Enhanced Adaptive Routing in Optical Communications: Leveraging the Power of ChatGPT
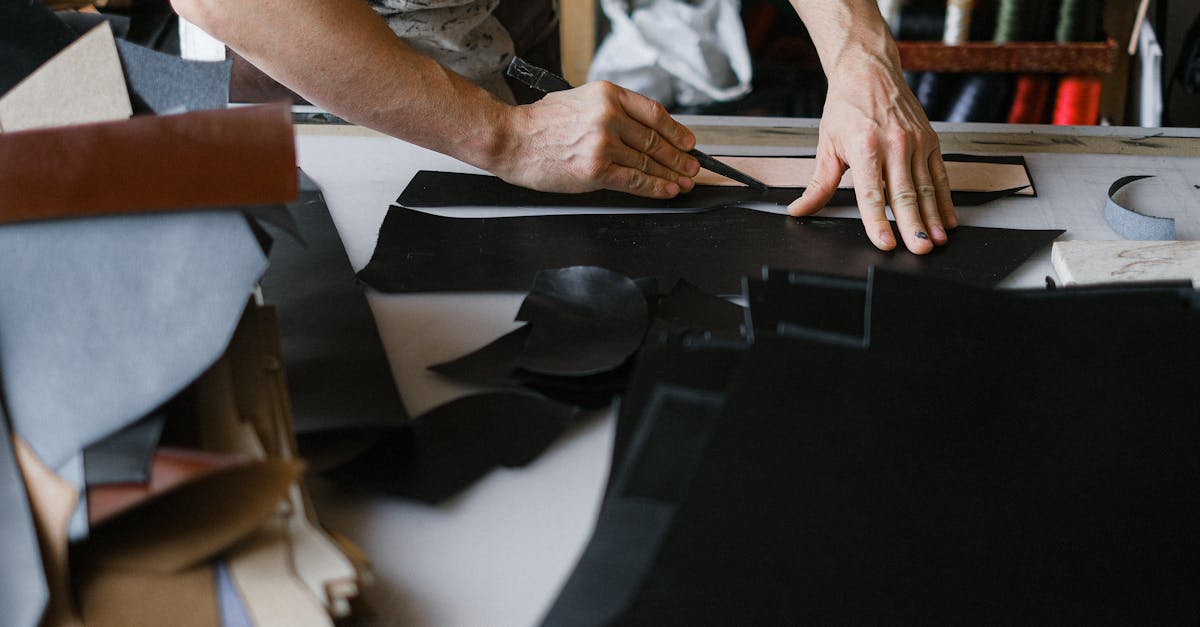
Optical communications play a crucial role in the fast and reliable transmission of data over long distances. With the ever-increasing demand for bandwidth, the need for efficient and effective routing of traffic in optical networks has become essential. To address this challenge, artificial intelligence (AI) can be employed to enable adaptive routing, allowing networks to dynamically respond to changing network conditions.
Adaptive routing refers to the ability of a network to dynamically select the most optimal path for data transmission based on current network conditions. In optical communications, this involves finding the best route through a network of interconnected nodes and fiber-optic links. Traditional routing algorithms often rely on predefined static routes, which may not always be the most efficient or reliable option.
AI algorithms can leverage real-time data and network analytics to make intelligent decisions about routing paths in optical networks. By continuously monitoring network performance metrics, such as latency, congestion, and available bandwidth, AI can determine the optimal route for data transmission. By adapting routing decisions in real-time, AI can optimize network efficiency, minimize delays, and enhance overall network performance.
One way AI can help in adaptive routing is through machine learning algorithms. These algorithms can analyze historical network data to identify patterns and trends, and use them to predict future network behavior. By understanding how network conditions have changed in the past, AI algorithms can make informed decisions about routing paths, even in unforeseen circumstances.
Another approach to adaptive routing in optical communications is the use of reinforcement learning. Reinforcement learning algorithms interact with the network environment, continuously learning from their actions and adjusting their behavior based on feedback. By rewarding or penalizing certain routing decisions, reinforcement learning algorithms can learn to choose the most effective routes in different network conditions.
AI-powered adaptive routing can have several benefits for optical networks. Firstly, it can optimize network resource utilization, ensuring that data traffic is distributed efficiently across the network. This can help prevent congestion and bottlenecks, improving overall network performance. Secondly, adaptive routing can enhance network resilience by dynamically rerouting traffic in the event of link failures or congestion. By quickly adapting to changing conditions, the network can maintain uninterrupted data transmission.
Furthermore, AI can enable proactive network management by predicting and pre-emptively addressing potential issues. By analyzing network data and identifying patterns, AI algorithms can anticipate future network congestion or failures, allowing for preventive measures to be taken. This proactive approach can significantly reduce network downtime and improve user experience.
In conclusion, AI plays a vital role in the adaptive routing of traffic in optical networks. By leveraging machine learning and reinforcement learning algorithms, AI can dynamically select the most efficient and reliable routes based on current network conditions. This helps optimize network performance, enhance resilience, and enable proactive network management. As the demand for high-speed data transmission continues to grow, AI-powered adaptive routing will play an increasingly important role in ensuring the efficiency and reliability of optical communications.
Comments:
Great article, Mark! I find the concept of leveraging ChatGPT for enhanced adaptive routing fascinating. It has the potential to greatly improve the efficiency and performance of optical communications.
I completely agree, Samantha! The ability to adaptively route optical communications can lead to faster and more reliable data transmission. Exciting times ahead!
I wonder if leveraging ChatGPT introduces any security concerns in optical communications. Mark, could you shed some light on that?
Hi Andrew! That's a great question. While leveraging ChatGPT for enhanced adaptive routing brings its benefits, it's crucial to address security concerns. Proper authentication and encryption mechanisms can be implemented to ensure the security of the communication channels.
Thank you for addressing the security aspect, Mark. It's indeed important to prioritize data safety while exploring new technologies.
The use of AI models like ChatGPT in optical communications sounds promising, but I'm curious about the computational requirements. Would implementing this approach be resource-intensive?
Interesting point, Benjamin! Mark, could you share some insights on the computational aspects involved in leveraging ChatGPT for enhanced adaptive routing?
Hi Benjamin and Jessica! Integrating ChatGPT into the optical communications infrastructure does introduce additional computational requirements. However, advancements in hardware acceleration and distributed computing can help mitigate the impact. Optimal system design can ensure efficient utilization of resources while leveraging the power of ChatGPT.
I'm excited about the potential impact of enhanced adaptive routing in optical communications. It can improve the overall network performance and enable more seamless data transmission across different devices and platforms.
Absolutely, Olivia! The ability to dynamically adapt the routing in optical communications can optimize network utilization and provide better end-to-end service quality.
I wonder how the integration of ChatGPT into optical communications could impact latency. Mark, do you think there might be any latency concerns with this approach?
Hi Sophia! Integrating ChatGPT into the routing decisions requires some computational time, which can introduce a slight increase in latency. However, with efficient system design and optimization, the overall impact on latency can be minimized to ensure smooth and timely data transmission.
Thanks for the clarification, Mark! It's evident that thoughtful design and optimization are crucial in implementing routing decisions powered by ChatGPT.
I'm curious about the compatibility of enhanced adaptive routing with existing optical communication infrastructure. Are there any specific requirements for implementing this approach?
Good question, Jacob! Mark, could you elaborate on the compatibility aspect and any necessary requirements for adopting enhanced adaptive routing?
Jacob and Ella, excellent questions! The compatibility of enhanced adaptive routing with existing infrastructure depends on factors like protocol support and hardware capabilities. While some adjustments may be needed, implementation can be facilitated by utilizing software-defined networking (SDN) techniques and collaborating with industry partners.
Thank you for addressing the latency concern, Mark. It's crucial to strike a balance between enhanced routing capabilities and maintaining acceptable latency levels for real-time applications.
I agree, Natalie. Achieving low latency is critical, especially in applications like video streaming and cloud gaming that are sensitive to delays. It's encouraging to see efforts to optimize the overall performance.
Faster and more reliable data transmission is always a positive outcome. Adaptive routing has the potential to revolutionize optical communications and enable a more interconnected world.
Indeed, Liam. The world is becoming increasingly connected, and technologies like adaptive routing will play a vital role in ensuring seamless communication across various networks and devices.
Data safety should always be a priority. I'm glad to see that security concerns are being addressed to maintain the integrity and confidentiality of optical communications.
Optimizing resource utilization is essential for efficient network operation. Advancements in hardware and computing techniques are key to realizing the full potential of leveraging ChatGPT in optical communications.
Absolutely, Oliver! Properly managing the computational requirements plays a crucial role in ensuring scalability and cost-effectiveness when implementing enhanced adaptive routing.
I can imagine how seamless data transmission would enhance user experiences, especially when it comes to services like video conferencing and online gaming.
Exactly, Harper! Reduced latency and improved network performance can make a noticeable difference in delivering real-time applications without disruptions.
Minimizing latency should be a priority, but it also depends on the complexity of routing decisions made using ChatGPT. Balancing speed and accuracy will be crucial.
You're absolutely right, Connor. Striking the right balance between speed and accuracy in routing decisions is a key challenge. Continuous optimization and improvement can help to achieve the desired balance while minimizing latency.
I wonder if adopting enhanced adaptive routing would require a complete overhaul of existing networking infrastructure, or if it can be gradually integrated.
That's a valid concern, Blake. Mark, could you elaborate on the feasibility of integrating enhanced adaptive routing into existing infrastructure without major disruptions?
Good question, Blake and Ella! A complete overhaul of the infrastructure may not be necessary. By leveraging techniques like SDN and conducting thorough testing and gradual integration, enhanced adaptive routing can be implemented without major disruptions to existing networks.
Interconnectedness brings its own challenges, but adaptive routing can help overcome them. The future of optical communications looks promising!
Indeed, Daniel! Embracing technologies that enable more efficient and reliable communication is key to building a robust and interconnected digital ecosystem.
The collaboration with industry partners is crucial when introducing new routing technologies. It ensures compatibility and promotes seamless adoption by leveraging shared expertise.
Absolutely, Sophie! Collaboration among industry partners is vital in driving technology adoption and fostering an ecosystem that supports enhanced adaptive routing solutions.
I'm curious if there are any known limitations of using adaptive routing with ChatGPT. Mark, could you highlight any challenges or potential drawbacks?
Good point, Gabriel! Identifying potential limitations and challenges will help us prepare adequately for the implementation of enhanced adaptive routing.
Certainly, Gabriel and Jacob! Some challenges include ensuring the model's accuracy in diverse network conditions, potential biases in decision-making, and the need for continuous model updates. Rigorous testing and close monitoring can help address these challenges throughout the implementation process.
Collaboration is key in driving innovation and ensuring smooth technology integration. It's great to see industry partners working together towards implementing enhanced adaptive routing solutions.
Indeed, Lucy! A collaborative approach encourages knowledge sharing, facilitates standardization, and promotes the development of holistic solutions that cater to diverse network environments.
It's exciting to witness the progress being made in the field of optical communications. Collaborative efforts and advancements in AI technologies like ChatGPT are shaping the future!