Enhancing Commodity Risk Management in Technology with Gemini
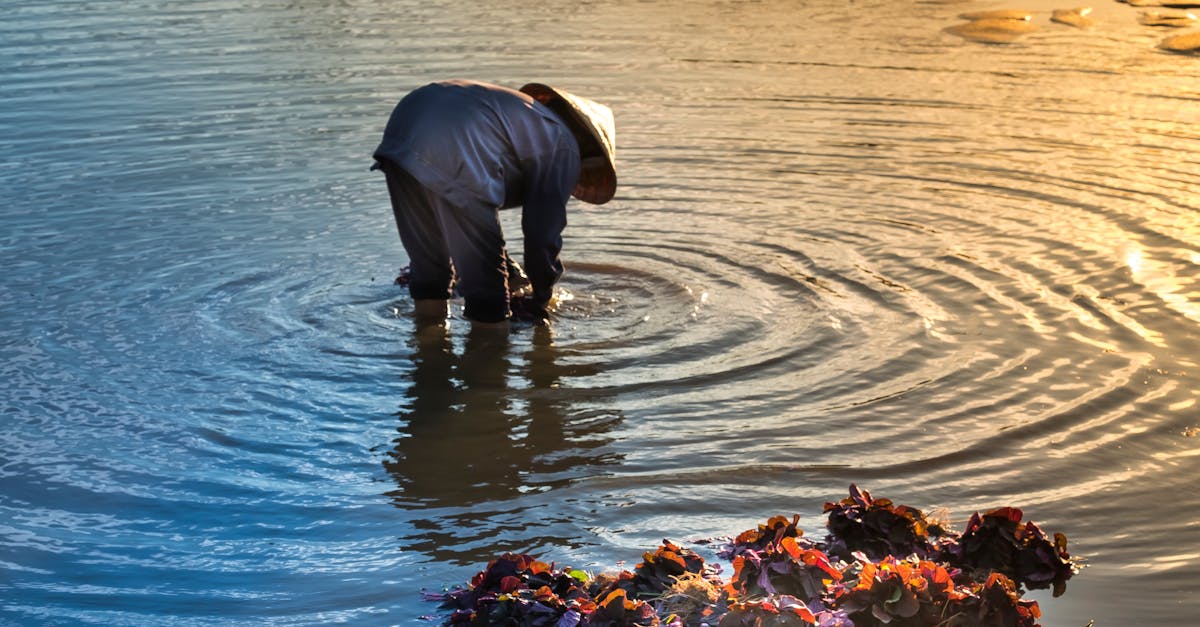
Commodity risk management is a crucial aspect of any business operating in the technology industry. With rapid advancements and evolving market dynamics, managing commodity risks effectively is essential to ensure sustainable growth and profitability. Traditionally, commodity risk management has been performed through human expertise, data analysis, and industry insights. However, recent developments in artificial intelligence (AI), specifically Natural Language Processing (NLP), have paved the way for more advanced and efficient risk management techniques.
The Power of Gemini
Gemini, developed by Google, is a state-of-the-art language model that utilizes advanced NLP techniques to understand and generate human-like text responses in a conversational manner. The technology has quickly gained popularity due to its ability to engage in meaningful discussions and provide accurate information on a wide range of topics.
By leveraging the power of Gemini, technology companies can enhance their commodity risk management strategies by improving forecasting accuracy, identifying potential risks, and making informed decisions based on real-time market insights.
Enhancing Forecasting Accuracy
Accurate forecasting is critical for effective commodity risk management as it helps businesses plan and mitigate potential risks associated with commodity price fluctuations. Leveraging Gemini's advanced NLP capabilities, companies can feed the model with historical and real-time data, enabling it to analyze patterns, identify trends, and generate more accurate forecasts. This empowers businesses to make well-informed decisions regarding inventory management, pricing strategies, and hedging options.
Identifying Potential Risks
Commodity markets are prone to various risks, such as supply chain disruptions, geopolitical instability, and regulatory changes. Gemini can help technology companies identify and assess potential risks by analyzing large volumes of unstructured data from diverse sources, such as news articles, social media feeds, and industry reports. By understanding the context and sentiment behind this data, the model can provide valuable insights on current and emerging risks, allowing businesses to proactively address them.
Making Informed Decisions
Informed decision-making plays a vital role in mitigating commodity risks and maximizing operational efficiency. Gemini can act as a virtual assistant, providing real-time market insights, answering queries, and offering suggestions based on its understanding of the industry and market trends. This technology can assist in decision-making processes related to procurement, inventory management, and contract negotiations, helping businesses optimize their commodity risk management practices.
Conclusion
As technology continues to evolve, so does the need for more advanced and efficient risk management techniques. Gemini, with its powerful NLP capabilities, is poised to revolutionize commodity risk management in the technology industry. By enhancing forecasting accuracy, identifying potential risks, and facilitating informed decision-making, Gemini empowers businesses to navigate the complexities of commodity markets, minimize risks, and achieve sustainable growth and profitability in a rapidly changing business environment.
Comments:
Thank you all for your comments! I appreciate your thoughts on the topic.
I found this article on Commodity Risk Management very informative. It's interesting how Gemini can be utilized to enhance this process.
Indeed, Alex! Integrating AI-powered technology like Gemini into risk management can assist in better decision making.
However, I'm concerned about the potential for inaccuracies in AI-driven risk management systems. Can Gemini really provide reliable insights?
That's a valid concern, Craig. While AI technologies like Gemini have their strengths, it's crucial to have proper checks and balances in place.
Agreed, Ely. AI should be seen as a tool to enhance decision making, not replace human judgment.
I think with proper training and validation, AI systems can provide valuable insights for risk management.
I wonder how Gemini can handle the complexities of commodity markets. There's so much data and factors to consider.
Great point, John. Gemini can analyze large datasets and generate suggestions, but human expertise is still essential in understanding market complexities.
Exactly, Ely. Successful commodity risk management requires a combination of AI capabilities and human judgment.
I'm curious about the potential risks and challenges in implementing Gemini for commodity risk management. Any thoughts?
One challenge could be ensuring the accuracy and reliability of the data fed into Gemini, as it heavily influences the outcomes.
You're right, Kim. Data quality is crucial, and ensuring it is accurate and up-to-date is vital for successful implementation.
Another risk could be overreliance on AI suggestions without proper human validation. Human oversight is necessary to avoid potential biases or incorrect conclusions.
Would an AI system like Gemini be able to adapt to changing market conditions? Commodity markets can be quite volatile.
Adaptability is an essential aspect, Jake. AI models like Gemini can be trained on historical data and continuously updated to stay relevant.
However, relying solely on historical data might not be sufficient for predicting future market behavior. External factors and events can significantly impact commodity prices.
Absolutely, Andrew. The AI system should be flexible enough to incorporate real-time data and external events to provide accurate risk assessments.
I'm concerned about the potential lack of transparency in AI systems like Gemini. It might be difficult to understand and justify the decisions made.
That's a valid concern, Sarah. Interpretability and explainability of AI models should be a key focus to address any lack of transparency.
Indeed, Tina. Ensuring transparency enables users to trust and validate the decisions made by AI systems.
I think incorporating AI technologies in commodity risk management can significantly improve efficiency and reduce manual effort.
True, Alex. Automation through AI can free up valuable time for risk managers to focus on strategy and critical thinking.
One important aspect is the collaboration between AI systems and risk management experts to achieve optimal outcomes.
What about the potential ethical implications of AI in commodity risk management? Are there any concerns regarding its impact on market fairness?
Ethics are indeed crucial, Craig. Bias detection and mitigation techniques should be implemented to ensure fairness in AI systems.
Agreed, Tina. It's vital to thoroughly evaluate and address any ethical implications to prevent unfair advantages in markets.
I think implementing AI in commodity risk management should be a gradual process to carefully assess its impact and make necessary adjustments.
That's a wise approach, Kim. A step-by-step implementation strategy allows for learning from challenges and improving the system.
Overall, I believe integrating AI technologies like Gemini in commodity risk management has great potential, but careful implementation and ongoing monitoring are necessary.
Thank you for sharing your thoughts, Jake. Continuous improvement and vigilance are key to harnessing the benefits of AI in risk management.
I agree with Ely. It's essential to strike a balance between AI capabilities and human judgment in order to effectively manage commodity risks.
Absolutely, Andrew. The synergy between AI and human expertise can lead to better decision making and risk management outcomes.
Thank you all for this insightful discussion! It's been great exchanging ideas on how Gemini can enhance commodity risk management.
Indeed, Tina. I'm glad to see such diverse perspectives and valuable insights from everyone.
Thank you, Ely, for initiating this discussion and providing us with this informative article. It's been a pleasure participating.
I second that, Kim. It was an engaging discussion, and I've learned a lot from the insights shared by the participants.
Thank you all for an enlightening conversation! This exchange of ideas has broadened my understanding of the topic.
You're most welcome, Sarah. I'm delighted that this discussion has been beneficial for everyone involved.
I appreciate being part of this discussion as well. Thank you, Ely, and fellow participants, for sharing your thoughts.
Thank you too, John. Your insights added valuable perspectives to the conversation.
It was a pleasure interacting with all of you. Let's continue exploring ways to utilize AI for effective risk management.
Indeed, Jake. This discussion has provided food for thought and motivated me to further explore this topic.
I'm thrilled to hear that, Craig! Feel free to reach out if you have any further questions or insights.
Thank you all once again! Let's stay curious and keep advancing technology-driven risk management.
Absolutely, Alex! Continuous learning and innovation are key to staying ahead in the ever-evolving risk management landscape.
Couldn't agree more, Tina. Let's embrace the opportunities AI offers while ensuring responsible and effective risk management.
Thank you, everyone! Looking forward to further discussions and advancements in this exciting field!
Likewise, Kim. Let's keep pushing the boundaries of commodity risk management with the power of AI.
Thank you all once again for joining this discussion. Your participation has been invaluable. Let's continue this journey together!
Great article, Ely! The use of Gemini for commodity risk management in technology sounds promising. Can you provide some examples of how it can be applied?
Thank you, Alex! Gemini can be used to analyze market trends, predict commodity prices, and assist in decision-making by providing insights into potential risks and strategies. It can also help automate risk assessment processes. Do you have any specific questions?
I'm curious about the data requirements for training Gemini to handle commodity risk management. How much data does it need and what kind of data works best?
Great question, Olivia! Training Gemini for commodity risk management requires a significant amount of relevant data, such as historical commodity prices, market data, and risk factors. The more diverse and representative the data, the better the model's performance. However, it's important to clean and preprocess the data to ensure accuracy. Do you have any experience working with training data?
Thanks for the response, Ely! I do have some experience working with training data, mainly in the financial sector. Are there any specific challenges or considerations when applying Gemini to commodity risk management?
Certainly, Olivia! One challenge is that commodity markets can be affected by various factors, including geopolitical events, weather conditions, and supply chain disruptions. These factors can introduce volatility and unpredictability, making risk management more complex. Gemini can help analyze these factors but may not capture all possible contextual nuances. Human expertise is still essential for critical decision-making. What other thoughts or questions do you have?
I wonder how effectively Gemini can adapt to changing market conditions. Commodity prices can fluctuate rapidly. Can it keep up with real-time data and provide accurate insights?
That's a valid concern, Mark. Gemini can be updated with new data to adapt to changing market conditions, but it may not be able to process real-time data in an instant manner. Depending on the application, a hybrid approach combining Gemini and real-time data feeds or APIs could provide more accurate and up-to-date insights. It's crucial to strike a balance between automation and human oversight in real-time decision-making. Any further thoughts on this?
Thank you, Ely! Striking the right balance is indeed important. It seems like Gemini can be a valuable tool if integrated well within a comprehensive risk management system. Are there any limitations or potential risks we should be aware of when using Gemini for commodity risk management?
Absolutely, Mark. While Gemini has shown great potential, it's important to be aware of its limitations. It can generate plausible-sounding but potentially incorrect or biased outputs, especially when dealing with scarce or incomplete data. Careful validation and risk assessment processes need to be in place. Additionally, keeping the model up-to-date and monitoring its performance over time is crucial. Balancing human expertise and machine assistance is key. Any other concerns or questions?
I'm impressed by the possibilities Gemini offers in enhancing commodity risk management. How accessible is Gemini for businesses, and what are the potential costs involved in implementation?
Thanks for your interest, Grace! Access to Gemini is available through Google's API, which provides businesses with programmatic access. The costs involved depend on usage and can vary. You can find more information on Google's pricing page to estimate the specific costs for your implementation needs. Let me know if there's anything else I can assist with!
Gemini sounds like a valuable addition to commodity risk management, but what are the potential implementation challenges? Are there any technical requirements or skill sets needed?
Good question, Jasmine! Implementing Gemini for commodity risk management may require technical expertise in data preprocessing, model fine-tuning, and API integration. You'll need access to relevant data sources and infrastructure to facilitate training and deployment. Collaborating with domain experts and data scientists can be beneficial. It's essential to evaluate the resources, time, and skills required for successful implementation. Let me know if you'd like more details!
I'm curious about the ethical considerations when using Gemini for commodity risk management. How can we ensure fair and unbiased outcomes?
Ethical considerations are crucial, Liam. Bias can emerge from training data or the model's own biases. It's important to carefully curate and evaluate training data for fairness and inclusivity. Regularly monitoring for bias during model operation is also recommended. Employing diverse teams during model development and considering external audits can further help mitigate biases. Transparency in model behavior and disclosure of limitations are essential for responsible implementation. Any other concerns you'd like to discuss?
How does Gemini handle complex financial terms and jargon commonly used in commodity risk management? Can it accurately interpret and generate meaningful insights based on such terms?
That's a valid concern, Sarah. Gemini can understand and generate text based on various financial terms and jargon used in commodity risk management. However, it's important to note that the model's responses are based on patterns and examples seen during training, which may not always capture the full complexity or subtleties of specific terms. Providing clear context and, if necessary, post-processing the model's output can help ensure accurate interpretation and meaningful insights. Let me know if you have any further questions!
This article brings up an interesting point. How can Gemini assist in managing risks associated with volatile technology commodity prices?
Thanks for your question, Daniel! Gemini can help analyze price trends, identify potential risk factors impacting technology commodity prices, and provide insights on risk mitigation strategies. By combining historical data and market information, it can assist in making informed decisions regarding volatile technology commodity prices. Are there any specific concerns or use cases you'd like to discuss?
I'm curious about the scalability of using Gemini for commodity risk management. Can it handle large volumes of data and provide timely responses?
Scalability is an important consideration, Sophie. While Gemini can handle large volumes of data, the processing time may vary depending on the complexity and size of the input. Generating responses may take a few seconds, which may not be suitable for real-time scenario. Additionally, the cost of API usage should be taken into account. It's crucial to assess the specific requirements of your application and determine the trade-offs between real-time responsiveness and computational resources. Let me know if there's anything else I can help you with!
What level of accuracy can we expect when using Gemini for commodity risk management? Are there any benchmarks or performance metrics?
Good question, Nathan! The accuracy of Gemini for commodity risk management depends on various factors, including the quality and diversity of the training data, model tuning, and the complexity of the problem at hand. It's difficult to provide precise benchmarks or performance metrics as it can vary based on the specific implementation. Thorough evaluation of the model's performance on relevant metrics like precision, recall, or profitability measures is recommended. Let me know if you have any more inquiries!
Gemini seems promising, but what are the potential limitations when dealing with rare or extreme events in commodity risk management?
Excellent question, Emily! Gemini's reliance on training data means it performs better on common scenarios rather than rare or extreme events. These events may not have enough representative data to generate accurate insights. Human expertise and external information sources are valuable in complementing Gemini's capabilities, especially when dealing with rare events. Rapid adaptation and fallback mechanisms must be in place to handle unpredictable situations. Feel free to ask for additional information!
Considering the potential benefits of Gemini, are there any known successful implementations of using it for commodity risk management?
Certainly, Lucas! While specific details may not be publicly available for all cases, there are successful implementations of using AI models like Gemini in conjunction with human expertise for commodity risk management. Financial institutions, commodity trading companies, and technology firms have explored utilizing such models to improve their risk management strategies. Regular monitoring, evaluation, and adaptation are key to ensure continued success. Let me know if you want more examples or have any further queries!
This article opens up exciting possibilities for commodity risk management, but what are the potential data privacy and security concerns when implementing Gemini?
Great point, Sophia! Data privacy and security are crucial considerations. When implementing Gemini, it's important to handle sensitive or proprietary data cautiously. Steps should be taken to ensure compliance with relevant regulations, secure data transmission, and protect user confidentiality. Proper anonymization or aggregation techniques can be applied to maintain privacy. Regular risk assessments and updates to security protocols also help mitigate potential risks. Do you have any other concerns or questions on this topic?
I'm curious about the timeframe for training Gemini for commodity risk management. How long does it typically take, and is it an ongoing process?
Good question, Max! Training Gemini for commodity risk management can take time, depending on the size of the training data and the computational resources available. Training durations range from days to weeks, with larger models requiring more time. Additionally, it's an ongoing process as new data needs to be continuously incorporated to keep the model up-to-date. Regular fine-tuning and performance monitoring are necessary. Let me know if you'd like more details!
I'm intrigued by the potential of Gemini for commodity risk management. Can it assist in identifying opportunities in addition to managing risks?
Absolutely, Alice! Gemini can analyze market trends, which can help identify potential opportunities in addition to managing risks. By providing insights into commodity price movements, supply chain dynamics, or demand patterns, it can assist in making informed decisions about entering new markets, optimizing trading strategies, or leveraging emerging opportunities. It's a versatile tool for both risk management and exploring growth prospects. Let me know if you have any more thoughts or questions!
I'm curious to know how Gemini can handle non-financial factors that impact commodity risk management, such as climate change or regulatory changes. Can it incorporate such factors into its analysis?
Good question, Samuel! Gemini can handle non-financial factors by incorporating relevant data that reflects climate change impacts, regulatory changes, or other external influences. By training the model with diverse and comprehensive datasets, it can capture patterns and correlations to provide insights into how these factors can affect commodity risk management strategies. Combining domain expertise with model assistance is crucial for accurate interpretation. Let me know if you need further information!
What are the potential use cases for Gemini in commodity risk management? Can it be tailored for specific industries or sectors?
Great question, Emma! Gemini's flexibility allows for various use cases in commodity risk management across different industries and sectors. It can be tailored to specific domains by training it on relevant datasets and incorporating industry-specific risk factors. Examples include agriculture, energy, technology, and metals industries, among others. By capturing domain expertise and sector-specific trends, Gemini can provide valuable insights for risk management in different commodity markets. Feel free to discuss specific industry scenarios if you'd like!
How does Gemini handle multi-commodity risk management? Can it analyze interdependencies between different commodities and potential spillover effects?
Multi-commodity risk management is an interesting aspect, Noah. Gemini can handle it by training on datasets that include interdependencies between different commodities and associated spillover effects. By capturing correlations and patterns across multiple commodities, it can help assess how price movements or risk factors in one commodity can impact others. However, it's essential to ensure input data represents these interdependencies accurately to avoid potential biases or inaccuracies. Let me know if there's anything else you'd like to know!
This article highlights the potential of AI in enhancing commodity risk management. Are there any regulatory considerations or challenges that businesses should be aware of when using Gemini?
Regulatory considerations are important, Peter. Businesses should ensure compliance with relevant regulations, especially regarding data privacy, financial services, and market manipulation. Transparent and explainable AI models, like Gemini, can aid in meeting regulatory requirements. It's prudent to assess the legal and operational impact of implementing AI in commodity risk management and involve legal and compliance teams in the decision-making process. Let me know if you have any specific concerns!
I'm interested in the integration of Gemini with existing risk management systems. How can businesses ensure seamless integration and minimize disruptions?
Seamless integration is crucial, Adam. To integrate Gemini with existing risk management systems, businesses should ensure compatibility and data interoperability. APIs or custom integration can be used to connect Gemini with other systems. Conducting thorough testing and validating outputs against existing processes can help minimize disruptions. It's recommended to gradually introduce Gemini while maintaining fallback mechanisms and gradually expanding its role based on performance. Let me know if you need further guidance!
What are the potential trade-offs in using Gemini for commodity risk management compared to traditional risk management approaches?
Excellent question, Harry! Gemini offers benefits but also trade-offs. Its ability to analyze large volumes of data and provide quick insights can be advantageous. However, it has limitations when it comes to handling rare events, biases, or dealing with real-time data. Traditional risk management approaches often involve expert judgment, experience, and domain-specific insights that Gemini may not fully capture. Combining AI assistance with human expertise helps strike a balance and harness the advantages of both approaches. Let me know if you'd like to discuss further!
Can Gemini be trained on proprietary company data for more accurate and tailored risk management insights?
Certainly, Luke! Gemini can be trained on proprietary company data to provide more accurate and tailored risk management insights. Using internal datasets can make the model more contextually aware of your company's specific risk factors, market dynamics, or operational considerations. However, it's important to ensure proper anonymization and take data privacy and security into account. Collaborating with data scientists and domain experts during the training process can further enhance the model's performance. Any other questions on this topic?
What considerations should be made when implementing Gemini for commodity risk management in organizations with different levels of AI maturity?
Implementing Gemini for commodity risk management should consider an organization's AI maturity, Maria. For organizations new to AI, it's essential to build a solid understanding of AI concepts, establish appropriate infrastructure, and identify suitable use cases. Collaboration with external experts or consultants can help bridge knowledge gaps. Organizations more advanced in AI can focus on data quality, model evaluation, and integration with existing systems. It's a journey that requires continuous learning and adaptation. Feel free to discuss organization-specific scenarios if you'd like!