Enhancing AML Technology with Gemini: Improving Efficiency and Accuracy in Anti-Money Laundering
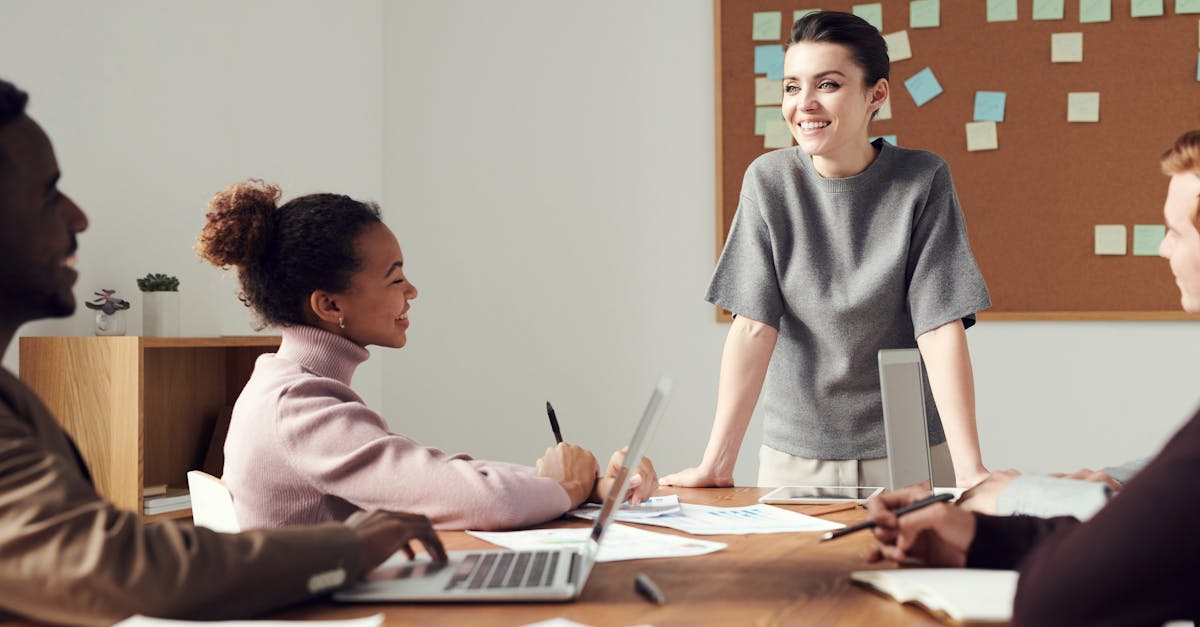
Money laundering has long been a major concern for financial institutions and regulatory bodies worldwide. Criminals constantly evolve their strategies to exploit vulnerabilities in anti-money laundering (AML) systems, making it increasingly challenging for organizations to detect suspicious activities and prevent illicit transactions. However, recent advances in natural language processing (NLP) and machine learning have opened up new possibilities for AML technology.
The Power of Gemini
One such breakthrough is Gemini, an advanced language model developed by Google. Gemini is based on the Transformer architecture and is trained on a vast corpus of text from the internet, enabling it to generate coherent and contextually relevant responses to textual input. While originally designed for chatbot applications, Gemini's capabilities have proved to be far-reaching and adaptable.
By integrating Gemini into AML technology, financial institutions can leverage its language processing prowess to enhance the effectiveness of their systems. Gemini can analyze textual data from various sources, such as customer communications, transaction records, public forums, and news articles, to identify potential patterns indicative of illegal activities.
Improving Efficiency in AML Processes
AML processes often involve the manual review of large volumes of data, which is a time-consuming and resource-intensive task. However, with the integration of Gemini, the workload can be significantly reduced. Gemini can automatically scan and analyze textual data, flagging suspicious transactions or communications for further investigation.
Furthermore, Gemini's ability to generate coherent responses eliminates the need for human operators to construct individual replies, saving valuable time and effort. This improved efficiency allows AML teams to focus on higher-level tasks, such as interpreting complex data patterns and developing more robust risk mitigation strategies.
Enhancing Accuracy in AML Systems
The accuracy of AML systems is crucial in detecting and preventing money laundering. Traditional rule-based systems often struggle to adapt to evolving tactics employed by criminals. In contrast, Gemini's machine learning capabilities enable it to learn from new data and improve over time.
By continuously feeding Gemini with up-to-date information on emerging money laundering techniques and patterns, organizations can enhance the accuracy of their AML systems. Machine learning algorithms help identify subtle anomalies that may have eluded rule-based systems, facilitating the detection of suspicious activities and minimizing false positives.
Considerations and Challenges
While the potential benefits of integrating Gemini into AML technology are significant, there are several considerations and challenges to be mindful of:
- Data Privacy: Financial institutions must ensure that sensitive customer data is handled securely and in compliance with relevant data privacy regulations.
- Model Bias: It is crucial to train Gemini on diverse datasets to avoid bias and ensure that the system provides fair and objective outcomes.
- Human Oversight: While Gemini can automate various aspects of AML processes, human oversight remains essential to ensure the accuracy and ethical use of the technology.
Conclusion
The integration of Gemini into AML technology holds the potential to revolutionize the fight against money laundering. By harnessing the power of natural language processing and machine learning, financial institutions can enhance their efficiency and accuracy in detecting suspicious activities. However, careful consideration and human oversight are necessary to address privacy concerns, model bias, and ethical implications. With continued advancements in NLP and machine learning, the future of AML technology looks promising.
Comments:
Thank you all for your comments! I appreciate your feedback on the article.
Gemini seems like a promising technology for improving AML efforts. I'm curious about its implementation in real-world scenarios.
I agree, Michael. Do you think Gemini can handle the complexity and volume of AML data effectively?
Good point, Jennifer. I believe the scalability of Gemini will be crucial for its success in AML applications.
Scalability is definitely important, but what about the accuracy of Gemini? Can it detect sophisticated money laundering techniques?
That's a valid concern, David. AML technology must be highly accurate to identify subtle patterns and anomalies in transactions.
Exactly, Samantha. I wonder how well Gemini performs compared to traditional AML systems?
In terms of accuracy, Gemini could potentially outperform traditional systems by leveraging its deep learning capabilities.
Jennifer makes a good point. Deep learning can enable Gemini to identify complex patterns that might be missed by rule-based systems.
True, Michael. However, explainability is also crucial in AML. Can Gemini provide clear insights into its decision-making process?
I share your concern, David. Transparency is vital to ensure AML decisions can be audited and understood.
While deep learning models are often considered black boxes, there are techniques to improve interpretability. Perhaps Gemini can adopt such methods.
I'm excited about the potential of Gemini in AML. It could revolutionize suspicious activity monitoring and save significant time.
Emily, I agree. Automated solutions like Gemini have the potential to reduce manual efforts and focus on higher-value tasks.
I've been working in AML for years, and I'm cautious about relying solely on AI. Human expertise is invaluable in detecting complex money laundering schemes.
You're right, Anne. It's crucial to strike a balance between AI and human intelligence in AML processes.
I completely agree, Anne. AI should augment human efforts rather than replace them.
Absolutely, Jennifer. The combination of human expertise and AI technology like Gemini can lead to more efficient and accurate AML operations.
Regarding Gemini's handling of complexity and volume, utilizing advanced machine learning techniques could help it adapt and analyze vast amounts of data.
That's a great point, Robert. Continuous learning and adaptation are vital to keep up with evolving money laundering tactics.
However, Samantha, can Gemini keep up with the pace of evolving AML regulations and compliance requirements?
Anne, that's a valid concern. AML technology must constantly adapt to changing regulations to remain effective.
The potential of AI in AML is enormous. I hope Gemini can minimize false positives and reduce the burden on analysts.
Indeed, Daniel. False positives can be a significant problem, leading to wasted resources and potentially overlooking real threats.
AI systems like Gemini have the advantage of continuous learning, which can help reduce false positives over time as they become more finely tuned.
I believe Gemini can leverage its adaptability to stay on top of regulatory changes, while also learning from analysts' inputs.
One potential challenge is the ethical use of AI in AML. How can we ensure it avoids biases and protects sensitive customer data?
You're right, Emily. To build trust in AI-powered AML, organizations need to establish robust ethical frameworks and data privacy measures.
Emily, you mentioned saving time. However, human verification and oversight are crucial before taking any legal actions based on AI-generated alerts.
That's a valid concern, Anne. AI should serve as a powerful tool that enhances analysts' capabilities rather than making autonomous decisions.
Absolutely, Emily. Human judgment should always be the final decision-maker in AML operations.
Anne, you're right. AML systems must provide decision support to analysts rather than fully automated solutions.
Anne, I completely agree. Human oversight is essential to prevent false accusations and ensure fairness in investigations.
Well said, Robert. Collaborative intelligence, combining AI capabilities with human judgment, is the way forward.
Transparency and accountable governance are essential to ensure AI systems like Gemini are unbiased and respect privacy regulations.
Absolutely, Anne. Adhering to ethical guidelines and conducting regular audits can help mitigate potential biases and enhance data protection.
Robert, you mentioned continuous learning. Could Gemini leverage data from various sources and public databases to enhance its accuracy?
That's a great question, David. Combining public data with internal sources could provide richer context for AML analysis.
Agreed, Jennifer. A comprehensive data integration strategy could strengthen Gemini's ability to detect suspicious activities.
I'm curious to know if Gemini has been deployed in any real-world financial institutions yet. Does anyone have information on that?
Jennifer, I recently read about a pilot project where Gemini was tested in a large bank's AML department. The initial results were promising.
That's interesting, Daniel. It would be great to see more case studies and real-world applications of Gemini in the AML domain.
Agreed, Michael. Real-world implementation will provide valuable insights into Gemini's effectiveness and potential limitations.
While AI in AML can assist with routine tasks, it's important not to underestimate the creative and adaptive abilities of human analysts.
You make a valid point, Emma. Human intuition and reasoning are still vital in understanding complex money laundering schemes.
Absolutely, Samantha. AI should complement human intelligence, not replace it, in the fight against financial crimes.
Finding the right balance between AI and human involvement is crucial. We shouldn't solely rely on either side.
I think the potential of AI in AML is remarkable, but responsible adoption is crucial to avoid unintended consequences.
Agreed, Daniel. Implementing AI in AML should involve thorough risk assessment and rigorous testing before widespread adoption.
Thank you all for the insightful discussion! It's great to see your perspectives and questions regarding Gemini and its application in AML.
I believe combining AI and human judgment will lead to more effective and efficient AML processes in the long run.
Indeed, Emma. The synergy between AI and human expertise holds the key to successfully combatting money laundering.
Emma, I couldn't agree more. Human analysts bring crucial qualities like intuition and contextual understanding to the AML landscape.
Thank you all once again. Your contributions to this discussion have been immensely valuable.
This article provides an interesting perspective on how Gemini can enhance AML technology. It seems like a promising approach to improving efficiency and accuracy in anti-money laundering.
I agree, John. The potential of using language models like Gemini in AML is exciting. It could help in identifying suspicious patterns and transactions more effectively.
While the idea of incorporating Gemini into AML technology sounds intriguing, I wonder about the potential risks. Could relying on AI models lead to false positives or false negatives?
Thanks for raising that concern, Adam. False positives and negatives are valid concerns in AML. However, the goal is to use Gemini as an additional tool to improve efficiency, not replace existing systems. It can assist human analysts in their decision-making process.
I think it's important to strike the right balance between human expertise and AI assistance. Gemini can provide valuable insights, but human oversight and judgment will still be essential to ensure accuracy.
As a compliance officer, I'm always looking for ways to enhance AML processes. Gemini seems promising, but it's crucial to thoroughly test and validate its effectiveness before widespread implementation.
I totally agree, Sarah. AML systems deal with sensitive matters, and any technological enhancement should go through rigorous testing and validation to ensure it meets regulatory requirements.
Exactly, Sarah and Jane. The implementation of any AI technology should be done cautiously, with proper monitoring and evaluation to mitigate potential risks.
I appreciate your cautious approach, Sarah and Jane. Thorough testing and validation are indeed crucial before any widespread deployment, especially in a domain as critical as AML.
Besides improving efficiency, I believe Gemini could also assist in analyzing unstructured data, such as text from customer communications, to identify potential AML risks.
That's an excellent point, John. Natural language processing capabilities of Gemini could prove invaluable in detecting suspicious language and uncovering hidden connections within textual data.
I have concerns about the potential biases that AI models like Gemini might inherit. It's crucial to ensure these models are trained on diverse and representative data to avoid discriminatory outcomes.
You're right, Robert. Bias mitigation should be a top priority while developing these models and incorporating them into AML systems. Ethical AI practices are essential to maintain fairness and avoid discrimination.
Valid concerns, Robert and Adam. Bias mitigation is an integral part of model development. Transparency and fairness in AI systems are crucial to ensure unbiased decision-making in AML processes.
I'm curious about the scalability aspect. AML systems deal with vast amounts of data. Can Gemini handle the volume and provide real-time insights, or are there limitations?
Scalability is indeed a significant consideration, Sarah. While Gemini has shown promising results in language understanding, it's essential to assess its performance and resource requirements for large-scale AML operations.
I believe Gemini can be used as a preliminary analysis tool to triage suspicious patterns and transactions, reducing the workload on human analysts. However, a well-designed infrastructure would be crucial for efficient utilization.
Considering the sensitive nature of AML, security is paramount. It's essential to have robust measures in place to protect against potential data breaches or unauthorized access to the Gemini system.
Absolutely, Adam. Security is of utmost importance in AML. The deployment of Gemini or any AI system must adhere to stringent security protocols to safeguard sensitive data and prevent any potential breaches.
It's great to see advancements in AML technology. I hope that organizations and regulators work together to address any challenges and ensure the effective and responsible implementation of AI in combating money laundering.
I share the same sentiment, Robert. Collaboration between industry professionals, regulators, and AI experts is crucial to harness the full potential of Gemini and other AI technologies for AML.
Ultimately, the success of AI in AML will heavily depend on continuous monitoring, evaluation, and iterative improvements. It's an evolving field, and we need to be adaptable in our approaches.
I agree, Jane. As the technology evolves, it's vital to keep regulatory frameworks updated, ensuring they account for the nuances of AI and provide clear guidelines for its responsible utilization in AML.
Adam, I agree with you on the importance of security. Robust security measures must be implemented to ensure the confidentiality and integrity of data in AML systems incorporating Gemini.
Thank you all for engaging in this informative discussion. Your points and concerns are valuable in shaping the future of AI in AML. Let's continue working towards enhancing efficiency and accuracy in the fight against money laundering.
Adiv, it was enlightening to hear your perspective as the author of this article. Your insights have helped us understand the potential of Gemini and the importance of human involvement in AML.
Sarah, scalability is a crucial aspect. While Gemini has made significant strides, it's essential to address resource requirements and optimize the system for efficient handling of large datasets.
I completely agree with you, John. AI should augment human analysis, not replace it. Combining the strengths of both humans and AI can lead to more accurate and efficient AML processes.
I appreciate your response, Adiv. Transparency and fairness should be at the core of any AI solution. A collaborative approach among experts, regulators, and organizations is needed to achieve these goals.
I fully agree, Adiv. Security protocols must be stringent to prevent any unauthorized access or misuse of sensitive AML data. Maintaining the trust of customers and stakeholders is paramount.
Thank you, Adiv, for initiating this discussion. It has provided valuable insights and raised important considerations for the effective implementation of AI in AML.
Collaboration is the key to success in implementing AI in AML. By working together, we can address challenges, share best practices, and ensure AI solutions align with regulatory requirements.
Continuous monitoring and improvement are essential to maximize the benefits of AI in AML. Organizations should actively invest in research and development to stay ahead of evolving money laundering techniques.
Jane, you make an excellent point. Gemini's ability to analyze unstructured data can be a game-changer in identifying potential money laundering activities hidden in customer communications.
I completely agree, Adam. Bias in AI systems can have serious repercussions, especially in AML. It's imperative to address and minimize biases during the development and implementation stages.
A well-designed infrastructure is crucial for the efficient utilization of Gemini in AML. It should streamline data processing, enable real-time analytics, and facilitate effective integration with existing systems.
Transparency and fairness should always be top priorities. Auditing and ongoing monitoring can help identify and rectify any biases or discriminatory outcomes that may arise from using Gemini.
Jane, continuous investment in research and development is key. Staying ahead of money laundering techniques requires the development and deployment of cutting-edge technology.
Collaboration between industry professionals, regulators, and AI experts is pivotal for responsible and effective AI adoption in AML. It can lead to the formulation of robust guidelines and frameworks.
Agreed, Adam. As AI technology evolves, regulatory frameworks should keep pace and adapt to the unique challenges and opportunities the technology presents in the fight against money laundering.
Adiv, I appreciate your involvement in this discussion. The collaboration between stakeholders is essential to navigate the complexities and ensure responsible AI adoption in AML.
Thank you, Adiv, for initiating this conversation and sharing your insights. These discussions are valuable in driving the responsible use of AI and technological advancements in AML.
Combining human expertise and AI capabilities is the way forward. It can improve accuracy and efficiency while also benefiting from the ability of AI models to analyze large volumes of data.
Monitoring biases and ensuring transparency should be an ongoing effort. A collaborative approach involving experts from multiple domains can help maintain fairness in AI systems.
Absolutely, Adam. The ability to analyze unstructured data can empower AML efforts by uncovering crucial information hidden within customer communications, enabling proactive risk mitigation.
An effectively designed infrastructure can ensure the seamless integration of Gemini into AML systems, facilitating real-time insights and enhancing the overall efficiency of the anti-money laundering process.
Addressing bias in AI systems is vital. Regular audits, diversity in training data, and ongoing monitoring can help identify and correct any potential biases, ensuring fair and reliable AML outcomes.
Investing in research and development will equip organizations with robust tools to combat evolving money laundering techniques effectively. Continuous adaptation is necessary to stay ahead.
A collaborative and multidisciplinary approach can help mitigate biases and ensure adopted AI systems align with ethical and regulatory requirements in the context of AML.
A well-designed infrastructure can be a game-changer, streamlining AML processes while leveraging the potential of Gemini to identify and mitigate money laundering risks effectively.