Enhanced Polymer Aging Studies: Leveraging ChatGPT for Advanced Polymer Characterization
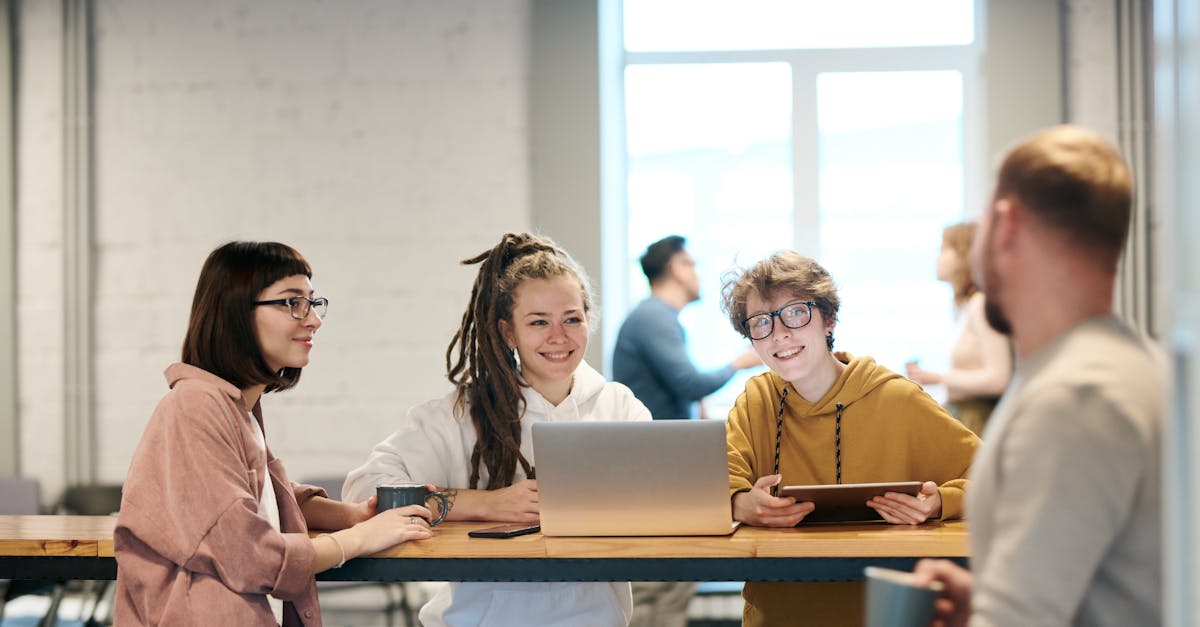
Polymer aging is an essential field in material science that focuses on understanding how polymers change and degrade over time. Polymer aging studies play a crucial role in various industries, including automotive, aerospace, and packaging. By analyzing and interpreting the aging process of polymers under different conditions, researchers can develop strategies to improve their durability and longevity.
What is Polymer Characterization?
Polymer characterization refers to the process of analyzing the physical, chemical, and mechanical properties of polymers. It involves studying their molecular structure, thermal behavior, mechanical strength, and other key characteristics. Polymer characterization techniques provide valuable insights into the properties and performance of polymers, aiding in understanding their aging process.
Importance of Polymer Aging Studies
Polymers are widely used in various applications due to their desirable properties, such as flexibility, lightweight, and durability. However, over time, polymers can experience degradation, leading to reduced mechanical properties and structural integrity. Polymer aging studies help researchers identify the underlying mechanisms responsible for this degradation and develop effective strategies to mitigate it.
Polymer aging can occur due to various factors, including exposure to environmental conditions, such as heat, light, and chemicals, as well as mechanical stresses and oxidation. By subjecting polymers to accelerated aging tests and analyzing the changes in their properties, researchers can simulate long-term aging effects and predict the lifespan of polymers in different applications.
Understanding the Aging Process
Polymer characterization techniques provide valuable information on the aging process by analyzing the changes in the polymer's molecular structure, thermal properties, and mechanical behavior. By studying the polymer's molecular weight distribution, researchers can determine the extent of chain scission, cross-linking, and degradation that occurs during aging.
Thermal analysis techniques, such as differential scanning calorimetry (DSC) and thermogravimetric analysis (TGA), help measure the changes in the polymer's thermal stability and decomposition temperature. This information is crucial in identifying the temperature ranges at which polymer degradation accelerates.
Mechanical testing, such as tensile and impact testing, allows researchers to evaluate the changes in the polymer's mechanical properties, such as tensile strength, elongation at break, and impact resistance. These tests help identify the loss of mechanical strength and toughness that occur during aging, enabling the prediction of the polymer's performance under different loading conditions.
Application of Polymer Characterization in Aging Studies
Polymer characterization techniques are widely used in aging studies to evaluate the effects of different aging factors and develop strategies to mitigate polymer degradation. For example, researchers can analyze the changes in the polymer's surface morphology using scanning electron microscopy (SEM) to determine the formation of cracks, voids, and other surface defects during aging. This information helps identify potential failure modes and develop strategies to enhance durability.
Furthermore, researchers can utilize spectroscopic techniques such as Fourier-transform infrared spectroscopy (FTIR) and nuclear magnetic resonance (NMR) to study the chemical changes in the polymer structure during aging. These techniques provide insights into the formation of new functional groups and chemical bonds, which can lead to polymer degradation or stabilization.
Conclusion
Polymer characterization plays a crucial role in understanding the aging process of polymers. By analyzing and interpreting the changes in molecular structure, thermal properties, and mechanical behavior, researchers can gain valuable insights into polymer degradation mechanisms. This knowledge can be used to develop strategies to enhance the durability and longevity of polymers in various applications, ultimately improving the overall performance and sustainability of polymer-based products.
Comments:
Thank you all for taking the time to read and comment on my article!
This article is very informative. The potential applications of ChatGPT in advanced polymer characterization seem promising.
Michael, do you think ChatGPT can be applied to studying other types of polymer aging besides degradation?
Emily, definitely! While degradation is a significant aspect, ChatGPT can also be used to study aging phenomena like cross-linking, crystallization, and changes in mechanical properties over time. It offers a versatile tool to examine various aspects of polymer aging.
I agree, Michael. The ability to leverage ChatGPT for enhanced polymer aging studies opens up new possibilities for materials science research.
Interesting article! I would like to know more about the specific techniques used to study polymer aging and how ChatGPT fits into the process.
Thomas, great question! In the article, I discussed various accelerated aging techniques used to study polymer degradation. ChatGPT is employed as a tool to analyze and interpret the vast amounts of data generated by these techniques.
Jesse, could we expect AI systems like ChatGPT to replace human experts in polymer aging studies in the future?
Thomas, while AI systems like ChatGPT offer valuable analytical capabilities, they are not intended to replace human experts. The scientific knowledge, creativity, and critical thinking abilities of human experts are vital in interpreting complex results, formulating new research questions, and ensuring the relevance of AI insights to real-world applications. AI should be viewed as a supportive tool in the hands of experts rather than a replacement.
Jesse, are there any ongoing projects or initiatives exploring the integration of AI in polymer aging studies?
Thomas, several projects and initiatives are underway. Collaborative efforts between materials research institutions, AI companies, and government agencies are exploring the potential of AI for polymer aging studies. These initiatives focus on developing new AI models, creating standardized datasets, and establishing guidelines for the responsible use of AI in the field.
Jesse, what are some potential collaborations between academia, industry, and government that can further advance AI in materials research?
Thomas, collaborations across academia, industry, and government are vital for advancing AI in materials research. Joint research projects, technology transfer programs, and funding initiatives can facilitate knowledge exchange and promote the translation of AI research into real-world applications. Partnerships can also ensure that AI systems address industry challenges while considering ethical and regulatory aspects, leading to responsible and impactful integration of AI in materials research.
It's fascinating to see how artificial intelligence is being integrated into polymer aging studies. Jesse, could you provide some examples of the specific insights ChatGPT can offer?
Certainly, Alexandra! ChatGPT can help identify patterns, trends, and correlations in polymer aging data. It can provide insights into the effects of various factors such as temperature, humidity, and material composition on the degradation process. Additionally, it can help in predicting the lifespan and performance of polymers under different conditions.
Alexandra, ChatGPT can also assist in identifying unknown degradation mechanisms and degradation products, aiding in the development of improved polymer formulations and stability evaluation.
The potential of ChatGPT in polymer aging studies is clear, but what are some limitations or challenges associated with its usage in this context?
Good point, Daniel. One challenge is the need for reliable and accurate data input to obtain meaningful insights. The quality and representativeness of the data used to train ChatGPT are crucial. Moreover, ChatGPT might not capture all the nuances of complex polymer chemistry and may struggle with rare or novel materials. Combining AI with expert knowledge can address some of these limitations.
Jesse, you mentioned combining AI with expert knowledge to address limitations. How can this collaboration be effectively implemented in polymer aging studies?
Daniel, effective collaboration can involve developing interdisciplinary research teams with materials scientists, chemists, and AI experts. Regular interactions and knowledge sharing between domain experts and AI researchers help in building mutual understanding. This collaboration should occur throughout the research process, from experimental design to data analysis and interpretation.
Jesse, how can we foster more collaborations between materials science researchers and AI experts?
Daniel, fostering collaborations requires building bridges between the materials science and AI research communities. Conferences, workshops, and funding opportunities that encourage interdisciplinary research can facilitate these collaborations. Establishing joint research projects, forming research consortia, and providing training programs that promote knowledge exchange across disciplines are also effective approaches.
The ethical implications of AI in materials research are worth discussing. How do we ensure that biases or unintended consequences don't influence the outcomes?
Sophia, that's a crucial aspect. Ensuring data integrity, transparency, and diversity in training datasets is vital to mitigate biases. Regular monitoring, validation, and involving domain experts in the AI systems' development can help reduce unintended consequences. Open dialogue within the scientific community is also essential to address these concerns.
Jesse, how do you see the collaboration between AI systems like ChatGPT and domain experts evolving in the future?
Sophia, collaboration between AI systems and domain experts will likely become more synergistic. While AI can assist in data analysis, pattern recognition, and prediction, domain experts' knowledge and intuition are crucial for interpreting the results, ensuring relevance to real-world problems, and making informed decisions based on AI insights.
Jesse, how can the scientific community stay updated on the latest advancements and best practices in AI for materials research?
Sophia, staying updated requires active participation in scientific conferences, workshops, and seminars that focus on the intersection of AI and materials research. Subscribing to relevant journals, joining online communities, and following AI and materials science experts on platforms like social media and research gateways can also provide valuable insights and opportunities for knowledge exchange.
Sophia, apart from scientific collaboration, public engagement and interdisciplinary dialogue can also help address ethical concerns and ensure AI systems are developed and deployed responsibly.
I find the potential of ChatGPT in polymer aging studies intriguing, but since it's an AI system, how do we know if the outputs and predictions are trustworthy?
Validating the outputs of AI systems is crucial, Rachel. A combination of experimental validation and testing against known polymer behavior can help establish trust. The outputs should be analyzed critically, and confidence intervals or uncertainty estimations can be provided to aid in assessing trustworthiness.
Jesse, what are some key research challenges that need to be addressed to further leverage AI in polymer aging studies?
Rachel, there are several research challenges. Developing AI models that can handle the complexity of polymer chemistry, including intermolecular interactions, chain dynamics, and long-term aging predictions, is one. Creating more publicly available and well-curated polymer aging datasets for training and validation is another. Exploring explainable AI methods and addressing ethical concerns related to AI deployment are also important areas of research.
Jesse, what steps are being taken to ensure responsible and equitable access to AI tools like ChatGPT?
Rachel, responsible and equitable access is a critical consideration. Steps are being taken to provide open-source AI tools, promote public-private collaborations, and ensure affordability and accessibility to researchers worldwide. Efforts are also underway to address biases in training data and improve the transparency and interpretability of AI systems, making them more accountable and fair.
Jesse, how can ChatGPT help researchers in making decisions related to choosing suitable polymers for specific applications?
Rachel, ChatGPT can assist researchers in identifying polymer characteristics relevant to specific applications and predicting how different polymers will perform under specific conditions. It can analyze and compare large amounts of data, helping researchers make informed decisions by considering factors like mechanical properties, stability, and environmental impact. ChatGPT can act as a valuable tool in the polymer selection process.
I'm curious about the computational resources required to deploy ChatGPT for polymer aging studies. Are they readily available in typical materials research labs?
Emily, that's a valid concern. The computational resources required for deploying ChatGPT can be significant, especially for large-scale polymer aging studies. However, with advancements in cloud computing and availability of powerful GPUs, these resources are becoming more accessible to materials research labs. Collaboration with AI experts can also help overcome resource limitations.
Jesse, are there any potential risks associated with the adoption of AI systems like ChatGPT in the materials research domain?
Emily, there are risks to be mindful of. One is relying solely on AI-generated insights without critically evaluating them, which can lead to erroneous conclusions. Another is the potential for biased or skewed results if the training data is not diverse or representative. Incorporating transparency, interpretability, and human-in-the-loop approaches can help mitigate such risks.
Jesse, AI seems to have wide-ranging applications. Can ChatGPT be used beyond polymer aging studies?
Laura, absolutely! ChatGPT can be adapted and applied to various fields beyond polymer aging. It can assist in data analysis, decision-making, and providing insights in fields such as drug discovery, materials design, climate modeling, and more. The versatility of AI systems like ChatGPT makes them valuable assets across different scientific domains.
Jesse, do you foresee the development of AI systems designed specifically for polymer aging studies, or will general-purpose AI systems like ChatGPT continue to be adapted for this purpose?
Emily, it's possible to have AI systems specifically designed for polymer aging studies. These systems can incorporate domain-specific knowledge and models tailored to the unique characteristics of polymers. However, general-purpose AI systems like ChatGPT can also continue to play a significant role by offering a versatile platform that can be adapted and fine-tuned for specific applications, including polymer aging studies.
The integration of AI in polymer science is exciting. How do you envision the future of this technology in the field of materials research?
Great question, Benjamin. The future of AI in materials research holds immense potential. AI can aid in accelerating materials discovery, optimizing material properties, developing sustainable alternatives, and enabling predictive maintenance. It can revolutionize how materials are designed, characterized, and utilized across various industries.
Jesse, how can AI contribute to the development of more sustainable and eco-friendly polymer materials?
Benjamin, AI can aid in materials discovery and optimization, facilitating the development of sustainable alternatives to traditional polymers. By analyzing large datasets, AI can guide the design of materials with desirable properties, enhanced biodegradability, reduced environmental impact, and improved recyclability. It can also assist in the identification of potential toxicants and help design safer materials.
Jesse, what other AI technologies or advancements do you foresee augmenting materials research in the future?
Benjamin, apart from ChatGPT, other AI technologies like deep learning, reinforcement learning, and generative adversarial networks (GANs) hold promise in materials research. These technologies can assist in material property prediction, accelerated materials discovery, and generating novel materials with desired properties. Combining AI with advanced experimental techniques like high-throughput screening can unlock new frontiers in materials science.