ChatGPT: Revolutionizing Roof Damage Assessment with AI Technology
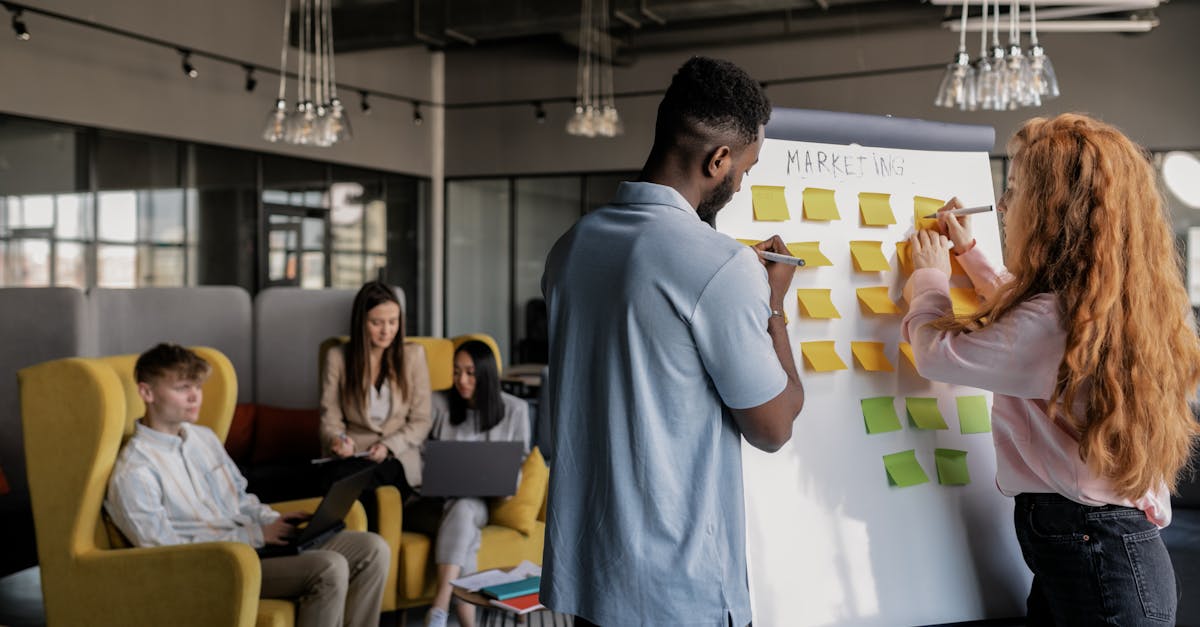
Introduction
Roof damage assessment is a crucial task in the field of building maintenance and inspection. Traditional assessment methods often involve extensive manual inspection and evaluation, which can be time-consuming and subjective. In recent years, technology has played a vital role in simplifying this process. One such technology is the use of GPT-4 (Generative Pre-trained Transformer 4), an advanced language model that can be utilized in preparing reports based on the analysis of drone footage for roof damage assessment.
How Does It Work?
GPT-4 is a state-of-the-art language model developed by OpenAI. It uses deep learning techniques to understand and generate human-like text. When it comes to roof damage assessment, GPT-4 can be trained on a vast amount of data, including aerial images and corresponding damage evaluations.
By analyzing drone footage of roofs, GPT-4 can identify various types of damage, such as cracks, leaks, missing shingles, and structural issues. It can provide accurate evaluations by contextualizing the captured images and comparing them to its learned patterns from the training data.
Advantages of GPT-4 in Roof Damage Assessment
GPT-4 offers several advantages in the field of roof damage assessment:
- Efficiency: GPT-4 can process a large amount of drone footage data quickly, significantly reducing the time required for manual inspection.
- Accuracy: With its advanced analysis capabilities, GPT-4 can provide accurate assessments based on patterns learned from the training data.
- Consistency: Unlike human inspectors, GPT-4 provides consistent evaluations, eliminating subjective biases.
- Cost-effectiveness: By automating the roof damage assessment process, GPT-4 can help save costs associated with manual inspections.
Limitations and Future Potential
While GPT-4 offers significant benefits, it is important to acknowledge its limitations. The technology heavily relies on the quality of the training data and may struggle in analyzing complex or ambiguous cases. Further advancements in training data and the integration of additional visual analysis techniques may overcome these current limitations.
In the future, GPT-4 could be enhanced to incorporate real-time data from drone footage to provide immediate assessments during emergency situations, such as after natural disasters. Additionally, improvements in data collection and integration could lead to a more comprehensive analysis of roof damage, including predictive maintenance capabilities.
Conclusion
Roof damage assessment using GPT-4 and drone footage analysis presents a promising solution to traditional manual inspection methods. The technology offers efficiency, accuracy, consistency, and cost-effectiveness. While there are limitations, continued advancements and integration with real-time data could bring further potential in the field of roof damage assessment. Overall, GPT-4 provides an exciting opportunity to streamline and improve the assessment process, benefiting both building owners and inspectors.
Comments:
Thank you all for reading my article on ChatGPT and its application in roof damage assessment! I'm excited to hear your thoughts and opinions.
Great article, Dash! AI technology has the potential to revolutionize so many industries, and roofing assessment is definitely one of them. It can save time and resources while improving accuracy.
I agree, Mike! ChatGPT can analyze images and identify damage much faster than human inspectors. It would be interesting to see how it performs in real-world scenarios.
As someone working in the roofing industry, I'm curious about the integration challenges. How would ChatGPT be implemented in existing assessment processes?
Good question, Emily! Integrating AI into existing processes can sometimes be a complex task. In the case of ChatGPT for roof damage assessment, it would require developing a user-friendly interface and training the model with a large dataset of annotated images.
I'm a bit skeptical about AI completely replacing human expertise. Roof damage assessment requires experience and knowledge that AI might not possess. Wouldn't it be better to use AI as a support tool for human inspectors?
That's a valid concern, Jerry. AI should indeed be used as a support tool rather than a replacement. ChatGPT can help human inspectors analyze large volumes of data quickly and accurately, enhancing their capabilities.
I'm impressed by the potential of ChatGPT in roof damage assessment. Not only can it detect damages, but it can also classify them, allowing for better prioritization of repairs based on severity. This could save a lot of money for homeowners and insurance companies.
You're absolutely right, Sara! ChatGPT's capability to detect and categorize damages can be invaluable in determining the urgency and extent of repairs needed, making the process more efficient.
I understand the advantages of using AI in roof damage assessment, but what about the cost? Implementing AI technology can be expensive for small businesses or individual homeowners.
Good point, John. Implementing AI can come with upfront costs, but in the long run, it can lead to cost savings through increased efficiency and accuracy. As the technology advances and becomes more accessible, we can expect the costs to reduce as well.
In addition to cost, there are also ethical considerations. AI algorithms should be unbiased and reliable. How can we ensure ChatGPT doesn't inadvertently discriminate or provide inaccurate assessments?
Ethical considerations are crucial, Mike. It's essential to train AI models with diverse datasets and regularly monitor their performance to mitigate biases. Human oversight and validation can also help ensure reliable results. Transparency is key.
I can see the potential benefits of ChatGPT, but I'm concerned about its limitations. Are there any specific types of roof damage that it struggles to identify? And what about complex roof structures?
Valid concerns, Emily. Currently, ChatGPT performs well in identifying common types of roof damage, but it may struggle with more rare or unusual cases. Complex roof structures might require additional training and customization to obtain accurate results.
Dash, you mentioned the need for training the model with annotated images. How time-consuming is that process? And how frequently does the model require retraining?
Training the model can indeed be time-consuming, Jerry. It requires a significant amount of annotated data and computational resources. The frequency of retraining depends on various factors like the evolution of roof damage patterns and the availability of new data.
I can imagine ChatGPT being useful not only for roof damage assessment but also for preventive maintenance. It can help identify potential weak spots before they turn into significant problems.
Absolutely, Sara! ChatGPT can analyze past data and identify patterns that indicate areas prone to damage. This proactive approach can save homeowners from more extensive repairs and increase the lifespan of their roofs.
Dash, do you think ChatGPT can be applied to other types of property assessments aside from roof damages?
Indeed, Mary! AI technologies like ChatGPT have the potential to be applied to various property assessments, such as detecting structural issues, assessing insulation efficiency, or even evaluating energy consumption in buildings.
While the benefits of AI in roof damage assessment are evident, we shouldn't forget about the human element. Building relationships and trust with customers is vital in the roofing industry. AI should support, not replace, human inspectors.
I agree, John. AI should augment human capabilities, not replace them. Combining the expertise of humans with the efficiency and accuracy of AI can lead to better outcomes and improved customer satisfaction.
Dash, what are some other potential applications where ChatGPT or similar AI technology could be utilized?
Great question, Emily! ChatGPT's natural language processing capabilities can be useful in customer support chatbots, virtual personal assistants, or even content creation. Its potential applications stretch across various industries.
Dash, do you think there will be regulatory hurdles for implementing AI in the roofing industry?
Regulatory challenges are a possibility, Mike. As AI becomes more prevalent, it's essential to have guidelines and standards in place to ensure ethical and responsible use. Collaboration between industry experts and policymakers will be crucial.
I appreciate the potential efficiency and accuracy improvements, but let's not forget the potential job displacement caused by AI. How can we address the concerns of those whose livelihoods depend on roof damage assessment jobs?
You raise an important point, Jerry. As technology evolves, new jobs can emerge alongside automation. It's essential to invest in retraining and upskilling programs to help workers transition into roles that require human expertise alongside AI support.
Roof damage assessment is critical after natural disasters. Do you think ChatGPT can be deployed quickly for large-scale assessment in such situations?
In emergency situations, speed is of the essence, Sara. Deploying ChatGPT for large-scale assessments would require pre-trained models and access to reliable image data. Adequate preparation can help leverage AI effectively during disaster recovery.
Dash, what challenges do you see in convincing insurance companies to adopt AI technology for roof damage assessment?
Insurance companies prioritize accuracy and efficiency in claim assessments, John. Demonstrating the benefits of AI, including increased accuracy and faster assessments, along with cost savings, can help in convincing them of its value.
How can homeowners be assured of the reliability and accuracy of ChatGPT's assessments if they are unfamiliar with the technology?
Building trust is essential, Emily. Homeowners should be provided with transparent reports that include details about the technology used, data sources, and validation processes. Educating homeowners about the benefits and limitations of ChatGPT can also create awareness and transparency.
Dash, are there any concerns regarding data privacy and security while using AI technology like ChatGPT?
Data privacy and security are top priorities, Mary. While using ChatGPT or any AI technology, it's crucial to comply with privacy regulations, ensure secure data handling, and implement robust security measures to protect both the users and their data.
Beyond assessments, can AI play a role in optimizing roof repair and replacement processes, Dash?
Absolutely, Mike! AI can help optimize repair and replacement processes by analyzing historical data, identifying common issues, and suggesting cost-effective solutions. It can assist in decision-making, resource allocation, and overall project management.
Dash, what steps can be taken to ensure that AI technology like ChatGPT remains unbiased and doesn't reinforce existing societal inequalities?
Addressing bias in AI is critical, Jerry. Diverse and representative training data, model audits, and involving ethicists and social scientists in the development process can help minimize biases and ensure fairness in AI assessments.
With ChatGPT's potential to recognize patterns, could it also be used to identify the causes of roof damage, such as poor construction or faulty materials?
Certainly, Sara! By analyzing large amounts of roofing data, ChatGPT can identify recurring issues that may be attributed to poor construction or faulty materials. This information can be valuable for improving industry practices and avoiding future damages.
Dash, how would you address concerns regarding AI technology malfunctioning or providing incorrect assessments?
Ensuring the reliability of AI assessments is vital, Emily. Implementing quality control measures, validating the outputs with human experts, and continuously monitoring the system's performance can help minimize the possibility of malfunctions and incorrect assessments.
AI is advancing rapidly. Can we expect more sophisticated AI models than ChatGPT specifically designed for roof damage assessment in the near future?
Absolutely, Mary! AI research and development are ongoing, and we can expect more specialized models with improved accuracy and performance in roof damage assessment. The field is evolving, and there's exciting potential for further advancements.
Dash, what are some steps that industry professionals can take to adapt to the implementation of AI technologies like ChatGPT in their workflows?
Adapting to AI technologies involves upskilling, embracing change, and being open to collaboration with AI systems. By focusing on leveraging the unique strengths of humans and AI together, industry professionals can enhance their work and deliver better results.
Thank you all for your insightful comments and questions! It was a pleasure discussing the potential of ChatGPT and AI technology in roof damage assessment with you. Remember, the future is about human-AI collaboration. Let's embrace it!