Empowering Credit Risk Assessment with Gemini: Revolutionizing Technology's Role
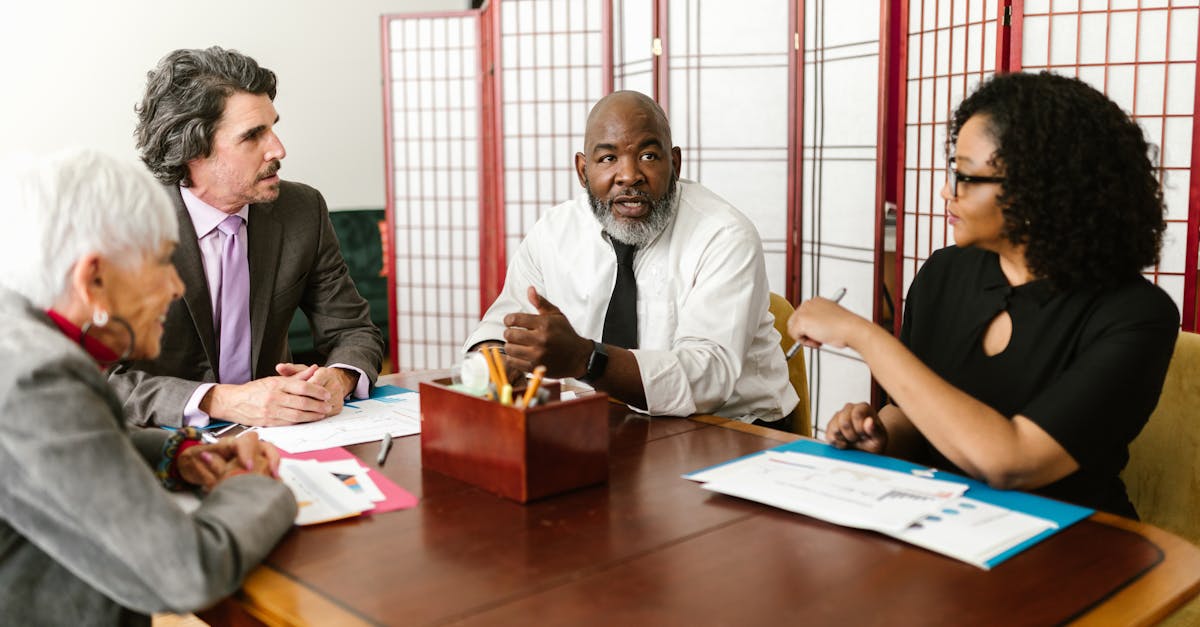
As financial institutions continue to evolve in an increasingly digital landscape, the need for accurate and efficient credit risk assessment remains paramount. Traditionally, credit risk assessment involved manual evaluation and analysis of various factors such as credit history, income levels, and other financial indicators. However, with the advancements in technology and the rise of artificial intelligence, the industry has witnessed a significant transformation in the way credit risk assessment is conducted.
One such groundbreaking technology that has revolutionized the role of technology in credit risk assessment is Gemini. Gemini, developed by Google, is an AI-powered language model that utilizes natural language processing techniques to provide human-like responses to textual queries. By using Gemini in credit risk assessment, financial institutions can leverage its capabilities to enhance the accuracy and efficiency of the assessment process.
Technological Advancements
With its ability to understand and respond to natural language, Gemini simplifies the credit risk assessment process. Instead of relying solely on complex algorithms and predefined criteria, financial institutions can now engage in a conversation with Gemini to discuss specific cases and gain insights into an applicant's creditworthiness. This interaction enables a more comprehensive evaluation of an individual's financial situation, considering factors that might not be explicitly captured by traditional credit scoring models.
In addition to the conversational aspect, Gemini can also analyze large volumes of textual data, including financial statements, loan applications, and other relevant documents. It can quickly extract and interpret key information, enabling financial institutions to make informed decisions based on a comprehensive analysis of the applicant's financial situation.
Enhanced Accuracy and Efficiency
By incorporating Gemini into the credit risk assessment process, financial institutions can achieve enhanced accuracy in their decision-making. Gemini's advanced language understanding capabilities enable it to recognize complex patterns and correlations within textual data, which may not be immediately apparent to human analysts. Moreover, the model can consider and weigh multiple factors simultaneously, leading to more accurate risk assessments.
Additionally, Gemini improves the efficiency of credit risk assessment by automating manual tasks and reducing the overall processing time. The model's ability to comprehend and analyze textual data at a rapid pace allows financial institutions to expedite their decision-making processes, enabling quicker responses to loan applicants.
Future Applications
The application of Gemini in credit risk assessment extends beyond traditional banking institutions. Fintech startups and online lenders can also integrate the technology into their platforms, providing more inclusive and accessible credit opportunities.
Moreover, as Gemini continues to learn from user interactions and receives regular feedback, its performance and accuracy in credit risk assessment will undoubtedly improve. This iterative learning process can contribute to the model's ongoing development and refinement, making it an even more valuable tool for financial institutions in the future.
Conclusion
Gemini represents a significant transformation in credit risk assessment, empowering financial institutions with an AI-powered conversational agent capable of understanding and evaluating complex textual data. With its enhanced accuracy, efficiency, and future potential, Gemini is revolutionizing the role of technology in the assessment process. Financial institutions that embrace this technology are poised to not only streamline their credit risk assessment but also make more informed and inclusive lending decisions.
Comments:
Thank you all for reading my article on Empowering Credit Risk Assessment with Gemini. I'd love to hear your thoughts and opinions on this topic!
Great article, Timothy! Gemini seems like a powerful tool that could revolutionize credit risk assessment. It can potentially save time and improve accuracy. However, I'm curious about its limitations, especially in handling complex financial data. What are your thoughts?
Hi Emma! Thank you for your comment. You make a valid point. While Gemini has proven effective in many areas, including natural language understanding, it may face challenges in complex financial data analysis. It's important to have proper data preprocessing and domain-specific fine-tuning to ensure accurate results. However, it still has the potential to assist credit risk assessment teams and reduce manual effort.
Timothy, I found your article fascinating! The application of natural language processing in credit risk assessment is a game-changer. With Gemini, financial institutions can process vast amounts of textual data more efficiently. I wonder how this technology handles non-standard or informal language used in some credit reports?
Hi James! Thank you for your kind words. Gemini has shown promise in understanding non-standard and informal language to some extent. It has been trained on diverse internet data, which helps it comprehend a wide variety of writing styles. Nonetheless, there might still be cases where additional customization is necessary, especially for specific financial jargon and industry-specific terms.
I believe using Gemini for credit risk assessment could be game-changing, but we must be cautious. How can we ensure the fairness and absence of biases in the system's decision-making process? Are there any specific measures in place to address this?
Hi Laura! You raise an important concern. Bias mitigation is essential in any AI model, including Gemini. It requires careful data curation, bias identification, and systematic evaluation. Google is actively working on reducing biases and increasing transparency. However, it's crucial for organizations to continuously monitor and evaluate the results when deploying such technologies to ensure fairness and accountability.
One potential downside I see with using Gemini for credit risk assessment is the lack of interpretability. How can we trust the decisions made by the model if we can't understand the reasoning behind them?
Hi Sophia! Interpretability is indeed a challenge with modern AI models. Gemini operates as a black box, making it difficult to directly interpret its reasoning. However, research is being done in the field of explainable AI to enhance transparency and interpretability. It's essential to strike a balance between model performance and interpretability, especially in critical domains like credit risk assessment.
Timothy, great article! I'm wondering about the scalability of implementing Gemini in the credit risk assessment process. Can it handle a large volume of credit applications efficiently?
Hi David! Thank you for your feedback. Gemini can indeed scale reasonably well when deployed with the necessary infrastructure. However, handling a large volume of credit applications in real-time requires robust computational resources. Efficient deployment architecture and optimization strategies are crucial to ensure its scalability in credit risk assessment workflows.
I can see the benefits of using Gemini, but what about potential security risks? How can we be confident that malicious actors won't exploit vulnerabilities in the system?
Hi Oliver! Security is a valid concern when implementing AI systems. Google and other organizations take security seriously and employ robust mechanisms to identify and address vulnerabilities. Extensive testing, regular updates, and security audits help ensure the system's resilience. Collaboration between researchers, developers, and security experts is key to proactively mitigate potential risks.
The advancements in AI for credit risk assessment are impressive, but the human touch must not be forgotten. How can we strike the right balance between automated systems and human expertise?
Hi Sophie! That's an excellent point. The ideal approach is to use Gemini as an aid to human experts rather than a replacement. By leveraging the strengths of AI, such as processing large volumes of data and providing insights, credit risk assessment teams can make better-informed decisions efficiently. Human expertise remains invaluable for contextual understanding, subjective evaluation, and assessing unique cases.
Timothy, do you foresee any regulatory challenges in adopting Gemini for credit risk assessment? Are there specific compliance considerations that financial institutions must keep in mind?
Hi Jack! Yes, there might be regulatory challenges and compliance considerations when implementing AI technologies like Gemini. Financial institutions need to ensure compliance with data privacy regulations, transparency requirements, and potential bias issues. Additionally, proper governance frameworks and extensive testing are necessary to meet regulatory mandates and maintain ethical standards.
The potential of Gemini for credit risk assessment is exciting, but how can we effectively manage the integration and adoption of this technology within existing business workflows?
Hi Emily! Integrating Gemini or any AI technology should be approached strategically. It requires a well-defined plan, stakeholder buy-in, and proper change management processes. An incremental adoption approach, starting with specific use cases and gradually expanding, can help manage the integration within existing workflows effectively. Collaboration between technical teams and business units is vital for successful implementation.
Indeed, Timothy. Your article has sparked valuable conversations and highlighted the importance of responsible AI integration.
Timothy, fascinating article! What are the typical challenges faced in implementing Gemini for credit risk assessment in real-world scenarios? Are there common pitfalls organizations should be aware of?
Hi Liam! Thank you for your kind words. Implementation challenges can vary, but some common pitfalls include: lack of sufficient labeled training data, overreliance on AI models without human oversight, interpretability issues, and potential biases. Organizations should also consider the scalability, security, regulatory compliance, and ethical aspects of integrating AI. Awareness of these challenges and careful planning can lead to successful implementation.
I'm concerned about the potential job displacement caused by technologies like Gemini in the finance industry. What can organizations do to prepare employees and reskill them for future roles?
Hi Grace! Job displacement is an important consideration. Organizations should proactively prepare for these shifts by investing in employee training and reskilling programs. Identifying tasks that can be automated and providing opportunities for upskilling in areas like data analysis, decision-making, and domain expertise will help employees transition to new roles effectively. A human-AI symbiosis approach rather than complete replacement is often the best strategy.
Timothy, I enjoyed reading your article! However, I'm concerned about the potential ethical implications of using AI in credit risk assessment. How can companies ensure ethical use of these powerful technologies?
Hi Isabella! Thank you for bringing up ethics. Ethical considerations are crucial when using AI, especially in sensitive domains like credit risk assessment. Companies should establish clear policies and guidelines for the ethical use of AI, regularly assess the system for biases and fairness, prioritize transparency, and work towards avoiding discriminatory outcomes. Collaboration with AI ethics experts and incorporating diverse perspectives is essential for responsible deployment.
Gemini can certainly enhance credit risk assessment, but what are the potential risks associated with overreliance on AI models? How can organizations strike the right balance?
Hi Henry! Overreliance on AI models can pose risks, including lack of human oversight, diminished interpretability, and potential biases. Organizations should approach AI as a tool rather than a complete solution. Establishing a human-in-the-loop approach, incorporating periodic human evaluations, maintaining interpretability measures, and considering AI as an aid to human judgment can help strike the right balance between AI and human expertise.
I'm excited about the possibilities of Gemini in credit risk assessment. However, how can organizations ensure transparency and build trust with customers when using AI-based systems?
Hi Eva! Transparency and trust are crucial for the successful implementation of AI systems. Organizations can ensure transparency by providing clear information on how AI is used in credit risk assessment, explaining the limitations of the system, and offering avenues for customer clarification. Real-time explanations of decisions, when feasible, can also contribute to building trust. Ultimately, effective communication and proactive customer engagement play a significant role in building trust.
Great article, Timothy! How do you envision the future of credit risk assessment with AI? Are there any exciting upcoming advancements we can look forward to?
Hi Aaron! Thank you for your feedback. The future of credit risk assessment with AI looks promising. We can expect advancements in more accurate models through continued research, improved interpretability techniques, increased customization capabilities, and refined methods to tackle biases. Additionally, reinforcement learning and automated data acquisition may further enhance credit risk assessment. Exciting times lie ahead as AI continues to evolve in this field!
Timothy, how does Gemini handle real-time updates and evolving credit risk assessment models? Can it adapt quickly to changing regulations or industry standards?
Hi Lisa! Gemini itself does not handle real-time updates. However, it can be incorporated into systems that are regularly updated to adapt to changing regulations or industry standards. Continuous monitoring, regular model retraining, and efficient update deployment processes are necessary to ensure the system's responsiveness to evolving requirements. The AI technology acts as a component in a larger system that handles real-time updates and model adaptability.
The potential of AI like Gemini in credit risk assessment is enormous, but what data privacy considerations should organizations keep in mind?
Hi Nathan! Data privacy is vital when implementing AI in credit risk assessment. Organizations must comply with applicable data protection regulations and ensure secure handling of sensitive information. Anonymization techniques, data encryption, access controls, and proper consent mechanisms are important aspects to consider. Implementing privacy-by-design principles and conducting thorough impact assessments can help address potential privacy risks effectively.
Gemini indeed has potential, Timothy! However, how can we ensure the system's robustness to adversarial attacks aimed at manipulating credit risk assessments?
Hi Chloe! Robustness to adversarial attacks is an active area of research. To enhance the system's resilience, organizations should focus on adversarial training, where the model is exposed to potential attack scenarios during training. Regular vulnerability testing, incorporating defenses against adversarial inputs, and monitoring system behavior for any anomalies are crucial. By continuously refining the model's defenses, we can strive to improve its robustness to adversarial attacks.
Great article, Timothy! Considering the potential benefits, what challenges do organizations typically face in adopting AI technologies like Gemini for credit risk assessment?
Hi Daniel! Adoption of AI technologies comes with a unique set of challenges. Some common ones include the need for domain-specific fine-tuning, data availability and quality, model interpretability, potential biases, privacy concerns, regulatory compliance, and integrating AI systems into existing workflows. Organizations must carefully address these challenges through collaboration between business and technical teams, ensuring proper resourcing, and developing a comprehensive implementation strategy.
Timothy, as AI systems advance, research on ethical considerations is also expanding. Are there any specific ethical frameworks or guidelines you recommend for organizations using Gemini in credit risk assessment?
Hi Sophie! Ethical frameworks and guidelines provide valuable insights into responsible AI deployment. Notable ones include the IEEE Ethically Aligned Design, the EU's Ethics Guidelines for Trustworthy AI, and the AI Code of Ethics by professional organizations. These frameworks emphasize fairness, transparency, accountability, and avoiding discriminatory outcomes. Organizations should adapt and customize these guidelines to their specific contexts while considering their stakeholders' perspectives.
Gemini could be transformative in credit risk assessment, Timothy! However, how can organizations ensure proper governance and accountability when AI systems make crucial decisions?
Hi Maxwell! Proper governance and accountability are essential to address decision-making by AI systems. Establishing clear ownership, responsibilities, and decision frameworks is crucial. Regular auditing, transparency mechanisms, monitoring for bias, and involving designated oversight teams can help ensure proper governance. Additionally, organizations should have mechanisms for handling biases, providing explanations, and enabling appeals or human intervention in critical decisions to enhance accountability.
Great article, Timothy! How can organizations measure the impact of incorporating AI like Gemini into their credit risk assessment processes?
Hi Ava! Measuring the impact of AI in credit risk assessment is crucial to assess its effectiveness. Organizations can track key performance indicators (KPIs) such as accuracy improvements, time savings, reduction in manual efforts, and consistency in decision-making. Comparative analysis with pre-AI workflows can help quantify the benefits. Furthermore, gathering feedback from credit assessment teams, monitoring downstream impacts, and refining the system iteratively contribute to measuring the impact.
Gemini presents promising opportunities, Timothy! Are there any known biases that organizations should be cautious about when using AI in credit risk assessment?
Hi Sophia! Biases in AI systems are a concern. In credit risk assessment, organizations should be cautious about several biases, such as gender, race, or socioeconomic status. Biases might be present due to historical data patterns, uneven representation in training data, or systemic issues. Regular bias assessments, diverse training data, and ongoing monitoring can help identify potential biases and rectify them to ensure fairness and equitable credit assessments.
Timothy, fascinating article! How can organizations address the challenges of data quality and availability when implementing AI technologies in credit risk assessment?
Hi Connor! Data quality and availability are vital for reliable AI systems. Organizations should invest in data preprocessing techniques to handle incomplete or inconsistent datasets. Collaboration with data providers and proper data governance can enhance data quality and availability. In cases where data might be limited, techniques like transfer learning or synthetic data generation can be explored. Ultimately, a multidisciplinary approach combining analytics, data science, and subject matter expertise is crucial for addressing data challenges.
Gemini has enormous potential in credit risk assessment, Timothy! What are the primary factors organizations should consider while selecting AI models for their specific needs in this domain?
Hi Joshua! When selecting AI models for credit risk assessment, organizations should consider factors such as model performance on relevant metrics, compatibility with their existing infrastructure, scalability, interpretability, and adaptability to domain-specific requirements. The availability of pretrained models, fine-tuning capabilities, and research support are also important. Conducting proof-of-concept experiments, benchmarking different models, and involving domain experts in the selection process can aid in finding the most suitable AI model.
Great article! I never thought about using chatbots for credit risk assessment before.
Indeed, Sarah! Chatbots have the potential to revolutionize so many industries.
Absolutely, Robert! The advancements in natural language processing have opened up endless possibilities.
I wonder if Gemini is capable of handling complex scenarios that involve multiple risk factors.
That's an interesting point, Mark. I'm also curious about the level of accuracy it can achieve.
Gemini's ability to process large amounts of data and learn from it should contribute to accurate risk assessment.
I've had some experience with AI-driven credit risk assessment tools, and they have greatly improved accuracy compared to traditional methods.
Daniel, can you share more about your experience? I'm interested in hearing some real-world examples.
I agree, Evelyn. Real-world examples would be helpful in understanding the practical applications of Gemini in credit risk assessment.
One example is when an AI chatbot identified inconsistencies in an applicant's financial information, leading to the discovery of potential fraud.
That's impressive! AI-based tools can really enhance fraud detection while saving time and resources.
Absolutely, Evelyn. It's fascinating how technology continues to advance in tackling financial risks.
I wonder if there are any ethical considerations to be aware of when employing AI for credit risk assessment.
Linda, ethics should definitely be a prime focus. Bias and discrimination could be significant concerns if not properly addressed in the development and implementation of such tools.
I agree, Emily. Developers and users should be vigilant about potential biases and regularly evaluate the fairness of the system.
Inclusivity is crucial as well. AI tools should be designed to consider a diverse range of inputs to avoid exclusionary practices.
You are absolutely right, Robert. AI should strive to create a more inclusive and fair credit assessment process.
I wonder what the potential impact of AI-driven credit risk assessment will be on job opportunities in the banking industry.
AI may reduce the need for manual assessment, but it can also create new job roles involving AI oversight and refinement.
That's a valid point, Oliver. Human expertise will still be valuable in ensuring the accuracy and ethical use of AI in credit risk assessment.
I believe AI can complement human expertise rather than replace it. It can assist in handling large datasets and complex calculations.
As long as the human factor remains crucial, the integration of AI should lead to more efficient and effective credit risk assessment processes.
I worry about data security. How can we ensure that sensitive financial information is protected when using AI-powered tools?
Claire, data security is indeed paramount. Encryption, secure servers, and implementing strict access controls are some methods to safeguard confidential data.
Additionally, adherence to data privacy regulations and obtaining user consent for data usage are critical for maintaining trust.
I appreciate this discussion. It's valuable to consider the potential benefits and challenges associated with implementing AI in credit risk assessment.
I agree, Mark. It's important to have open discussions to ensure responsible and effective integration of AI technology.
Indeed, Evelyn. Collaboration between industry experts, developers, and regulators is key in navigating the transformation brought by AI.
I'm impressed with how Gemini can revolutionize credit risk assessment. The possibilities are endless!
Absolutely, Hannah! It's exciting to witness the innovation and transformation happening in the field of credit risk assessment.
The potential impact of AI in this industry is immense. It's great to see financial institutions embracing technological advancements.
I'm delighted to see that AI-driven solutions are spreading to various sectors, enhancing efficiency while making accurate risk assessment more accessible.
Emily, the emerging technologies indeed hold great promise for streamlining processes and improving outcomes.
The integration of AI technologies in credit risk assessment demonstrates the need for continuous learning and adaptation in the finance industry.
Absolutely, Robert! Staying ahead of the technological curve is crucial to thrive in today's fast-paced world.
I'm glad to see that AI-driven technology is being used for more than just customer service. This can bring immense value to the finance industry.
Amy, you're right. AI is transforming various aspects of the finance industry, from fraud detection to risk assessment.
However, we should ensure that the benefits of AI-driven credit risk assessment are accessible to a wide range of financial institutions, including smaller ones.
Daniel, I completely agree. The adoption of these technologies should not widen the existing gaps between larger and smaller institutions.
The democratization of AI and ensuring its affordability is crucial for equitable progress in the finance industry.
This article has provided great insights into the advancements happening in credit risk assessment. Exciting times lie ahead!
Indeed, Claire! It's an exciting time to be part of the ever-evolving intersection of finance and technology.
I appreciate the author's clear explanation of how Gemini can empower credit risk assessment. Well-written article!
Yes, Linda. Kudos to Timothy Hoke for shedding light on the potential of chatbots in revolutionizing credit risk assessment.
I thoroughly enjoyed reading this article and the ensuing discussion. It's exciting to witness the positive impact of AI in the banking sector.
I couldn't agree more, Hannah. AI has the potential to bring significant improvements to the finance industry.
Thank you, everyone, for engaging in this insightful discussion. Your perspectives contribute to a better understanding of the potential of AI in credit risk assessment.
Thank you, Timothy, for sharing your expertise with us. It was a pleasure learning more about the applications of AI in credit risk assessment.
Thank you all for your thoughtful participation. Let's continue enhancing our collective knowledge and shaping the future of credit risk assessment.