Enhancing Content Moderation with ChatGPT: A Breakthrough in Neural Networks Technology
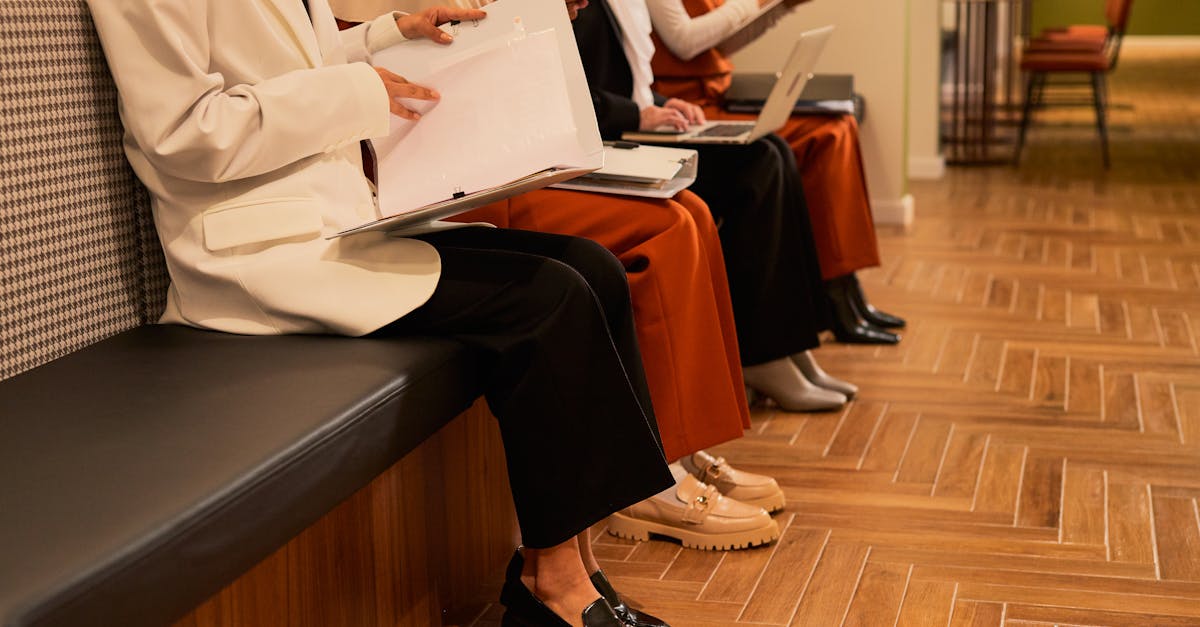
Neural networks have emerged as a powerful technology for various applications, and one such area is content moderation. With the exponential growth of user-generated content across different platforms on the internet, the need for efficient and automatic content moderation has become increasingly important.
Content moderation refers to the process of monitoring and reviewing user-generated content to ensure it complies with community guidelines, policies, and legal regulations. Inappropriate content such as hate speech, nudity, violence, or spam can have a negative impact on online communities and brand reputation. Traditional methods of content moderation often rely on manual review, which can be time-consuming, costly, and susceptible to human bias.
Neural networks offer a promising solution to automate the content moderation process. They can be trained to recognize patterns and features in large datasets, enabling them to accurately identify inappropriate content across different languages. By leveraging machine learning algorithms, neural networks can learn from labeled training data to classify content as safe or unsafe, allowing platforms to proactively moderate content before it becomes visible to a wider audience.
One of the key advantages of neural networks in content moderation is their ability to handle multilingual content. With the internet connecting people from different parts of the world, platforms need to address inappropriate content in various languages. Neural networks can be trained on multilingual datasets, enabling them to detect and moderate inappropriate content regardless of the language used. This level of flexibility is crucial for platforms operating globally.
The usage of neural networks for content moderation extends beyond text-based content. They can also be trained to recognize inappropriate images or videos, making them versatile in tackling a broader range of content moderation challenges. By analyzing visual content, neural networks can identify explicit or violent imagery, adult content, or other types of content that violate platform guidelines.
Implementing neural networks for content moderation does come with its challenges. The training process requires a large amount of labeled data, which can be time-consuming and costly to acquire. Additionally, neural networks are not perfect and can occasionally make mistakes, leading to false positives or false negatives. Therefore, it is crucial to continuously evaluate and refine the models to improve their accuracy and minimize the risk of incorrectly moderating content.
In conclusion, neural networks offer a powerful and scalable solution for automatic content moderation in today's digital landscape. With their ability to handle multilingual content and recognize inappropriate text, images, and videos, neural networks can assist platforms in maintaining a safe and engaging online environment. While there are challenges in implementing and fine-tuning these models, the potential benefits in terms of efficiency, accuracy, and consistency make neural networks an indispensable tool for content moderation.
Comments:
Great article! Content moderation is such an important aspect of online platforms.
Indeed, Adam! With the increasing amount of user-generated content, better content moderation is crucial.
I completely agree! How does ChatGPT aim to enhance content moderation specifically?
Thank you all for your comments! Mark, ChatGPT is designed to analyze and understand text-based conversations to improve content moderation processes.
I'm curious, does ChatGPT have any specific techniques to identify and filter harmful content?
That's a good question, Emily. I believe ChatGPT uses a combination of keyword filtering, context analysis, and machine learning algorithms to detect potentially harmful content.
I hope ChatGPT can successfully filter out hate speech and offensive comments. It's a growing problem on many platforms.
Absolutely, Linda. Ensuring a safe and inclusive online environment is crucial.
Content moderation is a challenging task. How accurate is ChatGPT in identifying problematic content?
Good point, Grace. The accuracy of AI systems like ChatGPT is an ongoing challenge, but continuous learning and feedback can help improve it.
I'm a content moderator, and I'm always looking for better tools. Can ChatGPT automate the moderation process effectively?
Hi Mike, while ChatGPT can assist in the moderation process, it's essential to combine it with human moderation to ensure accuracy and fairness.
Do you think AI moderation like ChatGPT could potentially lead to censorship or over-moderation?
I have the same concern, Amy. Striking the right balance is crucial to avoid stifling free speech.
I agree, Linda. It's important to ensure that AI moderation is transparent and respects diverse viewpoints.
ChatGPT seems like a step in the right direction, but we need ongoing research and development to tackle the evolving challenges of content moderation.
Absolutely, Mark. Collaborative efforts between researchers, technology experts, and content moderators are key.
AI-based moderation holds great potential, but we should always be cautious about unintended biases and ethical considerations.
I hope developers continue to prioritize user safety and privacy while implementing AI moderation systems like ChatGPT.
Thanks for the insightful discussion, everyone! It's essential to keep exploring innovative solutions to improve content moderation.
Thank you all for your valuable comments and perspectives. It's heartening to see the interest in advancing content moderation for a safer online environment.
Thank you, Breaux Peters, for writing this informative article and engaging with us.
As an AI enthusiast, the potential of ChatGPT in enhancing content moderation is exciting.
I'm glad to see organizations investing in advanced technologies like ChatGPT to combat online abuse.
This article showcases the potential of AI in solving real-world challenges. Kudos to the researchers behind ChatGPT.
The continuous improvement of content moderation technologies is crucial for a safer digital space.
AI tools like ChatGPT can save a lot of time and effort for content moderators, enabling them to focus on more complex cases.
I'm excited to see how AI evolves in the field of content moderation in the coming years.
The use of natural language processing in ChatGPT seems promising for identifying and handling user-generated content.
The responsibility still lies with platform owners to ensure AI moderation is fair, effective, and implemented responsibly.
Content moderation is a multi-faceted challenge, and it's encouraging to see advancements like ChatGPT.
I appreciate the efforts of developers who work on tech solutions that aid content moderation.
ChatGPT's ability to understand text-based conversations can have positive implications for improving online interactions.
I hope ChatGPT can assist in detecting deceptive or fake content as well.
Consistently adapting and fine-tuning ChatGPT will be crucial to ensure it keeps up with evolving moderation needs.
The integration of AI-based content moderation systems should be accompanied by clear guidelines and community feedback loops.
I hope ChatGPT can effectively handle regional and cultural nuances to avoid undue censorship.
Ensuring accountability and transparency in AI moderation is vital to build users' trust.
AI moderation is a complex field, and it's exciting to see advancements like ChatGPT that push the boundaries.
I agree, Jason. It's an ongoing journey where collaboration and continuous improvement are paramount.
This article has shed light on the efforts towards building safer online spaces. Keep up the good work!
ChatGPT's potential to automate some moderation tasks can help alleviate the workload of human moderators and improve overall efficiency.
It's fascinating to see how AI is being applied to address the challenges of content moderation.
AI moderation tools like ChatGPT can complement the efforts of human moderators and create safer digital spaces.
As technology continues to evolve, it's important to stay vigilant and address the new risks and challenges it brings.
I appreciate the discussion around the potential benefits and caveats of AI content moderation.
Thank you all for participating! Let's continue to explore innovative ways to ensure a safer online community.
Thank you once again for your engagement, everyone. Your insights are valuable.
This article has given me a better understanding of the advancements in content moderation. Thank you, Breaux Peters.
It's heartening to see the collective commitment to building a safer and more inclusive online world.