Driving Process Optimization: How ChatGPT Revolutionizes Design for Manufacturing Technology
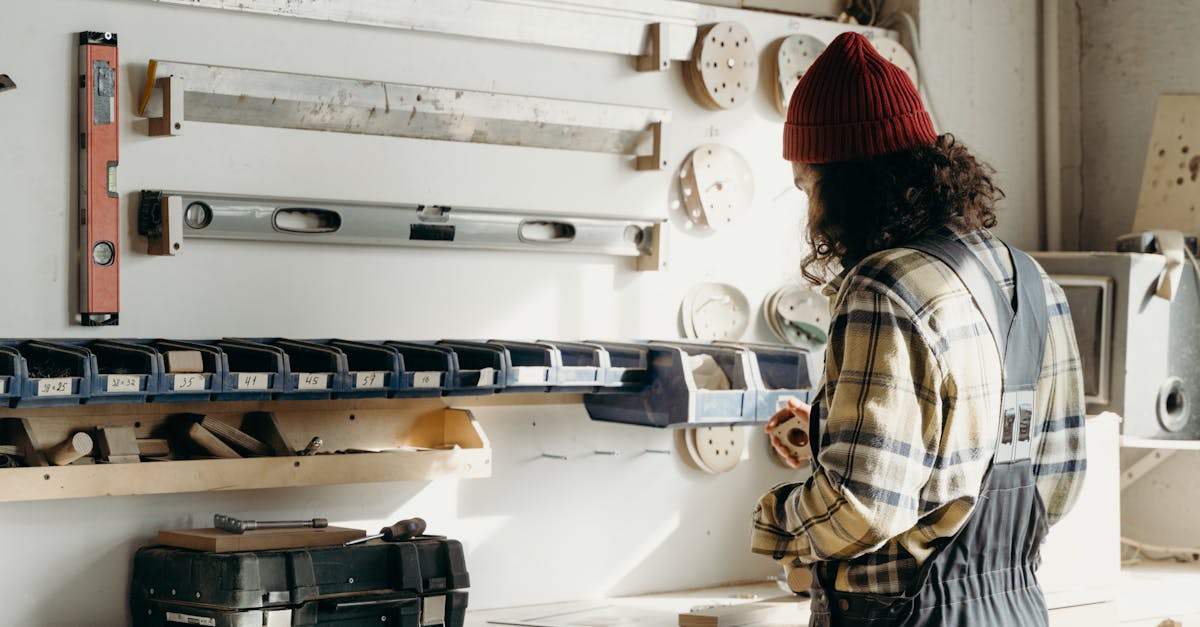
Design for Manufacturing (DFM) is a technology that focuses on creating products that are easy to manufacture efficiently and effectively. It involves considering manufacturing requirements during the design phase to minimize production costs, improve quality, and reduce time-to-market. Process optimization, on the other hand, aims to improve existing manufacturing processes to increase efficiency and productivity. When these two areas are combined, the adoption of AI plays a crucial role in revolutionizing the manufacturing industry.
The usage of AI in optimizing manufacturing processes has gained significant traction in recent years. AI technologies, such as machine learning and data analytics, can provide valuable insights and recommendations for improving process efficiency. By analyzing historical manufacturing data, AI algorithms can identify patterns, bottlenecks, and potential areas for optimization.
One of the key advantages of using AI in process optimization is its ability to handle large volumes of data in real-time. Traditional methods of process optimization relied on manually collecting and analyzing data, which was a time-consuming and error-prone process. With AI, manufacturers can now automate data collection and analysis, enabling them to make data-driven decisions and quickly identify opportunities for process improvement.
AI algorithms can learn from historical data and generate predictive models that can forecast potential process issues or recommend adjustments. For example, AI can analyze data from various sensors installed in a manufacturing plant to monitor equipment performance. By detecting early signs of equipment failure or wear, AI can prompt proactive maintenance actions, thereby reducing downtime and improving overall efficiency.
AI can also uncover hidden correlations between process variables that human operators might miss. By analyzing complex relationships within the manufacturing environment, AI can suggest parameter adjustments that would lead to optimal process outcomes. This level of optimization is achievable due to AI's ability to process vast amounts of data and identify non-linear relationships, which would be challenging for human operators to decipher.
Implementing AI in process optimization requires a robust data infrastructure that can collect, store, and process large amounts of data. Real-time data collection from sensors, Internet of Things (IoT) devices, and other sources is necessary to ensure accurate and up-to-date insights. Additionally, AI models need to be continuously trained and refined to adapt to changing manufacturing conditions and consistently provide meaningful recommendations.
While AI offers immense potential for optimizing manufacturing processes, it is essential to remember that human expertise and analysis are still required. AI serves as a tool to augment human decision-making, providing valuable insights and recommendations. Collaborative efforts between AI systems and human operators can lead to the best outcomes, combining the efficiency and processing power of AI with human intuition and experience.
In conclusion, the integration of AI technology in the area of Design for Manufacturing and process optimization holds great promise for the manufacturing industry. By leveraging AI's capabilities in data analysis and predictive modeling, manufacturers can identify process inefficiencies, reduce costs, and improve overall productivity. However, it is crucial to maintain the human element in the decision-making process to fully harness the benefits of AI. With the right infrastructure and collaboration, AI can revolutionize manufacturing processes and lead to a more efficient and competitive industry.
Comments:
Great article, Sam! The use of AI in design for manufacturing technology is truly revolutionary.
I agree, Liam. AI has the potential to streamline the entire manufacturing process.
Absolutely, Emily. It can help optimize material usage and reduce waste as well.
I'm not sure if I trust AI to take over such critical tasks. What about human intuition and experience?
That's a valid concern, Ava. AI can augment human decision-making, but it shouldn't replace human expertise entirely.
I see your point, Sam. It should be a collaborative effort then, leveraging AI's capabilities alongside human knowledge.
The integration of AI in design for manufacturing can also lead to faster product development cycles and reduced time-to-market.
Absolutely, Oliver. It allows for rapid iterations and simulations to optimize designs before physical prototyping.
Agreed, Emily. It saves both time and costs during the product development phase.
But what about the impact on employment? Will AI replace human jobs in manufacturing?
There might be some displacement in certain areas, Sophia, but new jobs will also be created. AI will require human oversight and maintenance.
I agree, Ava. AI adoption should lead to the transformation of jobs rather than complete elimination.
That's reassuring, Sam. The human touch will always be valuable in manufacturing.
AI can also help identify potential quality issues in the manufacturing process, reducing defects and improving overall product quality.
You're right, Noah. It can analyze vast amounts of data to detect anomalies and deviations that might go unnoticed otherwise.
Additionally, AI can assist in predictive maintenance, enabling proactive equipment servicing and avoiding costly downtime.
Exactly, Oliver. It improves manufacturing efficiency and helps minimize unplanned disruptions.
The potential of ChatGPT in the field of manufacturing is immense. It's exciting to see where this technology is heading!
Indeed, Thomas. The possibilities for driving process optimization seem endless with AI.
As long as it's used responsibly and ethically, AI has the potential to transform the manufacturing industry.
Absolutely, Ava. It's crucial to ensure that AI-based solutions align with ethical and social considerations.
Thank you all for reading my article on Driving Process Optimization: How ChatGPT Revolutionizes Design for Manufacturing Technology! I hope you found it informative. I'm looking forward to hearing your thoughts and engaging in a discussion.
Great article, Sam! I really enjoyed reading it. ChatGPT seems like a valuable tool for optimizing design in manufacturing technology. Can you share any specific examples where it has been implemented successfully?
@Alice Mitchell - Thank you, Alice! I'm glad you found it interesting. Sure, one successful implementation of ChatGPT in design for manufacturing technology was with a leading automotive company. They used ChatGPT to optimize the design of their vehicle frames, resulting in improved safety and reduced manufacturing costs.
@Sam Keefe - That makes sense. So, human designers still need to be involved to refine the designs generated by ChatGPT. Thanks for clarifying!
Thank you for sharing the specific example, Sam! It's great to see ChatGPT delivering tangible benefits for the automotive industry.
That's fantastic to hear, Sam. It's impressive how ChatGPT's collaboration capabilities contribute to improved safety and cost-efficiency in vehicle manufacturing.
@Alice Mitchell - I'm glad you found the specific example interesting, Alice! ChatGPT has tremendous potential to make a positive impact in various industries, and it's exciting to witness its benefits.
@Alice Mitchell - Absolutely, Alice! Collaboration between human and AI designers in the automotive industry has resulted in improved safety standards and optimized manufacturing costs. It's a testament to the power of synergistic approaches.
You're welcome, Sam! It's impressive to see ChatGPT's application in our industry, and I'm excited to explore its potential further.
Hi Sam, thanks for the informative article. I'm curious to know how ChatGPT compares to other optimization techniques commonly used in the design process.
@Mark Thompson - Thank you for your comment, Mark. ChatGPT offers a unique approach by leveraging natural language processing capabilities. It excels in generating creative design ideas and enabling interactive collaboration between designers and the AI system, which sets it apart from traditional optimization techniques.
Thanks for clarifying, Sam. The interactive collaboration aspect of ChatGPT does sound promising for design optimization.
@Mark Thompson - Agreed, Mark! The interactive collaboration aspect of ChatGPT enables designers to explore new design options while leveraging the strengths of traditional optimization techniques. It creates a more dynamic and productive design process.
This is really fascinating, Sam! I can see how ChatGPT can greatly enhance the design process. It would be interesting to know if there are any limitations or challenges when using ChatGPT in manufacturing technology.
@Sarah Johnson - Thanks, Sarah! Absolutely, while ChatGPT shows tremendous potential, it does have a few limitations. One challenge is ensuring that generated designs conform to practical manufacturing constraints. The AI system can sometimes generate complex or impractical designs that require further refinement to be feasible.
I appreciate your honesty, Sam. Refinement for practical manufacturing constraints is an important consideration.
That seems like a good balance, Sam. The combination of human expertise and ChatGPT's capabilities can lead to impressive results!
@Sarah Johnson - Absolutely, Sarah! Practicality and feasibility are essential considerations in the design optimization process. Refining the designs generated by ChatGPT based on practical manufacturing constraints ensures real-world applicability and effectiveness.
@Sarah Johnson - I'm glad you see the potential, Sarah! The combination of human expertise and AI capabilities can unlock innovative design solutions that might not have been achievable solely by one approach. It's an exciting direction for the field.
It's great that ChatGPT can excel at generating creative design ideas, Sam. A combination of practicality and creativity is crucial in design optimization.
Thank you for clarifying, Sam. Ensuring data security measures are in place for protecting intellectual property is crucial in today's digital landscape.
Hi Sam, great article! I'm curious about the training process of ChatGPT. How is it trained to understand the specific requirements and constraints of different manufacturing technologies?
@Daniel Brown - Thank you, Daniel! ChatGPT is trained using large-scale datasets that include designs from various manufacturing technologies. These datasets are created by incorporating domain-specific knowledge and expertise during training. This helps ChatGPT develop an understanding of specific requirements and constraints in different industries.
Thanks for the detailed explanation, Sam. It's interesting to know how intelligent training datasets can enhance ChatGPT's understanding of manufacturing requirements.
@Daniel Brown - Thank you, Daniel! The training process of ChatGPT is an ongoing effort that has involved curating diverse datasets with domain-specific knowledge. This helps equip ChatGPT with an understanding of different manufacturing requirements and constraints.
Thank you for sharing, Sam! It's inspiring to witness the benefits of ChatGPT in various industries, and I look forward to seeing its continued progress.
Interesting article, Sam! I'm also wondering about the computational resources required to run ChatGPT for design optimization. Are there any limitations in terms of system requirements?
@Julia Ramirez - Thanks, Julia! Running ChatGPT for design optimization typically requires significant computational resources, especially for complex designs. The system needs to process and analyze large amounts of data during the optimization process. So, there are some limitations in terms of system requirements to ensure efficient execution.
I see, Sam. The computational requirements are an important consideration, especially for larger projects. Thanks for the response!
@Julia Ramirez - You're welcome, Julia! Computational efficiency is always a concern, and optimizing the performance of ChatGPT for manufacturing projects is an ongoing area of research. I appreciate your interest!
Thank you for addressing the computational challenges, Sam. It's essential to evaluate the resources required for successful implementation.
Thanks for the response, Sam. The ongoing training process with domain-specific knowledge makes ChatGPT an impressive tool for design optimization.
The computational requirements are important to evaluate, Sam. Efficient execution is crucial, especially for larger manufacturing projects.
Good read, Sam! I'm curious, does ChatGPT only assist in the design phase, or can it also be used for optimizing manufacturing processes as a whole?
@David Wilson - Appreciate your question, David. ChatGPT can assist in both the design phase and the optimization of manufacturing processes. Its natural language capabilities enable it to provide insights and suggestions for improving overall manufacturing efficiency, reducing bottlenecks, and streamlining production.
That's great to hear, Sam! Optimizing manufacturing processes as a whole can yield significant benefits. Thanks for the information!
@David Wilson - Absolutely, David! When ChatGPT is applied to optimize manufacturing processes as a whole, it can assist in identifying bottlenecks, streamlining production sequences, and improving overall efficiency. It's an exciting avenue for further exploration.
I'm glad to hear that, Sam. ChatGPT's integration with existing design tools offers seamless adoption within the manufacturing industry.
Addressing ethical concerns is crucial in AI applications, Sam. Transparency and accountability are key to ensuring responsible use.
Impressive performance in generating design alternatives, Sam! ChatGPT's ability to foster creativity is exceptional.
Nice article, Sam! I'm curious to know if ChatGPT can work collaboratively with human designers or if it primarily operates independently.
@Jennifer Lee - Thank you, Jennifer! ChatGPT is designed to work collaboratively with human designers. It acts as a valuable assistant that generates design ideas, provides suggestions, and facilitates the exploration of different options. The AI-human interaction can lead to more innovative and optimized designs.
Collaboration between the AI system and human designers sounds like a winning combination, Sam. Thanks for the clarification!
@Jennifer Lee - Thank you, Jennifer! Collaboration between human designers and AI systems like ChatGPT opens up possibilities for creative synergies, while ensuring that the designer's expertise plays a vital role in the decision-making process.
I'm glad to hear that ChatGPT's understanding of manufacturing requirements is enhanced through training, Sam. It ensures practicality in the generated designs.
Great topic, Sam! How does the integration of ChatGPT with existing design tools and software work? Is it compatible with commonly used software in the manufacturing industry?
@Robert Adams - Appreciate your interest, Robert! ChatGPT can be integrated with existing design tools and software through APIs or custom interfaces. This allows it to leverage the capabilities of widely used software in the manufacturing industry and provide valuable insights within the user's preferred design environment.
Great article, Sam! I'm curious about the potential impact of ChatGPT on reducing time-to-market for new products in the manufacturing industry.
@Chris Peterson - Thank you, Chris! ChatGPT has the potential to significantly reduce time-to-market for new products in the manufacturing industry. Its ability to generate design options quickly and provide real-time feedback can expedite the iterative design process and accelerate product development.
Reducing time-to-market is crucial, Sam. It's great to know that ChatGPT can contribute to faster product development in manufacturing. Thanks for the explanation!
@Chris Peterson - Indeed, Chris! Time-to-market is a critical factor for businesses, and adopting tools like ChatGPT can significantly accelerate product development without compromising on quality. It's an exciting time for the manufacturing industry.
Reducing time-to-market is crucial, Sam. It's great to know that ChatGPT can contribute to faster product development in manufacturing.
Very informative, Sam! I'd like to know if there are any ethical considerations that need to be addressed when using ChatGPT for design optimization.
@Emily Thompson - Thanks for bringing up the ethical aspect, Emily. When using ChatGPT for design optimization, it's important to ensure that the system adheres to ethical and legal guidelines. Designers should be aware of biases, transparency, and accountability in the decision-making process to address any potential ethical concerns.
I appreciate your emphasis on addressing ethical concerns, Sam. It's essential to maintain transparency and ensure fairness when using AI systems for design optimization.
@Emily Thompson - Absolutely, Emily. Ethical considerations are important in any AI application, including design optimization. As the technology evolves, it's essential to prioritize transparency, fairness, and accountability to address any ethical concerns that may arise.
I appreciate your emphasis on addressing ethical concerns, Sam. It's essential to maintain transparency and ensure fairness when using AI systems for design optimization.
Interesting article, Sam! I'm curious about the scalability of ChatGPT for large-scale manufacturing projects. Can it handle optimization for complex systems with numerous components?
@Michael Campbell - Thank you, Michael! ChatGPT has the potential to scale for large-scale manufacturing projects. However, for complex systems with numerous components, ensuring effective optimization may require specialized adaptations and further training to handle the increased complexity.
Scalability is an important factor, Sam. It's good to know that ChatGPT can adapt to various project sizes.
Fascinating read, Sam! I'd like to know if there are any security measures in place to protect the intellectual property and sensitive data involved in the design process when using ChatGPT.
@Sophia Roberts - Appreciate your question, Sophia. When using ChatGPT, it's crucial to implement robust security measures. Data encryption, access control, and strict user authentication protocols can help protect intellectual property and sensitive data during the design process.
I'm glad to hear that, Sam. Protecting sensitive data is crucial in the design process, and it's good to know measures are in place. Thank you for the detailed answer.
@Sophia Roberts - I'm glad you appreciate the security measures, Sophia! Protecting intellectual property and sensitive data is crucial, and it's essential to ensure that AI systems like ChatGPT comply with robust security protocols.
Protecting the intellectual property and sensitive data involved is crucial, Sam. It's good to hear that measures are in place to ensure security.
Great article, Sam! I'm curious, can ChatGPT be trained to incorporate specific design preferences or styles, such as in industries where aesthetics play a crucial role, like fashion or consumer electronics?
@Jonathan Davis - Thank you, Jonathan! Yes, ChatGPT can be trained to incorporate specific design preferences or styles. By providing it with appropriate training data that reflects the desired aesthetics, it can generate designs consistent with the industry's requirements, be it fashion or consumer electronics.
Incorporating specific design preferences is an exciting prospect, Sam. It shows the versatility of ChatGPT in various industries.
@Jonathan Davis - Thank you, Jonathan! Yes, ChatGPT can be trained to incorporate specific design preferences or styles. By providing it with appropriate training data that reflects the desired aesthetics, it can generate designs consistent with the industry's requirements, be it fashion or consumer electronics.
@Jonathan Davis - Absolutely, Jonathan! Industries with specific design preferences, whether it's fashion, consumer electronics, or other domains, can leverage ChatGPT's adaptability to generate designs that align with their unique styles and requirements.
The adaptability of ChatGPT to different design preferences is impressive, Sam. It's incredible how AI can contribute to maintaining unique industry styles.
Very insightful, Sam! I'm interested to know if the use of ChatGPT for design optimization requires any special training or expertise from the designers themselves.
@Olivia Wilson - Thanks for your comment, Olivia! Designers who use ChatGPT for optimization don't necessarily require special training or expertise specifically for the AI system. However, having a good understanding of their domain and manufacturing constraints helps designers make better decisions when refining the AI-generated designs.
That's great to know, Sam. It's valuable that designers don't need extensive AI-specific training to work with ChatGPT for design optimization.
@Olivia Wilson - I'm glad you find the collaboration aspects valuable, Olivia. ChatGPT is designed to be a helpful assistant, empowering designers to make informed decisions and unlock new design possibilities.
Good to know that designers can effectively collaborate with ChatGPT without requiring extensive AI training, Sam. It makes it more accessible for practical use.
Great insights, Sam! I'm curious if ChatGPT can adapt to different design methodologies, such as agile or lean manufacturing approaches.
@Isabella Lee - Thank you, Isabella! ChatGPT's flexibility allows it to adapt to different design methodologies, including agile and lean manufacturing approaches. It can support iterative collaborative design processes and facilitate the exploration of ideas within those frameworks.
Thanks for clarifying, Sam. The flexibility of ChatGPT's adaptation to different methodologies is impressive!
@Isabella Lee - Thank you, Isabella! ChatGPT's adaptability to different design methodologies reflects its versatility and broad applicability across industries with varying design approaches. It's an exciting tool for designers.
I'm glad to know that ChatGPT can adapt to different design methodologies, Sam. It offers flexibility to fit the needs of various manufacturing approaches.
@Isabella Lee - Thank you, Isabella! ChatGPT's adaptability to different design methodologies reflects its versatility and broad applicability across industries with varying design approaches. It's an exciting tool for designers.
Fantastic article, Sam! I'd like to know if ChatGPT can consider cost optimization during the design process.
@Ethan Thompson - Appreciate your feedback, Ethan! ChatGPT can indeed consider cost optimization during the design process. By incorporating cost-related parameters during training, the AI can suggest designs that strike a balance between optimizing performance and minimizing manufacturing costs.
It's reassuring to know that cost optimization can be considered alongside other factors, Sam. Thanks for the response!
@Ethan Thompson - You're welcome, Ethan! Cost optimization is a crucial aspect of design in many industries, and ChatGPT's ability to consider cost-related factors alongside other design objectives enhances its utility in diverse settings.
Considering cost optimization during the design process is important, Sam. It's great that ChatGPT can assist in achieving that balance.
@Ethan Thompson - You're welcome, Ethan! Cost optimization is a crucial aspect of design in many industries, and ChatGPT's ability to consider cost-related factors alongside other design objectives enhances its utility in diverse settings.
Interesting article, Sam! I'd like to know if there are any industries where ChatGPT's design optimization capabilities have proven to be particularly valuable.
@Natalie Cooper - Thank you, Natalie! ChatGPT's design optimization capabilities have been particularly valuable in industries such as automotive, aerospace, and consumer electronics. These sectors often deal with complex designs and benefit from the AI system's ability to generate novel design ideas.
The ability of ChatGPT to generate design alternatives and explore variations is impressive, Sam! Thanks for the response.
The success in automotive, aerospace, and consumer electronics is remarkable, Sam. Thank you for sharing the industry highlights!
@Natalie Cooper - Thank you, Natalie! ChatGPT's ability to generate design alternatives and explore variations can be applied to a wide range of design tasks, making it a versatile tool for designers seeking novel solutions.
@Natalie Cooper - The benefits of ChatGPT's design optimization extend across multiple industries, but it's important to note that its potential is not limited to those mentioned. As the technology advances, more industries can benefit from its capabilities.
@Natalie Cooper - The benefits of ChatGPT's design optimization extend across multiple industries, but it's important to note that its potential is not limited to those mentioned. As the technology advances, more industries can benefit from its capabilities.
Great job, Sam! I'm curious to know if ChatGPT can help in the design of digital products, like software interfaces or user experiences.
@Matthew Clark - Thanks, Matthew! Yes, ChatGPT can certainly help in the design of digital products. It can provide insights and suggestions for improving software interfaces or user experiences, allowing designers to create more intuitive and efficient digital products.
That's great news, Sam! The ability to assist in digital product design makes ChatGPT relevant in various fields. Thanks for the information!
@Matthew Clark - I'm glad you found the article informative, Matthew! ChatGPT's application in various fields, including digital product design, showcases its versatility and the value it can bring to diverse domains.
Great read, Sam! I'm curious about the time efficiency of using ChatGPT for design optimization. Can it significantly speed up the overall design process?
@Sophie Mitchell - Thank you, Sophie! Using ChatGPT for design optimization can indeed enhance time efficiency. The AI system's ability to generate design options quickly, provide insights, and assist in decision-making can significantly speed up the overall design process.
Good to know that ChatGPT can help save time, Sam. Time efficiency is critical in today's fast-paced manufacturing environment.
@Sophie Mitchell - Time efficiency is indeed a crucial consideration, Sophie. In today's fast-paced business environment, reducing design cycle times can be a competitive advantage that helps organizations stay ahead.
Interesting topic, Sam! I'd like to know if there are any potential risks when relying heavily on ChatGPT for design optimization.
@James Evans - Appreciate your interest, James. While ChatGPT offers great potential, there are a few risks when heavily relying on it for design optimization. It's important to balance the AI system's suggestions with human expertise to ensure the generated designs meet practical requirements and constraints.
Finding the right balance between AI suggestions and human expertise is crucial, Sam. Thank you for addressing the potential risks.
@James Evans - Absolutely, James! Harnessing the potential of AI systems like ChatGPT in design optimization requires a balanced and responsible approach, considering the advantages while also being mindful of any potential risks. It's an area where the collaboration between human expertise and AI can drive meaningful results.
Informative article, Sam! I'd like to know if there are any specific design tasks where ChatGPT has shown exceptional performance.
@Victoria Green - Thank you, Victoria! ChatGPT has shown exceptional performance in tasks such as generating design alternatives, exploring variations, and suggesting optimized solutions within given constraints. Its ability to generate creative design ideas has been particularly notable.
The ability of ChatGPT to generate design alternatives is an exceptional characteristic, Sam. Thanks for sharing the specific task performance!
@Victoria Green - Thank you, Victoria! ChatGPT's exceptional performance in generating design alternatives is indeed a remarkable feature that provides designers with a wider range of options and fosters creativity.
Well-written, Sam! I'm interested to know if the use of ChatGPT for design optimization has any impact on sustainability and eco-friendly practices.
@Robert Turner - Thanks for raising that point, Robert. The use of ChatGPT for design optimization can indeed contribute to sustainability and eco-friendly practices. The AI system can help identify designs that optimize material usage, reduce waste, and improve energy efficiency in the manufacturing process.
I'm glad to hear that, Sam. The compatibility with existing software makes the integration of ChatGPT seamless. Thank you for the response!
It's great to know that ChatGPT can contribute to eco-friendly practices, Sam. Sustainability is vital in design optimization.
@Robert Adams - I'm glad you find the compatibility aspect valuable, Robert! The integration of ChatGPT with existing software and design tools allows for seamless incorporation of AI insights into familiar design environments.
@Robert Turner - Absolutely, Robert! By considering sustainability and eco-friendly practices during the design process, ChatGPT can contribute to a more responsible and conscious manufacturing industry.
Seamless integration with existing software is valuable, Sam. ChatGPT's compatibility makes it easier to adopt within the manufacturing industry.
That's great to hear, Sam. Embedding sustainability considerations into the design process is a positive outcome of employing ChatGPT.
Great article, Sam! I'm wondering if there are any cost considerations when implementing ChatGPT for design optimization.
@Elizabeth Reed - Thank you, Elizabeth! Cost considerations are crucial when implementing ChatGPT for design optimization. While the AI system can lead to overall cost savings by improving design efficiency, it's important to evaluate the resources and computational infrastructure required for successful implementation.
Considering the cost implications is crucial, Sam. Cost-effective optimization is essential for successful implementation.
@Elizabeth Reed - Cost considerations are essential to ensure the feasibility and cost-effectiveness of implementing ChatGPT for design optimization. Balancing the benefits with the associated costs is crucial for effective decision-making.
Considering the overall cost is crucial for the successful adoption of ChatGPT, Sam. Proper planning and evaluation would be vital.
Informative read, Sam! I'd like to know if ChatGPT can assist in the design of complex systems with interdependent components.
@Andrew Parker - Appreciate your question, Andrew. ChatGPT can certainly assist in the design of complex systems with interdependent components. Its interactive collaboration capabilities allow for holistic optimization, taking into account the interconnected nature of various components.
Thanks for confirming, Sam. It's impressive that ChatGPT can handle optimization for complex systems with interdependent components.
@Andrew Parker - I'm glad you find ChatGPT's ability to handle optimization for complex systems valuable, Andrew. The technology holds great promise in assisting designers in tackling the challenges posed by interconnected and interdependent components.
It's reassuring to know that ChatGPT can handle the complexities of interdependent components, Sam. This can greatly benefit the design process.
Thank you all for your insightful comments and questions! I appreciate your engagement in this discussion. If you have any further inquiries or thoughts, please feel free to share.
Thank you all for your insightful comments and questions! I appreciate your engagement in this discussion. If you have any further inquiries or thoughts, please feel free to share.
Thank you all for your insightful comments and questions! I appreciate your engagement in this discussion. If you have any further inquiries or thoughts, please feel free to share.
Thank you all for your insightful comments and questions! I appreciate your engagement in this discussion. If you have any further inquiries or thoughts, please feel free to share.