ChatGPT Revolutionizes DataStage: Transforming Technology with AI Conversation
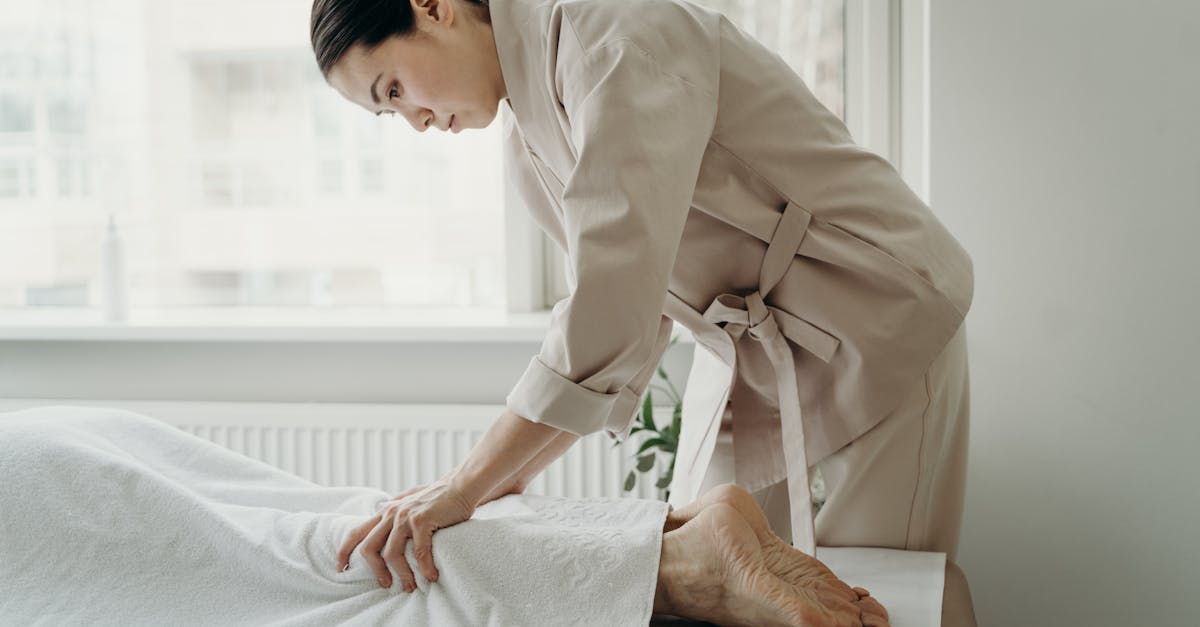
DataStage is a powerful data integration and transformation tool used in various industries for managing and processing large volumes of data. In the field of data cleaning, DataStage provides a comprehensive set of functionalities that help in identifying and resolving errors, redundancies, and inconsistencies within datasets.
One of the challenges in data cleaning is the manual effort required to identify and rectify errors or inconsistencies within datasets. This process can be time-consuming and error-prone. However, with the advent of advanced artificial intelligence tools like ChatGPT-4, data cleaning has become more efficient and accurate.
ChatGPT-4, powered by OpenAI, is a state-of-the-art language model capable of understanding and generating human-like text. It can be integrated into DataStage workflows to assist data analysts and scientists in cleaning datasets effectively.
Identifying Errors, Redundancies, and Inconsistencies
DataStage, combined with ChatGPT-4, excels in identifying errors, redundancies, and inconsistencies within datasets. By leveraging its powerful data profiling and analysis capabilities, DataStage can automatically scan datasets to identify potential issues.
ChatGPT-4 then comes into play by understanding the data structures, patterns, and context to provide valuable insights and suggestions for resolving the identified problems. Its ability to understand natural language queries allows data analysts to interact with the model and ask specific questions related to data cleaning.
Suggesting Solutions
Once errors or inconsistencies are identified, ChatGPT-4 can suggest potential solutions to data cleaning problems. By analyzing the dataset and understanding the underlying data rules and patterns, the model can propose appropriate transformations or modifications to address the issues at hand.
For example, if ChatGPT-4 identifies a column with inconsistent date formats, it can suggest a transformation to standardize the dates uniformly. It can also identify and recommend solutions for common data quality issues like missing values, duplicate records, or inaccurate data.
Streamlining Data Cleaning Workflow
DataStage, along with ChatGPT-4 integration, streamlines the data cleaning workflow by automating the identification and resolution of data quality problems. This not only saves time but also ensures greater accuracy in the cleaning process.
By automating repetitive and time-consuming tasks, data analysts and scientists can focus more on analyzing the data and deriving meaningful insights. This combination enables faster decision-making and improves the overall data quality within an organization.
Conclusion
Data cleaning is a critical step in data integration and analysis. With DataStage and the integration of ChatGPT-4, data cleaning becomes more efficient and accurate. The powerful data profiling capabilities of DataStage, combined with the language model expertise of ChatGPT-4, greatly enhance the overall data cleaning process.
By leveraging the capabilities of these technologies, organizations can ensure that their datasets are error-free, consistent, and ready for analysis. This leads to more reliable insights and better business decisions based on accurate data.
Comments:
Thank you all for taking the time to read my article on ChatGPT Revolutionizing DataStage! I'm excited to hear your thoughts and engage in a discussion.
Great article, Jessie! AI conversation technology like ChatGPT has indeed revolutionized the field of data transformation. It helps automate tasks and improves efficiency in handling large datasets.
I completely agree, Robert! The conversational interface of ChatGPT makes it easy for non-technical users to interact with data and perform complex transformations. It's user-friendly and powerful.
I agree with Robert. ChatGPT has greatly simplified the process of transforming data. It's impressive how AI can now engage in meaningful conversations, giving us the ability to interact with data in a more intuitive way.
Exactly, Anna! This technology bridges the gap between data experts and those who need to work with data. It allows for collaboration and ensures that data is transformed accurately and efficiently.
I've been using ChatGPT for a while now, and it's been a game-changer. It makes the tedious task of data transformation more enjoyable while streamlining the entire process. Highly recommended!
I agree with both of you! The natural language capabilities of ChatGPT make it feel like you're having a conversation with a human expert. It's a breakthrough in data transformation.
I can't imagine going back to manual data transformation after using ChatGPT. It has saved me so much time and effort. Plus, it's continuously learning, which means it gets better over time!
While ChatGPT is undoubtedly impressive, I believe we should also consider the potential risks associated with relying too heavily on AI for data transformation. What are your thoughts on that?
Oliver, you raise a valid point. While AI conversation technology has its advantages, we must also be cautious about relying solely on automation. It's important to strike a balance between human expertise and AI assistance.
Thanks for considering my perspective, Jessie. Striking the right balance between human and AI involvement is crucial for effective and reliable data transformation.
Indeed, Oliver. Finding the right balance between human expertise and AI assistance is key to leveraging the full potential of technology while mitigating potential risks.
I couldn't agree more, Jessie. The power of human judgment and the capabilities of AI conversation technology can complement each other to achieve optimal results.
Finding that balance is crucial, Jessie. It's about capitalizing on AI's efficiency while retaining human expertise, judgement, and accountability in critical data transformation processes.
I completely agree, Oliver. AI should be seen as a valuable tool in our data transformation toolkit, augmenting our capabilities and helping unlock new insights efficiently.
Absolutely, Emily. The synergy between AI and human expertise drives innovation and ensures accurate and efficient data transformation in today's constantly evolving landscape.
Well said, Daniel. AI conversation technology can learn from human expertise and adapt to evolving data transformation needs, enabling us to push the boundaries of what's possible.
That's true, Emily. Accessibility is vital in empowering users to leverage the benefits of data transformation efficiently, regardless of their technical background.
Exactly, Anna. The democratization of data transformation empowers individuals across various domains to make more informed decisions based on valuable insights.
I agree with Oliver. AI can make mistakes or misinterpret certain data scenarios. Human oversight is crucial to ensure the accuracy and integrity of the transformed data.
You're right, Ethan. While AI is powerful, the human factor is indispensable. Human expertise can identify data patterns and nuances that AI might miss, leading to more accurate transformations.
I completely agree, Robert. Humans bring a level of intuition and critical thinking that complements the power of AI, ensuring the best possible outcomes in data transformation tasks.
Absolutely, Ethan. AI should be seen as a tool to assist and enhance human capabilities, rather than a substitute. The human touch is vital in data transformation to ensure accuracy and reliability.
Well said, Alice. The collaboration between humans and AI technology like ChatGPT opens up new possibilities in data transformation, ensuring accuracy, and improving efficiency.
I've encountered a few instances where ChatGPT struggled to understand complex data transformations. While it's a powerful tool, human intervention and expertise are still necessary in certain cases.
I've had similar experiences, Timothy. It's important to thoroughly review and validate the output of AI-powered transformation processes to catch any potential errors.
I completely agree, Maria. Human review and validation are essential to maintain data quality and address any potential inaccuracies. AI conversation technology like ChatGPT can augment our capabilities but shouldn't replace them entirely.
Thank you, Daniel, Alice, and Anna, for your valuable input. Collaboration between humans and AI is indeed crucial to ensure accurate and efficient data transformation.
ChatGPT has truly transformed the way we interact with data and perform data transformations. It has made the process more efficient and accessible to a wider range of users.
Collaboration between humans and AI is the way forward. It allows us to leverage the strengths of each while mitigating the limitations encountered in complex data transformation scenarios.
Absolutely, Timothy. The key is to work together seamlessly to ensure the best outcomes and drive innovation in the field of data transformation.
Well put, Anna. Collaboration paves the way for advancements in the field, enabling us to handle ever-increasing amounts of data and create meaningful insights.
Well said, Timothy. Collaboration enables us to tackle complex data transformation challenges effectively, making the most of AI technology while maintaining data integrity and reliability.
Adding AI conversation technology to DataStage has significantly improved my productivity. It simplifies the process, and the conversational interface makes it more accessible to users.
The collaboration aspect is critical. ChatGPT can assist non-experts in executing complex transformations, but data experts are necessary to ensure quality and alignment with the desired outcome.
The accessibility aspect is often overlooked. ChatGPT empowers users without technical backgrounds to engage with data effectively, democratizing data transformation to a broader audience.
Collaboration between humans and AI also fosters innovation. By combining our expertise with the capabilities of AI, we can unlock new possibilities and deliver transformative results.
Absolutely, Alice. The synergy between human knowledge and AI capabilities creates a powerful force for driving meaningful change and achieving better outcomes in data transformations.
Absolutely, Alice. The combination of human understanding and AI's ability to process vast amounts of data presents us with unprecedented opportunities for innovation.
Well said, Alice. AI conversation technology amplifies our capabilities and empowers us to address complex data transformation challenges with greater efficiency.
ChatGPT's intuitive interface allows users to focus on the data and the business context, without getting overwhelmed with technical details. It makes the entire process more efficient.
I couldn't agree more, Grace. ChatGPT's conversational approach eliminates the technical barriers and allows users to focus on the data itself, leading to faster and better decision-making.
Absolutely, Grace. It's like having a data expert at your fingertips. ChatGPT simplifies the complexities of data transformation for users, irrespective of their technical background.
Well said, Daniel. AI conversation technology makes data transformation more accessible and bridges the gap between technical and non-technical users.
Exactly, Emily. AI conversation technology democratizes data transformation, making it accessible to a wide range of users and fostering collaboration between different stakeholders.
I agree completely, Daniel. ChatGPT's user-friendly interface breaks down barriers and enables effective collaboration between technical and non-technical users in data transformation tasks.
Collaboration between humans and AI brings improvements from both ends, making it a win-win situation. It enhances our capabilities while expanding the potential scope of data transformation.
Exactly, Sophia. The collaboration ensures that data transformation is not limited by technical know-how while maintaining accuracy and trust in the final output.
Correct, Timothy. Human intervention becomes essential in handling complex situations where AI struggles. It ensures the accuracy and reliability of the transformed data.
Striking the right balance between human expertise and AI assistance is crucial for effective and reliable data transformation.
Collaboration enables us to leverage technology effectively and adapt to the growing demands of data transformation. It's an exciting time to be in this field!
The impact of AI conversation technology in data transformation cannot be overstated. It's reshaping the way we work with data, opening doors to new possibilities.
Striking a balance between human expertise and AI assistance in data transformation is crucial to take full advantage of the technology while ensuring accuracy and reliability.
Absolutely, Alice. Data transformation must be accessible to all stakeholders, regardless of their technical background. AI conversation technology empowers users to engage effectively in the process.
Exactly, Alice. We must leverage AI's capabilities while ensuring human intervention to address any potential limitations AI might have in complex data transformation scenarios.
Absolutely, Oliver. By democratizing data transformation, we unlock the potential of a more data-driven future, where insights are available to a broader audience.
Absolutely, Maria. The human touch is essential to ensure the reliability and accuracy of the transformed data. AI should be a helpful aid, not the sole decision-maker.
Correct, Maria. The democratization of data transformation ensures that valuable insights are not limited to a select few but are accessible to stakeholders across the organization.
Absolutely, Emily. Democratizing data transformation democratizes the decision-making process, ensuring that insights are available to those who need them to drive positive change.
I completely agree, Oliver. Data transformation should no longer be confined to specialists. With AI conversation technology, it empowers everyone to leverage the potential of data.
Well said, Sophia. The democratization of data transformation allows us to tap into the collective intelligence of diverse individuals and drive innovation through collaboration.
Indeed, Daniel. AI conversation technology empowers non-experts to actively participate in the data transformation process, breaking down barriers to entry and enhancing collaboration.
Absolutely, Daniel. Democratizing data transformation leads to better decision-making, improved efficiency, and unleashes the full potential of data-driven insights.
Completely agree, Sophia. The era of democratized data transformation opens up opportunities for both individuals and organizations to leverage data for growth and innovation.
You're right, Alice. Maintaining a balance between AI assistance and human expertise is vital to ensure accurate and reliable data transformation for decision-making.
Couldn't agree more, Daniel. ChatGPT's conversational approach simplifies the complexities of data transformation, empowering users to handle data with confidence.
Well said, Grace. ChatGPT's conversational interface makes interacting with data transformation more accessible, improving user experience and efficiency.
Well said, Daniel. The democratization of data transformation democratizes decision-making. It empowers individuals from various disciplines to contribute effectively to the process.
Collaboration between humans and AI technology enables us to create an ecosystem where data transformation can be executed efficiently, accurately, and at scale.
Well said, Grace. The combination of human expertise and AI capabilities sets the stage for ongoing innovation and advancement in the field of data transformation.
The ease of use and accessibility of ChatGPT make it a valuable tool for businesses across industries. It empowers users to make data-driven decisions efficiently.
Human expertise helps in guiding AI technology to achieve optimal outcomes in data transformation and derive meaningful insights from the data analyzed.
Striking the right balance between human expertise and AI assistance is crucial for achieving accurate and reliable data transformation, while harnessing the full potential of AI technology.
Exactly, Alice. ChatGPT's user-friendly approach enables wider adoption and enables businesses to unlock the value of their data effortlessly.
Collaboration with AI conversation technology like ChatGPT drives efficiency and helps businesses leverage their data to make informed decisions. It's a game-changer!
Collaboration between human experts and AI technology strengthens data transformation practices, ensuring quality, reliability, and accuracy in the results derived from transformed data.
Indeed, Timothy. Human expertise in conjunction with AI technology enables us to overcome challenges and deliver meaningful insights through data transformation.
Exactly, Maria. The collaboration between humans and AI technology adds value and helps us uncover insights that can drive growth and innovation.
Collaboration enables the best of both worlds: the efficiency and scalability of AI technology combined with the expertise and creativity of human data practitioners.
Absolutely, Robert. The collaboration between humans and AI technology allows us to combine the strengths of each and drive innovation in data transformation.
Indeed, Daniel. Collaboration between humans and AI technology drives exponential progress in data transformation, offering more accurate and valuable outcomes.
Absolutely, Sophia. The partnership between humans and AI technology ensures that data transformation efforts lead to meaningful insights and enable informed decision-making.
Exactly, Sophia. The collaborative approach enables us to tap into the full potential of data transformation and make more informed decisions based on accurate insights.
Well said, Daniel. AI conversation technology doesn't replace human expertise but amplifies it, enabling us to achieve more accurate and impactful data transformation outcomes.
Well put, Robert. The synergy between human and AI capabilities amplifies our collective potential and accelerates data-driven decision-making.
Balance is key. The combined efforts of humans and AI conversation technology ensure that data transformation yields accurate, reliable, and actionable results.
Collaboration powers innovation. By leveraging AI conversation technology, we can enhance our capabilities and deliver transformative results in data transformation.
Collaboration between humans and AI conversation technology like ChatGPT unlocks new possibilities and accelerates progress in the field of data transformation.
Collaboration between humans and AI technology is not just about efficiency, but also about enabling creativity and exploring uncharted territories in data transformation.
Absolutely, Timothy. The collaboration fuels innovation, allowing us to push the boundaries of what's possible in data transformation and uncover new insights.
Absolutely, Timothy. The combination of human creativity and AI capabilities in data transformation drives innovation and fuels progress across industries.
I completely agree, Emily. Collaboration between humans and AI harnesses the power of both to deliver impactful data transformation outcomes.
Exactly, Sophia. Collaboration enables us to leverage AI's capabilities and human expertise to drive innovation, accuracy, and efficiency in data transformation.
The collaborative efforts between humans and AI conversation technology bring us closer to unlocking the full potential of data and transforming it into actionable insights.
Collaboration empowers us to leverage AI conversation technology like ChatGPT in data transformation, opening doors to new insights and driving innovation in various domains.
Striking the right balance between human expertise and AI assistance is crucial to ensure accurate data transformation that is aligned with business objectives.
Balance is key, Oliver. The combination of AI assistance and human expertise results in accurate and reliable data transformation, empowering organizations to leverage their data.
Well said, Alice. Achieving the right balance between AI technology and human judgment is vital to ensure effective and impactful data transformation outcomes.
Indeed, Oliver. The combination of AI technology and human expertise allows us to unlock the full potential of data transformation and drive positive change.
Collaboration between humans and AI in data transformation opens doors to innovation, efficiency, and accurate insights for informed decision-making.
Thank you all for taking the time to read my article! I'm excited to hear your thoughts on how ChatGPT is revolutionizing DataStage. Feel free to share your opinions and ask any questions you may have.
Great article, Jessie! It's amazing to see how AI conversation is transforming technology. ChatGPT seems like a powerful tool. Are there any specific industries where it has shown exceptional performance?
Thank you, Hannah! ChatGPT has shown promising results in industries like customer support, content creation, and even legal research. Its ability to generate human-like responses makes it versatile for various applications.
Impressive article, Jessie! AI conversation certainly has the potential to revolutionize DataStage. I'm curious about the challenges in training ChatGPT. Could you elaborate on that?
Thanks, Ethan! Training ChatGPT involves a massive amount of data and computational resources. The model is trained using unsupervised learning methods, but ensuring it produces safe and unbiased responses can be a challenging task.
Great article, Jessie! ChatGPT seems like a groundbreaking tool for fostering more interactive conversations. Are there any limitations to its capabilities that we should be aware of?
Thank you, Megan! While ChatGPT has made significant advancements, it can sometimes generate incorrect or nonsensical answers. Additionally, it might not always ask clarifying questions when faced with ambiguous queries. Ongoing research aims to address these limitations.
Great post, Jessie! AI conversation has vast potential. I'm curious about the ethical considerations surrounding the use of ChatGPT in DataStage. How do we ensure unbiased outcomes?
Thank you, Oliver! Ensuring unbiased outcomes is a crucial consideration. OpenAI has employed safeguards to reduce biases during ChatGPT's training. They also seek external input, perform audits, and encourage feedback from the community to identify and address any potential biases.
Excellent article, Jessie! ChatGPT is undoubtedly transforming the way we interact with technology. Do you think it will replace traditional chatbots?
Thanks, Sophia! While ChatGPT offers more natural and dynamic conversations, it might not entirely replace traditional chatbots. Different use cases will determine which approach is most suited, and traditional chatbots can still excel in certain scenarios.
Insightful article, Jessie! I'm excited about ChatGPT's potential. How can businesses effectively integrate this technology with their existing systems?
Thank you, Daniel! Integrating ChatGPT involves developing appropriate APIs or chat interfaces. Businesses can leverage its conversational capabilities by integrating it into their customer support platforms, content creation workflows, or other data-driven processes.
Great read, Jessie! AI conversation is a fascinating field. How do you see the future of AI conversation technology evolving?
Thanks, Liam! The future of AI conversation holds immense potential. We can expect more refined models, increased customization options, and further advancements in areas like multi-turn conversations, context understanding, and better control over generated responses. It's an exciting time!
Impressive work, Jessie! ChatGPT's impact on DataStage is evident. Are there any risks associated with the adoption of AI conversation at such a large scale?
Thank you, Chloe! There are risks to consider, such as misuse of AI-generated content or potential security vulnerabilities. Responsible deployment, strong governance, and continuous monitoring are essential to mitigate these risks and ensure the safe and ethical use of AI conversation models at scale.
Great article, Jessie! ChatGPT's ability to transform conversations is incredible. Are there any known limitations in terms of language support?
Thanks, Emily! Currently, ChatGPT primarily supports English. However, OpenAI has plans to expand its language support, allowing more users worldwide to benefit from the technology.
Informative article, Jessie! AI conversation is an exciting domain. What are some potential privacy concerns associated with the use of ChatGPT?
Thank you, Isaac! Privacy concerns can arise when AI conversation models handle sensitive user information. It is crucial to implement robust privacy measures, such as data anonymization, user consent management, and secure infrastructure, to safeguard user privacy and data confidentiality.
Insightful post, Jessie! ChatGPT is making significant strides. Can you shed some light on how it handles biases?
Thanks, Ella! OpenAI actively works towards reducing biases in ChatGPT's responses during training. They employ a two-step approach: pre-training on a large dataset to learn from internet text and fine-tuning using a narrower dataset with human reviewers who follow guidelines provided by OpenAI.
Great article, Jessie! The potential of AI conversation is immense. Could you provide some insights into the process of fine-tuning ChatGPT?
Thank you, Aiden! Fine-tuning involves using a dataset generated with human reviewers participating in a feedback loop with OpenAI. These reviewers follow guidelines to review and rate possible model outputs. This iterative process helps narrow down the model's behavior and align it with human values.
Impressive work, Jessie! ChatGPT has immense potential for driving innovation. Could you share some interesting use cases that have emerged from its deployment?
Thank you, Grace! Some interesting use cases include enhancing customer support by automating responses, improving content creation workflows by assisting writers with creative suggestions, and aiding educational platforms to provide personalized tutoring. The possibilities are vast!
Excellent article, Jessie! The impact of AI conversation on DataStage is commendable. Are there any specific guidelines or best practices to follow while using ChatGPT?
Thanks, Ava! OpenAI provides guidelines for safe and responsible use of ChatGPT. These guidelines help ensure the system produces useful and respectful outputs. Businesses and users should familiarize themselves with these guidelines to leverage the technology effectively.
Great read, Jessie! ChatGPT has the potential to revolutionize interactions. Are there any research challenges in the field of AI conversation that still need to be addressed?
Thank you, Carter! Some research challenges include improving model control to avoid generating malicious or harmful content, enhancing robustness to handle inputs that aren't well-formed questions, and developing methods to better handle ambiguous or out-of-scope queries.
Insightful article, Jessie! ChatGPT is transforming the way we interact with technology. Could you share some of the potential risks associated with AI conversation systems?
Thanks, Liam! Risks include the propagation of misinformation or fake news, offensive or inappropriate content generation, and potential exploitation by malicious actors. Proper safeguards and responsible deployment are vital to mitigate these risks effectively.
Great article, Jessie! ChatGPT's ability to revolutionize DataStage is remarkable. Are there any language-specific challenges it faces in understanding and generating accurate responses?
Thank you, Noah! While ChatGPT performs well with English, it can struggle with nuanced language understanding and generating responses in certain contexts. Extending its capabilities to other languages and improving language-specific nuances are areas that researchers are actively working on.
Insightful post, Jessie! ChatGPT seems to offer great potential. Are there any limitations in its ability to understand context and generate coherent responses?
Thanks, Sophia! While ChatGPT has improved context understanding, it can still occasionally lose track of context, resulting in slight deviation from coherency. This is an active area of research, and future updates will focus on enhancing contextual understanding capabilities.
Informative article, Jessie! ChatGPT's impact on DataStage is impressive. How does it handle user queries for information it may not have been trained on?
Thank you, Benjamin! When faced with queries it hasn't been trained on, ChatGPT tries to generate a plausible response based on its training and available knowledge. However, it may not always ask clarifying questions, leading to potential incorrect or speculative answers.
Great post, Jessie! ChatGPT is transforming the world of DataStage. How can businesses leverage this technology to enhance their decision-making processes?
Thanks, David! Businesses can leverage ChatGPT's conversational abilities to gather insights, support decision-making processes, and assist in tasks such as market research, competitive analysis, or data exploration. Its natural language processing capabilities offer a flexible and interactive interface for smarter decision-making.
Great article, Jessie! ChatGPT's impact on DataStage is extraordinary. What are the future possibilities for AI conversation models beyond ChatGPT?
Thank you, Lily! Future AI conversation models may expand to handle specialized domains, improve multi-turn conversation flow, and enhance personalization capabilities. We can also expect more seamless integration with existing systems, enabling users to have natural language interactions with various applications and services.
Excellent article, Jessie! ChatGPT's potential for DataStage is immense. How do you envision its role in human-computer collaboration?
Thanks, Daniel! ChatGPT can play a crucial role in improving human-computer collaboration by providing dynamic and interactive interfaces for data exploration, system configuration, or problem-solving. Its ability to generate human-like responses fosters a more intuitive interaction between users and machines.
Impressive work, Jessie! ChatGPT is revolutionizing the world of DataStage. How can developers contribute to the improvement and expansion of AI conversation models?
Thank you, Hannah! Developers can contribute to the improvement of AI conversation models by providing feedback, reporting potential issues or biases, and testing the systems in various contexts. Their insights play a crucial role in identifying areas for improvement and shaping the direction of future research and development.
Great read, Jessie! ChatGPT's potential is impressive. How can organizations ensure transparency and accountability while using AI conversation systems?
Thanks, Olivia! Organizations can ensure transparency and accountability by clearly disclosing when users are interacting with AI conversation systems, making it evident that the responses are AI-generated. They should also showcase responsible data collection practices, following legal and ethical guidelines to foster trust and accountability.
Excellent article, Jessie! ChatGPT holds great potential for DataStage. How does it handle situations that require the system to ask clarifying questions?
Thank you, Ethan! While ChatGPT can sometimes ask clarifying questions, it might not always do so. This can lead to inaccurate responses when faced with ambiguous queries. Researchers are actively exploring methods to improve the system's capability to ask informative questions when needed.