Empowering Predictive Modeling in Tournaments with ChatGPT: Revolutionizing the Competitive Tech Sphere
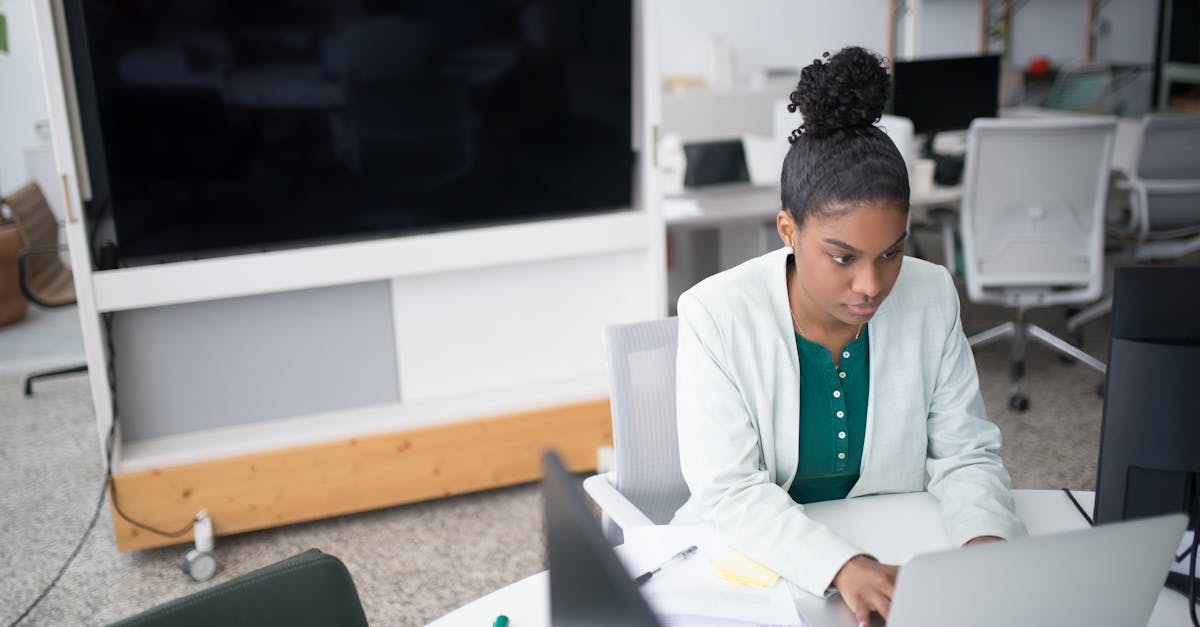
Tournaments have been a popular form of competition for as long as human civilization has existed. From ancient gladiatorial battles to modern-day esports events, people have always been fascinated by the thrill of watching highly skilled individuals or teams compete against each other for supremacy.
With the advancement of technology and the availability of large amounts of data, predictive modeling has emerged as a valuable tool to analyze and predict tournament outcomes. Predictive modeling, in the context of tournaments, refers to the process of using historic match data to create models that can accurately predict the outcome of future games.
Usage of Predictive Modeling in Tournaments
Predictive modeling in tournaments has found applications in various fields, including sports, gaming, and finance. One of the most common uses is in sports analytics, where predictive models are employed to forecast the outcome of games in sports such as soccer, basketball, and tennis.
In the gaming industry, predictive modeling can be used to create models for competitive video games, such as Dota 2 or League of Legends. By analyzing past match data, these models can provide insights into the performance of players or teams, helping fans and bettors make informed predictions.
Additionally, predictive modeling has been used in financial sectors to predict stock market movements during trading tournaments or to forecast the outcome of investment competitions. By analyzing historical data, traders and investors can make more informed decisions and increase their chances of success in these high-stakes environments.
How Predictive Modeling Works
Predictive modeling in tournaments typically involves the following steps:
- Collecting and preprocessing data: Historic match data is collected and prepared for analysis. This may include cleaning the data, removing outliers, and transforming variables.
- Feature engineering: Relevant features that can influence game outcomes are selected or derived from the data. These features could include players' past performance, team composition, or environmental factors.
- Model selection: A suitable predictive model is chosen based on the nature of the data and the specific problem being addressed. Common models include decision trees, logistic regression, and neural networks.
- Training the model: The selected model is trained using the prepared data. This involves feeding the model with a labeled dataset, where the outcome of each game is known, and allowing it to learn the patterns and relationships between the features and the desired outcome.
- Evaluating and fine-tuning the model: The trained model is evaluated using a separate test dataset to assess its accuracy and performance. If necessary, the model may be fine-tuned by adjusting its parameters or retraining it on different data subsets.
- Deploying the model: Once the model is deemed accurate and reliable, it can be deployed to predict the outcome of future games. This can be done in real-time or on-demand, depending on the application and requirements.
Predictive modeling in tournaments can provide valuable insights and assist in making more informed decisions. However, it is important to note that no model is perfect, and there will always be uncertainties and factors beyond the scope of the data analyzed. It is crucial to interpret the model's predictions with caution and consider other relevant factors when making decisions based on the predictions.
Conclusion
Predictive modeling in tournaments using historic match data has emerged as a powerful tool in various domains. Its applications range from sports analytics to gaming and finance. By leveraging the power of data analysis and machine learning algorithms, predictive models can help enthusiasts, bettors, and professionals make more accurate and informed predictions about tournament outcomes. Although these models are not foolproof, they contribute valuable insights and enhance decision-making processes in tournament settings.
Comments:
This article on empowering predictive modeling in tournaments with ChatGPT is fascinating! It's incredible to see how AI is revolutionizing the competitive tech sphere.
Indeed, Alice! The advancements in predictive modeling can bring a new level of efficiency and accuracy to various tournament scenarios. Exciting times ahead!
As a data scientist, I'm thrilled about the potential of ChatGPT in tournaments. Can't wait to explore the possibilities it offers.
I agree, Charlie! Having AI-powered predictive modeling can definitely enhance decision-making and make tournaments more competitive and engaging.
This is a game-changer! Integrating ChatGPT in tournaments will provide valuable insights and strategies, improving the overall experience for participants.
Agreed, Eve! Gaming tournaments could also benefit from ChatGPT's predictive modeling, enhancing the strategic aspect of gameplay.
Jack, absolutely! In gaming tournaments, predictive modeling can assist players in analyzing opponents' moves, adapting strategies, and predicting their next moves.
Charlie, I can imagine AI-powered strategy recommendations during gaming tournaments taking the competition to a whole new level. It'll be thrilling!
Thank you all for your positive comments! The potential of ChatGPT in predictive modeling for tournaments is indeed groundbreaking. Let's further discuss the specifics and implications.
I'm curious about the types of tournaments where ChatGPT could have the most impact. Any specific examples come to mind?
With ChatGPT, I think prediction-based sports tournaments could greatly benefit. AI-informed strategies can provide an edge in games like chess or poker.
I wonder if ChatGPT's predictive modeling could be used to improve forecasting in financial tournaments where market predictions matter?
Absolutely, Hannah! Financial competitions could leverage ChatGPT's insights to make more informed decisions based on market trends and patterns.
Frank, George, Hannah, and Ivy, excellent points! ChatGPT can indeed be applied across various tournament domains such as sports, finance, and gaming to empower predictive modeling.
Hannah, ChatGPT in forecasting financial tournaments can also aid risk assessment by analyzing market trends and predicting potential downside scenarios.
I'm curious about the potential ethical considerations surrounding the use of AI like ChatGPT in tournaments. Any thoughts on that?
Kate, you make a valid point. We need to ensure responsible AI use and address any potential biases and fairness concerns when utilizing predictive models in competitions.
I completely agree, Liam! Ethics should be a priority when deploying AI systems like ChatGPT in tournaments to avoid any unintended negative consequences.
Ethical guidelines and rigorous testing are crucial in maintaining a fair playing field while leveraging AI-powered predictive modeling in tournaments.
Nathan, I couldn't agree more! Transparency and explainability are crucial in AI-powered competitions. Participants should understand how the AI models inform decisions.
Xavier, transparency is key not only in AI competitions but also to foster trust and acceptance of AI's role across various domains and industries.
Kate, Liam, Mia, and Nathan, you've highlighted an important aspect. Ensuring ethics and fairness are maintained is essential in the widespread adoption of AI models in competitions.
General Line, indeed, transparency helps build trust among participants and ensures a level playing field. Keeping AI decision-making explainable is essential.
Yara, absolutely! Explaining AI decisions and promoting transparency will not only build trust but also encourage the responsible adoption of AI in various sectors.
Jack, you're spot on! By establishing transparency and promoting understanding, AI will find broader acceptance and integration in our daily lives.
I can imagine ChatGPT being used in eSports tournaments to analyze player performance data and generate insights for better training strategies.
Olivia, that's a great point! AI-driven performance analysis can provide valuable feedback to players and teams, improving their skill levels and overall competitiveness.
Peter, I can also see ChatGPT being used in board game tournaments, analyzing past moves to detect patterns and suggest optimal strategies.
Zara, that's an interesting idea! Analyzing historical gameplay data can help participants improve their skills and adapt strategies in board game tournaments.
Bob, building upon your point, analyzing past moves in board games can also lead to AI-assisted matchmaking, creating fairer and more evenly balanced tournaments.
Zara, your idea about using ChatGPT in board game tournaments reminds me of how AI has been utilized in chess tournaments. It's exciting to think about the possibilities in other games!
Additionally, in eSports broadcasting, ChatGPT's predictive modeling can offer real-time analysis and highlight key moments, enhancing the viewing experience.
That's true, Quinn! Real-time analysis during eSports broadcasts with ChatGPT can engage viewers by offering deeper insights, similar to sports commentators.
Within financial tournaments, ChatGPT's predictive capabilities could assist participants in making well-informed investment decisions, bringing greater transparency.
I think it's crucial to consider potential bias in the data used to train ChatGPT for predictive modeling in tournaments. Bias can impact fairness.
Tom, you're absolutely right! Bias in the training data can perpetuate unfair advantages or disadvantages, which must be carefully addressed when implementing AI models.
Tom, addressing bias is indeed crucial. Adopting fairness measures during the training and deployment of AI models is essential to ensure impartiality.
Ethical use of AI like ChatGPT also involves continuous monitoring and evaluation to identify and mitigate any biases that might arise during its deployment.
William, continuous monitoring and evaluation can help identify any shortcomings or biases, enabling the necessary adjustments for responsible AI use.
Overcoming biases requires diversity in the development teams and rigorous scrutiny of the training datasets to ensure a fair and inclusive playing field.