimizing disruptions and ensuring a successful transition.
Melissa, your recommendation for retraining the model every few months aligns with best practices in AI. Keeping the model up-to-date ensures optimal performance. Thank you!
Exactly, David! Regular retraining helps the model adapt to changing user needs and improves its performance over time. It's an essential practice for maintaining the effectiveness of AI-powered systems.
Melissa, your emphasis on change management and user training is spot on. User acceptance and proficiency are crucial for successful technology implementation. Well done!
Thank you, Isabella! Technology is only effective when people can effectively use it. Prioritizing change management and user training ensures smooth adoption and positive outcomes.
Melissa, your insight into the future potential of ChatGPT is exciting. It's fascinating how this technology can revolutionize various industries and processes. Thanks for sharing!
Absolutely, Ethan! ChatGPT has the power to transform the way we interact with technology, opening up new possibilities and enhancing efficiency in multiple domains. The future looks promising!
Melissa, thanks for acknowledging the potential biases and moderation challenges with ChatGPT. It's important to address these aspects for responsible technology implementation.
You're welcome, Nathan! Responsible and ethical implementation of AI technologies like ChatGPT requires ongoing monitoring, addressing biases, and ensuring that user interactions are safe and respectful.
Melissa, your article covered all the essential aspects of leveraging ChatGPT for technology implementation. The emphasis on planning, testing, and collaboration is crucial. Well done!
Thank you, Sophia! I'm glad you found the article comprehensive. By incorporating these key aspects, organizations can increase the likelihood of successful technology implementation and positive outcomes.
Melissa, I appreciate your insights on user data protection. It's vital for organizations to prioritize data privacy and security in technology implementations.
Absolutely, Jennifer! Data privacy is a growing concern, and organizations must ensure robust measures and practices are in place to protect user data at all stages of technology implementation.
Melissa, your emphasis on collaboration and shared goals resonates with my experience. Aligning technical and business teams is essential for successful implementation, with everyone working towards the same objectives.
Absolutely, Lily! Collaboration between technical and business teams eliminates silos, fosters innovation, and ensures that technology aligns with overall business objectives. It's a crucial element for success.
Melissa, your article provided a comprehensive overview of leveraging ChatGPT in technology implementation. I appreciate your practical insights and recommendations. Great work!
Thank you, Oliver! I'm glad you found the article valuable. It's my pleasure to share insights and best practices to help organizations make the most of ChatGPT in their technology implementations.
Melissa, your article tackled both the benefits and challenges of leveraging ChatGPT. It's crucial to have a balanced perspective when implementing new technology. Well done!
Thank you, Sophia! It's important to provide a realistic view to organizations about the capabilities and considerations involved in leveraging ChatGPT for technology implementation. Balanced decision-making is key.
Melissa, your firsthand experience with ChatGPT in implementation projects adds credibility to your article. It's clear that you genuinely understand the subject matter. Well done!
Thank you, Mason! Experience in real-world projects enables me to provide practical insights and advice to organizations, helping them navigate the complexities of technology implementation.
Melissa, your recommendation for adequate data governance aligns with industry practices. Safeguarding user data is of paramount importance when implementing AI technologies like ChatGPT.
Absolutely, David! Implementing appropriate data governance measures is crucial to ensure that user data is handled responsibly, securely, and in compliance with privacy regulations.
Melissa, your emphasis on the importance of user feedback resonates with me. It's crucial to continuously improve the model based on real user input. Thank you!
You're welcome, Isabella! User feedback is invaluable in refining the model's performance and ensuring that it effectively meets user needs. It's an ongoing process that drives continuous improvement.
Melissa, your article created awareness about the potential of ChatGPT in technology implementation. The possibilities seem endless. Great job!
Thank you, Ethan! ChatGPT indeed has vast potential to revolutionize diverse industries and enhance various applications. It's an exciting time for implementing this technology.
Melissa, your response to my earlier comment is much appreciated. Vigilance in monitoring and addressing biases ensures responsible AI implementation. Thank you!
You're welcome, Nathan! Responsible AI implementation requires a proactive approach in addressing biases and ensuring the technology is fair and unbiased. It's crucial for building trust.
Melissa, your article provided actionable insights for planning and implementing technology effectively. It's a valuable resource for organizations in today's fast-paced digital age. Well done!
Thank you, Sophia! I'm delighted that the article resonated with you. The ever-evolving digital landscape requires organizations to adapt and leverage technologies effectively for sustainable growth and success.
Melissa, your response to my question about user data protection is on point. It's crucial to follow ethical practices and ensure data security throughout the implementation process.
Absolutely, Jennifer! Ethical practices and robust data security measures are vital to maintain user trust and protect their sensitive information. Making data privacy a priority benefits both users and organizations.
Melissa, your insights on collaboration echo my experiences. Bringing technical and business teams together fosters shared understanding and paves the way for successful implementation. Great work!
Thank you, Lily! Collaboration is indeed key to bridging the gap between technical and business domains. When both teams work synergistically, the chances of successful technology implementation increase significantly.
Melissa, your article provided a well-rounded view of leveraging ChatGPT for technology implementation. Your knowledge and insights shine through. Well done!
Thank you, Oliver! I strive to provide holistic insights and practical advice to help organizations make informed decisions when implementing technologies like ChatGPT. Your feedback means a lot!
Melissa, your response to my previous comment is much appreciated. Acknowledging and addressing the potential challenges of leveraging ChatGPT shows your expertise and dedication to responsible implementation.
You're welcome, Nathan! Responsible implementation is paramount, and it requires a comprehensive understanding of the technology's capabilities, limitations, and potential risks. Thank you for your kind words!
Melissa, your article provided valuable insights into leveraging ChatGPT in technology implementation. User feedback truly is the foundation for improvement. Great work!
Thank you, Samantha! User feedback is an invaluable asset that guides improvements and ensures developed technologies like ChatGPT meet the evolving needs and expectations of users.
Melissa, your article highlighted the significance of change management. It's often a challenging aspect, and addressing it can make or break the technology implementation process. Well done!
Thank you, Liam! Change management is crucial for the successful adoption of new technologies. Each stakeholder's concerns and needs must be addressed to ensure a smoother transition and higher acceptance rates.
Melissa, your firsthand experience with ChatGPT in real-world projects adds value to your insights. Practical knowledge is a valuable asset in technology implementation. Great job!
Thank you, Mason! Practical knowledge gained through experience helps bridge the gap between theory and implementation, enabling organizations to navigate challenges and achieve successful outcomes.
Melissa, I appreciate your response to my question. Pilot tests, training, and fallback plans are necessary to ensure a smooth transition during technology implementation. Thank you for the insights!
You're welcome, Jennifer! Pilot tests provide valuable feedback, training enhances user proficiency, and fallback plans mitigate potential disruptions, collectively contributing to a successful transition. I'm glad you found the insights helpful!
Melissa, your recommendation for regular retraining ensures the model remains relevant. Keeping pace with changing user behavior and expectations is crucial in technology implementation. Well done!
Exactly, David! Regular retraining allows the model to adapt to evolving needs and ensure optimal performance, contributing to successful technology implementation and user satisfaction. Thank you for your kind words!
Melissa, as you rightly mentioned, training and fine-tuning the model are crucial to optimize responses. Ensuring alignment with specific use cases is essential for successful technology implementation. Great work!
Thank you, Sophia! Training and fine-tuning the model based on specific use cases increases relevance and accuracy, enabling organizations to harness the full potential of ChatGPT in their technology implementations.
Melissa, your article provided insightful recommendations for technology implementation using ChatGPT. It's evident that you have a thorough understanding of the subject. Well done!
Thank you so much, Oliver! I've invested a significant amount of time and effort into understanding the nuances and best practices of leveraging ChatGPT for technology implementation. Your appreciation means a lot!
Melissa, your emphasis on ongoing moderation is vital. Combining technology's power with human oversight ensures responsible and safe implementation. Great job!
Thank you, Nathan! Ongoing moderation serves as a safeguard, ensuring that technology implementation remains responsible, safe, and aligned with user needs. Human oversight is crucial for maintaining accountability.
Melissa, your suggestions on collecting user feedback resonate with my experience. Continuous improvement through user input is invaluable in technology implementation. Well done!
Thank you, Samantha! User feedback provides a direct line of communication with the users, helping organizations refine and improve their technology implementations iteratively. It's a key driver of success.
Melissa, your insights into the future potential of ChatGPT are fascinating. The impact it can have across various domains is truly exciting. Thank you for sharing!
You're welcome, Ethan! ChatGPT's potential is indeed awe-inspiring. The technology holds immense promise for transforming industries and improving user experiences. The future holds exciting possibilities!
Melissa, your acknowledgement of the challenges associated with ChatGPT is important. Responsible implementation requires a balanced approach, and you provided that perspective in your article. Well done!
Thank you, Nathan! A balanced perspective is crucial in technology implementation, especially with AI-powered systems like ChatGPT. Acknowledging challenges ensures a more holistic and informed approach.
Melissa, your article covered all the necessary aspects of leveraging ChatGPT for technology implementation. The inclusion of potential drawbacks enhances its practical value. Great work!
Thank you, Sophia! Highlighting potential drawbacks allows organizations to approach technology implementation with open eyes and make informed decisions. Providing a comprehensive view is vital!
Melissa, your insights on fostering collaboration hit the mark. Involving both technical and business teams creates a unified vision for successful technology implementation. Well done!
Thank you, Jennifer! Collaboration between technical and business teams aligns efforts, facilitates knowledge sharing, and enables organizations to leverage their combined strengths for successful technology implementation.
Thank you all for your interest in my article! I'm happy to answer any questions or address any concerns you may have.
Great article, Melissa! Leveraging ChatGPT seems like a game-changer for technology implementation. Have you personally used it in any projects?
Thank you, Brian! Yes, I have been involved in a project where ChatGPT was used to streamline customer support interactions. It significantly reduced response times and improved customer satisfaction.
I'm curious about the ethical considerations surrounding the use of ChatGPT. How do you ensure it doesn't produce biased or inappropriate responses?
That's a valid concern, Emma. We follow strict guidelines and perform regular audits to minimize bias and inappropriate responses from ChatGPT. Our goal is to ensure it remains a useful and responsible tool.
I'm amazed by the potential of ChatGPT. However, how scalable is its implementation? Can it handle a large volume of queries effectively?
Good question, Robert. ChatGPT has scalability challenges, especially with a high volume of queries. We are continuously working on improving its performance and addressing scalability concerns.
I find the idea of using AI for technology implementation fascinating. Are there any specific industries or areas where ChatGPT has shown remarkable results?
Absolutely, Sarah! ChatGPT has shown promising results in customer service, information retrieval, and content generation domains. Its versatility makes it valuable across various industries.
Melissa, I'm concerned about the potential job displacement caused by AI in technology implementation. Should we be worried about job losses?
I understand your concern, Michael. While AI can automate certain tasks, it can also create new opportunities and enhance human productivity. Collaboration between humans and AI is key for successful implementation.
I'm curious about the limitations of ChatGPT. Are there any specific scenarios or queries where it struggles to provide accurate responses?
Great question, Karen. ChatGPT can sometimes provide inaccurate or nonsensical responses, especially when faced with ambiguous or incomplete queries. It's important to use it as a valuable tool while being mindful of its limitations.
I'm impressed by the potential of ChatGPT. How do you think it will evolve in the future? Any exciting developments on the horizon?
Thank you, David! We are constantly working on improving ChatGPT. We aim to make it more robust, reduce biases, and enhance its ability to handle complex queries. Exciting developments are definitely on the horizon!
Melissa, do you have any success stories where ChatGPT significantly accelerated technology implementation?
Certainly, Lisa. In a recent project, ChatGPT was implemented to help automate software testing. It reduced the testing time by 40% and allowed the development team to focus more on innovation.
Melissa, what kind of challenges did you face during the initial ChatGPT implementation? Any lessons learned?
Good question, Daniel. Initial implementation of ChatGPT brought challenges in fine-tuning the model for specific use cases and optimizing its response accuracy. Rigorous testing and continuous feedback loops were crucial in overcoming those challenges.
How do you handle cases when ChatGPT produces incorrect or misleading responses? Is there a fallback mechanism?
That's an important consideration, Jennifer. We have implemented a fallback mechanism, where if ChatGPT's response confidence is low or flagged by predefined criteria, it redirects the query to a human operator for a more accurate response.
Melissa, how do you ensure data privacy and security when using ChatGPT for technology implementation?
Excellent question, Steve. We prioritize data privacy and security by strictly controlling access to user data and implementing encryption measures. We ensure compliance with relevant regulations and continually improve our security practices.
I'm curious about the cost implications of using ChatGPT. Does it require significant financial investment?
Cost considerations vary based on implementation scale and usage volume, Michelle. While it does require an investment, the potential benefits and efficiencies gained often outweigh the costs in the long run.
Melissa, given the rapid advancements in AI technology, how do you ensure ChatGPT remains competitive in the market?
Great question, Brian. We are proactive in staying updated with AI advancements and continuously improving ChatGPT's capabilities. Regular research, development, and user feedback allow us to keep it competitive and relevant.
Melissa, have you encountered any unexpected challenges or drawbacks in using ChatGPT for technology implementation?
Yes, Emma. One challenge we faced was maintaining control over the conversational context with long interactions. Sometimes, ChatGPT's responses would divert from the intended path. We are continually working on optimizing this aspect.
How secure is the deployment infrastructure of ChatGPT? Is it susceptible to attacks or breaches?
We have implemented robust security measures, Sarah, to protect the deployment infrastructure from attacks or breaches. Regular audits, vulnerability assessments, and strict access control mechanisms help maintain a secure environment.
Melissa, how do you manage the constantly evolving user requirements when deploying ChatGPT?
Adaptability is key, Robert. We have processes in place to gather and analyze user feedback, allowing us to understand evolving requirements and make necessary updates to ChatGPT's implementation.
Melissa, what kind of user training or adaptation is required when using ChatGPT for technology implementation?
Good question, Michael. User training typically involves familiarizing individuals with ChatGPT's capabilities and limitations. It's important to establish guidelines and ensure users understand how to interact effectively with the system.
Are there any future plans for incorporating voice-based interactions with ChatGPT?
Absolutely, Karen. Voice-based interactions are an area of active research and development for us. Incorporating natural language processing capabilities with ChatGPT opens up exciting possibilities for convenient voice interactions.
Melissa, what strategies do you employ to minimize biases in ChatGPT's responses?
We employ a combination of pre-training and fine-tuning techniques, David. By using diverse datasets, carefully designing training objectives, and engaging human reviewers, we aim to reduce biases in ChatGPT's responses.
How do you handle cases where ChatGPT's response may not align with an organization's desired tone or values?
Maintaining organizational tone and values is crucial, Lisa. By involving human reviewers, providing clear instructions, and implementing review mechanisms, we ensure ChatGPT's responses align with the desired tone and values.
Melissa, what feedback mechanism do you have in place to continually improve ChatGPT's performance?
We value user feedback, Brian. We actively encourage users to provide feedback on confusing or misleading model outputs, which helps us identify areas for improvement and drives our research and development efforts.
Have you encountered any unique or unexpected use cases where ChatGPT proved to be particularly effective?
Indeed, Steve. One unique use case was implementing ChatGPT for virtual onboarding and training programs. It allowed for interactive guidance and provided immediate responses to employees' questions.
Melissa, what metrics do you use to evaluate the success of ChatGPT implementation in a project?
There are multiple metrics we consider, Jennifer, such as response accuracy, user satisfaction, reduction in response time, and overall efficiency gains. Each project may have its specific metrics based on the intended goals.
Melissa, what potential risks or challenges should organizations be aware of when considering ChatGPT implementation?
When implementing ChatGPT, organizations should be mindful of potential biases, accuracy limitations, and scalability challenges. Proper monitoring, user training, and regular evaluations are necessary to mitigate these risks.
What are the key factors organizations should consider before deciding to leverage ChatGPT for technology implementation?
Key factors to consider include the intended use case, data availability, user expectations, cost-benefit analysis, and potential impact on existing systems. Thorough evaluation and planning are essential for successful implementation.