ChatGPT Empowers Natural Language Understanding in Semantic Web Technology
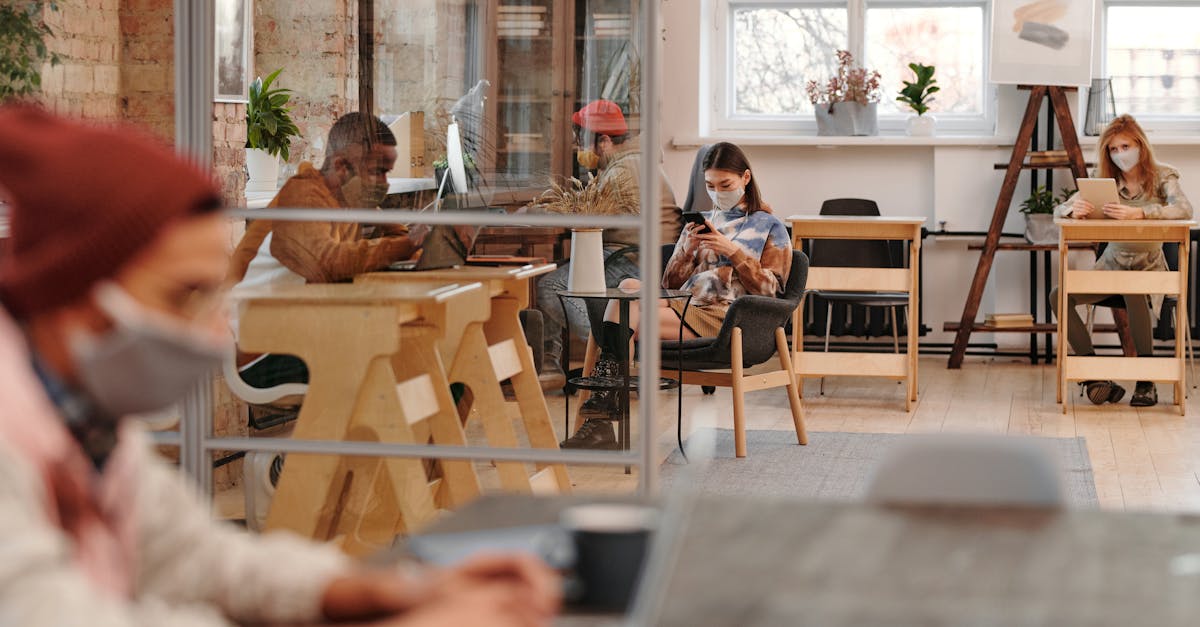
Introduction
In today's digital age, the vast amount of information available online requires efficient methods for understanding and classifying content. One technology that has revolutionized this area is the Semantic Web. By leveraging ontologies, Linked Data, and other semantic technologies, the Semantic Web enables improved natural language understanding, facilitating tasks such as content classification, sentiment analysis, and more.
Understanding Semantics in Natural Language
Traditionally, natural language processing techniques relied heavily on statistical approaches, which often struggled with nuances and the finer aspects of language. However, the Semantic Web offers a more sophisticated approach to understanding the semantics in natural language by utilizing structured data and ontologies.
Ontologies define a common vocabulary for a specific domain and establish relationships between entities. By incorporating ontologies into natural language processing tasks, the Semantic Web enables systems to recognize the meaning behind words and phrases, leading to more accurate content understanding.
Content Classification
One of the key applications of the Semantic Web in natural language understanding is content classification. By encoding knowledge about different topics in ontologies, it becomes possible to automatically categorize and organize textual content.
For example, consider a news aggregator application that needs to categorize news articles into various topics such as sports, politics, and entertainment. By leveraging ontologies that describe these topics and their relationships, the application can analyze the text of each article and accurately assign it to the appropriate category.
This automated content classification not only saves time and effort but also improves the user experience by allowing users to easily find relevant content based on their interests.
Sentiment Analysis
Another important application of the Semantic Web in natural language understanding is sentiment analysis. Sentiment analysis aims to determine the sentiment expressed in a piece of text, whether it is positive, negative, or neutral.
The Semantic Web enhances sentiment analysis by incorporating domain-specific ontologies that capture the nuances and context-related information required for accurate sentiment classification. By leveraging such ontologies, systems can recognize sentiment-bearing words or phrases and understand the overall sentiment expressed in a sentence or document.
For instance, in a customer review analysis system, the Semantic Web can help determine whether a review expresses satisfaction or dissatisfaction with a product by understanding the sentiment-bearing words in the text and their context.
Accurate sentiment analysis enables businesses and organizations to gain insights into public opinion, customer feedback, and market trends. This information can be invaluable for making informed decisions and improving products or services.
Conclusion
The Semantic Web offers significant advancements in natural language understanding, making it a crucial technology for tasks such as content classification and sentiment analysis. By incorporating ontologies, Linked Data, and other semantic technologies, the Semantic Web enables systems to grasp the semantics of natural language more accurately, leading to improved efficiencies and user experiences.
As the digital landscape continues to grow, the Semantic Web will continue to play a vital role in enabling machines to understand and process human language, bringing us closer to a more intelligent and intuitive web.
Comments:
Thank you all for joining the discussion! I appreciate your participation.
Great article, Tiffani! ChatGPT seems like a promising tool for enhancing natural language understanding in semantic web technology. Do you think it has the potential to significantly improve current systems?
Hi Carlos! Thank you for your kind words. Yes, I do believe ChatGPT has the potential to make significant improvements in natural language understanding. Its ability to generate coherent responses makes it valuable for various applications. However, it's important to carefully consider ethical considerations as well.
I found the article very informative. It's fascinating how ChatGPT can navigate the complex nuances of natural language. Tiffani, how do you think it compares to other natural language processing models?
Hi Melissa! I'm glad you found the article informative. ChatGPT incorporates advancements from models like GPT-3 and excels in generating human-like responses. While it has its strengths, there are always ongoing research and development to further improve these models.
Thank you, Tiffani, for shedding light on ChatGPT's role in semantic web technology. The potential of this technology to enhance human-computer interaction is exciting. Can you provide an example of its practical applications?
Hi Jennifer! Absolutely, ChatGPT can find applications in chatbots, virtual assistants, customer support systems, and even content generation. Its ability to understand and respond to natural language opens up a wide range of possibilities.
I can see how ChatGPT's natural language understanding can benefit semantic web technology, but what are the challenges it faces? Are there any limitations we should consider?
Hi Daniel! Good question. ChatGPT has some limitations, such as being sensitive to input phrasing and context. It can sometimes produce incorrect or biased responses. Ensuring ethical use and addressing these limitations are key areas of focus for further improvement.
I'm impressed with ChatGPT's capabilities. It's exciting to see advancements in natural language understanding. Tiffani, do you think future iterations of ChatGPT will be even more proficient?
Hi Michelle! Yes, future iterations of ChatGPT are expected to be more proficient and refined. OpenAI is continuously working on new models and techniques to enhance their language models, which will likely result in improved performance in the future.
I can see immense potential in incorporating ChatGPT in various industries. However, how do you address concerns about biases in language models like ChatGPT?
Hi Anthony! Addressing biases is crucial. OpenAI is investing in research to reduce both glaring and subtle biases in models like ChatGPT. They are also working on providing clearer instructions to users about influencing the system's behavior without relying on implicit biases.
ChatGPT seems like a powerful tool. Tiffani, what are the computational requirements for deploying and using ChatGPT effectively?
Hi Angela! Running ChatGPT might require considerable computational resources, especially for large-scale deployments. However, OpenAI is actively working on various approaches to make this technology more accessible and efficient.
The potential of ChatGPT in semantic web technology is impressive. Are there any specific use cases you envision for it?
Hi Steven! Absolutely, ChatGPT can be used for tasks like question-answering, document summarization, language translation, and more. Its versatility allows it to be applied to various domains requiring natural language understanding.
From the sound of it, ChatGPT is a significant leap in natural language understanding. Tiffani, what steps can developers take to responsibly integrate ChatGPT in their applications?
Hi Emily! Responsible integration of ChatGPT involves evaluating and mitigating biases in the model's outputs, setting clear guidelines, and incorporating user feedback loops for continuous improvement. It's crucial to ensure ethical usage and transparency when deploying these models.
I'm excited about the potential of ChatGPT in transforming how we interact with technology. Tiffani, do you think we'll witness widespread adoption of similar technologies in the future?
Hi Sophia! Yes, I believe we will witness widespread adoption of similar technologies as they continue to improve. Advancements in natural language understanding pave the way for more intuitive and efficient interactions between humans and machines.
ChatGPT's potential to enhance natural language understanding is evident. Tiffani, what are your thoughts on the ethical aspects and safeguards associated with its use?
Hi David! Ethical aspects of ChatGPT's use are crucial. Developing safeguards to prevent misuse, addressing biases, and ensuring transparent guidelines are important steps. OpenAI is committed to responsible AI development and continues to research and invest in mitigating such concerns.
This article has given me a new perspective on the possibilities of natural language understanding. ChatGPT's ability to generate coherent responses is impressive. Tiffani, what further advancements do you hope to see in this technology?
Hi Rachel! I'm glad the article provided a fresh perspective. In terms of further advancements, I hope to see improved bias mitigation, better handling of ambiguous queries, and increased domain-specific understanding to make interactive systems even more effective and reliable.
ChatGPT is undoubtedly an impressive advancement. Tiffani, what role do you see ChatGPT playing in the future development of the semantic web?
Hi Samuel! ChatGPT can contribute significantly to the development of the semantic web by enabling more natural language interactions with web applications, improving information retrieval, and enhancing user experience. It has the potential to make the web more accessible and user-friendly.
The article makes a compelling case for the impact of ChatGPT in semantic web technology. Tiffani, how do you suggest balancing the need for user-friendly interfaces while ensuring robustness and reliability?
Hi Nathan! Balancing user-friendly interfaces with robustness and reliability is indeed important. It requires careful design, usability testing, and continuous feedback loops to refine the system. Incorporating user trust and safety features is crucial to provide a positive user experience while maintaining reliability.
ChatGPT's impact on natural language understanding is remarkable. Tiffani, what are the key factors to consider when implementing ChatGPT for specific applications?
Hi Oliver! When implementing ChatGPT for specific applications, key factors to consider include data quality, potential biases in the training data, selecting appropriate prompts, and ongoing monitoring and evaluation of the system's responses. These factors help fine-tune the application to achieve desired outcomes.
The potential of ChatGPT is intriguing. Tiffani, could you explain the underlying technology that makes ChatGPT's natural language understanding possible?
Hi Sophie! ChatGPT builds on transformer-based language models like GPT (Generative Pre-trained Transformer) that use self-attention mechanisms to understand and generate text. These models are pre-trained on large-scale datasets and fine-tuned for specific tasks. The generative nature of ChatGPT enables it to produce contextually relevant responses.
I can see the potential of ChatGPT in revolutionizing conversational AI. Tiffani, in your opinion, what kind of impact will ChatGPT have on human-computer interaction in the coming years?
Hi Gregory! ChatGPT has the potential to greatly impact human-computer interaction by enabling more natural conversations with machines. This can lead to improved user experiences, increased accessibility, and more efficient and intuitive interactions. It has the potential to shape the future of AI-powered interfaces.
The advancements in natural language understanding demonstrated by ChatGPT are impressive. Tiffani, what are some practical steps organizations can take to adopt ChatGPT effectively?
Hi Jonathan! To adopt ChatGPT effectively, organizations can start by identifying specific use cases where it can add value. They should invest in quality training data, consider ethical implications, devise strategies for handling biases, and provide continuous feedback to improve the system's performance.
The potential applications of ChatGPT are extensive. Tiffani, in your opinion, what are some of the key challenges that need to be addressed for wider adoption?
Hi Liam! Some key challenges for wider adoption of ChatGPT include addressing biases, ensuring interpretability of the system's responses, improving consistency, refining error handling, and further reducing incorrect or nonsensical outputs. These challenges need to be addressed to enhance user trust and confidence.
ChatGPT's potential is fascinating. Tiffani, what are some of the key factors that influence the system's response quality?
Hi Harper! The response quality of ChatGPT is influenced by factors such as the input phrasing, relevance of the training data, prompt design, and the context provided. Iterative feedback from users and continuous retraining also play a crucial role in improving and refining the response quality.
ChatGPT can revolutionize human-computer interaction. Tiffani, can you provide some insights into the potential implications for user privacy?
Hi Victoria! User privacy is an important consideration. OpenAI is committed to maintaining user privacy and has implemented measures to protect data. Organizations deploying ChatGPT should also ensure adherence to privacy regulations and implement appropriate safeguards to protect user information.
The advancements in natural language understanding are remarkable. Tiffani, how do you see the coexistence of human and AI conversations evolving in the future?
Hi Lucas! The coexistence of human and AI conversations is likely to evolve towards more seamless and natural interactions. As AI models like ChatGPT continue to improve, they will complement human conversations by providing valuable assistance, answering queries, and enhancing productivity without completely replacing human involvement.
ChatGPT has the potential to reshape various industries. Tiffani, how do you envision the future of natural language understanding technology beyond ChatGPT?
Hi Abigail! Beyond ChatGPT, the future of natural language understanding holds immense possibilities. We can expect even more advanced models with improved contextual understanding, better handling of ambiguity, and increased application-specific adaptation. Natural language understanding technology will continue to contribute to diverse fields and revolutionize human-machine interactions.
ChatGPT's capabilities are impressive. Tiffani, do you think we are close to achieving human-level natural language understanding with such models?
Hi Lily! While models like ChatGPT exhibit remarkable natural language understanding, achieving true human-level understanding is still a complex challenge. These models have made significant progress, but there is more work to be done to bridge the gap between machine understanding and human communication.
Thank you all for your engaging discussion and insightful questions! It's been a pleasure discussing ChatGPT's potential with you. Let's continue exploring the exciting developments in natural language understanding together!