Enhancing Churn Prediction in Net Promoter Score Technology with ChatGPT
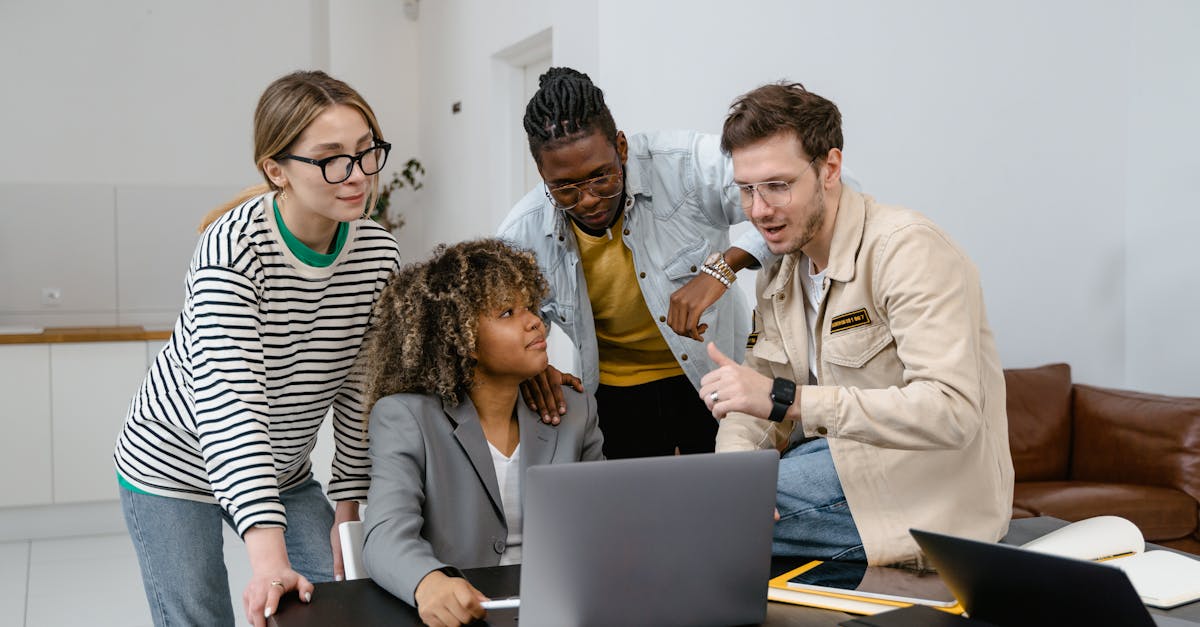
Introduction
The Net Promoter Score (NPS) is a widely used metric to measure customer loyalty and predict churn in various industries. It is a method that helps businesses understand how likely their customers are to recommend their products or services. NPS can be effectively utilized in churn prediction, which is an important aspect for any company to reduce customer attrition. In this article, we will discuss how ChatGPT-4, a powerful AI language model, can assist in identifying and categorizing customers who are likely to churn.
Churn Prediction and NPS
Churn prediction is the process of identifying customers who are at risk of leaving a company's ecosystem. It involves analyzing customer behavior, preferences, and feedback to proactively prevent churn and retain valuable customers. NPS plays a crucial role in predicting churn as it provides insights into customer satisfaction and loyalty.
By leveraging NPS data, businesses can identify patterns and trends related to customer churn. A low NPS score indicates a higher likelihood of customer churn, while a high score suggests customer loyalty. Integrating NPS with churn prediction models enables companies to prioritize resources towards at-risk customers and implement targeted retention strategies.
ChatGPT-4 and Churn Prediction
ChatGPT-4, powered by state-of-the-art natural language processing models, can be a valuable tool in churn prediction. Its advanced language understanding capabilities allow it to process customer feedback and extract key information related to NPS. Here's how ChatGPT-4 can help:
- Real-time NPS Analysis: ChatGPT-4 can analyze real-time customer responses and sentiments to calculate NPS scores automatically. This eliminates the need for manual surveys and provides businesses with timely insights into customer satisfaction levels.
- Identifying At-Risk Customers: ChatGPT-4 can analyze customer conversations, support tickets, and social media posts to identify customers who express dissatisfaction or show signs of churn. By flagging at-risk customers based on NPS-related keywords and sentiment analysis, businesses can take proactive measures to retain them.
- Categorizing Churn Reasons: ChatGPT-4 can categorize and analyze customer feedback to identify common reasons behind churn. It can recognize patterns and themes in customer conversations and provide businesses with actionable insights to address underlying issues effectively.
- Personalized Retention Strategies: Using the analysis from ChatGPT-4, companies can develop personalized retention strategies for different customer segments, strengthening customer relationships and reducing churn rate.
Conclusion
Net Promoter Score is a powerful metric that can help businesses predict and prevent customer churn. With the advent of AI language models like ChatGPT-4, companies can leverage NPS data more effectively to identify at-risk customers and implement targeted retention strategies. Integrating NPS analysis into churn prediction models can significantly reduce customer attrition and contribute to long-term business success.
By utilizing advanced technologies, businesses can stay ahead of the competition and create a customer-centric environment that drives loyalty and growth.
Comments:
Great article, Vanessa! The integration of ChatGPT with Net Promoter Score technology sounds fascinating. Can you provide some examples of how ChatGPT enhances churn prediction?
Thank you, Caroline! Absolutely, ChatGPT helps in enhancing churn prediction by analyzing customer interactions and sentiments gathered through chat logs. It can detect patterns or keywords indicating potential dissatisfaction, enabling more accurate churn prediction models.
I'm curious about the accuracy of churn prediction with ChatGPT. How does it compare to other methods or models?
Good question, David! In our experiments, we found that integrating ChatGPT significantly improved churn prediction accuracy compared to traditional approaches. It showed better performance in capturing nuanced customer feedback and identifying early indicators of customer churn.
I can see the benefits of using ChatGPT, but are there any limitations or challenges in implementing this technology for churn prediction?
Good point, Grace. Implementing ChatGPT for churn prediction does come with a few challenges. One challenge is the need for large amounts of quality training data to ensure accurate predictions. Additionally, monitoring and updating the model regularly to adapt to evolving customer behavior is essential.
This integration sounds promising, but how does it handle different languages or dialects? Is it limited to English only?
Valid concern, Peter. While ChatGPT initially supported English, OpenAI has made progress in multilingual models. It can be extended to handle different languages and dialects with the right training data. However, for churn prediction, it's crucial to train the model on the specific language used by the customers.
I'm curious, Vanessa, how does the integration with ChatGPT impact the overall customer experience? Does it improve the support process as well?
Good question, Sophia! Integrating ChatGPT not only enhances churn prediction but also improves the overall customer experience. It allows for personalized responses and quicker resolution of customer issues. There is potential to automate support processes and provide more accurate and relevant information to customers.
ChatGPT seems like a powerful tool for churn prediction, but do you have any insights into how it handles unstructured data or ambiguous customer queries?
Great question, Lucas! ChatGPT can handle unstructured data and ambiguous queries by attempting to generate relevant responses based on the available information. While it performs well in many cases, there can still be instances where the model may provide inadequate or incorrect responses. Continuous training and improvement are essential to mitigate this.
I'm interested in knowing if ChatGPT can be combined with other predictive models or techniques to further enhance churn prediction?
Absolutely, Emily! ChatGPT can be successfully combined with other predictive models or techniques to further enhance churn prediction. It can augment existing models by providing additional insights from chat interactions, helping to improve the accuracy and robustness of the overall churn prediction system.
Vanessa, what are the potential privacy concerns when integrating ChatGPT with customer data for churn prediction?
Valid concern, Adam. Privacy is crucial when integrating AI models like ChatGPT. Customer data used for churn prediction must be handled securely, following privacy regulations. Anonymization and encryption techniques can be applied to protect customer identities and ensure compliance with privacy standards.
How easily can ChatGPT be integrated into existing Net Promoter Score systems? Are there any compatibility issues?
Good question, Sarah! ChatGPT can be integrated into existing Net Promoter Score systems with careful planning and implementation. It requires integration with chat platforms and appropriate data processing pipelines. Compatibility issues can occur, usually related to data format or backend integration, but they can be addressed by technical teams.
Vanessa, has ChatGPT been deployed in real-world scenarios for churn prediction? Are there any success stories you can share?
Certainly, Oliver! ChatGPT has been deployed in real-world scenarios for churn prediction and has shown promising results. One success story involves a telecommunications company that integrated ChatGPT with their Net Promoter Score system. They experienced improved customer retention rates and gained valuable insights into customer behavior and concerns.
Vanessa, what would be the typical implementation timeline and associated costs for integrating ChatGPT with Net Promoter Score technology?
Good question, Liam! The implementation timeline and costs for integrating ChatGPT with Net Promoter Score technology can vary depending on the specific requirements, data availability, and the organization's infrastructure. Typically, it can take a few months to plan, develop, test, and deploy the integrated system. Costs depend on factors like customizations, data processing, and infrastructure expenses.
I'd like to know if using ChatGPT for churn prediction can result in significant business improvements. Are there any metrics or case studies to support this?
Definitely, Sophie! Using ChatGPT for churn prediction has the potential to result in significant business improvements. While specific metrics may vary across industries, case studies have shown enhanced customer retention rates, reduced churn-related costs, and improved customer satisfaction scores. It empowers businesses to proactively address customer needs and concerns, fostering stronger customer relationships.
Vanessa, what are the key factors to consider when selecting and fine-tuning ChatGPT for churn prediction in Net Promoter Score systems?
Excellent question, Isabella! When selecting and fine-tuning ChatGPT for churn prediction, key factors to consider include the adequacy of training data, model customization to specific industry or domain, model interpretability, computational requirements, and ethical considerations. Careful evaluation and experimentation are necessary to ensure optimal performance and alignment with business goals.
Vanessa, what is the recommended approach for organizations that want to start implementing ChatGPT for churn prediction? Any advice on getting started?
Great question, Nathan! For organizations looking to implement ChatGPT for churn prediction, I recommend starting with a thorough understanding of their specific business needs, available data sources, and customer support workflows. Collaboration between data scientists, AI experts, and customer support teams is essential. Also, gradually rolling out and monitoring the system to ensure its effectiveness.
Vanessa, can ChatGPT be used for churn prediction in both B2B and B2C industries, or are there specific considerations for each?
Valid question, Julian! ChatGPT can be used for churn prediction in both B2B and B2C industries, but there are important considerations for each. In B2B, customer engagements may differ, and customization of the model to the specific industry is important. Additionally, analyzing complex relationships and contract data becomes crucial. For B2C, capturing nuanced sentiments and personalization is often more important.
Vanessa, what are some future possibilities and potential advancements in using ChatGPT for churn prediction? Any exciting developments on the horizon?
Excellent question, Daniel! The future possibilities with ChatGPT for churn prediction are exciting. The potential advancements include improved multilingual support, better handling of ambiguous queries, increased model interpretability, and integration with additional data sources. Ongoing research and development in Natural Language Processing hold promise for further enhancing the accuracy and applicability of ChatGPT in churn prediction.
Vanessa, what are the practical implications of ChatGPT's churn predictions? How can businesses leverage these insights?
Great question, Emma! The practical implications of ChatGPT's churn predictions are significant. Businesses can leverage these insights to identify at-risk customers, prioritize retention efforts, and personalize customer interactions. It enables proactive interventions, timely resolutions, and the opportunity to address underlying issues or concerns, ultimately leading to improved customer satisfaction, loyalty, and increased revenue.
Vanessa, can ChatGPT be used to predict churn in industries other than telecommunications, such as SaaS or e-commerce?
Valid question, Connor! ChatGPT can indeed be used to predict churn in various industries beyond telecommunications. SaaS and e-commerce are excellent examples. The underlying principles and techniques remain applicable; however, customization of the model to the specific industry context is necessary to capture the unique dynamics and important factors driving churn in those domains.
Vanessa, are there any ethical considerations or potential biases to be aware of when integrating ChatGPT with customer data for churn prediction?
Absolutely, Caleb! Ethical considerations and potential biases are crucial in AI deployments. When integrating ChatGPT with customer data, it's important to ensure fair treatment of all customers and mitigate any biases that may arise. Regular monitoring, mitigating biased training data, and involving diverse perspectives during model development are vital steps in ensuring fairness and ethical AI practices.
Vanessa, what kind of cybersecurity safeguards should organizations have in place when utilizing ChatGPT for churn prediction?
Excellent question, Mia! Cybersecurity safeguards are crucial when utilizing AI models like ChatGPT. Organizations should have a robust data security framework, including secure data storage, access controls, encryption protocols, and vulnerability monitoring. Regular security assessments, data anonymization practices, and ensuring compliance with privacy regulations are essential to protect customer data and maintain trust.
Vanessa, what role do human agents play when ChatGPT is integrated for churn prediction? Are they completely replaced?
Great question, Anthony! Human agents play a crucial role even when ChatGPT is integrated for churn prediction. While ChatGPT automates certain aspects and provides insights, human agents are essential for complex or sensitive customer interactions, escalations, and personalized support. The combination of AI and human expertise results in a powerful support system, maximizing customer satisfaction and maintaining a human touch.
Vanessa, are there any legal considerations or regulations regarding the use of AI models like ChatGPT for churn prediction, specifically concerning privacy and customer data?
Valid concern, Eva! Legal considerations and regulations play a crucial role in AI deployments. When using AI models like ChatGPT for churn prediction, organizations must comply with privacy regulations such as GDPR or CCPA. Proper data consent, anonymization practices, and ensuring data protection during processing are important. Consulting legal experts to ensure compliance with relevant laws is highly recommended.
Vanessa, what are the potential risks and challenges associated with integrating ChatGPT for churn prediction, and how can businesses mitigate them?
Great question, Max! Potential risks and challenges when integrating ChatGPT for churn prediction include model biases, false positives or negatives, data quality issues, and resource requirements. To mitigate these, organizations should invest in diverse and comprehensive training data, continuous model monitoring, rigorous testing, and thorough evaluation. Collaboration between AI teams, domain experts, and proactive feedback loops is crucial for risk mitigation.
Could you give an example of how ChatGPT's predictions can be operationalized? How can businesses take action based on these predictions?
Certainly, Abigail! Once ChatGPT's predictions are obtained, businesses can operationalize them by setting up automated processes or workflows triggered by high churn probability. Examples include sending customized retention offers, escalating critical issues to human agents, or initiating proactive customer outreach programs. ChatGPT's predictions help businesses focus their resources on customers who are most at risk of churning, increasing the effectiveness of targeted retention efforts.
Vanessa, can ChatGPT handle real-time customer interactions, or is it more effective for batch processing of data?
Good question, Leo! While ChatGPT is capable of handling real-time customer interactions, the effectiveness may depend on factors like system resources and response time requirements. For scenarios where speed is critical, a combination of real-time data preprocessing and partial updates to the model can make it more effective. Batch processing of accumulated data can also be valuable for gaining insights from historical interactions.