Elevating Quality Control in Conditioning Technology: Harnessing the Power of ChatGPT
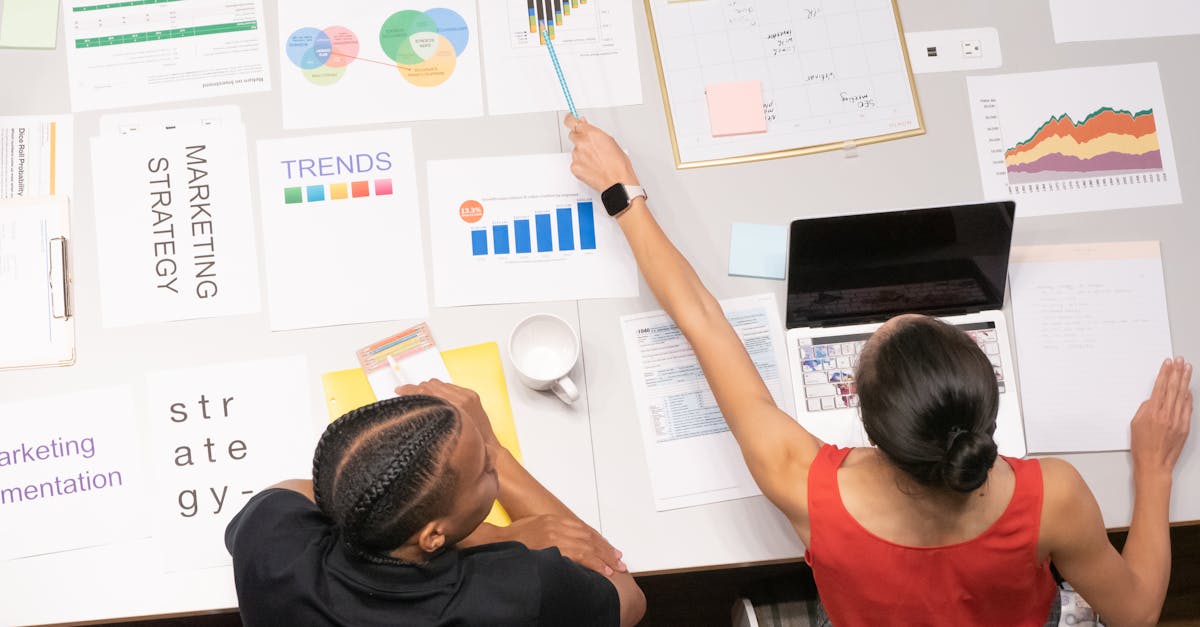
Conditioning is a powerful technology that can significantly enhance quality control processes in various industries. By leveraging the advancements in machine learning, specifically with the use of language models like ChatGPT-4, quality control measures can be efficiently implemented with features such as defect flagging, statistical analysis, and issue prediction.
Defect Flagging
One of the primary applications of ChatGPT-4 in quality control is defect flagging. ChatGPT-4 can be trained on large datasets of quality control logs, past defect reports, and industry-specific knowledge. This training allows the model to learn patterns and characteristics of defects, enabling it to accurately identify and flag potential defects in products or processes.
By integrating ChatGPT-4 into the quality control workflow, manufacturers can ensure that any anomalies or deviations from the desired specifications are promptly detected and brought to the attention of quality control personnel. This early detection helps prevent faulty products from reaching the market, improving overall product quality and customer satisfaction.
Statistical Analysis
Another area where ChatGPT-4 can prove invaluable is in statistical analysis. Quality control often involves analyzing large volumes of data to identify trends, correlations, and potential areas of improvement. ChatGPT-4 can be trained to process and analyze this data, providing meaningful insights and statistical summaries to quality control teams.
With its ability to understand complex instructions, ChatGPT-4 can assist in generating detailed reports on key quality metrics, such as defect rates, compliance percentages, and process performance indicators. By automating this analysis process, manufacturers can save time and resources, enabling quality control teams to focus on addressing critical areas that require attention.
Issue Prediction
Predicting potential issues is another crucial aspect of quality control. By training ChatGPT-4 on historical data, including past defect records and environmental factors, the model can learn to recognize patterns indicative of future issues. This predictive capability allows manufacturers to take proactive measures to prevent defects and maintain quality standards.
With the assistance of ChatGPT-4, quality control teams can receive real-time alerts and warnings about potential issues, enabling them to intervene before any significant problems occur. This proactive approach minimizes the occurrence of defects, reduces downtime, and improves overall operational efficiency.
Conclusion
Incorporating ChatGPT-4 into quality control processes opens up new possibilities for enhancing productivity, ensuring product quality, and meeting customer expectations. By leveraging the technology of conditioning, manufacturers can benefit from defect flagging, statistical analysis, and issue prediction capabilities.
As the field of AI continues to advance, ChatGPT-4 represents a significant step forward in intelligent quality control systems. Its ability to understand and process natural language makes it a powerful tool for improving quality control across various industries.
It is essential to note that while ChatGPT-4 provides valuable insights and support, human expertise and involvement remain crucial in ensuring the effectiveness of quality control measures. Humans play a vital role in interpreting the model's suggestions, making informed decisions, and continuously improving the quality control processes.
Comments:
Thank you all for taking the time to read my article on Elevating Quality Control in Conditioning Technology. I hope you found it informative and thought-provoking. I'm here to discuss any questions or comments you may have!
Great article, Zachary! The potential of using ChatGPT in quality control is fascinating. I imagine it could significantly improve efficiency and accuracy in the field. What are the potential challenges you see in implementing this technology?
Thank you, Alice! You raise a good point. One challenge is ensuring that the ChatGPT model is properly trained on high-quality data in order to provide accurate feedback. We also need to establish safeguards to prevent biases and misinformation. Additionally, integrating the technology into existing systems and processes can pose technical challenges that need to be addressed.
I'm impressed by the potential use of ChatGPT in quality control. It seems like a powerful tool. However, I wonder if it can fully replace human expertise and intuition. What are your thoughts, Zachary?
Hi Bob! That's an important consideration. While ChatGPT can offer valuable insights and assist with decision-making, it is not meant to replace human expertise. The ideal approach is to leverage the strengths of both human experts and AI technology. Human judgment and intuition are essential in complex scenarios where context plays a significant role.
I find the concept intriguing, but I worry about the potential for misuse or manipulation of the system. What measures can be put in place to prevent that?
Carol, that's a valid concern. Transparency and accountability are crucial in preventing misuse. One approach is to make the AI models and their training data publicly available for scrutiny. Developing clear guidelines, adhering to ethical standards, and continuously monitoring and updating the system can help mitigate potential risks.
I wonder if there are any industries or applications that might be more suitable for implementing ChatGPT in quality control? Are there any specific use cases you believe could benefit the most?
Good question, David! While ChatGPT can potentially be used in various industries, some areas that could benefit the most include manufacturing, software testing, customer support, and content moderation. These fields often have high volumes of data and could greatly benefit from an AI-powered system to enhance quality control processes.
I'm curious about potential limitations. Are there any types of quality control tasks that ChatGPT might struggle with, or certain contexts where it is less effective?
Hi Eve! ChatGPT has certain limitations. For instance, it may struggle with highly specialized or domain-specific tasks that require deep expertise. Due to the limitations of the training data, it may also provide inaccurate or unreliable feedback in some instances. Additionally, it's important to consider the potential for biases that may be present in the training data. Contextual understanding can be challenging for AI models, particularly in nuanced situations.
Zachary, what are some key steps organizations can take to harness the power of ChatGPT effectively and optimize their quality control processes?
Frank, great question! To harness the power of ChatGPT effectively, organizations should start by thoroughly understanding their specific quality control needs and challenges. They should invest in high-quality training data and ensure ongoing feedback loops to refine the system. It's essential to continuously test and evaluate the AI model's performance to fine-tune it. Additionally, providing proper training to employees who will utilize the system is critical for successful implementation.
The potential benefits are clear, but what about the costs associated with implementing ChatGPT in quality control? Are there significant financial considerations, and how would organizations justify the investment?
Hi Grace, implementing ChatGPT indeed involves cost considerations. Developing and training the AI model, as well as maintaining and improving it over time, require investment. However, organizations should evaluate the potential long-term benefits, such as improved efficiency, reduced errors, and enhanced customer satisfaction. A cost-benefit analysis can help justify the investment, and organizations can start with smaller-scale pilot projects before scaling up.
I'm excited about the prospects of using ChatGPT in quality control, but I wonder what the future holds. Can you foresee any potential advancements or developments in this field?
Henry, the future of AI in quality control is promising. We can expect advancements in natural language processing, enabling AI models like ChatGPT to better understand context and nuances. The development of more domain-specific models tailored to industry needs is also likely. Moreover, incorporating other AI techniques like computer vision can further expand the potential applications of AI in quality control.
What about privacy concerns? If ChatGPT is analyzing data and providing feedback, how can organizations ensure the confidentiality of sensitive information?
Isabella, privacy is indeed a critical consideration. Organizations must handle data in a secure manner and comply with relevant privacy regulations. Employing techniques such as differential privacy and implementing encrypted communication channels can help protect sensitive information. Establishing data access controls and obtaining explicit consent for data use are also important steps to safeguard privacy.
I'm curious about the potential impact of using ChatGPT on employment in quality control. Could it lead to job losses for human workers in the field?
Jack, while AI technologies like ChatGPT can automate certain tasks in quality control, it is unlikely to completely eliminate human involvement. Instead of job losses, this technology can contribute to job transformation. Human workers can be freed from repetitive or mundane tasks and focus on more complex work, utilizing their expertise alongside the AI system. It's crucial for organizations to ensure proper training and reskilling opportunities for employees in order to adapt to these changes.
How can organizations measure the effectiveness and success of integrating ChatGPT into their quality control processes? Are there specific metrics they should consider?
Karen, measuring the effectiveness of ChatGPT integration is essential. Organizations can track metrics such as error rates, customer satisfaction levels, and processing time to assess the impact. It's important to establish baseline metrics before implementation and regularly compare them to post-implementation metrics. Additionally, gathering feedback from employees who utilize ChatGPT can provide valuable insights into its effectiveness in real-world scenarios.
The use of AI in quality control is intriguing, but what happens when the AI system encounters new or unfamiliar scenarios? How can it handle situations outside its training data?
Liam, handling unfamiliar scenarios is indeed a challenge. While ChatGPT's performance may not be as reliable in such cases, organizations can employ techniques like active learning to continuously gather new data and expand the AI model's knowledge base. Regularly updating and retraining the model can help improve its handling of new situations. Organizations should also ensure there is a feedback mechanism for human experts to intervene and provide guidance when faced with unfamiliar scenarios.
I'm curious about the deployment process. Can you walk us through the steps involved in implementing ChatGPT in an organization's quality control workflow?
Certainly, Melissa! Implementing ChatGPT in a quality control workflow involves several steps. It starts with identifying the specific use case and quality control tasks where ChatGPT can provide value. Next, organizations need to gather and preprocess the relevant training data for the AI model. Then, the model is trained and fine-tuned on this data. After rigorous testing and evaluation, the model is integrated into the existing quality control workflow, and employees receive training to effectively utilize the system.
Zachary, what ethical concerns should organizations be mindful of when implementing AI technology like ChatGPT in quality control?
Nathan, ethical considerations are crucial. Organizations must ensure fairness, transparency, and accountability throughout the implementation of ChatGPT. Addressing biases in the training data and models is important to prevent discriminatory outcomes. Transparently communicating the role of AI to users is necessary, and human oversight should be maintained to prevent the undue influence of the AI system. Regular ethical assessments and continuous monitoring are essential to mitigate and manage ethical risks effectively.
What role do you see ChatGPT playing in helping organizations achieve higher quality standards in their products or services?
Olivia, ChatGPT can play a significant role in improving quality standards. It can help identify potential issues or discrepancies early on, ensuring timely corrective actions. The AI system can provide valuable insights and recommendations to enhance quality control processes. By leveraging the strengths of AI and human expertise, organizations can achieve higher accuracy, reduced errors, and ultimately deliver products or services with improved quality to their customers.
I'm curious about the scalability of ChatGPT for large organizations with complex quality control processes. Can it handle the high volume of data and diverse requirements?
Patrick, scalability is indeed an important consideration. ChatGPT can handle large volumes of data, but organizations need to ensure efficient infrastructure and computing resources to support the system's requirements. It's crucial to develop systems that allow parallel processing and optimize for performance. Adapting the AI model to suit diverse requirements may involve additional fine-tuning and customization. Careful planning and collaboration with experts in deploying AI at scale can help overcome these challenges.
Are there any real-world examples of organizations that have successfully implemented ChatGPT or similar AI models in their quality control processes?
Quinn, indeed! There are several real-world examples. For instance, manufacturing companies have utilized AI systems to detect defects and anomalies in their production lines. Content moderation platforms use AI models to identify and filter out inappropriate content. Customer support services employ AI chatbots to assist users and provide prompt responses. These examples showcase the potential of AI, including ChatGPT, in enhancing quality control across various industries.
What are the key factors an organization should consider before deciding to implement ChatGPT in their quality control processes? Are there any prerequisites?
Rachel, organizations need to consider several factors. Firstly, they should have a clear understanding of their quality control objectives and identify areas where AI can add value. Availability of relevant, high-quality training data is essential for effective implementation. Adequate computing resources and infrastructure to support the AI system are prerequisites. Additionally, it's important to evaluate the feasibility and cost-effectiveness of integrating ChatGPT into existing workflows and ensure the organization is ready to adapt to any changes that come with AI implementation.
Zachary, what are the potential risks associated with relying heavily on AI technology like ChatGPT for quality control? Should organizations have backup plans in case of system failures or errors?
Sarah, relying heavily on AI technology does come with risks. System failures or errors can occur, and organizations should have contingency plans in place to handle such situations. Maintaining human oversight and intervention is important to mitigate risks. Regularly monitoring the AI system's performance and accuracy is crucial. Organizations should also have backup procedures to ensure continuity of quality control processes in case of any unforeseen issues or limitations with the AI system.
Zachary, do you foresee any regulatory challenges when it comes to implementing ChatGPT in quality control processes? How can organizations navigate potential regulatory hurdles?
Thomas, regulatory challenges can indeed arise when implementing AI technology. Organizations should ensure compliance with relevant data protection and privacy regulations. They may need to work closely with legal experts to navigate any potential regulatory hurdles. Transparency and explainability of AI systems can help address concerns related to regulations. Staying informed about evolving regulations in the field of AI and quality control is important to ensure adherence to the required compliance standards.
I'm curious about the computational power required for running ChatGPT. Does it demand significant resources, and how can organizations efficiently handle the computational needs?
Victor, running ChatGPT can require significant computational resources depending on the scale of the implementation. Organizations can explore cloud-based infrastructure options that provide scalable and cost-effective solutions. Leveraging distributed computing techniques and frameworks like GPU acceleration can help optimize the processing power. It's important to evaluate the specific requirements of the AI model and ensure the availability of adequate computing resources to handle the computational needs efficiently.
Zachary, have there been any notable challenges or limitations observed in previous deployments of AI technology in quality control that organizations should be aware of?
Wendy, previous deployments of AI technology in quality control have highlighted some challenges. One notable limitation is the difficulty AI models often face in accurately interpreting or handling nuanced contexts. Biases in training data can also lead to biased outcomes. Implementing AI in real-world scenarios may encounter unforeseen challenges that require adaptation and continuous improvement. Organizations should be prepared to address such limitations and have mechanisms in place for ongoing evaluation and refinement of the AI system.
What potential impact do you envision ChatGPT having on the future of quality control and the wider field of AI applications?
Xavier, ChatGPT and similar AI models have the potential to revolutionize quality control processes. AI can assist in achieving higher accuracy, faster detection of defects, improved decision-making, and overall enhanced quality standards. With continuous advancements, AI models can handle increasingly complex scenarios and become an indispensable tool in quality control across various industries. This progress also contributes to the wider field of AI applications, influencing the development of more intelligent and adaptable systems.
What should organizations keep in mind when it comes to maintaining and updating ChatGPT to ensure its relevance and accuracy over time?
Yara, maintaining and updating ChatGPT is vital to ensure its continued relevance and accuracy. Organizations should establish feedback loops with users and domain experts to gather insights for improvements. Regularly updating the training data and retraining the model can help address any limitations or errors. Monitoring the performance and measuring the impact of ChatGPT on quality control processes enables organizations to identify areas for enhancement and ensure its ongoing effectiveness.
Thank you all for your valuable questions and comments. It has been a pleasure discussing the potential of ChatGPT in quality control with you. If you have any further inquiries, don't hesitate to ask. Let's continue exploring the exciting opportunities AI technology presents!