Boosting Efficiency: ChatGPT Revolutionizes Demand Response in Technology
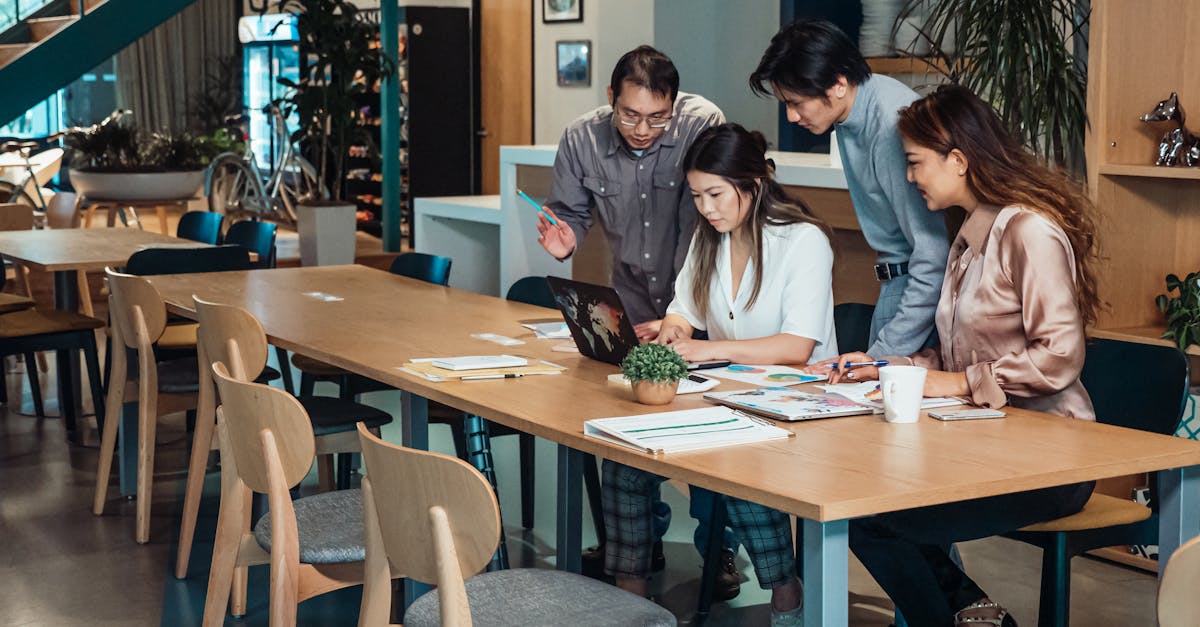
Demand Response (DR) is an essential technological innovation introduced in the energy industry to improve grid reliability and efficiency. Focused on altering the demand for power rather than adjusting the supply, DR encourages consumers to reduce their energy consumption during peak periods in response to time-based rates or other forms of financial incentives. It provides a valuable solution to the growing energy demands and the imperative to minimise the environmental footprint of the energy industry.
DR and Energy Consumption Forecasting
While DR has its advantages, the success of this model greatly depends on accurate energy consumption forecasting. Forecasting plays an integral role in the planning and operation of DR programmes. Without reliable forecasts of customers' reaction to price changes and their potential electrical usage, it would prove challenging to determine the effectiveness of the DR events.
Energy consumption forecasting is inherently a complex task due to several influencing elements like weather conditions, consumer behaviour, and price sensitivity. However, with the advent of more sophisticated and nuanced technologies, improved forecasting strategies are changing the landscape of DR.
ChatGPT-4 and Forecasting
Among these advancements, the capabilities of AI, in particular, ChatGPT-4, stand out for its promising implications for DR. As an advanced version of the GPT family developed by OpenAI, ChatGPT-4 can be programmed to understand context, draw from vast information pools, predict trends, and learn from its experience.
ChatGPT-4's programming basis of Machine Learning (ML) and Natural Language Processing (NLP) can predict energy usage patterns by processing past consumption data, weather predictions, pricing fluctuations, and other relevant factors. This newfound capacity to 'learn 'from patterns and remember information lets ChatGPT-4 generate more accurate energy consumption forecasts, which can, in turn, inform the optimisation of DR planning.
How it Works
ChatGPT-4 begins by collecting and analysing historical energy usage data, including the time, extent, and conditions of usage in the past. It matches this data against external factors, like weather conditions, holidays, working days, and other factors influencing energy usage patterns. This comprehensive analysis identifies patterns and provides a basis for predicting future consumption patterns.
Given the NLP capabilities, ChatGPT-4 can also analyse and predict how consumers will respond to DR price signal changes, enhancing the comprehensiveness and accuracy of the DR plan. By integrating these different elements, ChatGPT-4 provides a holistic view of energy consumption, factoring in both the quantitative and qualitative aspects influencing usage.
Conclusion
ChatGPT-4's application in energy consumption forecasting provides a dynamic, intelligent, and promising solution for more effective DR planning. The technology's ability to glean insights from vast sets of data, learn from past patterns, and predict future trends offers the potential for unprecedented accuracy in DR forecasting. It marks a significant step forward in making the energy industry more sustainable and efficient.
Comments:
Thank you all for your feedback and insights on the article! I'm glad to see the interest in ChatGPT's impact on demand response in technology.
ChatGPT seems like a promising tool for enhancing efficiency in demand response. Can you share some specific use cases where it has been successfully implemented?
Absolutely, Alice! ChatGPT has been widely utilized in various industries to optimize demand response. One example is its application in the energy sector, where it has improved load forecasting accuracy.
That's impressive, Nick! I can see how accurate load forecasting can have a significant impact on achieving efficient demand response.
Indeed, Bob! Accurate load forecasting helps grid operators make informed decisions to balance supply and demand effectively. It enables better utilization of resources and prevents unnecessary power shortages or excess.
Will ChatGPT replace human involvement in demand response completely? Or is it more of a complementary tool for decision-making?
Great question, Eva! ChatGPT is designed to augment human involvement in demand response, rather than replacing it entirely. It assists professionals by providing real-time insights and recommendations, leading to more informed decision-making.
That makes sense! It's always beneficial to have AI tools that can enhance human capabilities instead of replacing them altogether. Thanks for clarifying, Nick!
I'm curious about the scalability of ChatGPT in demand response. Can it handle large-scale systems or is it more suitable for smaller setups?
Good question, Chris! ChatGPT has demonstrated scalability in demand response applications. It can handle both smaller setups and large-scale systems, providing valuable insights and suggestions to optimize efficiency at any scale.
I wonder if ChatGPT's performance is affected by unpredictable variations in demand patterns. How adaptable is it to changing circumstances?
Excellent question, Dave! ChatGPT is designed to be adaptable to changing circumstances and demand patterns. It leverages advanced machine learning techniques to continually learn and update its models, ensuring it remains effective even in the face of unpredictable variations.
That's reassuring to know, Nick. Thanks for the information!
Do you have any insights into the potential challenges or limitations of implementing ChatGPT in demand response systems?
Certainly, Emily! While ChatGPT offers significant benefits, some challenges include the need for comprehensive data integration, ensuring data privacy and security, and managing human-AI collaboration effectively. Overcoming these challenges is crucial to maximize the potential of ChatGPT in demand response systems.
Thank you, Nick. It's important to consider these challenges to ensure successful implementation.
Are there any ethical considerations to keep in mind when utilizing ChatGPT in demand response?
Absolutely, Sarah! Ethical considerations are vital when integrating AI tools like ChatGPT. Transparency, accountability, and fairness should be prioritized to avoid potential biases or unintended consequences in decision-making processes.
I'm glad that ethical aspects are being highlighted. It's crucial to ensure AI technologies are used responsibly.
How can organizations justify the investment in ChatGPT for demand response? Are there any quantifiable benefits?
Great question, Tim! Implementing ChatGPT in demand response can lead to quantifiable benefits like improved operational efficiency, reduced costs, increased accuracy in load forecasting, and optimized resource utilization. These benefits justify the investment and contribute to achieving sustainable and resilient energy systems.
Thank you for the detailed response, Nick. It's helpful to understand the tangible benefits organizations can expect.
Do you have any real-world case studies where ChatGPT has been successfully deployed in demand response projects?
Certainly, Olivia! There have been several real-world case studies showcasing the successful deployment of ChatGPT in demand response projects. I can provide you with some references and resources to explore further. Please feel free to reach out to me via email.
Thank you, Nick! I'll definitely reach out to you for more information.
Do you think ChatGPT has the potential to revolutionize other areas beyond demand response in technology?
Absolutely, Kevin! ChatGPT's potential extends beyond demand response. Its capabilities can be harnessed in various domains, such as customer support, content generation, and virtual assistants. The possibilities for its application are vast.
That's exciting to hear, Nick. It seems like ChatGPT has immense potential in transforming several industries!
I'm concerned about the impact of AI on job roles. Could ChatGPT lead to job displacement in demand response professions?
A valid concern, Grace. While ChatGPT automates certain tasks and provides valuable assistance, it is not intended to replace demand response professionals. Instead, it enhances their capabilities, leading to more efficient and insightful decision-making. The human element remains crucial in demand response professions.
Thank you for addressing my concern, Nick. It's reassuring to know that human involvement is still valued in demand response.
What are the training requirements for professionals looking to work with ChatGPT in demand response?
To work effectively with ChatGPT in demand response, professionals should have a solid understanding of AI technologies and how they can be integrated into decision-making processes. Familiarity with data analysis, system modeling, and domain-specific knowledge is also beneficial.
Thank you, Nick! It's helpful to know the knowledge areas professionals should focus on.
Is ChatGPT accessible to small-scale demand response projects with limited resources?
Certainly, Max! ChatGPT's accessibility is not limited to large-scale projects. It can be tailored to suit the needs of small-scale demand response initiatives with limited resources. Its flexibility and versatility contribute to its widespread applicability.
That's great to know, Nick. Small-scale projects can also benefit from the efficiencies offered by ChatGPT!
How does ChatGPT handle real-time data in demand response? Can it adapt to rapidly changing conditions?
Excellent question, Sophia! ChatGPT is designed to handle real-time data in demand response and can adapt to rapidly changing conditions. It continually processes incoming data to provide up-to-date insights, ensuring timely decision-making even in dynamic scenarios.
That's impressive! Real-time capabilities are crucial for effective demand response. Thanks for the response, Nick.
You're welcome, Sophia! If any of you have further questions or require additional information, feel free to ask. I'm here to help!
Can ChatGPT be integrated with existing demand response systems or does it require a standalone implementation?
ChatGPT can be integrated with existing demand response systems, Tom. Its modular architecture allows seamless integration, ensuring compatibility and interoperability with different setups.
That's great news! Integration with existing systems simplifies the adoption process. Thanks, Nick!
What kind of technical support is available for organizations implementing ChatGPT in demand response?
Organizations implementing ChatGPT in demand response can benefit from technical support in terms of integration assistance, troubleshooting, and guidance on maximizing the tool's potential. Support channels like documentation, tutorials, and user forums are typically provided to ensure a smooth implementation experience.
Thank you, Nick. It's good to know that organizations can rely on technical support during the implementation phase.
Have there been any concerns raised regarding the accuracy or reliability of ChatGPT's recommendations in demand response?
Valid question, Henry. The accuracy and reliability of ChatGPT's recommendations depend on the quality of the underlying data and the models used. It's essential to ensure data integrity, conduct regular validations, and monitor the performance of the system. Continuous refinement and feedback incorporation are crucial in maintaining reliability.
Appreciate the insights, Nick. Rigorous quality control is indeed necessary to ensure accurate recommendations.
Are there any specific data requirements and data processing steps needed to implement ChatGPT effectively in demand response?
To implement ChatGPT effectively in demand response, organizations should ensure data quality, relevance, and diversity. Preprocessing steps like data cleaning, normalization, and feature extraction may be necessary. Additionally, training the models on up-to-date data and continually updating them is crucial to maintain accuracy.
Thank you, Nick. It's helpful to have an overview of the data requirements and processing steps involved.
You're welcome, Adam! Don't hesitate to ask if you need more guidance or have further questions.
Is it possible to customize ChatGPT for specific industry needs or is it a general-purpose tool for demand response?
ChatGPT is flexible and can be customized for specific industry needs within the field of demand response. By fine-tuning the models and incorporating domain-specific knowledge, organizations can tailor ChatGPT to meet their unique requirements and challenges.
That's fantastic, Nick! Customization capabilities enhance the tool's versatility. Thanks for the response!
What kind of computational resources are required to run ChatGPT effectively in demand response?
Running ChatGPT effectively in demand response typically requires computational resources like powerful GPUs or dedicated processors. Cloud-based infrastructure or on-premise high-performance computing systems can provide the necessary resources for efficient deployment and operation.
Thank you, Nick! Knowing the computational resource requirements helps organizations plan for a smooth implementation.
Does ChatGPT support multi-channel communication for demand response professionals to collaborate efficiently?
Multi-channel communication is essential for effective collaboration, Julia. ChatGPT can be integrated with existing communication tools or platforms, enabling demand response professionals to leverage its capabilities and collaborate efficiently across different channels.
That's great to hear, Nick. Integration with existing communication tools simplifies the workflow. Thanks for clarifying!
You're welcome, Julia! If anyone else has questions or needs further information, don't hesitate to ask. I'm here to assist you!
How does ChatGPT handle privacy and confidentiality concerns when dealing with sensitive demand response data?
Privacy and confidentiality are of utmost importance, Ben. ChatGPT follows strict data protection protocols and can be designed to operate within secure environments. Organizations should ensure compliance with relevant regulations and implement appropriate data security measures to mitigate any privacy concerns.
Glad to hear that privacy is taken seriously, Nick. It's crucial to maintain data integrity and protect sensitive information.
Are there any ongoing research or development efforts to enhance and expand the capabilities of ChatGPT in demand response?
The development of ChatGPT and its application in demand response is a dynamic field with ongoing research and development efforts. Continuous improvements in models, techniques, and data handling are being explored to further enhance the tool's capabilities and address emerging challenges.
That's great to know, Nick. Continuous research is crucial to keep pace with the evolving demands of the industry. Thanks for the response!
You're welcome, Sam! If you have any further inquiries or require additional insights, feel free to reach out. I'm here to assist you!
What level of technical expertise is required to integrate ChatGPT effectively in demand response systems?
Integrating ChatGPT effectively in demand response systems typically requires a certain level of technical expertise. Familiarity with AI technologies, data integration, and system architecture is beneficial. However, organizations can also seek assistance from AI implementation specialists or consultants to ensure a smooth integration process.
Thank you, Nick! It's helpful to have a sense of the technical know-how required for successful integration.
You're welcome, Mark! If you or anyone else has further questions or requires additional guidance, don't hesitate to ask.
How does ChatGPT handle demand response in complex systems with interconnected grids and diverse energy sources?
Handling demand response in complex systems with interconnected grids and diverse energy sources requires sophisticated modeling and analysis, Linda. ChatGPT can handle such complexities by incorporating system-wide data and leveraging its advanced machine learning capabilities to provide insights and recommendations that consider the interdependencies and diverse energy sources.
That's impressive, Nick. Managing demand response in complex systems is crucial for achieving efficient and sustainable energy usage.
Indeed, Linda! It's a challenging but vital aspect of demand response. If you need more information or have specific questions, feel free to ask.
Besides demand response, can ChatGPT be utilized in other areas of the energy industry, such as energy storage management?
Absolutely, Tommy! ChatGPT's capabilities can extend to various areas of the energy industry, including energy storage management. Its ability to provide real-time insights and recommendations makes it a valuable tool in optimizing energy storage operations and ensuring efficient utilization.
That's great news, Nick! The versatility of ChatGPT opens up numerous possibilities for enhancing energy industry operations.
Are there any known limitations to ChatGPT's performance in demand response that organizations should be aware of?
While ChatGPT offers significant benefits, organizations should be aware of its limitations. ChatGPT's performance can be hindered by insufficient or low-quality data, biased training data, and the need for regular model updates. Organizations should address these limitations through robust data management practices and continuous monitoring.
Thank you for highlighting the limitations, Nick. Addressing them appropriately will contribute to maximizing the benefits of ChatGPT in demand response.
How can organizations evaluate the effectiveness of ChatGPT in their demand response systems?
To evaluate the effectiveness of ChatGPT in demand response systems, organizations can compare key performance indicators like operational efficiency, load forecasting accuracy, and cost optimization before and after implementing ChatGPT. Additionally, gathering feedback from demand response professionals and monitoring decision-making improvements can provide valuable insights.
Thanks for the guidance, Nick! Evaluating the impact is crucial to measure the success of the implementation.
You're welcome, Jeff! If anyone else has questions or requires further assistance, feel free to ask. I'm here to help!
What kind of challenges can organizations expect during the initial deployment of ChatGPT in demand response?
During the initial deployment of ChatGPT in demand response, organizations may face challenges related to data integration, system compatibility, and user adaptation. Clear communication, proper training, and a phased deployment approach can help mitigate these challenges and ensure a smooth adoption process.
Thank you, Nick! Being aware of potential challenges can help organizations prepare and address them effectively.
Precisely, Oliver! If you or anyone else has additional inquiries or requires further information, feel free to ask.
How does the cost of implementing ChatGPT in demand response compare to the potential benefits it offers?
The cost of implementing ChatGPT in demand response may vary depending on the organization's specific requirements and infrastructure. However, the potential benefits like improved efficiency, cost optimization, and accurate decision-making can outweigh the initial investment, making ChatGPT a cost-effective tool in the long run.
Appreciate the response, Nick. It's essential to consider both costs and benefits when evaluating any implementation.
Absolutely, Hannah! Considering the costs and benefits leads to informed decision-making. If you need more insights or have further questions, feel free to ask.
Are there any recommended best practices for organizations implementing ChatGPT in demand response for the first time?
Certainly, Sophie! When implementing ChatGPT in demand response for the first time, organizations should start with specific use cases and clear objectives. Conducting pilot projects, ensuring user training, and gradually expanding the deployment can help achieve successful adoption. Collaboration with AI specialists and continuous evaluation are also recommended best practices.
Thank you for sharing the best practices, Nick. They provide a valuable framework for organizations venturing into ChatGPT integration.
Thank you all for taking the time to read my article! I'm excited to discuss how ChatGPT is revolutionizing demand response in technology. Please feel free to share your thoughts and any questions you may have.
Great article, Nick! The potential for ChatGPT in demand response is truly fascinating. I can see how leveraging its capabilities can greatly improve efficiency. One concern I have, though, is the reliability of the AI in critical situations. What measures are being taken to ensure its accuracy and responsiveness?
Jennifer, ensuring reliability is crucial. OpenAI has implemented fine-tuning techniques to make AI models more reliable in production. Additionally, regular auditing and monitoring help detect and rectify any inaccuracies or biases that may arise.
Hi Nick, thanks for sharing this informative article. I agree with Jennifer, the prospects of using ChatGPT in demand response are promising. However, my biggest worry is about potential cybersecurity risks. How is the system protected against malicious attacks or misuse?
Adam, cybersecurity is a significant concern. OpenAI employs robust security practices, including ongoing vulnerability assessments, third-party audits, and a strong data governance framework to prevent misuse and protect against potential attacks.
I find the idea of ChatGPT integration in demand response quite intriguing, Nick. It has the potential to enhance communication and flexibility in the field. Do you have any real-world examples of successful implementation?
Rebecca, absolutely! An example of successful implementation is in customer support services. ChatGPT has been used to automate responses, handle FAQs, and provide instant assistance to users, resulting in significant improvements in response time and customer satisfaction.
Nick, I appreciate your insights. The potential benefits of ChatGPT in demand response are immense. However, I'm curious about the limitations. Are there any scenarios where ChatGPT might struggle or not perform optimally?
Michael, while ChatGPT is impressive, it does have limitations. It may sometimes struggle with ambiguous or nonsensical queries, and occasionally provide inaccurate responses. OpenAI is actively working on improving these weaknesses and actively seeks user feedback to enhance the system's performance.
This article is eye-opening, Nick. The utility of ChatGPT in demand response is remarkable. I wonder, though, how training the AI model with industry-specific data can affect its performance and adaptability across different domains.
Emma, retraining the model with domain-specific data can significantly improve performance within that domain. However, adapting to new domains might require additional fine-tuning and data to ensure optimal performance. It's an ongoing research challenge for OpenAI.
I find the potential of ChatGPT in demand response fascinating, Nick. The advancements in AI never cease to impress. However, I wonder how the technology handles and adapts to dynamic and unpredictable situations.
Oliver, dynamic situations can be challenging, and ChatGPT's responses may not always be perfect. While it can handle some unpredictability, extreme scenarios or sudden changes might lead to less reliable outputs. Development in this area is ongoing to enhance performance in such dynamic environments.
Great article, Nick! ChatGPT's utilization in demand response can provide significant advantages. I'm curious though, how does the system handle multiple user inputs simultaneously, especially during peak demand periods?
Sophia, during peak demand periods, the system can handle multiple inputs simultaneously. However, to ensure efficient responses and avoid potential bottlenecks, optimization techniques like load balancing and priority queuing are commonly employed.
Interesting article, Nick! ChatGPT's potential in demand response is definitely exciting. However, I'm curious about how the AI model updates itself to incorporate new information and changes in demand patterns.
Daniel, updating the AI model requires retraining with new data. OpenAI periodically releases new models, and fine-tuning processes ensure the AI stays up-to-date with the latest information and changing demand patterns.
Nick, I appreciate your article. The use of ChatGPT in demand response can certainly streamline processes. I wonder, though, how user training and feedback contribute to improving the system's performance over time?
Sophie, user training and feedback play a vital role in improving the system. OpenAI actively encourages users to provide feedback on problematic outputs, which helps the model iterate and learn over time, leading to better performance.
Thanks for sharing, Nick. ChatGPT's incorporation in demand response is certainly a game-changer. My concern is its dependency on an internet connection. How does it handle scenarios where connection disruptions occur?
Liam, connection disruptions can indeed pose challenges. However, ChatGPT can still function partially offline by utilizing pre-trained components. Employing local caches or minimizing reliance on constant connectivity can help mitigate the impact of connection issues.
I found the article very informative, Nick. ChatGPT's potential in demand response is fascinating. However, I'm curious about the system's ability to handle regional or geographically specific demand patterns.
Zoe, regional or geographically specific demand patterns can be incorporated into the training process by providing relevant data from different regions. This helps the system learn and adapt to specific demands, improving its performance in region-specific scenarios.
Excellent article, Nick. ChatGPT's integration in demand response opens up numerous possibilities. However, what are the privacy concerns associated with user interactions and data storage?
Ethan, privacy concerns are taken seriously. While user interactions can provide valuable training data, OpenAI is dedicated to safeguarding personal information. Anonymizing and securely storing data while complying with privacy regulations ensures user privacy is protected.
Nick, your article presents a compelling use case for ChatGPT. I'm curious about the system's ability to handle unforeseen or exceptional scenarios that may arise during demand response operations.
Melissa, handling unforeseen or exceptional scenarios is an area where further improvements are being made. While ChatGPT aims to deliver reliable responses, it may not always handle complex and exceptional situations optimally. Research and development are ongoing to enhance performance in these scenarios.
The potential of ChatGPT in demand response is truly exciting, Nick. I wonder how the system handles evolving regulations and policy changes that impact the energy sector.
Abigail, staying up-to-date with evolving regulations and policies is essential. OpenAI ensures that as new policies come into effect, the AI model is updated and modified to meet the changing requirements in the energy sector.
Nick, your article sheds light on a fascinating development. ChatGPT's role in demand response is compelling. Can the system handle multi-lingual interactions, or is it limited to specific languages?
Lucas, ChatGPT has been predominantly trained on English text, so it performs best in English conversations. However, efforts are underway to improve its capability in handling multi-lingual interactions to make it more versatile and accessible.
Fascinating article, Nick. The potential for ChatGPT in demand response is immense. However, I'm curious about the system's ability to handle diverse and varying user preferences and needs.
Emily, handling diverse user preferences and needs is an ongoing challenge. ChatGPT can learn from user training data to adapt to specific preferences over time. As the system evolves, efforts are made to enhance personalization and cater to a wide range of user contexts.
Nick, this article is an excellent overview of ChatGPT's impact on demand response. Considering different user contexts and situations, how does the AI model handle personalized and context-specific recommendations?
Alexander, providing personalized and context-specific recommendations is a crucial aspect. By leveraging user inputs and historical data, ChatGPT can make personalized suggestions that align with the unique needs and contexts of users, further enhancing the user experience.
Impressive article, Nick! ChatGPT's role in demand response is intriguing. However, I'm concerned about the ethical implications of relying on AI for critical decision making. What strategies are in place to mitigate potential biases?
Grace, addressing potential biases in AI decision making is crucial. OpenAI is committed to reducing both glaring and subtle biases present in the models and actively seeks external input and audits to prevent unintended consequences and ensure fairness and ethical usage.
Nick, your article provides valuable insights into ChatGPT's integration in demand response. I'm curious about the system's ability to handle real-time data and time-sensitive operations.
Alexandra, ChatGPT is designed to handle real-time data and time-sensitive operations. Through continuous training and optimization, the system learns to process incoming data efficiently and provide prompt responses, ensuring it can meet the demands of time-sensitive applications.
Nick, your article truly showcases the transformative potential of ChatGPT in demand response. I'm curious about the system's adaptability to different scales, from individual users to large-scale energy management.
Luke, adaptability across different scales is a key strength. ChatGPT can serve individual users, providing personalized responses, and scale up to cater to large-scale energy management systems, offering insights and recommendations to optimize energy usage on a broader level.
Great article, Nick! ChatGPT's application in demand response is impressive. However, how does the system handle user error or ambiguous requests?
Lily, the system is designed to handle user errors and ambiguous requests. It can request clarifications or ask follow-up questions to ensure it understands the user's intent. OpenAI is continuously improving the model's ability to handle vague queries and minimize errors.
Nick, your article sheds light on a promising use of ChatGPT. I'm curious about the system's ability to learn from real-time feedback and adapt to changing user preferences.
Sarah, learning from real-time feedback is vital for the system's improvement. OpenAI actively encourages users to provide feedback, which is used to iteratively train and fine-tune the model, ensuring it adapts to changing user preferences and delivers more relevant responses.
This article provides valuable insights, Nick. I'm curious about the speed and efficiency of ChatGPT's responses, particularly when handling a large volume of user interactions.
David, speed and efficiency are essential. While ChatGPT strives for prompt responses, the system's performance can vary depending on the volume of users. Techniques like resource allocation and load balancing are employed to optimize response times during periods of high interaction.
Nick, your article highlights a fascinating application of ChatGPT. I'm curious if the system is capable of understanding and handling complex technical language or jargon in the energy industry.
Mia, the system can understand and handle technical language and jargon related to the energy industry to a certain extent. However, it may encounter challenges with highly specialized terminology or niche subdomains. Ongoing training and exposure to relevant data help improve the system's understanding and performance in this regard.
Great article, Nick! ChatGPT's utilization in demand response is impressive. Can the system handle multi-step requests that involve a series of actions or instructions from the user?
Noah, multi-step requests are within the system's capability. The model can handle a series of actions or instructions from the user and generate appropriate responses at each step. However, there might be limitations in handling highly complex sequences, and the system's performance can vary depending on the complexity of the requested actions.