Empowering Production Engineering with ChatGPT: Transforming Technology Through Natural Language Processing
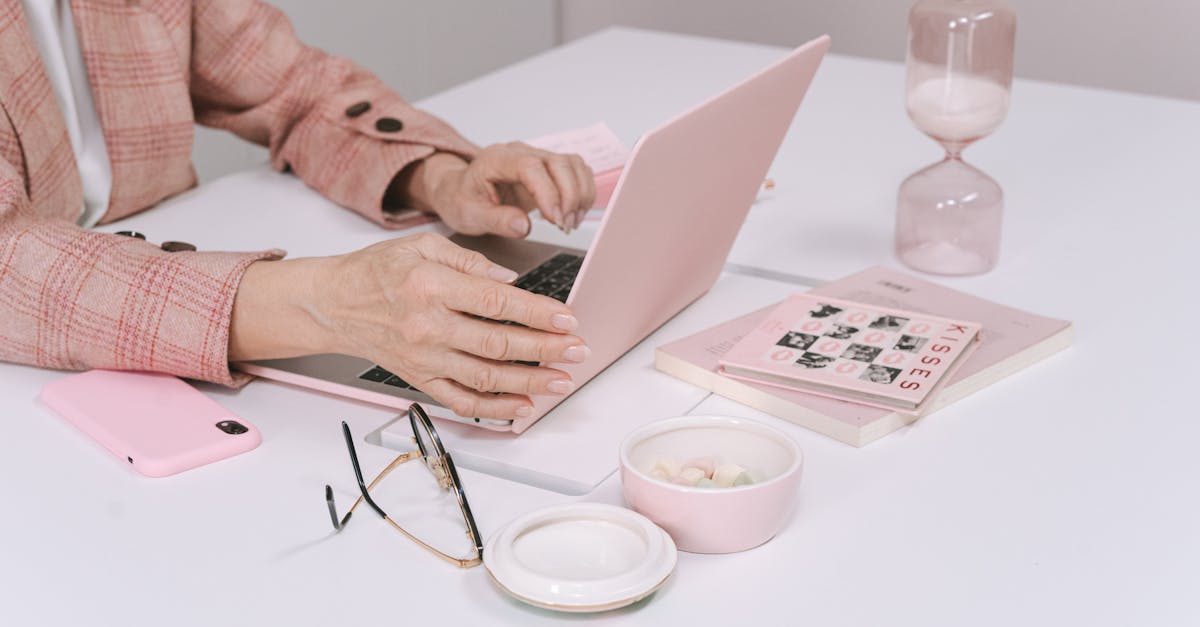
Production Engineering is a multifaceted field incorporating several areas of expertise. One such area is design optimization, which concentrates on enhancing product design and functionality while reducing costs. This article will explore how an intriguing technological tool, namely, ChatGPT-4, could potentially revolutionize production engineering, particularly in the area of design optimization.
What is Production Engineering?
Production engineering is a branch of engineering centered on manufacturing and production processes. It manages and optimizes complex production systems, primarily by drafting, analyzing, and testing designs to ensure they are as productive and efficient as possible.
Design Optimization in Production Engineering
The focus of design optimization is to refine a product's design to enhance overall performance while cost-effectively utilizing resources. Using various analytic techniques, engineers can determine the most efficient materials, sizes, shapes, and configurations for a product, adjusting them to improve the product's functionality, durability, and manufacturing efficiency.
Role of Chatgpt-4 in Design Optimization
ChatGPT-4, developed by OpenAI, is an advanced autoregressive language model that uses machine learning to produce human-like text. By training on a wide range of internet text, it can complete prompts given to it in a manner consistent with how a human might respond, offering new possibilities for user interaction.
Design optimization is a process that thrives on data: the more, the better. This is where ChatGPT-4 shines. Implementing AI systems like ChatGPT-4 can provide an in-depth analysis based on extensive data. One of the prominent features of the ChatGPT-4 is its ability to analyze and suggest optimizations for a given design based on the previous successful models. By learning from past successes, it can offer invaluable insight into improving design practices and production processes. However, the advantages don't stop there.
Speed and efficiency are also crucial factors in design optimization. Manually checking and adjusting design parameters can be a time-consuming process. By contrast, AI can quickly analyze vast amounts of data, making adjustments as needed and accelerating the optimization process.
Additionally, the use of predictive analytics by AI can identify potential issues early in the design stages, allowing engineers to proactively refine their designs and eliminate problems that could otherwise lead to wasted resources or suboptimal products.
Moreover, ChatGPT-4 can analyze trends in designs over time, understanding the evolution of a product's design, and offer suggestions based on this historical data. In an era where companies often iterate on designs to improve them over time, this benefit cannot be understated.
All these advantages culminate in a potent tool for design optimization, with ChatGPT-4 proving itself capable of automated data analysis, identification of key trends, and prediction of successful design strategies based on historical data.
Conclusion
By incorporating AI technologies like ChatGPT-4 into our design optimization process in production engineering, we open a world of possibilities. The potential for AI in this regard is vast and continuously evolving. As we continue to refine and develop AI technologies, the benefits to our production processes will undoubtedly continue to grow.
Comments:
Thank you all for your interest in my article on empowering production engineering with ChatGPT! I'm thrilled to see such enthusiasm. Please feel free to share your thoughts and ask any questions you may have.
This is a fascinating application of natural language processing to transform technology. I can see how ChatGPT can greatly benefit production engineering. How do you handle domain-specific terminology and jargon?
Great question, Lynn! ChatGPT can indeed handle domain-specific terminology and jargon. One way is by fine-tuning the model on a specific domain's dataset, which helps it learn the particular language used. Additionally, using prompts and clarifying queries can aid in ensuring accurate responses.
I'm curious about the potential challenges in implementing ChatGPT in a production engineering environment. Can you provide some insights on that, Aldo?
Certainly, Emma! While ChatGPT has shown great potential, there are some challenges to consider. First, ensuring the accuracy and reliability of responses is crucial. Secondly, handling and flagging any potential biases in the language model is important. Lastly, refining the model over time based on user feedback can help improve its performance in specific engineering contexts.
I can see the benefits of using ChatGPT, but I'm concerned about security and privacy. How can we address those concerns when implementing this technology?
Valid point, Mark. Security and privacy should always be taken into account. By implementing strict access controls, encryption mechanisms, and anonymizing user data, we can mitigate potential risks. Conducting regular security audits and complying with industry-standard protocols can help maintain a secure and private environment.
As a production engineer, I'm excited to see how ChatGPT can streamline processes and improve efficiency. Are there any success stories or real-world examples you can share?
Absolutely, Sophia! One success story is a manufacturing company that implemented ChatGPT in their production engineering department. It reduced the time spent on troubleshooting and problem-solving, leading to faster production cycles and improved overall efficiency. The engineers involved also reported higher satisfaction with the support provided by ChatGPT.
I've heard concerns about AI replacing human jobs. Do you think ChatGPT will have any negative impacts on the job market for production engineers?
That's a valid concern, Jason. While ChatGPT can automate certain tasks, it's important to note that it's designed to augment human capabilities, not replace jobs. By handling repetitive and routine queries, engineers can focus on more complex problem-solving and analysis. Ultimately, it can enhance productivity and enable engineers to perform at their best.
This article opened my eyes to the possibilities of NLP in production engineering. I'm curious about the scalability of ChatGPT. Can it handle a large number of simultaneous queries?
Indeed, scalability is crucial. ChatGPT can handle a significant number of simultaneous queries, thanks to its parallelizable architecture. By distributing the load across multiple instances and optimizing resource allocation, it can effectively handle high query volumes while maintaining responsiveness.
I've seen AI chatbots that sometimes generate inaccurate or nonsensical responses. How does ChatGPT ensure the quality of its answers in a production engineering context?
You raise a valid concern, Liam. ChatGPT has undergone an extensive training process and is continually refined based on feedback. However, ensuring quality responses in a production engineering context requires regular monitoring, user feedback, and fine-tuning to improve accuracy. It's an iterative process to maintain the quality of answers over time.
I'm impressed with the potential applications of ChatGPT in production engineering. How can one get started with implementing it in their organization?
Glad to hear your interest, Julia! Getting started with implementing ChatGPT involves assessing your organization's needs, identifying use cases, and determining the resources required for implementation. It's important to collaborate with relevant stakeholders, including production engineers, to ensure a successful integration. Seeking expert guidance and conducting thorough testing can pave the way for a smooth implementation process.
I'm concerned about potential biases in the language model. How do you address bias, especially in a production engineering context?
Addressing bias is indeed paramount. It involves training the model on diverse datasets, implementing inclusive data collection practices, and being mindful of the potential biases present in the training data. Ongoing evaluation processes and involving diverse perspectives during the model's development and testing phases can help identify and rectify biases, ensuring fair and unbiased responses in the production engineering context.
I'm excited to see the positive impact ChatGPT can bring to production engineering. Are there any limitations or areas where it may not be as effective?
Good question, Michelle. ChatGPT excels in various applications, but it does have limitations. It may struggle with ambiguous queries or those lacking sufficient context. Additionally, it's essential to validate its responses, especially when addressing critical or safety-related matters. Human oversight and feedback remain crucial for ensuring the best results and mitigating any potential limitations.
This article highlights the potential of NLP in revolutionizing production engineering. How do you see this technology shaping the future of the industry?
Great question, Natalie. ChatGPT and other NLP technologies have the potential to enhance productivity, provide real-time insights, and streamline processes in production engineering. By empowering engineers with better access to information, it can drive innovation, improve efficiency, and lead to more informed decision-making. The future of the industry will likely involve the seamless integration of NLP and other advanced technologies to unlock new possibilities.
Do you have any recommendations on how to evaluate the performance and reliability of ChatGPT in a production engineering setting?
Indeed, evaluating performance and reliability is crucial. Here are a few recommendations: 1) Establish clear evaluation metrics, such as accuracy and response time. 2) Create a robust test dataset that covers a wide range of possible queries. 3) Compare ChatGPT's responses with those from human experts to validate accuracy. 4) Collect user feedback and iterate on the model to improve its performance over time.
I find it impressive how ChatGPT can understand and generate human-like responses. Can you explain the underlying technology briefly?
Certainly, Lisa! ChatGPT is built upon a deep learning model called the Transformer architecture. It uses a technique known as self-attention, enabling it to analyze input sequences and generate coherent, context-aware responses. The model is trained on large-scale datasets and learns to predict the likelihood of words given their context. Through this process, it gains a strong understanding of language and can generate human-like responses.
Given the constant advancements in NLP and AI, do you have any predictions for the future of ChatGPT in the production engineering domain?
That's an interesting question, Jonathan. With ongoing advancements, I expect ChatGPT to become even more accurate, reliable, and tailored to the specific needs of production engineering. Its ability to handle complex queries and provide valuable insights will likely continue to improve. We can anticipate further integration with production engineering platforms, driving efficiency and innovation in the industry.
I appreciate the potential value ChatGPT can offer in production engineering. How do you ensure the model understands the context correctly to provide accurate answers?
Thanks for the question, Emily. Ensuring accurate context understanding is indeed crucial. Techniques like providing more explicit context in user prompts and using clarifying questions can help reduce ambiguity. Additionally, feedback loops and continuous training on real-world data can further improve the model's ability to understand context and provide accurate answers in production engineering scenarios.
This article shed light on the potential of ChatGPT in production engineering. Are there any considerations or best practices to keep in mind when interacting with ChatGPT?
Indeed, Oliver. When interacting with ChatGPT, it's important to: 1) Ask clear and specific questions to receive accurate responses. 2) Be aware of the model's limitations and cross-validate critical information. 3) Provide sufficient context when necessary to aid understanding. 4) Give feedback on incorrect or misleading answers to help improve the model's performance. Following these best practices can enhance the overall experience of interacting with ChatGPT.
I find this technology truly fascinating. How can ChatGPT be used to improve collaboration among production engineers?
Great question, Sophie. ChatGPT promotes collaboration by providing a centralized knowledge base and an accessible platform for production engineers to seek information and share insights. It can facilitate the exchange of ideas, best practices, and troubleshooting assistance. By making information readily available, it empowers engineers to collaborate more effectively and work towards common goals.
It's amazing how AI technology can transform production engineering. How do you plan to address any potential ethical concerns associated with the use of ChatGPT?
Ethical considerations are of utmost importance. We plan to address them by implementing transparent practices, ensuring user privacy, handling bias through inclusive training, and seeking external audits. Incorporating ethics and compliance into the development and deployment process will help us navigate potential challenges and ensure responsible and ethical use of ChatGPT in the production engineering domain.
As a production engineer, I'm excited about the potential of ChatGPT. What are the key steps to successfully implement this technology in an organization?
Glad to hear your excitement, Emma! Key steps for successful implementation include: 1) Assessing your organization's needs and use cases. 2) Collaborating with relevant stakeholders, including engineers, to define requirements. 3) Allocating necessary resources for integration and maintenance. 4) Conducting thorough testing and user feedback sessions. By following these steps, organizations can maximize the benefits of ChatGPT for production engineering.
Finding accurate information quickly is crucial in production engineering. How does ChatGPT ensure reliable and up-to-date responses?
You're absolutely right, Benjamin. ChatGPT's reliability and up-to-date responses are ensured through continuous training on real-world data. By leveraging the latest information, it stays knowledgeable about current practices and can provide reliable answers to production engineering queries. Ongoing monitoring and feedback loops also help to identify and rectify any outdated or incorrect responses, ensuring the information remains accurate.
I'm curious about the level of technical expertise required to interact effectively with ChatGPT. Can non-technical users benefit from this technology in production engineering?
Great question, Stella. ChatGPT is designed to be accessible to both technical and non-technical users. While technical expertise can help users in framing complex queries or understanding the limitations of the model, non-technical users can still benefit from ChatGPT by seeking assistance, troubleshooting, or getting answers to more straightforward production engineering questions. It's a tool that aims to augment users' capabilities, regardless of their technical background.
The implications of ChatGPT in production engineering are promising. How do you handle potential biases that might exist in the data used to train the model?
Addressing biases is a critical aspect of model development. When training ChatGPT, we actively work toward using diverse and representative datasets. We take measures to mitigate any biases that might already exist in the data by carefully curating and reviewing it. Additionally, continuous feedback, external input, and iterative improvement processes help in identifying and rectifying biases, ensuring the model's responses are fair and unbiased in the production engineering context.
Will ChatGPT be able to handle complex engineering calculations and simulations in a production engineering setting?
Complex engineering calculations and simulations pose challenges even for advanced AI models. While ChatGPT may not be suitable for performing complex calculations directly, it can assist engineers by providing insights into methodologies, guiding them to appropriate resources, or even suggesting relevant formulas or approaches. Pairing ChatGPT with specialized engineering tools can be an effective way to handle complex calculations and simulations in a production engineering setting.
This article got me thinking about the potential impact of ChatGPT on training new production engineers. Can it be used as an educational tool?
Great point, Grace. ChatGPT can indeed be used as an educational tool for training new production engineers. By providing instant access to information, troubleshooting guidance, and best practices, it can help accelerate the learning process. However, it's crucial to supplement ChatGPT with formal training, experience-based learning, and hands-on mentorship. The model can serve as a valuable resource, but it should complement a comprehensive educational framework.
I'm impressed by the potential of ChatGPT in production engineering. How can engineers provide feedback to improve the model's performance?
Engineer feedback is vital to improving the model's performance. Engineers can provide feedback by submitting corrections, pointing out inaccuracies, or suggesting improvements to responses given by ChatGPT. User feedback helps identify any weaknesses or areas of improvement, which can then be incorporated in the training process to enhance the model's performance over time. Collaborative feedback loops play a critical role in continuously refining and fine-tuning ChatGPT for production engineering.
Can ChatGPT be integrated with existing production engineering systems or platforms? How can engineers leverage its benefits alongside their current tools?
Absolutely, Liam. ChatGPT can be integrated with existing production engineering systems or platforms. Engineers can leverage its benefits by incorporating it as a support tool within their current tools or as an additional module for real-time insights. Integration can be done via APIs or custom interfaces, allowing engineers to seamlessly access ChatGPT's capabilities while working within their familiar environments.
I'm intrigued by the potential of ChatGPT in production engineering. How does the model handle complex and context-dependent manufacturing processes?
Handling complex and context-dependent manufacturing processes is a challenge that requires a combination of advanced models and domain expertise. ChatGPT can provide insights, offer troubleshooting guidance, and answer questions related to manufacturing processes. However, the model's responses should always be cross-validated with domain experts and verified against existing manufacturing guidelines and standards to ensure accuracy and safety in production engineering.
I'm intrigued by the potential applications of ChatGPT in production engineering. Are there any plans to integrate it with virtual assistants or voice-enabled platforms?
Indeed, Oliver. Integrating ChatGPT with virtual assistants or voice-enabled platforms is an exciting direction. It can enhance the accessibility of production engineering knowledge in real-world scenarios. By enabling voice-based interactions or integrating with voice-enabled platforms, production engineers can have hands-free access to ChatGPT's capabilities, making it even more convenient and seamlessly integrated into their workflows.
I'm concerned about the potential misuse of ChatGPT. What measures are in place to prevent malicious use or misinformation?
Preventing malicious use and misinformation is a priority. OpenAI works diligently to reduce possible risks by implementing safety mitigations, using human reviewers during the training process, and improving default behaviors to avoid harmful responses. Additionally, user feedback is crucial for identifying and resolving any issues promptly. OpenAI actively collaborates with the user community to enhance safety protocols and ensure responsible deployment of ChatGPT in production engineering and other domains.
I'm curious about the computational resources required to run ChatGPT effectively. What are the hardware and infrastructure considerations?
Good question, Jack. Running ChatGPT effectively requires sufficient computational resources. Depending on the scale of implementation, organizations may consider using powerful GPUs or distributed computing frameworks to handle the model's computation needs. Additionally, optimizing infrastructure for efficient load distribution, scalability, and responsiveness is essential to provide seamless performance when using ChatGPT in a production engineering environment.
I'm impressed by ChatGPT's capabilities. Can it handle industry-specific standards and regulations that apply to production engineering?
Absolutely, Sophie. ChatGPT can handle industry-specific standards and regulations used in production engineering. By incorporating relevant information during the training process and cross-validating responses against established guidelines, it can ensure adherence to necessary standards. However, compliance with industry-specific regulations and guidelines should always involve human oversight and domain expertise to guarantee the accurate interpretation and application of those standards.
I can see the potential for ChatGPT in production engineering, but what are the main limitations of this technology?
Great question, Emma. While ChatGPT is a powerful tool, it does have some limitations. It may generate responses that are plausible-sounding but incorrect or make unjustified inferences. It can be sensitive to input phrasing, and a slight variation in the query might lead to different responses. Additionally, ChatGPT may require careful handling to address safety and ethical concerns. Recognizing these limitations is crucial for making informed and contextual use of ChatGPT in production engineering.
The potential of ChatGPT in production engineering is exciting. Are there any plans to make it open-source or offer a self-hosted version for organizations?
OpenAI has plans to improve upon ChatGPT and make subsequent models more accessible. While the details about open-source or self-hosted versions are yet to be decided, OpenAI is exploring options to provide increased availability and customization to better serve the needs of organizations using ChatGPT in various domains, including production engineering.
I'm excited about the potential of ChatGPT to enhance production engineering. How do you see it complementing other AI technologies in the field?
Great question, Grace. ChatGPT can complement other AI technologies in production engineering with its natural language processing capabilities. It can work alongside advanced analytics, simulation tools, and optimization algorithms to provide human-like interactions and assist engineers with information retrieval, troubleshooting, decision support, and data analysis. By integrating various AI technologies, production engineering can benefit from a holistic approach that leverages the strengths of each tool.
This article has sparked my interest in ChatGPT's potential. How do you foresee its evolution and improvement in the coming years?
Glad to hear your interest, Oliver. Looking ahead, I expect ChatGPT to continue evolving and improving. Ongoing research and fine-tuning, along with user feedback, will drive its advancement. OpenAI's commitment to safety, transparency, and collaboration will ensure responsible development. With time, we can anticipate even more accurate, reliable, and domain-specific versions of ChatGPT that cater to the unique needs of production engineering and other industries.
I'm excited to explore the possibilities of ChatGPT in production engineering. Are there any resources available to learn more about this technology?
Certainly, Ella! To learn more about ChatGPT and its applications in production engineering, I recommend visiting OpenAI's website. They provide detailed information, research papers, and resources related to the development, capabilities, and responsible use of ChatGPT. Exploring the broader NLP domain and staying updated with advancements in AI can also foster a deeper understanding of ChatGPT's potential in various industries.
Considering the dynamic nature of production engineering, how does ChatGPT handle rapidly evolving industry practices and emerging technologies?
Adapting to rapidly evolving industry practices and emerging technologies is vital for ChatGPT's effectiveness. By training the model on up-to-date datasets and receiving continuous user feedback, ChatGPT can stay informed about the latest trends, best practices, and technologies in production engineering. Collaboration with industry experts and integration of real-time knowledge sources can further enhance its ability to address the constantly changing landscape of the industry.
This article highlighted the immense potential of ChatGPT in production engineering. How do you strike a balance between providing helpful responses and avoiding overwhelming users with excessive information?
Striking a balance is crucial, Natalie. ChatGPT can avoid overwhelming users by providing concise and relevant responses that directly address the query. User prompts can be tailored to extract the necessary information effectively. Additionally, implementing features like progressive disclosure, where the model asks clarifying questions to understand the user's intent better, can help in avoiding unnecessary or excessive information while ensuring helpful responses in a production engineering context.
ChatGPT seems like a powerful tool. How can it be used to promote continuous learning and professional development among production engineers?
ChatGPT can be a valuable resource for continuous learning and professional development among production engineers. It can offer insights into new methodologies, provide access to up-to-date best practices, and help engineers stay updated with the latest advancements in the field. By serving as an accessible knowledge base and engaging platform, ChatGPT can foster an environment of continuous learning, enabling engineers to expand their skills and stay at the forefront of production engineering practices.
I'm intrigued by ChatGPT's potential impact on production engineering workflows. How does it handle complex production schedules and resource allocation?
Handling complex production schedules and resource allocation requires a combination of AI models, optimization algorithms, and domain expertise. While ChatGPT can assist in providing guidance and suggestions, it's advisable to integrate it with specialized production planning and resource allocation tools. By leveraging the natural language processing capabilities of ChatGPT, engineers can interact with such tools more intuitively, facilitating efficient decision-making and optimizing production engineering workflows.
I'm excited about the possibilities of ChatGPT. How do you envision its impact beyond production engineering?
Good question, Oliver. ChatGPT's impact can extend beyond production engineering to various other fields. Industries like customer support, content generation, and knowledge acquisition can benefit from its capabilities. Additionally, incorporating ChatGPT with virtual assistants, voice-enabled platforms, or educational tools can revolutionize the way people interact with information. The broader impact of ChatGPT lies in making AI-driven insights and support more accessible and human-like across diverse domains.
I have concerns about the model answering sensitive or confidential queries. How can confidentiality and data privacy be maintained when using ChatGPT?
Maintaining confidentiality and data privacy is essential. By applying strict access controls and encryption mechanisms, organizations can safeguard sensitive queries and user information. Anonymizing and aggregating user data, when necessary, helps protect individual identities. It's crucial to implement data privacy measures compliant with industry and legal standards to ensure secure and confidential usage of ChatGPT in production engineering and other sensitive domains.
This article has opened my eyes to the potential of ChatGPT in production engineering. Are there any ongoing research initiatives related to further expanding its capabilities?
Absolutely, Sophie. Ongoing research initiatives are continuously improving ChatGPT and expanding its capabilities. Some areas of focus include enhancing its understanding of nuanced queries, addressing response inconsistencies, and providing better context-awareness. OpenAI explores ways to make ChatGPT more customizable, allowing users to define preferences and fine-tune the model according to their specific production engineering requirements. These research efforts aim to unlock the full potential of ChatGPT in the industry.
The potential applications of ChatGPT are fascinating. How does it handle queries that require a combination of text and visual data in a production engineering context?
Handling queries that require both text and visual data integration is an interesting challenge. While ChatGPT primarily focuses on understanding textual input, it can still assist by providing relevant textual information, explanations, or suggestions. For queries involving visual data, integration with computer vision models or specialized tools that process images and provide contextual insights can be a valuable addition alongside ChatGPT in a production engineering context.
I'm impressed by ChatGPT's potential for enhancing production engineering. How can engineers contribute to the training and improvement of the model?
Engineers can contribute significantly to the training and improvement of the model by providing feedback on responses, pointing out inaccuracies, suggesting clarifications, or highlighting any ambiguities. User feedback plays a vital role in identifying areas for improvement and helps in training the model to produce more accurate and context-aware responses. Collaborative engagement between engineers and the development team ensures ChatGPT reflects the needs and nuances of the production engineering domain.
This article has expanded my understanding of ChatGPT's applications in production engineering. How do you envision its integration with the broader field of industrial automation?
Integrating ChatGPT with the broader field of industrial automation holds tremendous potential. By combining the natural language processing capabilities of ChatGPT with automation technologies like robotics, machine vision, and data analytics, engineers can interact with automated systems more intuitively. ChatGPT can provide real-time insights, offer troubleshooting assistance, and serve as a bridge between engineers and automated systems in industrial automation, facilitating more efficient and informed decision-making.
I'm curious about the training process of ChatGPT. Could you shed some light on how it learns and acquires knowledge?
Certainly, Ella. ChatGPT is trained through a process called unsupervised learning. It leverages a vast amount of text data from the internet to learn language patterns, relationships, and how to generate coherent responses. The model is trained to predict the likelihood of words given their context in the training data. Through this training process, ChatGPT learns associations, semantics, and syntactic structures, granting it the ability to generate human-like responses in production engineering and other domains.
Considering the usefulness of ChatGPT in production engineering, are there any efforts to make it available in languages other than English?
Extending ChatGPT to languages other than English is indeed an ongoing effort. OpenAI recognizes the importance of multilingual support and is actively working towards it. By training and fine-tuning models on diverse language datasets, future versions of ChatGPT can cater to the language requirements of various production engineering contexts, enabling a more inclusive and comprehensive deployment of this technology worldwide.
This article presents an exciting perspective on the use of ChatGPT in production engineering. How does OpenAI address concerns about potential biases in the model's responses?
Addressing potential biases is a priority for OpenAI and ChatGPT. The model's training includes data from diverse sources to mitigate inherent biases. Conducting regular audits and analysis helps identify biases and improve the model's understanding across different demographics. OpenAI actively seeks external input and engagement to uncover and address biases in ChatGPT's responses, ensuring they are fair, unbiased, and reflective of varied production engineering perspectives.
The potential benefits of ChatGPT in production engineering are promising. How can we stay updated on the latest developments and enhancements related to ChatGPT?
To stay updated on the latest developments and enhancements related to ChatGPT, I recommend following OpenAI's official channels, including their website, blog, and social media accounts. OpenAI regularly shares insights, research papers, and updates about ChatGPT and similar technologies. Engaging with the broader research and AI community can also provide valuable insights into the future developments and ongoing work related to ChatGPT in production engineering.
Thank you all for reading my article on empowering production engineering with ChatGPT! I'm excited to hear your thoughts and answer any questions you may have.
Aldo, I'm curious if there are any potential limitations or challenges when implementing ChatGPT in production engineering? Are there any concerns about accuracy or reliability?
Daniel, that's a great question. While ChatGPT can be highly useful, it's crucial to ensure the accuracy and reliability of the information it provides. There might be instances where the model generates plausible but incorrect responses, so it's important to have checks and validation mechanisms in place. Regular updates and refinement of the model can also help address any limitations.
Thanks for your response, Aldo! It's important to have systems in place to handle potential inaccuracies. Continuous improvement and refinement will surely make ChatGPT an even more reliable tool!
I completely agree, Aldo! Continuous improvement and addressing potential limitations are key aspects to ensure the reliability and usefulness of AI models like ChatGPT.
Also, I'm interested to know, Aldo, how large-scale adoption of ChatGPT in production engineering can impact job roles and skill requirements?
Daniel, the adoption of ChatGPT can augment and enhance job roles rather than replace them. It can empower engineers by automating repetitive tasks, freeing up time for more creative problem-solving and strategic decision-making. Skill requirements may evolve to include a foundational understanding of AI technologies, natural language processing, and effective collaboration with AI systems.
Thank you for your insightful response, Aldo! It's reassuring to know that AI integration can lead to skill enhancement for engineers rather than job displacement.
Great article, Aldo! I found the concept of leveraging natural language processing in production engineering fascinating. It seems like ChatGPT could significantly streamline communication and improve efficiency in this field.
Robert, do you think implementing ChatGPT could potentially replace human interaction in production engineering?
Michael, while ChatGPT can automate certain tasks and improve communication, human interaction will still be essential in production engineering. ChatGPT can support engineers, facilitate problem-solving, and provide quick access to information, but human expertise is invaluable in decision-making and complex problem-solving scenarios.
Robert, thanks for clarifying. I agree that while AI can streamline processes, human expertise will always be critical. Balance is key!
Exactly, Robert! Balance and collaboration between AI and human expertise will drive the future of production engineering.
Well said, Michael! The combination of AI and human skills can lead to incredible advancements and problem-solving abilities in production engineering.
I agree, Robert! It's interesting to see how AI can be applied to make complex processes like production engineering more accessible and efficient for everyone involved.
Emily, do you think ChatGPT can also be applied in other fields beyond production engineering?
Absolutely, Oliver! Natural language processing and AI models like ChatGPT have the potential to revolutionize various domains, ranging from customer service and healthcare to education and content creation. It's exciting to imagine the possibilities!
Emily, I couldn't agree more. The potential applications of ChatGPT in various industries and sectors are limitless. Exciting times ahead!
Absolutely, Emily! The future is AI-assisted, and it's fascinating to think about the positive impact it can have across various industries.
Oliver, I couldn't agree more. AI-assisted workflows have the potential to drive innovation, efficiency, and create new opportunities for growth.
Absolutely, Emily! AI-assisted workflows can unleash human potential and pave the way for new discoveries and innovations.
Sarah, I couldn't have said it better! The synergy between human expertise and AI capabilities holds immense promise for the future.
Emily, indeed! The future will rely on symbiotic relationships between humans and AI systems, enabling innovation and enriching our lives.
I loved reading this article, Aldo! I can see how ChatGPT can greatly enhance collaboration between different teams in production engineering. The ability to access knowledge quickly and easily in natural language is truly empowering!
I completely agree with you, Sophia! ChatGPT seems like a game-changer for collaboration between different teams, enabling smoother communication and knowledge sharing.
Absolutely, Sophia! The democratization of knowledge and information exchange among teams can lead to significant progress and innovation in production engineering.
Grace, I couldn't agree more. ChatGPT has the potential to bridge the knowledge gap across teams, increasing efficiency and facilitating better decision-making.
Grace, democratization of knowledge through tools like ChatGPT fosters a culture of shared learning and interdisciplinary collaboration, leading to better outcomes.
Great article, Aldo! I'm interested to know, have any companies already started implementing ChatGPT in their production engineering workflows?
Thank you, Sophie! Yes, several companies have started exploring the integration of ChatGPT in their production engineering workflows. While it's still in its early stages, initial results and feedback have been positive. It will be fascinating to witness the widespread adoption and further advancements!
That's great to hear, Aldo! Exciting times indeed, as more companies embrace the possibilities of AI in their workflows.
Aldo, are there any potential ethical concerns surrounding the use of ChatGPT in production engineering? How can these challenges be addressed?
Paul, ethical concerns are definitely important to consider. The use of AI models like ChatGPT must be guided by principles of fairness, transparency, and accountability. It's vital to have clear guidelines and mechanisms in place to prevent biases, protect user privacy, and ensure responsible deployment. Ongoing research and collaboration across interdisciplinary fields can help address these challenges.
Thank you, Aldo. It's reassuring to know that ethical considerations are being actively addressed in the implementation of AI technologies.
Indeed, ethical considerations are crucial in any AI implementation. It's encouraging to see industry experts actively working towards responsible AI practices.
Definitely, Paul! As with any transformative technology, it's essential to navigate the ethical landscape to harness its benefits responsibly.
Working hand in hand, AI and human professionals can create a powerful partnership to drive advancement and efficiency in production engineering.
Exactly, Michael! It's about leveraging the strengths of both AI and human skills to achieve the best outcomes.
AI-assisted industries can unlock immense potential for growth, productivity, and even new job opportunities as our world becomes increasingly digital.
Oliver, absolutely! With the right implementation and utilization, AI can be a driving force behind positive economic and societal changes.