ChatGPT: Revolutionizing Technology with its 'Heavy Lifting' Capabilities
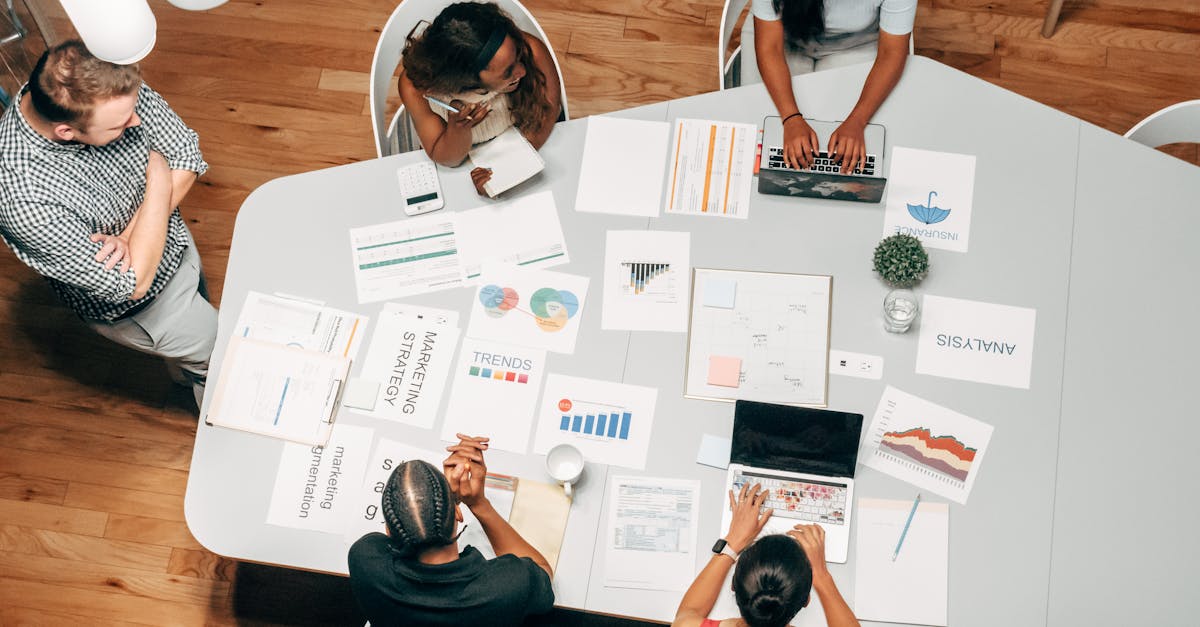
Heavy lifting technology's landscape is witnessing an unprecedented revolution, rooted in the rise of massive datasets processing and AI-based solutions. One of the most intriguing implications of this dawning era is the potential of employing advanced language models like ChatGPT-4 in predictive maintenance. The invaluable insights gained from data analysis are more than a mere industrial luxury; they are fast becoming a necessity for competitive companies vying for a place in the future of heavy lifting technologies.
The manufacturing industry has long been in pursuit of technological advancements that would increase machine reliability and reduce unnecessary costs. The ability to predict machine failures before they occur is a ground-breaking game-changer for heavy lifting technologies. Predictive maintenance, as it's aptly coined, allows industries to anticipate and rectify errors, reduce downtime, and increase overall productivity. The implementation of such advanced predictive systems can mark a pivotal turning point in heavy lifting technology.
ChatGPT-4's potential in predictive maintenance is a fascinating exploration on its own. As an AI language model developed by Open AI, it has been trained on vast amounts of internet text. It can generate relevant and creative responses based on the prompts given. In the context of predictive maintenance, ChatGPT-4 can be applied to analyze data from machinery sensors and predict patterns that may indicate impending machinery failure.
Detailed sensor data analysis is critical in heavy lifting technology. The data can range from vibrational frequencies to temperature readings, all of which can offer significant insights about the machine's health. Given the enormous data complexity, AI models like ChatGPT-4 offer a fitting solution. It can process large quantities of data in real-time, discern patterns and anomalies, and consequently predict potential machine failures.
The Benefits of Applying ChatGPT-4 in Predictive Maintenance
Upgrading to an AI-based predictive maintenance from a typically reactive or preventative approach delivers several striking benefits in heavy lifting technologies.
1. Decreased Downtime: By analyzing sensor data in real-time, ChatGPT-4 can flag abnormalities and predict potential issues well ahead of time, preventing sudden machine breakdowns. Regular rerouting from reactive to proactive monitoring significantly reduces unexpected downtime, thus increasing productivity.
2. Cost Reduction: Predictive maintenance enabled by AI requires less frequent machinery checks and less manual intervention. By anticipating problems and solving them before they cause breakdowns, maintenance costs can be kept minimal. The use of predictive maintenance tools like ChatGPT-4 can lead to significant cost savings.
3. Prolonged Equipment Life: Proactive repair and replacement of parts arising from predictive maintenance tend to prolong the heavy machinery's lifespan. Over time, productive machine life can lead to a sizeable return on the initial investment in the machinery.
The Future of Heavy Lifting and Predictive Maintenance with GPT-4
As artificial intelligence continues to evolve and make strides in various industries, heavy lifting technologies will likely see marked growth in their ability to conduct efficient and cost-effective predictive maintenance.
The integration of ChatGPT-4 and similar AI models for predictive maintenance is just the beginning of the journey. As technology advances and these AI models grow more refined, we will likely see a multitude of applications across a broad range of sectors. From heavy lifting to manufacturing, transportation to utilities, AI-based predictive maintenance is set to become the new norm.
In conclusion, the potent combination of heavy lifting technologies, predictive maintenance strategies, and advanced AI tools like ChatGPT-4 paint a promising future. Not only does it bolster the industrial sector's efficiency, but it also paves the way for smart manufacturing and the wider adoption of AI in industrial applications.
Comments:
Thank you all for taking the time to read my post on ChatGPT! I'm excited to hear your thoughts and opinions about its 'heavy lifting' capabilities.
Great article, Randall! ChatGPT seems like a promising technology. Can you share any specific applications where its 'heavy lifting' capabilities excel?
Maria, ChatGPT's 'heavy lifting' capabilities can be particularly valuable in tasks like drafting emails, writing code, answering factual questions, or providing detailed explanations.
Randall, thank you for sharing those specific applications where ChatGPT's capabilities excel. The potential for productivity improvement is impressive!
Randall, thank you for elaborating on the specific applications of ChatGPT's 'heavy lifting' capabilities. Those use cases would definitely save a lot of time and effort!
I'm really impressed with ChatGPT's capabilities! However, I'm curious about the limitations it might have. Are there any scenarios where it struggles?
Ryan, while ChatGPT has seen significant improvements, it can sometimes produce incorrect or nonsensical responses. It may also be sensitive to input phrasing and can generate output that sounds plausible but is irrelevant or inaccurate.
Ryan, one important limitation to consider is that ChatGPT might not always ask clarifying questions when faced with ambiguous queries or insufficient information. This can sometimes lead to inaccurate responses.
Melissa, that's a valid point. It's crucial to understand the limitations of ChatGPT, especially in contexts where ambiguous or incomplete queries are common.
Melissa, aside from the limitations, it's impressive to see the progress made in natural language processing. ChatGPT can still be highly useful in various applications, as long as users are aware of its capabilities and limitations.
Brian, absolutely! Understanding the strengths and limitations of AI systems like ChatGPT allows us to leverage them effectively while being aware of their boundaries.
Brian, as you rightly said, ChatGPT can be a valuable tool across various applications as long as we are mindful of its capabilities and rely on expert verification when needed.
Sophia, exactly! Understanding and acknowledging the limitations of ChatGPT can help us make informed decisions and utilize AI systems effectively.
Interesting article, Randall! How does ChatGPT handle complex and technical questions or discussions?
Laura, ChatGPT is designed to handle complex and technical questions to some extent but might lack specific domain knowledge. It can provide general explanations and understand high-level concepts but might miss finer details.
Of course, Laura, ChatGPT can certainly handle complex discussions, but it's always good practice to cross-verify the information by consulting human experts in technical or specialized areas.
Sophia, it's always important to critically evaluate the information received from AI systems, especially when accuracy is crucial. ChatGPT can provide a starting point, but human verification is key!
Laura, while ChatGPT can handle complex questions, it's always advisable to consult domain experts for critical or specific technical discussions. It complements human expertise but doesn't replace it.
Randall, great job explaining the technology behind ChatGPT! Have there been any notable real-world applications of this technology yet?
Paul, ChatGPT has been used in areas like drafting content, brainstorming ideas, and even providing programming help. While there have been promising results, there's still ongoing research to improve its limitations and biases.
I appreciate the transparency, Randall. It's important to understand the limitations and potential pitfalls of AI technologies like ChatGPT.
Randall, do you think ChatGPT could be used as a virtual personal assistant, similar to Siri or Alexa, but with more advanced capabilities?
Emily, while it's possible to explore ChatGPT as a virtual assistant, it currently lacks the ability to remember prior information or maintain a coherent personality over multiple interactions like Siri or Alexa. But it's an area of active development!
Randall, are there any privacy concerns when using ChatGPT? How does OpenAI address them?
James, privacy is a significant concern. OpenAI actively aims to reduce biases and strives to make ChatGPT customizable by users while maintaining safeguards to prevent malicious use. OpenAI is forthcoming about limitations and encourages user feedback to improve the system.
Randall, I'm worried about the potential misuse of ChatGPT for generating false information or spreading misinformation. How does OpenAI address this?
Olivia, OpenAI is committed to addressing misuse and misinformation. They are actively improving the default behavior of ChatGPT to reduce both obvious and subtle harmful outputs. They also plan to allow users to customize its behavior within broad societal bounds.
Randall, can ChatGPT be fine-tuned to avoid biased or controversial responses in specific contexts?
Oliver, OpenAI is developing an upgrade to ChatGPT to allow users to easily customize its behavior to align with individual values. This will enable avoiding biased or controversial responses in line with specific contexts.
Randall, in scenarios where ChatGPT generates incorrect responses, how can we ensure the accuracy and reliability of the information?
Sophia, while ChatGPT tries to provide reliable responses, it's always good practice to verify the information from multiple sources, especially in critical or sensitive situations. OpenAI is actively working on providing clearer indications of confidence in responses to help users better evaluate reliability.
Randall, how does ChatGPT handle voice input? Is it limited to text-based interactions only?
David, currently ChatGPT is designed for text-based interactions. Although OpenAI has seen some exciting research on multimodal models that can understand both text and image inputs, it's not available in ChatGPT yet. It's an area they're actively exploring!
Randall, how can ChatGPT be used in educational settings? Can it assist teachers or provide personalized learning experiences?
Jack, ChatGPT can have potential applications in education. It can provide additional information or explanations to students, answer questions, help with research, or assist teachers with lesson planning. However, further research and development are needed to ensure its efficacy and suitability in educational contexts.
Randall, how does ChatGPT handle language biases? Is it capable of understanding and responding equally well to different dialects?
Hannah, ChatGPT is trained on a large corpus of internet text, which can introduce biases. While it strives to be a helpful tool, it might not always recognize or respond to different dialects or languages equally well. OpenAI aims to improve this aspect and reduce biases through ongoing research and user feedback.
Randall, are there any plans to integrate ChatGPT with existing educational platforms or tools to enhance the learning experience?
Robert, integrating ChatGPT with educational platforms could potentially enhance the learning experience and provide better access to information. However, such integrations would require careful consideration to address specific educational needs, maintain privacy, and ensure a positive impact.
Nice article, Randall! I'm curious about the computational resources required to power ChatGPT. Is it accessible to everyone or limited to specific organizations?
Megan, thanks for your question! Initially, OpenAI offered ChatGPT in a research preview to gather user feedback. Access was gradually expanded, and they also introduced a subscription plan, ChatGPT Plus, to provide additional benefits. They aim to refine availability based on user needs and ensure broader accessibility over time.
Randall, how does ChatGPT handle generating human-like responses? Can it pass as a human in a conversation?
Anna, while ChatGPT has shown remarkable progress, it might still produce responses that seem human-like initially but can lack consistency or coherent long-term interaction. It's not designed to intentionally deceive or pass as a human.
Randall, is OpenAI planning to explore voice-based interaction with ChatGPT in the future?
Daniel, while I don't have specific details, OpenAI recognizes the potential of voice-based interaction and wants to make future models that can understand and respond to spoken language. So, it's definitely an area they plan to explore and develop.
Randall, the ability to remember and maintain coherence over multiple interactions would indeed make ChatGPT more powerful as a virtual assistant. I'm excited to see how it evolves!
Sophie, ensuring inclusive and fair AI systems is crucial for building trust and ensuring equal opportunities for all individuals.
Randall, do you think the ability to generate human-like responses might lead to potential misuse or deception by malicious users?
Daniel, OpenAI acknowledges the risks and concerns of AI systems being used maliciously, but they are actively working on making the AI robust against vulnerabilities and deploying safeguards to prevent misuse.
Randall, voice input capability can significantly improve user experience by allowing more convenient and rapid interactions. I look forward to its potential integration!
Andrew, indeed! Voice input can make interaction with AI systems more natural and intuitive. It holds great promise for future enhancements and accessibility.
Randall, being transparent about limitations and actively seeking feedback to improve AI systems like ChatGPT is commendable. It shows a commitment to responsible development.
Randall, the applications you mentioned for ChatGPT's 'heavy lifting' capabilities are diverse! It's exciting to see how it can assist in various domains and tasks.
Rachel, indeed! ChatGPT's versatility makes it a powerful tool across multiple tasks, and it's fascinating to witness its potential in different use cases.
Randall, ensuring privacy and ethical usage of AI systems is crucial. OpenAI's approach to addressing biases and allowing user customization is commendable.
Randall, integrating voice-based interaction can make AI systems like ChatGPT more accessible and useful in scenarios where text input might not be ideal.
Randall, voice-based interaction would be a game-changer. It could make AI more accessible and intuitive, benefiting a wide range of users.
Randall, it's great to see that OpenAI is gradually expanding access to ChatGPT and introducing subscription plans to accommodate different user needs. This ensures broader availability and usability.
Randall, gradual expansion and subscription plans help strike a balance between accessibility and resource requirements. This allows more people to benefit from and contribute to ChatGPT's development.
Megan, Nathan, and Sophia, thank you for the positive feedback! OpenAI values user input and aims to make AI accessible and useful while addressing concerns and improving reliability.
Randall, OpenAI's user-focused approach to refining availability and considering user feedback ensures that ChatGPT continues to meet evolving user demands. It also fosters greater trust and satisfaction among users.
Randall, integrating ChatGPT with educational tools could greatly enhance the learning experience, especially by providing personalized assistance to students.
Robert, personalized assistance and additional resources provided by ChatGPT could indeed make education more accessible and tailored to individual needs.
Randall, OpenAI's dedication to reducing biases and improving the understanding of different dialects is reassuring. Language inclusivity is crucial for AI systems to be truly effective and equitable.
Randall, it's encouraging to know that OpenAI acknowledges the need to improve ChatGPT's understanding and response capabilities across different languages and dialects. That will help make AI more inclusive and accessible on a global scale.
Randall, ChatGPT's potential in education is fascinating. Leveraging AI systems can help personalize learning experiences and provide additional support to students.
Randall, integrating ChatGPT with existing educational platforms can help enrich the learning experience and provide students with access to additional resources.
Randall, understanding the current text-based limitations of ChatGPT and OpenAI's exploration of multimodal models gives insight into its future potential for voice-based interactions. Exciting times ahead!
David, I agree! Voice-based interactions can make AI systems like ChatGPT more intuitive and seamlessly integrated into our daily lives.
David, integrating voice-based interaction would democratize access to AI systems, making them more inclusive and usable for all individuals.
David, voice-based interactions could greatly enhance ChatGPT's accessibility, especially for people with visual impairments or those who prefer spoken communication.
David, voice-based interaction could also benefit individuals with motor disabilities, offering them a more accessible way to interact with AI systems like ChatGPT.
Emma, you're right. Voice-based interactions provide an additional dimension of accessibility and inclusion, benefiting a wider range of users.
Randall, clear indications of response confidence would indeed help users better evaluate the reliability and accuracy of ChatGPT's outputs. It's great to see efforts in that direction.
Sophia, you're right! ChatGPT's ability to complement human expertise can be incredibly valuable in education and various technical domains.
Thank you, Randall, for addressing my question. It's reassuring to know that efforts are being made to allow customization and align AI systems with individual values.
Randall, it's great to know that future upgrades will allow users to customize ChatGPT's behavior, effectively addressing biased or controversial responses.
Oliver, it's essential to fine-tune AI models like ChatGPT to ensure fairness and inclusivity. Biased responses can perpetuate stereotypes or discriminate against certain groups.
Sophie, you're absolutely right. It's crucial to address biases in AI systems to promote fairness and equality.
Randall, it's reassuring to know that OpenAI is committed to reducing harmful outputs and addressing the potential for misinformation. Transparency is key in building trust!
Randall, OpenAI's commitment to addressing privacy concerns and preventing malicious use of ChatGPT instills confidence in its responsible deployment.
Randall, I appreciate your response! It's fascinating to see the potential future developments of ChatGPT in the virtual assistant domain.
Randall, it's interesting to know that ChatGPT lacks the ability to remember prior information. It would indeed be an exciting improvement if it develops that capability in the future.
Randall, it's exciting to hear about potential future developments in ChatGPT. AI-powered virtual assistants have come a long way, and it will be fascinating to see what lies ahead!
Emily, personalized learning experiences empowered by AI systems like ChatGPT can have a significant positive impact on education, adapting to individual needs and enhancing educational outcomes.
Paul, ChatGPT's integration into existing applications has shown promise. It has been used to generate content, provide writing assistance, and even deliver programming tips within tools that people already use.
Ethan, that's impressive! The ability to seamlessly integrate ChatGPT into existing tools expands its potential applications significantly.
Ethan, the integration of ChatGPT into existing tools opens up possibilities for enhanced productivity and creativity. It's exciting to witness the real-world impact of AI technologies like ChatGPT!
Paul, absolutely! Real-world applications that leverage AI technologies like ChatGPT can transform workflows and inspire new possibilities.
Paul, AI technologies like ChatGPT are transforming various industries, and their integration into existing tools creates a synergistic effect that enhances productivity and unlocks new potentials!
The potential of ChatGPT is impressive, but we need to be cautious and address ethical concerns surrounding AI. It should be used responsibly and transparently.
Julia, I absolutely agree. AI technologies should be developed and used ethically, with a focus on minimizing biases and ensuring accountability.
Adam, I completely agree. Responsible development and usage of AI technologies will shape their impact and ensure a positive outcome for society as a whole.
Adam, ethics should be at the forefront of any AI development. It's encouraging to see responsible approaches being taken to address biases and ensure accountability.
Julia, absolutely! Being mindful of ethical considerations not only minimizes potential harm but also builds trust and fosters wider adoption of AI technologies.
Adam, I couldn't agree more. Responsible development and usage of AI technologies will shape their impact and ensure a positive outcome for society as a whole.
Julia, being mindful of ethics and promoting responsible AI development is essential for harnessing the potential benefits of AI while minimizing potential harms.
Julia, absolutely! Being mindful of ethical considerations not only minimizes potential harm but also builds trust and fosters wider adoption of AI technologies.
Voice-based interactions can also make ChatGPT more user-friendly in scenarios where typing might not be convenient, like when multitasking or using smaller devices.
Transparency and user feedback are vital in refining AI systems like ChatGPT. OpenAI's approach demonstrates a commitment to responsible AI development and improvement.
Great article, Randall! ChatGPT's capability to handle heavy lifting tasks is truly impressive. It's undoubtedly revolutionizing technology.
I agree, Sarah. ChatGPT's advancements are game-changing. The potential it holds for various industries is immense.
I'm curious about the scalability of ChatGPT. Randall, could you provide more insights into how it can handle large-scale tasks?
Linda, from my understanding, ChatGPT has been trained on massive amounts of data, allowing it to handle diverse tasks and adapt to different contexts effectively.
The model's architecture incorporates self-attention mechanisms, which helps it understand the dependencies among words and provide meaningful responses.
Thank you for the explanation, Michael. It sounds really impressive!
I wonder how ChatGPT ensures the accuracy of its responses. Can it reliably provide correct information and avoid misinformation?
James, ChatGPT's responses are generated based on patterns it learned from data. While it can be accurate most of the time, it's always important to verify information from reliable sources, especially when it comes to critical matters.
However, OpenAI has made efforts to build safety measures to minimize harmful or misleading outputs from the model.
Thank you for the clarification, Sarah. So, it's crucial to use ChatGPT as a tool but not solely rely on it for accuracy. Makes sense!
Randall, could you explain how ChatGPT handles sensitive or inappropriate content? Is there a risk of it generating harmful responses?
Maria, OpenAI has implemented safety mitigations to address inappropriate content. They use a two-step process involving pre-training and fine-tuning, as well as extensive human review, to reduce harmful outputs. It helps in curbing risks.
The potential applications of ChatGPT seem endless. I can imagine it being incredibly useful in customer support and enhancing user experiences.
Absolutely, Ryan. ChatGPT's ability to engage in natural language conversation makes it an ideal tool for improving customer interactions.
It can provide prompt and useful responses, reducing the need for human intervention in certain scenarios.
Indeed, Maria! By delegating routine or common inquiries to ChatGPT, companies can focus their human resources on more complex and specialized tasks.
Randall, what steps are being taken to improve ChatGPT's limitations? Are there ongoing efforts to make it even more efficient and accurate?
Emily, OpenAI is actively working to refine and expand ChatGPT. Feedback from users is collected to identify its limitations and make necessary improvements. It's an ongoing process to enhance its capabilities continuously.
That's great to hear, Michael. Continuous improvement is crucial to ensure ChatGPT's reliability and address any potential shortcomings effectively.
Indeed, Emily. By incorporating user feedback and iteratively refining the model, OpenAI can ensure that ChatGPT becomes more powerful and dependable over time.
I wonder if ChatGPT has any limitations when it comes to understanding specific domain knowledge or technical jargon.
David, ChatGPT may sometimes struggle with nuanced or highly technical queries. Its responses are based on general knowledge from extensive training, so domain-specific understanding can be limited.
Thank you, Linda. It's good to be aware of those limitations and not rely on ChatGPT for highly specialized topics.
Randall, what measures are in place to handle potential biases in ChatGPT's responses?
Rachel, OpenAI is committed to addressing biases in AI systems. They make efforts to reduce both glaring and subtle biases through a combination of human reviewers and ongoing research. Transparency and fairness are crucial aspects they strive for.
That's reassuring, Sarah. It's important to ensure AI systems like ChatGPT are inclusive and unbiased in their responses.
I'm curious about the computational resources required to run ChatGPT. Does it demand significant processing power?
Patrick, running ChatGPT efficiently does require substantial computational resources, especially for training and fine-tuning the model. However, OpenAI is actively working on optimizing its performance to reduce such requirements.
This article is fascinating! Randall, do you think there will be potential ethical concerns arising from the use of ChatGPT?
Karen, the use of AI systems like ChatGPT indeed raises ethical concerns. It's crucial to employ responsible practices when using the technology, ensuring transparency, accountability, and respect for users' privacy. OpenAI acknowledges these concerns and is actively addressing them.
Thank you for your thoughtful response, Sarah. Responsible and ethical deployment of AI is indeed paramount to avoid any unintended consequences.
Randall, what are some other potential areas where ChatGPT can have a significant impact?
Aaron, aside from customer support, ChatGPT can be utilized in virtual assistants, content generation, language translation, and even assisting researchers and educators. The possibilities are vast!
Thank you, Michael. It's incredible to see how ChatGPT can be beneficial in numerous domains and revolutionize various industries.
I'm amazed by the potential of ChatGPT. Randall, are there any plans to make it more accessible to a wider range of users or developers?
Laura, OpenAI is actively exploring options to provide more accessibility to ChatGPT. They are working on developer-friendly tools and exploring partnerships to expand its reach. Ensuring a broader user base is definitely on their radar.
Randall, what does the future hold for ChatGPT? Can we expect even more advanced versions in the coming years?
Donald, OpenAI is committed to advancing AI technologies, including ChatGPT. They plan to refine and expand it based on user feedback and identified limitations. So, yes, we can expect more advanced versions in the future.
The potential of AI systems like ChatGPT is truly exciting. However, we must ensure that human control and human values remain at the forefront.
Absolutely, Alexandra. Striking a balance between the power of AI systems and maintaining human oversight and ethical considerations is vital for responsible and beneficial use.