Enhancing Confocal Microscopy with Gemini: Unleashing the Power of AI in Imaging Analysis
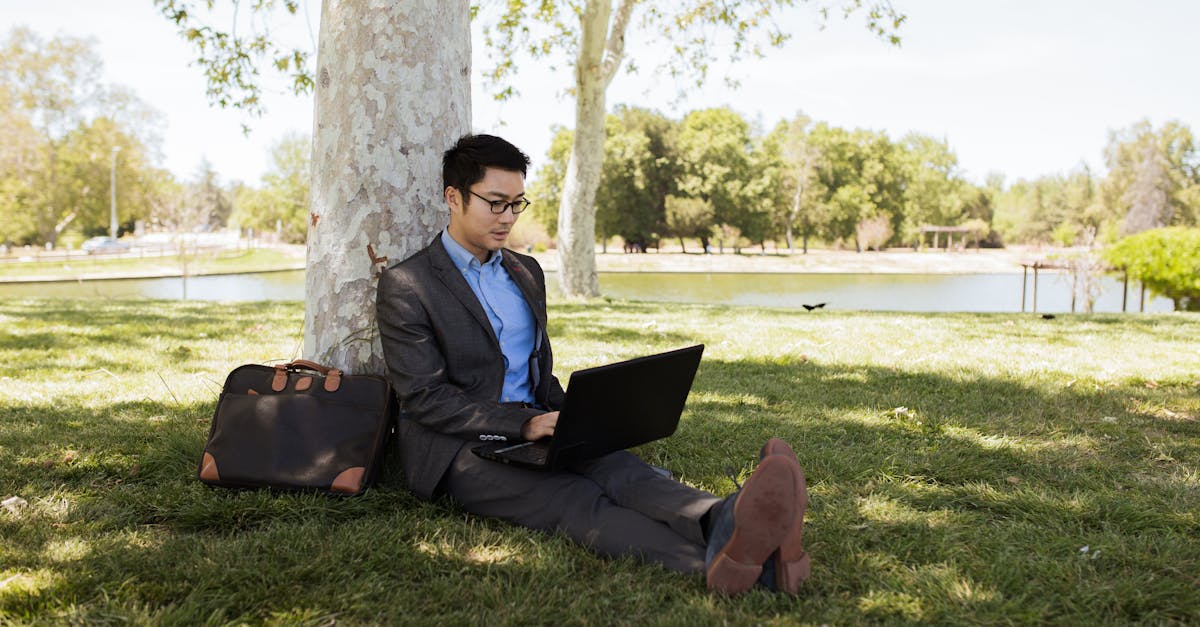
Introduction
Confocal microscopy has been a vital tool in biomedical research, allowing scientists to obtain high-resolution images of biological samples. It enables visualization of cellular structures and functions, aiding in various fields such as cell biology, neurobiology, and pathology. However, the analysis and interpretation of the extensive data generated by confocal microscopy can be a time-consuming and challenging task.
The Power of AI in Imaging Analysis
Artificial Intelligence (AI) has revolutionized various industries, and its application in biomedical imaging analysis holds great potential. One of the leading AI models, Gemini, has proven to be a promising tool for enhancing confocal microscopy analysis. Gemini, based on Google's LLM architecture, utilizes deep learning techniques to understand and generate human-like responses.
By leveraging the power of Gemini, researchers can streamline the image analysis process, gaining valuable insights from confocal microscopy data. The AI model can assist in identifying cellular structures, quantifying fluorescent signals, and even analyzing complex interactions within cells.
Benefits of Using Gemini for Confocal Microscopy
1. Automated Analysis: Gemini can automate time-consuming tasks such as cell counting, intensity measurements, and feature detection. This allows researchers to focus more on data interpretation and hypothesis generation.
2. Increased Efficiency: The integration of Gemini with confocal microscopy software enables real-time analysis, accelerating the overall research process. It reduces the need for manual interventions, saving both time and effort.
3. Improved Accuracy: AI-powered analysis reduces the risk of human errors and enables more precise measurements. Gemini can handle complex datasets and provide consistent results, ensuring reliable analysis.
Utilizing Gemini in Confocal Microscopy
The integration of Gemini with confocal microscopy platforms can be achieved through custom software plugins or APIs. Researchers can develop user-friendly interfaces that allow seamless interaction with the AI model. This empowers scientists with a powerful tool that assists in navigating and analyzing the complex confocal microscopy data.
Additionally, Gemini can be trained with large datasets specific to different biological applications. Training the model using labeled data enhances its understanding of various cell types, structures, and abnormalities, further improving its analysis capabilities.
Future Implications
The integration of AI, specifically Gemini, in confocal microscopy analysis is just the beginning. As the field of AI continues to advance, so will its potential in transforming the biomedical imaging landscape. The combination of AI-driven analysis and imaging techniques presents unprecedented opportunities for accelerating scientific discoveries and developing novel diagnostics in various medical fields.
Conclusion
The collaboration between confocal microscopy and Gemini AI technology is a significant milestone in biomedical imaging analysis. By leveraging the power of AI, researchers are able to enhance their abilities to analyze complex confocal microscopy data, leading to more efficient and accurate results. The future implications of this integration are promising, as AI continues to unlock new possibilities in the realm of biomedical research and diagnostics.
Comments:
Thank you all for your valuable feedback on my article. I'm glad to see so much interest in using AI to enhance confocal microscopy!
This is a fascinating article, Daniel. AI has the potential to revolutionize imaging analysis, and it's exciting to see it being applied to confocal microscopy.
I couldn't agree more, Alice. It's amazing how AI can help us extract meaningful insights from complex microscopy data.
I have some experience with confocal microscopy, and I must say that the integration of AI holds great promise in improving image quality and analysis speed. Great work, Daniel!
I'm curious about the specific AI techniques used in this context. Can you provide some details, Daniel?
Certainly, Erik! The article focuses on using Gemini, a language model, in conjunction with confocal microscopy. It helps automate image analysis, annotation, and even assists in real-time decision-making during imaging experiments.
AI can definitely help us handle the ever-increasing image volumes generated by modern microscopes. Daniel, do you think it's applicable to all types of microscopy?
That's a great question, Grace. While AI can provide benefits in various microscopy techniques, the applicability may depend on specific requirements and image characteristics. Gemini can be more effective with confocal microscopy due to its 3D imaging capabilities and resulting data complexity.
I'm impressed by the potential of AI in confocal microscopy, but how do we ensure the accuracy of AI-driven analysis?
Valid point, Frank. AI algorithms need to be trained on validated and diverse datasets to ensure accuracy. Additionally, it's crucial to have a feedback loop where experts can validate and refine the results. Human expertise remains invaluable in this process.
Are there any limitations to using AI in confocal microscopy? Daniel, I'd love to hear your thoughts.
Great question, Isabella. While AI offers tremendous potential, there are still challenges in handling image artifacts, variability in staining techniques, and rare cell type identification. However, with continuous improvement and expert oversight, we can mitigate these limitations.
I wonder how the integration of AI impacts the learning curve for researchers using confocal microscopy.
An excellent point, Jacob. The integration of AI aims to augment researchers' capabilities, reducing the learning curve by automating certain tasks and providing assistance. It allows researchers to focus more on data interpretation and hypothesis-driven analysis.
Daniel, have there been any practical implementations of AI in confocal microscopy so far?
Indeed, Karen. AI has been successfully applied in various areas, such as automated cell counting, object segmentation, and feature extraction from confocal images. It has also aided in understanding complex cellular interactions and dynamics within tissues.
I can see how AI could save a lot of time in analyzing confocal microscopy images. The article mentions Gemini assisting in real-time decision-making during imaging experiments. Can you explain more about that, Daniel?
Certainly, Laura. In real-time decision-making, Gemini can help researchers choose specific imaging parameters, suggest focus adjustments, or assist in image recognition and annotation while the experiment is ongoing. It optimizes the imaging process and reduces human intervention.
That sounds incredibly useful, Daniel. I can imagine how it would enhance research efficiency and reduce errors.
What are some other potential applications of AI in microscopy beyond confocal imaging?
Great question, Nathan. AI can be applied to various microscopy methods, such as wide-field microscopy, super-resolution microscopy, and even electron microscopy. It can aid in image enhancement, denoising, image restoration, and automated feature extraction across different imaging modalities.
Daniel, do you think there will ever be a point where AI can completely replace human analysis in microscopy?
That's an interesting question, Olivia. While AI can automate certain tasks and improve analysis efficiency, it is unlikely to replace human analysis entirely. Human expertise, critical thinking, and contextual understanding are still indispensable in interpreting complex microscopy data accurately.
Will the integration of AI into confocal microscopy introduce any ethical considerations, Daniel?
Ethical considerations are indeed crucial, Patrick. The use of AI should be transparent, respecting data privacy, and avoiding biases. Proper validation and oversight must be in place to ensure the responsible and ethical use of AI technologies in microscopy research.
Daniel, what are some challenges you faced while integrating Gemini with confocal microscopy?
Good question, Quentin. One of the challenges was training the language model with a diverse set of microscopy-related data to handle specific queries and nuances. Another challenge was fine-tuning the model to adapt to the dynamic nature of real-time decision-making in microscopy experiments.
Would you recommend researchers adopt AI-based solutions in their confocal microscopy workflow, Daniel?
Certainly, Rachel. AI-based solutions can significantly enhance the efficiency and accuracy of analysis in confocal microscopy. Researchers should consider integrating AI where appropriate, while also maintaining a balance by leveraging their expertise in the interpretation of complex data.
The combination of AI and confocal microscopy sounds exciting. Daniel, what trends do you foresee in this field in the coming years?
Great question, Samuel. In the future, we can expect more sophisticated AI algorithms tailored to the unique challenges of confocal microscopy. We may also see increased integration of AI with other imaging techniques, leading to multimodal analysis and deeper insights into cellular dynamics.
I look forward to witnessing the advancements in this field. Thank you for sharing your expertise with us, Daniel!
As an AI enthusiast, I'm thrilled to see AI being leveraged in scientific research areas like microscopy. It opens up new possibilities!
Indeed, Sarah! The marriage of AI and microscopy has immense potential, empowering researchers to extract valuable insights and further our understanding of biological systems.
I'm wondering if AI integration will require significant computational resources. What are your thoughts, Daniel?
Good question, Thomas. While AI does require computational resources for training and inference, advancements in hardware and optimization techniques have made it more accessible. With proper infrastructure and platform support, the computational requirements can be manageable.
In terms of accessibility, Daniel, do you think the AI integration will be limited to well-funded labs or can it be adopted by smaller research institutions too?
Excellent question, Ursula. While resource availability can play a role, the increasing availability of open-source AI frameworks and cloud-based computing platforms make it feasible for smaller research institutions as well. Collaboration and knowledge-sharing can further democratize the adoption of AI in microscopy.
Are there any specific regulatory guidelines or standards for utilizing AI in microscopy, Daniel?
Regulatory guidelines for AI in microscopy are still evolving, Violet. However, existing guidelines related to data privacy, scientific ethics, and responsible AI apply. It's essential for the scientific community to actively participate in establishing standards that promote the ethical use of AI technologies.
Thank you all for reading my article! I'm excited to join this discussion.
This article is really interesting. AI has so much potential in various fields, including microscopy. Looking forward to seeing more advancements!
Glad you found it interesting, Sarah! AI indeed has the potential to revolutionize imaging analysis by making it faster and more accurate.
I wonder what specific applications this enhanced confocal microscopy can have? Can someone provide examples?
Great question, Michael! Enhanced confocal microscopy with AI can be beneficial in studying cellular structures, tumor analysis, drug discovery, neuroscience research, and more. Its ability to analyze large volumes of data quickly can assist in finding patterns and anomalies.
I'm amazed by the potential of AI in assisting researchers. It can save so much time and effort!
Absolutely, Sophia! AI can automate repetitive tasks, allowing researchers to focus on more complex analysis and interpretation. It's a game-changer.
I'm curious about the limitations of this AI-powered microscopy. Are there any challenges or drawbacks?
Good question, Mark! While AI can enhance microscopy analysis, there are still challenges. One limitation is the need for large annotated datasets to train AI models effectively. Additionally, there are ethical considerations in data privacy and potential biases in AI algorithms.
The potential bias in AI algorithms is a critical concern. We need to ensure the algorithms are trained on diverse datasets to avoid discriminatory outcomes.
Absolutely, Emily! It's crucial to address biases by training AI models on diverse datasets and continuously monitoring their performance to minimize discriminatory outcomes.
I'm curious if this AI-powered analysis can be used on other imaging techniques apart from confocal microscopy?
Good question, Oliver! While this article focuses on confocal microscopy, AI-powered analysis can be applied to other imaging techniques like fluorescence microscopy, electron microscopy, and even medical imaging modalities like MRI or CT scan analysis.
I'm concerned about the potential job losses for researchers if AI takes over microscopy analysis completely.
Valid concern, Jennifer. While AI can automate certain tasks, it's important to note that researchers will still play a crucial role in experimental design, result interpretation, and guiding AI algorithms. AI-enhanced microscopy will augment and improve their work, not replace them.
The integration of AI in imaging analysis is indeed promising, but we should ensure that human expertise is not overshadowed by relying solely on AI predictions.
Well said, Robert! Human expertise is invaluable and should complement the power of AI. Interpretation of results and critical thinking will always require human involvement.
Could AI analysis help in identifying new rare cell populations or rare events? That would be game-changing for certain studies!
Absolutely, Kelly! AI algorithms can detect and analyze rare cell populations or events more efficiently than manual analysis, potentially uncovering new insights in various studies.
I'm concerned about the ethical implications of using AI in imaging analysis, especially in medical and biological research.
Ethics is indeed an important aspect, Sophia. As with any AI application, data privacy, algorithmic bias, and transparency should be carefully addressed. Collaborative efforts among scientists, ethicists, and policymakers are crucial to ensure responsible implementation and prevent misuse.
I'm impressed with the advancements in microscopy analysis. It's incredible how AI is transforming various scientific disciplines.
Indeed, Jacob! AI opens up possibilities for accelerating discoveries and gaining deeper insights across scientific disciplines. It's an exciting time for research and innovation.
Are there any limitations to the accuracy of AI algorithms in microscopy analysis?
Good question, Lisa! AI algorithms can achieve high accuracy, but it depends on the availability of well-curated training data and the quality of algorithm development. Continuous refinement and validation are important to ensure reliable results.
The potential integration of AI and microscopy may also lead to breakthroughs in personalized medicine.
Absolutely, Matthew! AI-powered microscopy can aid in personalized medicine by analyzing individual patient samples more efficiently, allowing for tailored treatment approaches and improving patient outcomes.
Can this AI-powered analysis be used in other scientific fields as well, like astrophysics or geology?
Interesting question, Ava! While the focus of this article is on microscopy analysis, AI has applications in various scientific fields. In astrophysics, it aids in analyzing large volumes of data from telescopes, and in geology, it can assist in identifying patterns in geological samples.
I'm curious about the computational requirements for running AI-powered microscopy analysis. Does it require significant computing power?
Good question, Emma! AI-powered microscopy analysis can be computationally demanding, especially when dealing with large datasets. High-performance computing resources and optimized algorithms are required to achieve efficient processing.
What are some of the popular AI techniques used in microscopy analysis?
Great question, Mike! Popular AI techniques for microscopy analysis include deep learning algorithms, convolutional neural networks (CNNs), and unsupervised learning methods like clustering and dimensionality reduction techniques.
As an aspiring researcher, I'm excited about the potential of AI-powered microscopy. It opens up new avenues for exploration and analysis.
That's wonderful to hear, Amy! AI can definitely enhance your research journey by providing powerful tools for analysis and aiding in discoveries. Best of luck with your future endeavors!
AI will definitely reshape the way we approach scientific research. Exciting times lie ahead!
Absolutely, Sophia! Embracing AI in scientific research will undoubtedly lead to exciting advancements and accelerate progress across various domains.
Thank you, Daniel, for sharing your insights on AI-powered microscopy. It's been an enlightening discussion!
You're most welcome, Michael! I'm glad you found the discussion enlightening. Thank you all for your valuable participation!
Indeed, thank you, Daniel, and everyone else for the insightful conversation!