Enhancing Cognitive Load Theory with ChatGPT: Leveraging Cognitive Science Technology for Superior Learning Experiences
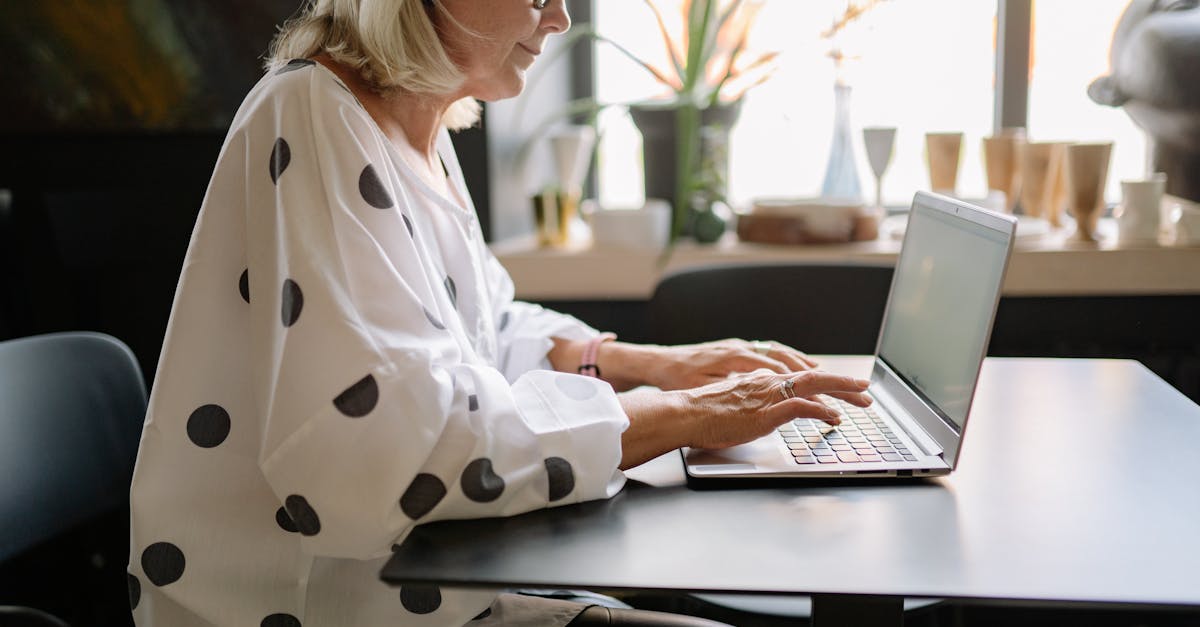
The realm of cognitive science unfoldss myriad intricacies of human brain and one such fasciating concept is Cognitive Load Theory (CLT). CLT is an instructional theory that utilizes our understanding of cognitive processes to create instructional materials and learning experiences that are effectively tailored to our cognitive architecture.
The cognitive load theory (CLT) is built on two fundamental concepts: the architecture of the human cognitive system and the structures and functions associated with this architecture. There are three types of cognitive load: intrinsic, extraneous, and germane. Intrinsic cognitive load is related to the inherent complexity of the material. Extraneous cognitive load is related to how the information is presented. Germane cognitive load refers to the cognitive processing associated with the formation of schemas which help in organizing and integrating information.
Understanding these intricate nuances can be challenging, but thanks to the advent of AI technologies like ChatGPT4, this has become much easier. An intriguing exploration reveals the potential of AI in shedding light on the cognitive load theory.
The Interface of ChatGPT-4 and Cognitive Load Theory
ChatGPT-4, an AI-based tool, replicates human-like text based on a given input. The application of AI models extends to mimicking cognitive processes, providing us with an opportunity to study and simulate cognitive load. This artificial intelligence model can serve as a unique approach to learning the concept of cognitive load theory by acting as a modeled student.
In this context, the interaction between AI and CLT creates a phenomenal scope for studying the cognitive processes more wholesomely. For instance, AI can simulate the effects of cognitive load on learning and thus provide a better understanding of how to create materials that align with our cognitive architecture.
The interplay between CLT and AI such as ChatGPT-4 emphasizes the significance and interdependence of cognitive science, technology, and artificial intelligence. Here’s how exactly ChatGPT-4 can assist.
Using ChatGPT-4 to Simulate Cognitive Load
One of the major utilities of ChatGPT-4 in this arena is its ability to simulate cognitive load. It can mirror the cognitive behavior of a learner and provide real-time adaptive responses. For instance, when presented with a complex concept, the AI can simulate the difficulty of understanding the concept and sequentially breaking the information down, representing cognitive overload.
The AI model can also emulate cognitive strategies employed by humans to handle the cognitive load, such as the use of schemas. For instance, it can demonstrate the creation and use of a schema to simplify and understand a complex idea, thus aiding in reducing the cognitive load.
Modifying Information Presentation
ChatGPT-4 can also assist in studying the extraneous cognitive load, i.e., how the presentation of information can affect the cognitive load. The AI can be modeled to react differently to variations in the presentation of the same information, thus helping to illustrate the impact of different instructional design choices on cognitive load.
Expanding Knowledge of the Schemas
Moreover, the AI’s capability to simulate the use of schemas for organizing and integrating information offers profound insights into how our cognitive system works to manage the germane cognitive load.
By observing how the AI organizes information into schemas and applies these schemas to understand new information, we can gain a better understanding of the construction and utilization of schemas in managing our cognitive resources.
Enhancing Educational Strategies
Lastly, the insights generated through these simulations can help enhance our educational strategies. For example, by learning how to manage cognitive load effectively, we can devise better instructional materials that align with our cognitive architecture and thus enhance the efficacy of learning and retention.
Conclusion
ChatGPT-4, with its exceptional ability to simulate cognitive processes, can greatly aid in the exploration and understanding of cognitive load theory. Through serving as an AI student, this technology provides an accurate and efficient way to observe cognitive load in action. Consequently, the interplay of AI and CLT opens up exciting avenues in the realm of cognitive science and education.
Comments:
Thank you all for reading my article on enhancing Cognitive Load Theory with ChatGPT! I'm excited to hear your thoughts and engage in some interesting discussions.
Great article, Jon! I particularly liked how you explained the benefits of leveraging Cognitive Science Technology for learning experiences. It definitely seems like a promising approach.
Thank you, Emily! I believe incorporating ChatGPT can greatly enhance the learning process by providing personalized and interactive experiences. Do you think there are any specific areas or subjects where this approach would be particularly effective?
Jon, your article really got me thinking about how technology can revolutionize education. I wonder though, could there be any potential drawbacks with incorporating AI like ChatGPT into learning environments?
That's a valid question, Mark. While AI technology has tremendous potential, it's important to consider the limitations. One concern could be the reliability of AI-generated responses and ensuring accurate information is provided. However, with proper training and monitoring, we can mitigate these challenges. It's an ongoing area of research.
I appreciate how you emphasized the role of cognitive load theory in learning, Jon. It's crucial to find ways to manage cognitive load and ensure optimal learning experiences. ChatGPT's ability to adapt to individual needs seems promising in this regard.
Thanks for your comment, Linda! Indeed, optimizing cognitive load is fundamental for effective learning. The adaptability of ChatGPT can help tailor content delivery and provide individualized support to learners. I'm glad you found it promising!
Interesting read, Jon! I'm curious to know how the incorporation of ChatGPT affects student engagement. Are there any studies or research that demonstrate its impact on motivation and attentiveness?
Thank you for your question, David. There are indeed studies suggesting that interactive and personalized experiences can enhance motivation and engagement in students. While specific research on ChatGPT's impact is still emerging, earlier work on similar technologies is promising. Further research and experimentation will help uncover the full potential.
Hi Jon! As an educator, I find the idea of incorporating AI in the learning process fascinating. However, do you think teachers might feel threatened by this technology? Could it potentially replace their role?
Hi Sarah! I understand the concerns some educators might have. However, rather than replacing teachers, AI technologies like ChatGPT can act as valuable aids. They can assist teachers in providing personalized feedback, addressing individual challenges, and amplifying the learning experience. It can augment the teaching process rather than replace it.
Great article, Jon! I wonder, though, how accessible and inclusive this technology will be. Are there any efforts being made to ensure it caters to learners with diverse needs and challenges?
Thank you, Ryan! Accessibility and inclusivity in education are crucial. Efforts are being made to ensure that AI technologies are accessible to learners with diverse needs. Customization options, usage guidelines, and ongoing research aim to address these aspects and make sure the technology can benefit all learners.
Hey Jon! This article got me thinking about the potential for using ChatGPT in corporate training environments. Do you think it could be an effective tool in the professional development sector as well?
Hi Alex! Absolutely, ChatGPT's adaptability can make it a valuable tool in corporate training as well. It can assist in providing interactive scenarios, personalized feedback, and on-demand learning resources. The technology holds great potential across various learning sectors.
Really interesting article, Jon! I can see how incorporating ChatGPT can make learning more engaging and interactive. However, how do you think this technology will evolve in the future? Any exciting advancements to look forward to?
Thanks, Sophie! The field of AI and cognitive science is rapidly advancing. In the future, we can expect more refined AI models, improved natural language processing, and enhanced adaptability. These advancements will enable even better personalized learning experiences, ultimately leading to superior learning outcomes.
Jon, your article convinced me of the potential benefits of incorporating AI into the learning process. However, do you think there are any ethical considerations we need to keep in mind while using AI technologies like ChatGPT in education?
Hi Peter! Ethical considerations are indeed important. Transparent and responsible use of AI technologies is crucial. We need to ensure privacy, data security, and avoid biases. Additionally, ongoing research and open dialogue can help address ethical concerns and ensure the technology is used ethically in educational settings.
Thank you for the informative article, Jon. I am excited about the potential of ChatGPT in personalized learning. Do you think this technology will be accessible to learners from different socio-economic backgrounds?
You're welcome, Laura! Accessibility is a crucial aspect. Efforts are being made to ensure that AI technologies like ChatGPT are made accessible and affordable to learners from different socio-economic backgrounds. Collaboration between researchers, policymakers, and educational institutions can help bridge any digital divide and make these tools widely accessible.
Hey Jon, great article! I was wondering how the incorporation of ChatGPT could impact collaborative learning or group activities. Do you think it can assist in facilitating group discussions effectively?
Hi Oliver! That's an interesting point. ChatGPT's interactive nature can indeed be leveraged to facilitate group discussions and collaborative learning. It can serve as a mediator, helping participants share ideas and providing additional insights. Proper integration and functionalities can make it a valuable tool for such activities.
Jon, the article presents an exciting perspective on the future of learning. However, are there any specific challenges we need to address while implementing ChatGPT in educational systems?
Thank you, Elena! While implementing ChatGPT, a challenge is ensuring a balance between automated AI responses and personal interactions with teachers. It's crucial to avoid over-reliance on technology and maintain human connections in the learning process. Striking this balance is essential for successful implementation.
Great article, Jon! I would like to know if ChatGPT can adapt to different learning styles, such as visual, auditory, or kinesthetic. How flexible is the technology in accommodating diverse learner preferences?
Thanks, James! ChatGPT's flexibility allows for customization based on varied learning styles. By incorporating multimedia elements, adaptive content delivery, and interactive interfaces, it can cater to visual, auditory, and kinesthetic learners. The adaptability makes it a powerful tool for personalized instruction across different modalities.
Hi Jon! Your article got me thinking about teacher training. Do you think incorporating AI technologies like ChatGPT can benefit teacher professional development and help them enhance their instructional strategies?
Hi Sophia! Absolutely, AI technologies can play a significant role in teacher professional development. ChatGPT can assist educators in gaining insights, suggesting teaching strategies, and providing ongoing support. It can act as a virtual mentor, helping teachers continuously improve their instructional approaches and adapt to changing educational landscapes.
Jon, your article raises an interesting point. However, do you think students might become overly dependent on ChatGPT and struggle with critical thinking and problem-solving skills?
That's a valid concern, Michael. Ensuring a balanced approach is essential. ChatGPT should be used as a tool to enhance learning, not replace critical thinking. It should be integrated with activities that develop problem-solving skills, encouraging students to think analytically and independently. Combining the best aspects of human guidance and AI assistance can strike this balance.
Jon, your article got me excited about the potential for ChatGPT in language learning. Can this technology effectively assist in language acquisition and practice?
Definitely, Amy! ChatGPT's ability to engage in conversations and provide language practice can be a valuable asset in language learning. Learners can practice their speaking and writing skills, and the technology can offer feedback and suggestions for improvement. It can create more interactive and immersive language learning experiences.
Jon, your insights into enhancing Cognitive Load Theory with ChatGPT are compelling. Could you please elaborate on how individualized content delivery can be achieved using this technology?
Certainly, Eric! ChatGPT's ability to adapt to individual needs and preferences allows for personalized content delivery. By analyzing learner interactions, it can identify knowledge gaps, adjust difficulty levels, and offer targeted explanations and examples. This way, learners receive content that matches their current level and learning pace, optimizing their cognitive load.
Hi Jon! Interesting article! I was wondering if ChatGPT can be effective for students with learning disabilities or special needs. Can it provide appropriate support tailored to their specific requirements?
Hi Rachel! That's an important consideration. ChatGPT's adaptability can be leveraged to provide individualized support to students with learning disabilities or special needs. Customizable interfaces, tailored feedback, and designated learning paths can ensure appropriate support based on their requirements. It holds promise for inclusive education.
Great article, Jon! I wonder if ChatGPT can provide real-time feedback during assessments or exams. Could it help in monitoring students' progress and identifying areas for improvement?
Thank you, Max! ChatGPT can indeed offer real-time feedback during assessments and exams. Its natural language processing capabilities can evaluate student responses and provide immediate feedback. This helps in monitoring students' progress, identifying areas for improvement, and offering personalized guidance. It adds an interactive element to the assessment process.
Jon, your article opens up exciting possibilities for the future of education. I'm curious to know if there are any potential privacy concerns associated with using AI technologies like ChatGPT?
Hi Natalie! Privacy concerns are indeed important. When handling data, it's essential to follow privacy regulations and ensure secure usage of AI technologies. Proper data protection measures and anonymization techniques help address these concerns. Maintaining trust and transparency with users is crucial in this regard.
Great read, Jon! I can see how ChatGPT can make learning more interactive and engaging. However, how can we design effective user interfaces to optimize the learning experience?
Thank you, Olivia! Designing effective user interfaces is essential for optimal learning experiences. The interfaces should be intuitive, visually appealing, and enable easy interaction. Incorporating multimedia elements, clear navigation, and context-based prompts can enhance user engagement and help learners derive maximum benefit from ChatGPT's functionalities.
Jon, your article highlighted the potential of ChatGPT for personalized learning experiences. I'm interested to know if this technology can also assist in vocational training or skill development.
Hi Ethan! Absolutely, ChatGPT's adaptability can extend to vocational training and skill development. By tailoring content to specific professions or desired skills, it can assist in providing industry-specific knowledge, practice simulations, and personalized guidance. The technology can be valuable in preparing individuals for the workforce and vocational success.
Jon, I enjoyed reading your article! How do you think the implementation of ChatGPT might affect the role of traditional textbooks and learning materials?
Thanks, Tom! While AI technologies like ChatGPT can augment learning experiences, traditional textbooks and learning materials still hold value. They provide comprehensive knowledge and serve as essential reference materials. ChatGPT can complement these resources by offering interactive support and personalized learning paths, creating a well-rounded learning approach.
Hi Jon! Your article got me thinking about the potential challenges in implementing AI technologies in resource-limited settings. How can we ensure that ChatGPT remains accessible in such contexts?
Hi Hannah! Ensuring accessibility in resource-limited settings is crucial. Efforts need to be made to optimize ChatGPT's performance on different devices, including low-end hardware. Additionally, collaborations with organizations and policymakers can help make AI technologies like ChatGPT accessible through initiatives focused on internet access, affordability, and bridging the digital divide.
Thank you all for your valuable comments and engaging in this discussion! Your insights and queries have added depth to the conversation and highlighted important aspects of incorporating AI technologies like ChatGPT in education. It was a pleasure discussing this exciting topic with each of you!