Enhancing Core Data Forecasting with ChatGPT: Unlocking the Potential of Conversational AI
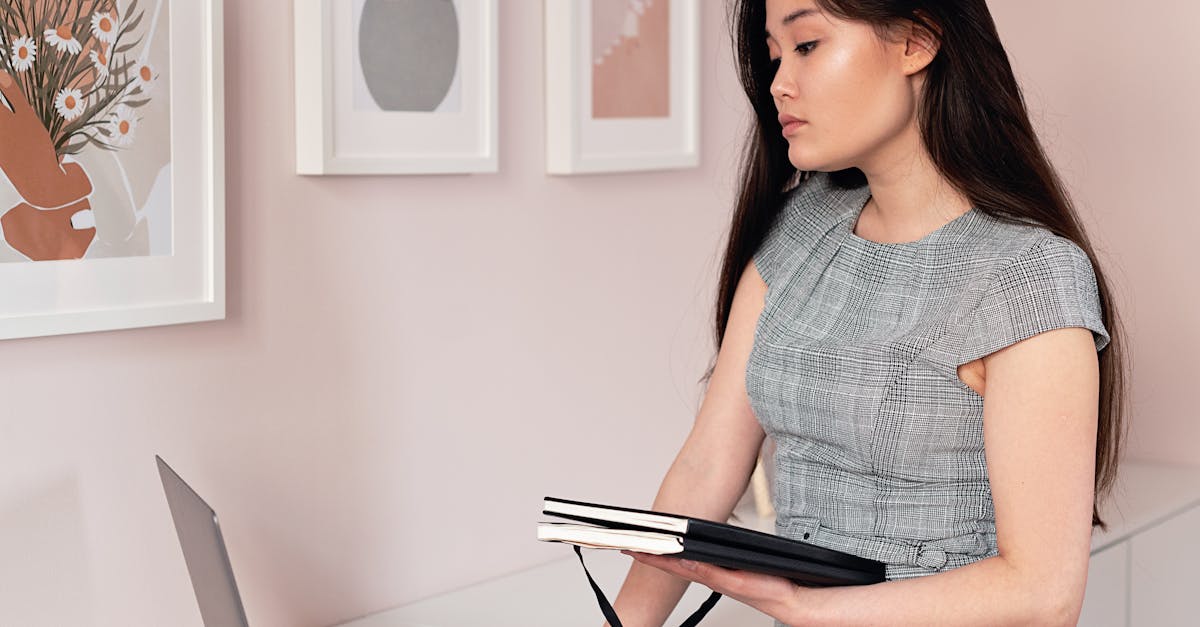
Core Data is a powerful technology that can be utilized for forecasting purposes. By leveraging historical data, ChatGPT-4, an advanced conversational AI model, can predict future events or trends accurately. This capability opens up a wide range of possibilities for businesses, researchers, and individuals who seek valuable insights and predictions for decision-making and understanding future trends.
Understanding Core Data
Core Data is a framework provided by Apple for managing the model layer objects in an application. It acts as an interface between the application's data and the underlying storage mechanism. While Core Data is primarily used for persisting data in iOS and macOS applications, its capabilities extend beyond storage. It includes a robust set of features for data manipulation and querying, making it a suitable tool for forecasting tasks.
Forecasting with ChatGPT-4
ChatGPT-4 is an advanced language model developed by OpenAI. It excels in generating human-like responses, understanding context, and providing meaningful insights through conversational interactions. By combining the power of Core Data with ChatGPT-4, we can create a forecasting model that utilizes historical data to predict future events or trends accurately.
The process typically involves training the ChatGPT-4 model with a dataset containing historical data. This dataset could be any relevant information that captures the past events or trends related to the area of interest. For example, it could be financial market data, customer behavior records, or climate patterns, depending on the forecasting requirements.
Once the model is trained on the historical data, it can make predictions about future events or trends based on new input. By inputting the current state or relevant parameters, the model uses its understanding of the historical data to generate a forecast. The predictions can be probabilistic, providing an estimate of the likelihood of different outcomes, or deterministic, offering specific predictions based on the available information.
Potential Applications
The applications of using Core Data and ChatGPT-4 for forecasting are vast. Here are some examples:
- Financial Forecasting: Financial institutions can utilize historical market data to predict investment trends, asset prices, or market movements. This can aid in making informed decisions regarding portfolio management, risk assessment, and trading strategies.
- Customer Behavior Analysis: Businesses can leverage customer data to forecast consumer preferences, product demand, or market trends. This information can guide marketing campaigns, product development, and inventory management, leading to more effective decision-making and resource allocation.
- Climate Prediction: Climate scientists can employ historical weather data to generate forecasts for temperature changes, rainfall patterns, or extreme weather events. These predictions can contribute to disaster preparedness, environmental planning, and sustainable resource management efforts.
- Supply Chain Optimization: Forecasting future demand based on historical sales data can enable businesses to optimize inventory levels, streamline logistics operations, and minimize supply chain disruptions.
Conclusion
Core Data, in combination with ChatGPT-4, offers a powerful solution for forecasting future events or trends. By leveraging historical data and utilizing the capabilities of ChatGPT-4, accurate predictions can be made to aid decision-making and planning in various domains. The applications of this technology extend across different sectors, from finance to climate science, revolutionizing how organizations and individuals anticipate and navigate the future.
Comments:
Thank you all for taking the time to read my article on enhancing core data forecasting with ChatGPT. I would love to hear your thoughts and insights!
Great article, Arthur! I really enjoyed how you highlighted the potential of conversational AI in improving core data forecasting. It has opened up new possibilities for my organization.
Thank you, Samantha! I'm glad you found it helpful. Conversational AI indeed has the power to revolutionize how we approach data forecasting.
I have some concerns about using ChatGPT for core data forecasting. How reliable is it in accurately predicting complex data patterns?
That's a valid concern, David. While ChatGPT can be incredibly useful, it does have limitations. It's essential to carefully evaluate its predictions and consider the specific dataset and problem domain.
I completely agree, Arthur. It's crucial to validate and verify the predictions made by ChatGPT. It should be used as a tool to support decision-making rather than being solely relied upon.
Absolutely, Linda! Validating the predictions against ground truth and leveraging human expertise is key to ensuring the accuracy and reliability of the forecasts.
I'm curious about the computational resources required for training and deploying ChatGPT. Can it be efficiently implemented in most organizations?
Good question, Robert! Training large-scale language models like ChatGPT can be computationally intensive. However, there are approaches and optimizations available to make it more feasible for deployment in various organizational setups.
I believe incorporating conversational AI in core data forecasting can greatly improve the accuracy and speed of predictions. It has immense potential to drive innovation in this field.
Well said, Gary! Conversational AI can enhance the forecasting process by facilitating a more interactive and intuitive experience, enabling analysts to uncover insights more effectively.
I'm curious about the training data required for ChatGPT. Can it handle various industry-specific domains or does it require specific data for each domain?
Good question, Emily! ChatGPT can be trained on diverse datasets, including industry-specific ones. However, providing relevant data for a specific domain can improve its performance and make it more tailored to the problem at hand.
This article highlights the immense potential of conversational AI for core data forecasting. It's exciting to see how technology is advancing in this area.
Indeed, Oliver! Technology advancements like ChatGPT are expanding the possibilities and capabilities of data forecasting. The future looks promising.
I wonder if ChatGPT can handle unstructured data effectively. Can it extract meaningful insights from textual data without prior formatting?
That's a great question, Sophia. ChatGPT can indeed handle unstructured data and extract insights. It excels at understanding and generating text, making it a valuable tool for working with various data formats.
While conversational AI can be beneficial, organizations must also consider the ethical aspects of deploying such technologies. How can we ensure responsible and unbiased use?
You raise an important point, Georgia. Ethical considerations are crucial when deploying conversational AI. Robust guidelines, oversight, and continuous monitoring can help ensure responsible and unbiased use.
I'm curious if ChatGPT can handle multilingual data effectively. Can it provide accurate forecasts using multiple languages?
Great question, Justin! ChatGPT can process and generate text in multiple languages, which makes it versatile for forecasting in various linguistic contexts.
Do you think the integration of conversational AI in core data forecasting will lead to a decrease in the need for human analysts?
Excellent question, Emma. While conversational AI can automate some aspects of the forecasting process, human analysts still play a crucial role in interpreting results, providing domain expertise, and ensuring the models are properly calibrated.
What are the major challenges in implementing conversational AI technologies like ChatGPT for core data forecasting?
Good question, Liam! Some significant challenges include properly training and fine-tuning the models, ensuring data privacy and security, handling bias, and carefully validating the predictions to avoid misleading or incorrect insights.
I find the concept of leveraging ChatGPT for core data forecasting intriguing. It has the potential to revolutionize how we approach and analyze data in our organization.
I'm glad you find it intriguing, Sarah! Leveraging conversational AI like ChatGPT can indeed bring new perspectives and efficiencies to the data analysis and forecasting process.
Has ChatGPT been extensively tested in real-world scenarios? I'm interested in its performance and reliability outside controlled environments.
Valid concern, Michael. While ChatGPT has shown impressive capabilities, testing and evaluating its performance in real-world scenarios is an ongoing area of research to ensure its reliability and effectiveness.
Are there any specific use cases you recommend for applying ChatGPT in core data forecasting? I'd love to explore its potential applications.
Great question, Evelyn! ChatGPT can be beneficial in various forecasting scenarios, such as demand forecasting, financial predictions, sales projections, and even anomaly detection in time series data.
Do you anticipate any regulatory challenges or restrictions associated with implementing conversational AI in core data forecasting?
Regulatory challenges are indeed a concern, Alexis. Organizations must navigate data protection, privacy laws, and ensure compliance when deploying conversational AI for core data forecasting.
The combination of conversational AI and core data forecasting can significantly improve decision-making and strategic planning. It's an exciting field to explore!
Absolutely, Hannah! The combination can unlock powerful insights and enable organizations to make data-driven decisions with more accuracy and confidence.
I appreciate the consideration you gave to the potential limitations of ChatGPT in the article. It's essential to have a balanced perspective on its capabilities and potential drawbacks.
Thank you, Daniel! It's important to acknowledge and understand the limitations of any technology to ensure its appropriate utilization and manage expectations.
I'm impressed by the potential of ChatGPT for enhancing core data forecasting. How can organizations get started with implementing it?
I'm glad you're impressed, Sophie! To get started with implementing ChatGPT or conversational AI in general, organizations can begin by identifying relevant use cases, gathering suitable data, and exploring the available frameworks and libraries for training and deployment.
This article provides valuable insights into the advantages and challenges of incorporating conversational AI into core data forecasting. Well-written and informative!
Thank you for the kind words, Nathan! I'm glad you found the article informative. Conversational AI can indeed bring numerous benefits to the field of data forecasting.
How can organizations address the potential biases within the training data and prevent them from affecting the accuracy of ChatGPT's forecasts?
Addressing biases within the training data is crucial, Elizabeth. Organizations can employ techniques like diverse dataset curation, careful data selection, and continuous monitoring to mitigate biases and improve the accuracy and fairness of ChatGPT's forecasts.
Conversational AI has the potential to enhance collaboration among data analysts and facilitate knowledge sharing. It can be a game-changer for organizations!
Absolutely, Lucas! Conversational AI can foster collaboration, allowing analysts to exchange insights, share knowledge, and collectively improve the quality of data forecasting.
I'm excited about the possibilities of integrating conversational AI into our core data forecasting process. It can streamline our workflows and empower analysts.
That's great to hear, Natalie! Integrating conversational AI can indeed bring efficiency and empower analysts to make accurate and timely forecasts.
ChatGPT seems like a powerful tool for core data forecasting. Are there any specific challenges associated with training and fine-tuning the model?
Training and fine-tuning language models like ChatGPT do come with challenges, Adam. Some common difficulties include selecting suitable hyperparameters, handling data biases, and optimizing the model's performance within available computational resources.
I'm interested in understanding the resource requirements for deploying ChatGPT in a production environment. Are there any guidelines or best practices available?
Resource requirements can vary based on the model size, deployment scale, and specific use case, Mark. OpenAI provides documentation and guidelines to help organizations optimize resource allocation and make informed decisions when deploying ChatGPT in production environments.