Enhancing Credit Analysis: Leveraging ChatGPT for Loan Covenant Monitoring
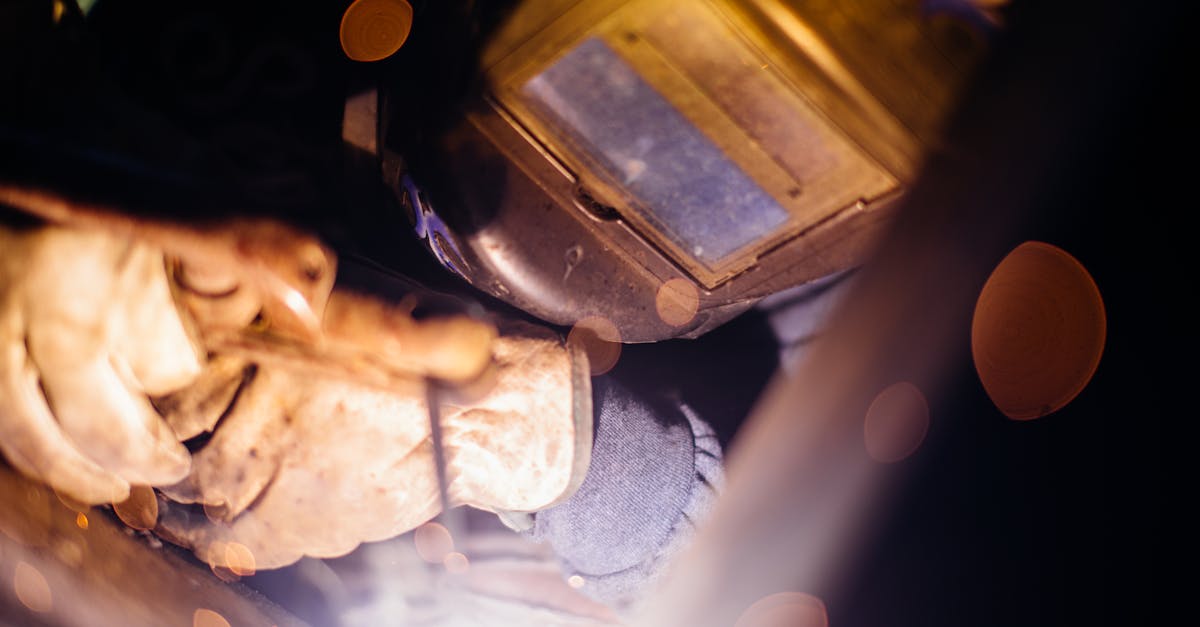
In the realm of credit analysis, monitoring loan covenants plays a vital role in ensuring the financial health and stability of lending institutions. Traditionally, this task required teams of financial analysts to manually review financial statements and performance metrics to identify potential covenant violations. However, with advancements in technology, specifically the emergence of ChatGPT-4, lenders can now leverage the power of artificial intelligence to streamline and enhance their loan covenant monitoring processes. ChatGPT-4, the latest version of the renowned language model, is designed to understand and generate human-like text responses. With its vast knowledge base and natural language processing capabilities, it can analyze financial statements and performance metrics to monitor loan covenants effectively. This technology can be a game-changer, as it not only saves time and resources but also improves the accuracy and efficiency of the monitoring process.
Loan covenants are conditions or restrictions imposed by lenders on borrowers to protect their interests and minimize risks. These covenants are included in loan agreements and are designed to ensure that borrowers maintain certain financial ratios, meet specific performance targets, or adhere to other agreed-upon conditions. Monitoring loan covenants enables lenders to proactively identify any potential breach and take remedial actions to mitigate risks. By utilizing ChatGPT-4, lenders can automate the monitoring process and receive alerts when loan covenants are at risk of being violated. This advanced technology can efficiently analyze vast amounts of financial data in real-time to detect any discrepancies or fluctuations that may put covenants at risk. Its ability to interpret complex financial information and identify patterns enables lenders to make more informed decisions and take timely actions to protect their investments.
When loan covenants are at risk of being breached, ChatGPT-4 can provide lenders with suggested remedial actions. It can generate recommendations based on financial analysis, industry best practices, and historical data. These suggestions empower lenders to navigate potential covenant violations proactively, helping them minimize losses and avoid costly legal disputes. With its AI-driven insights, ChatGPT-4 offers lenders valuable guidance on how to address potential breaches and maintain a healthy borrower-lender relationship.
Implementing ChatGPT-4 for loan covenant monitoring comes with several benefits. Firstly, it significantly reduces the manual workload for financial analysts, allowing them to focus on more strategic tasks. Secondly, it increases monitoring accuracy by eliminating human errors and biases. Additionally, ChatGPT-4's real-time monitoring capabilities facilitate prompt identification and response to covenant-related risks. By leveraging AI technology, lenders can strengthen their risk management practices and maintain healthy loan portfolios.
In conclusion, the development and implementation of ChatGPT-4 have brought a transformative change to the credit analysis field, especially regarding loan covenant monitoring. Through its ability to analyze financial statements and performance metrics, ChatGPT-4 helps lenders identify potential covenant violations and provides suggestions for remedial actions. By automating the monitoring process, it saves time, reduces human errors, and enhances the efficiency and accuracy of credit analysis. With ChatGPT-4 as a trusted ally, lenders can better safeguard their investments and maintain healthy lender-borrower relationships in the ever-evolving financial landscape.
Comments:
Great article, Alex! Leveraging AI for credit analysis seems like a game-changer. It can certainly enhance the accuracy and efficiency of loan covenant monitoring.
I agree, Emily. ChatGPT has the potential to revolutionize the credit analysis process. It can assist in identifying potential risks and deviations from loan covenants in real-time.
Thank you both for your comments! I'm glad you see the potential in leveraging AI for credit analysis. It can indeed provide valuable insights and help identify hidden patterns.
While AI can supplement credit analysis, it's crucial to ensure its algorithms are reliable and well-trained. Trusting AI blindly without proper context and human judgment may lead to errors.
That's a valid concern, Sophia. AI should be used as a tool to support decision-making rather than replacing human expertise. Proper validation and continuous monitoring are essential.
I can see how AI can streamline the loan covenant monitoring process, but what happens if the underlying data used for analysis is inaccurate or incomplete? How can AI address that?
Good question, Oliver. AI algorithms are as reliable as the data they are trained on. Ensuring accurate and complete data input is crucial. AI can also be programmed to identify data quality issues.
I'm concerned about the potential bias in AI models. How can we ensure that loan covenant analysis using AI doesn't perpetuate discriminatory lending practices?
Valid point, Natalie. Bias in AI models is a significant concern. Careful consideration and ongoing evaluation are necessary to ensure fairness and eliminate discriminatory outcomes.
Do you think AI can completely replace credit analysts in the future, or will it always require human judgment and oversight?
AI can automate various tasks, but it cannot replace human judgment, especially in complex credit analysis. Human analysts provide critical context, expertise, and can interpret nuances better.
I agree, Sophia. AI should augment and support credit analysts, but human judgment, experience, and qualitative analysis will continue to be vital.
One concern I have is that rapid automation may lead to fewer job opportunities for credit analysts. How can we ensure a balance between technology and human employment?
That's a valid concern, David. While AI can streamline processes, upskilling credit analysts and transitioning their roles towards higher-value tasks can help maintain employment opportunities.
AI can also assist in data cleansing and normalization processes, helping to address the issue of inaccurate or incomplete data. It can learn patterns and flag potential data inconsistencies.
Absolutely, Sophia! Human expertise combined with AI's pattern recognition capabilities can significantly enhance the accuracy and reliability of loan covenant monitoring.
I'm curious about the scalability of AI for credit analysis. Will it be accessible to smaller financial institutions, or will it be dominated by large players?
Good question, Rachel. As AI technology progresses and becomes more affordable, it should become accessible to smaller institutions as well. However, initial adoption might be led by larger players.
Privacy and data security are critical in the financial industry. How can we ensure that AI-powered credit analysis systems maintain adequate safeguards to protect sensitive information?
You're right, Sophie. Robust data encryption, access controls, and compliance with privacy regulations are essential. AI systems should prioritize data security as a fundamental requirement.
Thank you all for your valuable comments and insights! It's great to see the discussion around the considerations and challenges of leveraging AI for credit analysis.
I'm impressed by the potential AI has to extract actionable insights from vast amounts of data quickly. It can make loan covenant monitoring more efficient and proactive.
Absolutely, Sophie. AI's ability to process large datasets and identify relevant patterns can significantly improve monitoring capabilities and early risk detection.
Is there a risk of overreliance on AI? How can we strike the right balance and avoid unintended consequences?
That's a valid concern, Jacob. It's crucial to maintain human oversight and reassess AI systems regularly. An ongoing evaluation can help identify and mitigate potential unintended consequences.
Additionally, establishing robust governance frameworks and incorporating feedback loops can help ensure that AI systems continue to align with desired outcomes.
What potential challenges do you think financial institutions may face when implementing AI for credit analysis?
One challenge could be integrating AI systems with existing infrastructure and legacy systems. Seamless data flow and system compatibility might require careful planning and investment.
Another challenge is the need for skilled AI talent within financial institutions. The recruitment and retention of AI experts can be competitive, especially during the initial stages of adoption.
That's true, David. Building a strong team with expertise in both finance and AI is crucial to overcome implementation challenges and ensure effective utilization of AI in credit analysis.
I'm curious about the possible limitations of AI in credit analysis. Are there any specific scenarios where AI may struggle to provide accurate insights?
AI may struggle when faced with unprecedented events or sudden market shifts that lack historical data. Human judgment and adaptability will be crucial in such situations.
How do you see the future development of AI in credit analysis? Any exciting possibilities or advancements on the horizon?
I believe explainable AI will be a significant focus. Enhancing AI systems' transparency and interpretability can help build trust and regulatory confidence in their applications.
I think continuous advancements in Natural Language Processing (NLP) can also improve AI's ability to analyze qualitative information and strengthen credit analysis processes.
Absolutely, Rachel. NLP progress can enhance AI's capability to extract insights from unstructured data like financial news, reports, or even social media, enabling more comprehensive analysis.
With the increasing availability of alternative data sources, such as IoT devices and user-generated content, AI can leverage these sources to gain valuable insights for credit analysis.
Definitely, Oliver. Incorporating alternative data can provide a more holistic view of borrowers and help improve creditworthiness assessments, especially for individuals or businesses with limited traditional credit history.
What safeguards are in place to ensure that AI models used for credit analysis remain up-to-date and accurate as customer profiles and economic conditions change over time?
Regular model retraining and continuous data updates are necessary to maintain accuracy. Monitoring and adapting models to reflect changing conditions should be an ongoing process.
Additionally, incorporating feedback loops and involving credit analysts in model refinement can help align AI insights with evolving customer profiles and market dynamics.
External audits and regulatory oversight play crucial roles as well. Regular audits can verify the accuracy and fairness of AI models, ensuring compliance with industry standards.
Thank you all for your thought-provoking comments and questions! It's been a pleasure discussing the potential of AI in credit analysis with such an engaged audience.
I believe AI can provide proactive alerts by identifying emerging risks and early warning signals, allowing financial institutions to be more proactive in managing credit risks.
That's an excellent point, Rachel. AI-powered systems can continuously monitor various data sources, leading to timely detection of potential loan covenant violations or credit deterioration.
Another challenge financial institutions may face is ensuring that AI models remain explainable and compliant with regulatory requirements, especially in highly regulated domains.
Agreed, Oliver. Model explainability is essential not only for regulatory requirements but also to build trust and ensure AI's decision-making aligns with ethical and legal standards.
To address the challenge of explainability, there is ongoing research in the field of Explainable AI (XAI) that aims to develop techniques to provide more transparent AI models.
That's an exciting area, David. Explainable AI can play a crucial role in financial services, where the reasons behind AI-driven decisions need to be understandable and justifiable.
In addition to data security, proper data governance practices should be implemented to ensure ethical use of data and prevent misuse or unauthorized access.