ChatGPT in the AUTOCR Sphere: Leveraging Neural Networks for Advanced Conversational AI
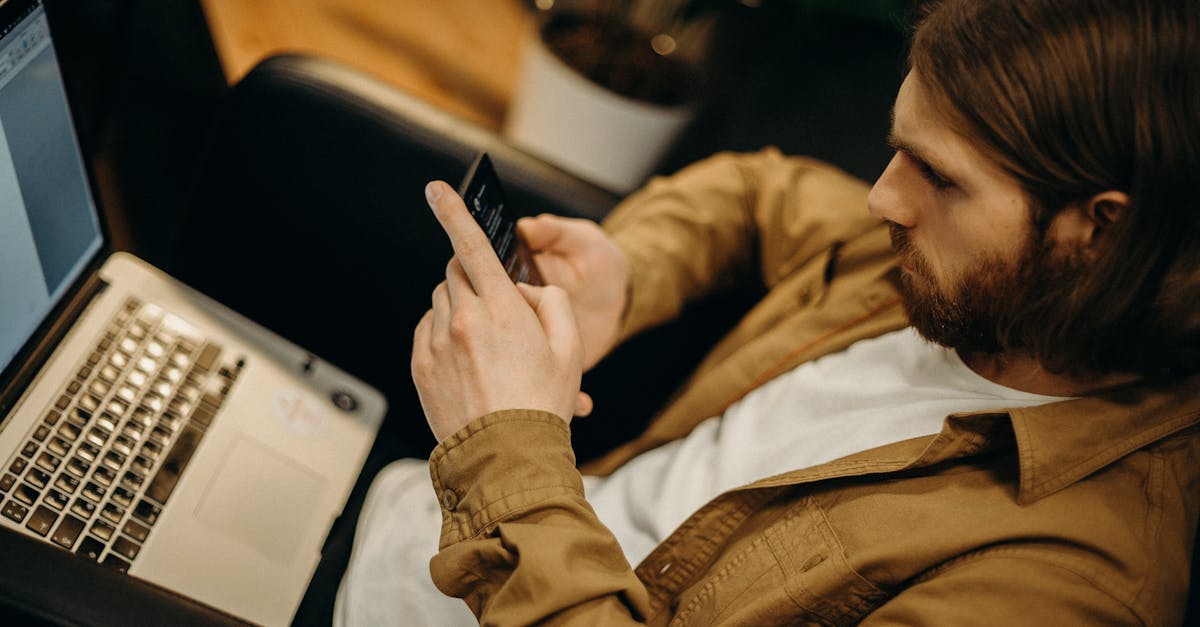
Automatic Number Plate Recognition (ANPR) systems have become an essential technology in various fields, including law enforcement, traffic monitoring, and parking management. The accuracy and efficiency of ANPR systems greatly depend on their ability to recognize and interpret number plates accurately and in real-time. In recent years, advancements in artificial intelligence, specifically in the field of neural networks, have paved the way for significant improvements in ANPR technology.
Neural networks, a subset of AI, have shown great potential in various domains, including image recognition and natural language processing. GPT-4 (Generative Pre-trained Transformer 4) is an advanced neural network architecture that has gained significant attention in the AI community. While GPT-4 is primarily known for its natural language generation capabilities, it can also be applied to enhance ANPR systems.
One of the challenges in ANPR systems is the ability to accurately recognize distorted, low-quality, or occluded number plates. Traditional ANPR systems rely on predefined rules and handcrafted features, which may not handle such variations effectively. By incorporating GPT-4 into the ANPR pipeline, the system can benefit from its ability to understand and generate contextually relevant information.
GPT-4 can improve the efficiency of automatic number plate recognition systems in several ways:
1. Enhanced Contextual Understanding
GPT-4 can analyze the contextual information surrounding a number plate and generate more accurate interpretations. By considering not only the number plate itself but also the surrounding environment, such as the type of vehicle, location, and time, the ANPR system can make more informed decisions.
2. Image Restoration
Neural networks have shown remarkable success in image restoration tasks. GPT-4 can be utilized to restore distorted or damaged number plates, improving the overall quality of input images and boosting the effectiveness of ANPR systems. This capability is particularly useful in scenarios where the number plate is partially occluded or captured under challenging lighting conditions.
3. Learning from Real-World Data
GPT-4 can be trained using large-scale datasets of number plates, allowing it to learn from the diverse range of variations and complexities present in real-world data. This enables the ANPR system to handle non-standard number plates, such as personalized or specialty plates, more effectively.
By incorporating GPT-4 and neural networks into ANPR systems, significant advancements can be achieved in terms of accuracy, efficiency, and overall performance. This technology has the potential to revolutionize the field of automatic number plate recognition, making it an indispensable tool for law enforcement, traffic management, and various other applications.
Comments:
Thank you all for taking the time to read my article on ChatGPT in the AUTOCR sphere. I'm looking forward to hearing your thoughts and engaging in a fruitful discussion!
Great article, Breaux! I found your insights on leveraging neural networks for conversational AI fascinating. It's exciting to see the advancements being made in this space.
I agree, Chris! The progress in conversational AI is truly impressive. Breaux, I appreciated your explanation of how ChatGPT can enhance the AUTOCR experience. It seems like it has a lot of potential.
Thank you, Chris and Lisa, for your kind words! The use of neural networks in conversational AI opens up new possibilities, and ChatGPT has indeed shown promising results in AUTOCR applications.
I have some concerns about relying too heavily on ChatGPT in the AUTOCR sphere. While it can generate impressive responses, we must also consider the potential risks of AI taking control of critical decision-making processes.
David, I understand your concerns. We should definitely take precautions when deploying AI in critical domains like AUTOCR. Ethical considerations and adequate human oversight are crucial to mitigate any potential risks.
Great points, Breaux! I'm particularly interested in the technical challenges you mentioned when using ChatGPT in the AUTOCR sphere. Can you provide more details on how you tackle those challenges?
Certainly, Alex! One of the main challenges is ensuring the reliability of ChatGPT's responses and minimizing errors that could impact AUTOCR operations. We employ rigorous testing, domain-specific fine-tuning, and human-in-the-loop feedback loops to continuously improve its performance.
Breaux, it's fascinating to see how you address those challenges. I'm curious, though, if ChatGPT has any limitations in understanding complex scenarios in the AUTOCR sphere where contextual understanding is crucial.
Valid question, Sara. While ChatGPT has improved contextual understanding, it may have limitations in complex AUTOCR scenarios. We mitigate this by incorporating specific training data and fine-tuning models to enhance its understanding in the AUTOCR domain.
It's amazing how far conversational AI has come! Breaux, your article showed the potential of ChatGPT in AUTOCR applications. How do you see it evolving in the future?
Thank you for your question, Kim! In the future, I envision ChatGPT evolving to handle even more complex AUTOCR scenarios, improved training with larger datasets, and increased integration of user feedback to enhance its performance and safety.
Breaux, I appreciate the insights you shared in your article. However, I wonder if there are any legal and privacy concerns associated with deploying ChatGPT in the AUTOCR sphere.
Good question, Mike. Legal and privacy concerns are indeed important considerations. When deploying ChatGPT, complying with data protection regulations, ensuring secure data handling, and maintaining transparency with users are key aspects we focus on.
Breaux, your article highlighted the benefits of conversational AI in the AUTOCR sphere. However, do you think integrating explainability mechanisms into ChatGPT is necessary for its adoption in critical applications?
A valid concern, Jessica. Explainability in AI models is crucial, especially for critical applications. While ChatGPT has limitations in this regard, additional research is ongoing to enhance its explainability and transparency.
Hi Breaux, I found your article very informative. One question I have is, do you see any potential uses of ChatGPT in other industries apart from AUTOCR?
Hi Rachel, glad you found the article informative! Absolutely, ChatGPT has potential applications in various industries like customer support, content generation, and even virtual personal assistants. Its versatility is captivating!
Breaux, I appreciate the insights you provided in your article. How scalable is the implementation of ChatGPT in the AUTOCR sphere, especially in high-demand scenarios?
Thank you, Eric! The scalability of ChatGPT in high-demand AUTOCR scenarios is an important consideration. By utilizing powerful hardware infrastructure and optimizing the system architecture, we aim to ensure its scalability without compromising performance.
Breaux, your article shed light on the promising capabilities of ChatGPT in the AUTOCR domain. Have you encountered any significant challenges during its development?
Thank you, Sam! Yes, there have been challenges, such as addressing biases in training data, improving reliability, and refining the balance between providing accurate responses and acknowledging uncertainty. It's a continuous learning process.
Breaux, your article got me curious about the training process for ChatGPT in the AUTOCR sphere. How do you ensure the model understands and handles diverse user queries?
Good question, Natalie! Training ChatGPT involves a combination of diverse datasets related to AUTOCR, extensive fine-tuning, and continuous evaluation and improvement based on user feedback. This iterative process helps in handling varied user queries effectively.
Breaux, thanks for sharing your article. One concern I have is the potential bias in ChatGPT's responses. How do you ensure fairness and mitigate bias in the AUTOCR context?
Thank you, Mark. Ensuring fairness and mitigating bias is crucial. We actively work on reducing bias in ChatGPT's responses by diverse data collection, adversarial testing, and regular audits to identify and address any biases that may arise.
Breaux, your article was well-written! Can you explain how you handle liability and accountability in the AUTOCR context when ChatGPT is involved?
Thank you, Laura! Liability and accountability are significant considerations. In the AUTOCR context, we ensure adequate human oversight, establish clear boundaries for ChatGPT, and provide safeguards to prevent any unintended consequences and assign responsibility when necessary.
Impressive article, Breaux! Can you share any real-world examples where ChatGPT has been successfully deployed in the AUTOCR sphere?
Thank you, Daniel! While ChatGPT is still in its early stages, it has been deployed successfully in certain AUTOCR scenarios, such as autonomous fleet management, dynamic route optimization, and proactive maintenance support.
Breaux, your article explored the advantages of ChatGPT in the AUTOCR domain. How do you handle situational awareness and real-time updates to ensure the system remains responsive and up-to-date?
Thank you, Hannah! Situational awareness and real-time updates are critical for AUTOCR systems. We integrate data streaming technologies, leverage sensor data, and establish efficient communication channels to ensure ChatGPT remains responsive and up-to-date.
Breaux, it's impressive to see how ChatGPT advances the conversational AI field in the AUTOCR context. How do you handle system reliability and minimize potential downtime?
Thank you, Oliver! System reliability and minimizing downtime are top priorities. We implement redundant systems, perform regular maintenance and updates, and closely monitor the system's performance to ensure reliability while minimizing any disruption.
Breaux, your article brings attention to the benefits of ChatGPT in the AUTOCR sphere. How do you see the human-AI collaboration evolving in the future?
An excellent question, Grace! In the future, I envision stronger collaborations between humans and AI, leveraging the strengths of each. Human expertise, creativity, and adaptability combined with AI's speed, accuracy, and scalability can unlock immense potential in the AUTOCR sphere.
Breaux, your article highlighted the potential of neural networks in AUTOCR. How do you ensure user privacy and manage data security when utilizing ChatGPT?
Thank you, Sophia! We prioritize user privacy and data security. By implementing robust encryption, securely handling user data, and adhering to privacy regulations, we ensure that ChatGPT maintains a high standard of privacy and data protection.
Breaux, great article! Can you provide some insights into the integration of ChatGPT with existing AUTOCR systems? Any challenges you've encountered?
Thank you, Justin! Integrating ChatGPT with existing AUTOCR systems can pose challenges, such as ensuring compatibility with legacy architectures, handling high-volume requests, and seamless integration with other components. However, we work closely with system integrators and maintain a modular design to ease the integration process.
Breaux, your article shed light on the potential of ChatGPT in AUTOCR. How do you handle system reliability and minimize potential downtime?
Hi Emily! System reliability and minimizing downtime are crucial considerations. We employ redundant systems, rigorous testing, automated monitoring, and proactive maintenance to ensure ChatGPT remains reliable, minimizing any potential disruptions.
Breaux, your article has piqued my interest in conversational AI in the AUTOCR domain. Are there any specific challenges you faced while applying ChatGPT in this context?
Thank you, Noah! Applying ChatGPT in the AUTOCR domain presents unique challenges, such as handling real-time constraints, adapting to complex traffic scenarios, and ensuring efficient communication with other AUTOCR components. Addressing these challenges requires continuous improvement and close collaboration with domain experts.
Breaux, great article! How do you ensure the safety and reliability of ChatGPT's responses in critical AUTOCR scenarios?
Thank you, Lucas! Ensuring the safety and reliability of ChatGPT's responses is crucial. We follow a rigorous verification process, incorporate safety checks, and frequently evaluate performance to mitigate risks and provide dependable responses in critical AUTOCR scenarios.
Breaux, your article highlighted the potential of ChatGPT in AUTOCR. Have you encountered any limitations or challenges specific to the AUTOCR sphere?
A valid question, Joshua! While ChatGPT has shown promising results, it may face challenges in understanding rare or niche AUTOCR scenarios. Addressing these limitations through continuous improvement, dedicated dataset curation, and fine-tuning is vital for wider adoption.
Breaux, your article got me thinking about the potential impact of ChatGPT on the job market. Do you see it replacing certain human roles in the AUTOCR sphere?
Good question, Abigail! While ChatGPT can automate certain tasks in the AUTOCR sphere, I see it more as a tool to augment human capabilities rather than replacing roles entirely. Collaboration between AI systems and human experts is key to harnessing the full potential of ChatGPT in AUTOCR.