Enhancing Chromatography Technology through Machine Learning Integration: Leveraging the Power of ChatGPT
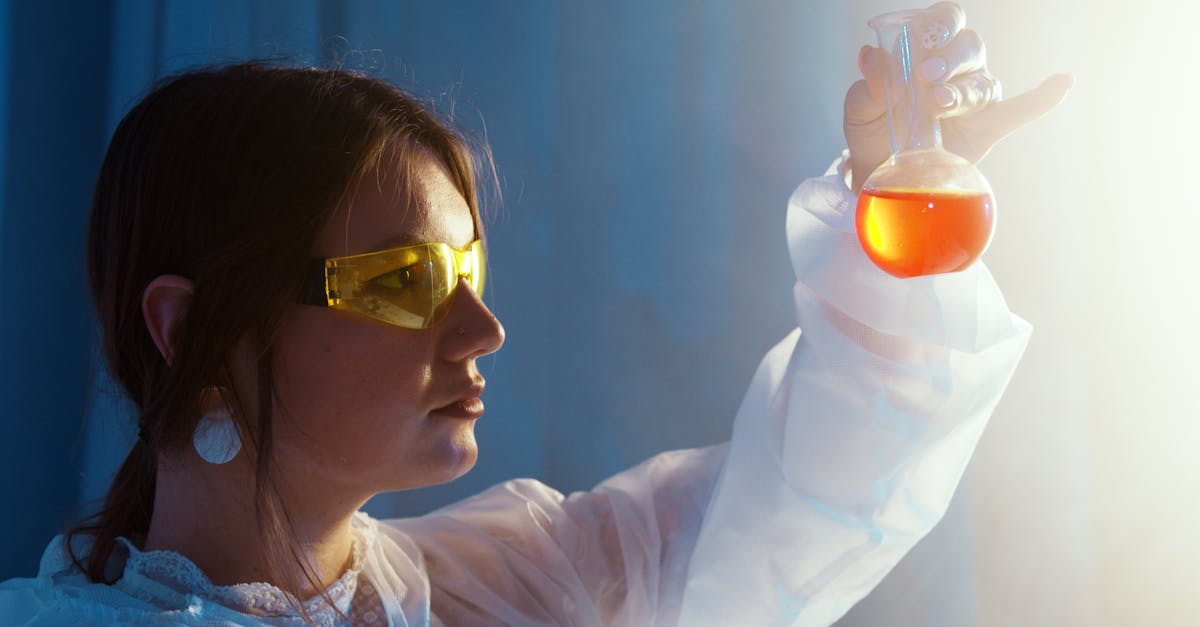
Chromatography and Machine Learning Integration
Chromatography is a widely used analytical technique to separate and identify different components within a mixture. It plays a crucial role in various industries such as pharmaceuticals, environmental analysis, food and beverage, forensics, and more. With the advancements in technology, there is a growing interest in integrating machine learning techniques into chromatography data analysis to enhance its capabilities and improve efficiency.
Understanding Chromatography
Chromatography involves the separation of analytes based on their physicochemical properties. It relies on the principle of differential migration of analytes through a stationary phase and a mobile phase. The stationary phase can be a solid or a liquid, while the mobile phase is typically a liquid or a gas.
During the separation process, analytes interact differently with the stationary and mobile phases, resulting in varied migration rates. This differential migration creates distinct peaks in the chromatograms, which can be analyzed to identify and quantify the components within the mixture.
The Role of Machine Learning
Machine learning involves the development of algorithms that can automatically learn patterns and make predictions or decisions without being explicitly programmed. By integrating machine learning techniques into chromatography data analysis, it is possible to improve the accuracy and efficiency of the analytical process.
One area where machine learning can be particularly beneficial in chromatography is in the identification and classification of chromatographic peaks. Traditionally, analysts manually identify and quantify peaks based on predefined criteria and expertise. However, this process can be time-consuming and subjective.
With the integration of machine learning, algorithms can be trained to recognize patterns in chromatographic data and automatically identify peaks with high accuracy. This can reduce the burden on analysts and improve the consistency of peak identification across different samples. Additionally, machine learning models can continuously learn and adapt, leading to improved performance over time.
ChatGPT-4: A Communication Bridge
ChatGPT-4, an advanced language model developed by OpenAI, can play a vital role in integrating machine learning techniques into chromatography data analysis. As a state-of-the-art chatbot model, ChatGPT-4 has excellent capabilities in natural language understanding and generation, making it an ideal communication bridge between analysts and machine learning algorithms.
Analysts can interact with ChatGPT-4 using natural language queries and instructions, allowing them to easily access the power of machine learning models without the need for extensive programming or technical expertise. ChatGPT-4 can provide real-time insights, answer questions, and assist in various data analysis tasks related to chromatography.
Moreover, ChatGPT-4 can be trained to understand and interpret specific scientific terms and concepts related to chromatography, allowing for more accurate and context-aware interactions. This contributes to a seamless integration between human expertise and machine learning algorithms, resulting in more efficient and reliable chromatography data analysis.
Conclusion
The integration of more advanced machine learning techniques into chromatography data analysis holds great promise in improving the accuracy, efficiency, and consistency of analytical processes. With the help of ChatGPT-4 as a communication bridge, analysts can seamlessly interact with machine learning models and leverage their capabilities in a user-friendly and intuitive manner.
As technology continues to evolve, we can expect exciting advancements in the integration of machine learning and chromatography, leading to enhanced analytical capabilities and discoveries across various industries.
Comments:
Thank you all for reading and commenting on my article! I'm excited to discuss this topic with you.
Great article, Hank! Integrating machine learning into chromatography technology has the potential to revolutionize the field. Do you think this approach will be applicable to all types of chromatography?
Emily, I believe that the integration of machine learning can improve the accuracy and speed of chromatography analysis. Different analytes may require specific adaptations, but overall, it seems like a promising approach.
Hi Hank, thanks for sharing your insights. I agree with Emily, it's an interesting concept. However, I wonder how much data would be required to train the machine learning models effectively and avoid bias.
Emily and David, thank you for your comments. Machine learning integration can be applicable to various types of chromatography, but the extent of its usefulness might vary. Regarding data requirements, it's crucial to have diverse and representative datasets to train the models and minimize bias.
I find the idea of leveraging ChatGPT for chromatography fascinating. Hank, can you please explain how ChatGPT can enhance the technology specifically?
Sophie, ChatGPT can enhance chromatography technology by providing real-time assistance in method development, troubleshooting, result interpretation, and parameter optimization. It can also help researchers discover patterns and correlations in complex chromatographic data.
Hank mentioned leveraging the power of ChatGPT, but what are the limitations of using such language models in chromatography?
Oliver, the limitations of ChatGPT include its tendency to generate plausible-sounding but incorrect answers. It also heavily relies on the training data, so if there are gaps or biases in the dataset, the model might not provide accurate suggestions. Regular updates and continuous improvement of the models are necessary to address these limitations.
Hank, have there been any practical applications of machine learning in chromatography so far? Any success stories?
Nathan, yes, there have been successful applications. Machine learning has been used to predict retention times, optimize separation conditions, and automate peak detection. These advancements save time, improve accuracy, and could lead to better insights in various fields like pharmaceutical analysis, environmental monitoring, and more.
I wonder if integrating machine learning into chromatography will reduce the need for manual analysis and expert knowledge. What are your thoughts, Hank?
Sara, while machine learning can automate some aspects of chromatographic analysis, it won't eliminate the need for expert knowledge. Expert input is still crucial for training models, interpreting complex results, and making informed decisions based on the analysis. Machine learning is a tool to assist experts, not replace them.
Hank, do you think there will be any ethical concerns when integrating machine learning into chromatography? For example, biases in the data or potential for misinterpretation?
Rachel, ethical concerns are indeed important to consider. Biases in training data can lead to biased results, and misinterpretation of machine-generated suggestions or outputs is always a possibility. To address this, it's crucial to maintain transparency in the training process, diversify datasets, and have human experts involved in decision-making and interpretation.
Hank, what challenges do you foresee in the practical implementation of machine learning integration in chromatography labs?
Adam, there are a few challenges to overcome, such as the availability and quality of data, integration with existing workflows and instruments, and the need for specialized expertise to develop and maintain the machine learning models. Additionally, ensuring data privacy and security is crucial in handling sensitive analytical data.
Hank, do you think machine learning integration will make chromatography more accessible to researchers without extensive analytical chemistry backgrounds?
Jennifer, machine learning integration can simplify certain aspects of chromatographic analysis, making it more accessible to researchers without extensive analytical chemistry backgrounds. However, it's still important for researchers to have a basic understanding of chromatography principles to make informed decisions and interpret results accurately.
Hank, what are the future prospects for machine learning in chromatography? Any emerging trends or exciting possibilities?
Eric, the future looks promising for machine learning in chromatography. Some emerging trends include deep learning approaches, improved data integration from different instruments, real-time monitoring and feedback systems, and even the potential use of augmented reality in lab settings. These advancements will enhance efficiency and accuracy in chromatographic analysis.
Hank, how can researchers get started with machine learning integration in chromatography? Any recommended resources or tools?
Julia, researchers interested in machine learning integration can start by familiarizing themselves with the basics of machine learning and data analysis. There are various online courses, tutorials, and textbooks available. Additionally, open-source tools like TensorFlow and scikit-learn can be used to implement machine learning algorithms. Collaborating with experts in both chromatography and machine learning can also be beneficial.
Hank, how do you see the role of AI in the future of chromatographic instrumentation?
Mark, AI will play a significant role in the future of chromatographic instrumentation. It will enable smarter instrument control, automated method development, adaptive system optimizations, and real-time data analysis. AI-powered instruments can contribute to greater accuracy, higher throughput, and improved efficiency in chromatographic analysis.
Hank, what do you think is the timeline for widespread adoption of machine learning in chromatography labs?
Liam, the timeline for widespread adoption of machine learning in chromatography labs is difficult to predict precisely. However, with the increasing availability of data, advancements in machine learning techniques, and growing interest in automation and efficiency, we can expect a gradual adoption over the next few years as more success stories and practical applications emerge.
Hank, what are the risks associated with relying too heavily on machine learning in chromatography?
Victoria, relying too heavily on machine learning in chromatography can be risky. Over-reliance may lead to blindly accepting model-generated results without critical evaluation, potentially overlooking important factors or introducing errors. It's crucial to maintain a balance between the capabilities of machine learning and human expertise to ensure reliable and accurate analysis.
Hank, what are the possible impacts of machine learning integration on the job market for chromatography professionals?
Christopher, machine learning integration may change the job market for chromatography professionals. While some routine tasks may be automated, the demand for experts who can develop and maintain machine learning models, interpret complex results, and provide critical analyses will likely increase. There will be a shift toward more specialized roles that combine domain knowledge with machine learning expertise.
Hank, as a chromatography professional, how can I prepare myself for the future job market influenced by machine learning?
Alan, staying updated on the latest advancements in machine learning and exploring its applications in chromatography can help you prepare for the future job market. Acquiring knowledge in areas such as data analysis, statistics, and programming will also be beneficial. Collaboration with experts in both chromatography and machine learning can provide valuable insights and learning opportunities.
Hank, what are the cost implications of integrating machine learning into chromatography systems?
Isabella, integrating machine learning into chromatography systems can have cost implications. Initially, there may be costs associated with acquiring the necessary hardware, software, and expertise. However, in the long run, it can lead to cost savings through improved efficiency, reduced analysis time, and better utilization of resources. The cost-benefit analysis would depend on specific lab requirements and the scale of implementation.
Hank, what steps can be taken to ensure the ethical usage of machine learning in chromatography?
Daniel, ensuring ethical usage of machine learning in chromatography requires several steps. These include transparency in data collection, addressing biases and data limitations, regular model updates, human oversight in decision-making, and appropriate data privacy and security measures. Collaboration between experts in both machine learning and chromatography fields is crucial to ensure responsible and ethical implementation.
Hank, can you provide some examples of how ChatGPT can assist with troubleshooting in chromatography labs?
Emma, ChatGPT can assist with troubleshooting in chromatography labs by providing guidance on unexpected results, suggesting possible causes of issues, recommending adjustments to method parameters, and offering insights into instrument performance. It can act as a real-time virtual assistant, helping researchers resolve problems and optimize their chromatographic experiments.
Hank, how can machine learning integration expand the capabilities of chromatography beyond what is currently possible?
Luke, machine learning integration can expand the capabilities of chromatography in several ways. It can improve method development by suggesting optimal conditions for specific sample types. It can also enhance data analysis by identifying hidden patterns and correlations in large datasets. Furthermore, machine learning can enable real-time monitoring, feedback, and control systems, leading to more accurate and efficient chromatographic analysis.
Hank, do you anticipate any regulatory challenges when implementing machine learning in chromatography?
Samuel, implementing machine learning in chromatography might introduce certain regulatory challenges. Ensuring data integrity, validation of the models, and maintaining compliance with existing regulations will be important considerations. It will require collaboration between regulatory authorities, industry experts, and researchers to establish guidelines and best practices for the ethical and accurate use of machine learning in chromatographic analysis.
Hank, what are some of the risks associated with not adopting machine learning in chromatography labs?
Grace, not adopting machine learning in chromatography labs may carry risks. These include slower analysis times, limited capacity for complex data analysis, increased human error, and the inability to leverage the full potential of available data. In a rapidly evolving scientific landscape, harnessing the power of machine learning can help labs stay competitive, improve efficiency, and make more informed decisions.
Hank, are there any concerns about security risks associated with the integration of machine learning into chromatography?
Rebecca, security risks associated with machine learning integration in chromatography need to be addressed. Protecting sensitive data, ensuring secure data transfer and storage, and implementing proper access controls are essential. Additionally, considering potential vulnerabilities in the machine learning models and guarding against adversarial attacks is crucial for maintaining data integrity and system security.
Hank, do you think integrating machine learning will significantly impact the training requirements for chromatography professionals?
Olivia, integrating machine learning will certainly impact the training requirements for chromatography professionals. They will need to acquire knowledge and skills in data analysis, statistics, and machine learning techniques. Furthermore, understanding the underlying principles of machine learning algorithms and the limitations and potential biases associated with them will be essential for effective and responsible use of machine learning in chromatography.
Hank, what are some potential collaborations or interdisciplinary partnerships that can advance the integration of machine learning in chromatography?
Aiden, interdisciplinary partnerships can greatly advance the integration of machine learning in chromatography. Collaboration between chromatography experts, data scientists, machine learning researchers, and software developers can foster new ideas, facilitate knowledge exchange, and drive innovation. Additionally, collaborations with instrument manufacturers, regulatory bodies, and academic institutions can further enhance the application of machine learning in chromatography.