Enhancing Data Acquisition Technology with ChatGPT: A Breakthrough in Predictive Modelling
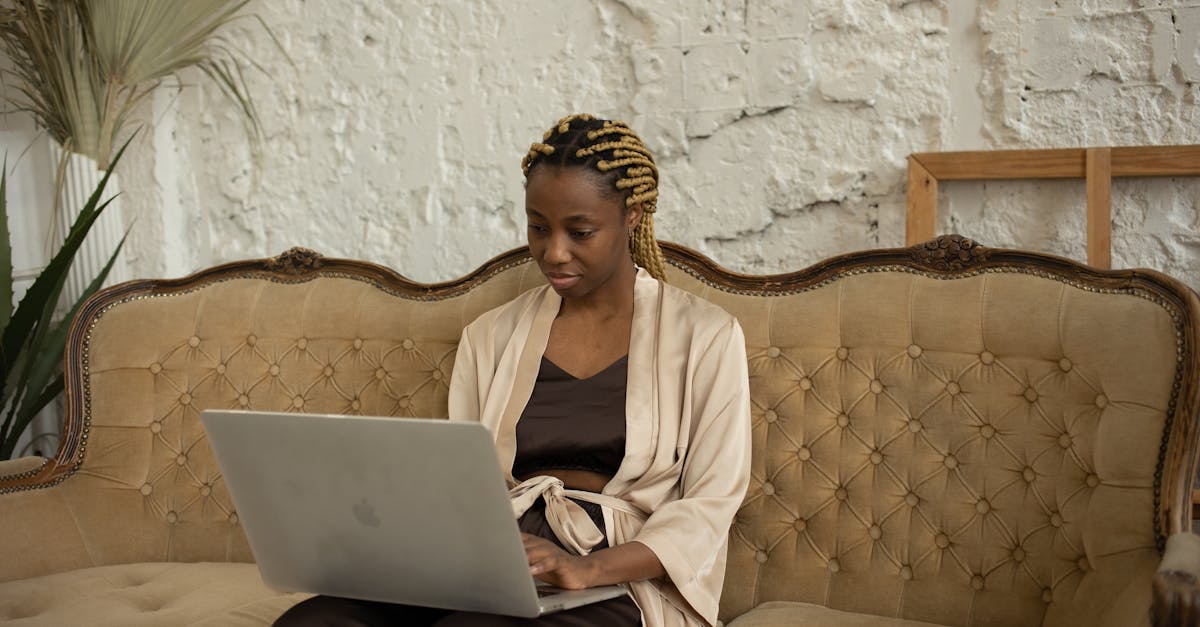
Data acquisition is a critical component in the field of predictive modelling. It involves the process of gathering and collecting relevant data from various sources to develop accurate models that can predict future outcomes based on historical data. In this article, we will explore the importance of data acquisition in predictive modelling and its various applications.
Technology Overview
Data acquisition in predictive modelling relies on advanced technologies and techniques to gather data efficiently. These technologies include sensors, devices, and software tools that can collect and store data from different sources such as databases, files, and real-time streams. The collected data is then processed, cleansed, and transformed into a suitable format for analysis.
Area of Application
Predictive modelling finds its application in various areas, such as finance, healthcare, marketing, and manufacturing, to name a few. By utilizing advanced algorithms and statistical techniques, predictive models can forecast future trends, identify potential risks, and guide decision-making processes. The accuracy and reliability of these models largely depend on the quality and relevance of the acquired data.
Importance of Data Acquisition
Data acquisition plays a crucial role in the success of predictive modelling projects. It ensures that the models are built upon a solid foundation of reliable and relevant data. Without proper data acquisition, predictive models may provide inaccurate, unreliable, or incomplete predictions, leading to erroneous decisions and potential business risks.
Good data acquisition practices involve the following steps:
- Data Identification: Identifying the data elements required for building predictive models.
- Data Collection: Gathering data from various sources, such as databases, APIs, web scraping, or direct measurements.
- Data Cleaning: Removing or correcting any inconsistencies, errors, or missing values in the collected data.
- Data Transformation: Converting the collected data into a suitable format for analysis, such as numerical or categorical variables.
- Data Integration: Combining data from different sources to create a unified dataset for modelling purposes.
Challenges in Data Acquisition
Data acquisition is not without challenges. Some common challenges include:
- Data Quality: Ensuring the accuracy, completeness, and consistency of acquired data.
- Data Privacy: Adhering to privacy regulations and ensuring the protection of sensitive or personal information.
- Data Volume: Dealing with large volumes of data and implementing efficient storage and processing mechanisms.
- Data Variety: Handling diverse data types, formats, and structures from multiple sources.
- Data Latency: Managing real-time data streams and processing data in near real-time.
Conclusion
Data acquisition is a fundamental step in the field of predictive modelling. It enables the collection, processing, and transformation of data to build accurate models that can predict future outcomes based on historical data. By understanding the importance of data acquisition and overcoming its challenges, businesses can harness the power of predictive modelling to gain valuable insights, make informed decisions, and stay ahead in today's competitive landscape.
Comments:
Thank you all for reading my article on Enhancing Data Acquisition Technology with ChatGPT! I'm excited to hear your thoughts and answer any questions you may have.
Great article, Maureen! It's fascinating how ChatGPT can be leveraged for predictive modeling. Do you think it's applicable to any type of data or are there specific domains where it works best?
Thanks, Alex! ChatGPT can be applied to a wide range of data domains, including text, images, and even code. It's a versatile language model that can provide valuable insights across different industries.
I found your article very informative, Maureen. How does ChatGPT handle unstructured data where there might be missing values or noise?
Thank you, Emily! ChatGPT can handle unstructured data reasonably well, but it’s important to preprocess the data and handle missing values or noise beforehand. It’s always recommended to clean and structure your data to improve the accuracy of the predictive model.
Hi Maureen, thanks for sharing your insights. I'm curious, what methods did you use to validate the accuracy of the ChatGPT predictive model?
Hi David! To validate the accuracy of the ChatGPT predictive model, I adopted various techniques like cross-validation, train-test splits, and comparing predicted results against ground truth. It's important to evaluate the model performance using appropriate metrics to ensure reliable predictions.
Interesting article, Maureen! How does ChatGPT handle complex datasets with a large number of attributes? Are there any limitations?
Thanks, Sophia! ChatGPT can handle complex datasets with numerous attributes, but there are limitations regarding computation and memory resources. Depending on the scale, preprocessing and feature engineering techniques might be required to extract relevant information and reduce the dimensionality of the data.
Really enjoyed your article, Maureen! How do you think the integration of ChatGPT with data acquisition technology will impact the future of predictive modeling?
Thank you, Liam! I believe the integration of ChatGPT with data acquisition technology has the potential to revolutionize predictive modeling. It can enhance the efficiency and accuracy of acquiring data while enabling faster and more insightful analysis. This combination opens up new possibilities for various industries, leading to better decision-making and improved outcomes.
Maureen, this is such a breakthrough! Do you have any tips for organizations looking to adopt ChatGPT for their predictive modeling initiatives?
Thanks, Chloe! When adopting ChatGPT for predictive modeling, it's crucial to start with well-defined objectives and a clear understanding of the problem you're trying to solve. Additionally, effective data preprocessing, feature engineering, and model evaluation are key steps to ensure successful implementation. It's also important to stay informed about the latest advancements and best practices in using ChatGPT for predictive modeling.
Great article, Maureen! I'm particularly interested in the ethical considerations of using ChatGPT in predictive modeling. How do you address potential biases in the data and outputs?
Thanks, Oliver! Addressing biases in ChatGPT predictive modeling is crucial. It involves careful dataset curation, identifying and mitigating biases in training data, and incorporating fairness metrics to evaluate the model's outputs. It's an ongoing process that requires continuous monitoring and evaluation.
Hi Maureen, great work on this article! As ChatGPT relies on pre-existing human conversations, could it potentially introduce any privacy concerns?
Thank you, Sophie! Privacy concerns can arise if not handled properly. OpenAI, the creator of ChatGPT, has protocols in place to protect user privacy and anonymize data. Adoption of appropriate safeguards and compliance with relevant data protection regulations can help mitigate any privacy concerns when using ChatGPT for predictive modeling.
Maureen, well done on the article! I'm curious, what are the hardware and software requirements for implementing ChatGPT in predictive modeling?
Thanks, Maxwell! Implementing ChatGPT in predictive modeling requires hardware with sufficient computational power, such as GPUs or TPUs. As for software, frameworks like TensorFlow or PyTorch can be used. It's essential to have the necessary resources for training large language models effectively.
Nice article, Maureen! What are some potential limitations or challenges in using ChatGPT for predictive modeling?
Thank you, Grace! Some limitations include potential biases learned from the training data, the need for substantial computational resources, and the challenge of generalizing to new or unseen data. Continuous monitoring, evaluation, and improvement are essential to address these limitations and ensure reliable predictive modeling.
Great insights, Maureen! How do you see the interpretability of ChatGPT models in predictive modeling? Can we trust the explanations it provides for its predictions?
Thanks, Mia! Interpretability is an important aspect of any predictive modeling technique. While ChatGPT can provide some explanations for its predictions, it's important to approach them with caution. Additional techniques, like model-agnostic approaches or attention mechanisms, can help gain insights and improve the interpretability of ChatGPT models.
Well-written article, Maureen! How does ChatGPT deal with datasets that include sensitive or personal information?
Thank you, Connor! To handle datasets with sensitive or personal information, it's crucial to follow data protection regulations and properly anonymize or remove any identifiable information. Organizations should employ appropriate privacy policies and take necessary measures to ensure ChatGPT models do not expose sensitive data during predictive modeling tasks.
This article was a great read, Maureen! Could you share some use cases where ChatGPT has shown promising results in predictive modeling?
Thanks, Ella! ChatGPT has shown promising results in various fields, including healthcare, customer service, and content generation. It has been used for tasks like analyzing medical records, improving chatbot interactions, and assisting with creative writing. The versatility of ChatGPT makes it an exciting tool for predictive modeling in many domains.
Maureen, thank you for sharing your expertise through this article. Do you think ChatGPT could eventually replace traditional predictive modeling techniques?
You're welcome, Sebastian! While ChatGPT is a powerful tool, I don't foresee it completely replacing traditional predictive modeling techniques. It can augment existing methods and provide additional insights, but a combination of approaches is often needed to achieve the best results. Leveraging the strengths of both traditional techniques and ChatGPT can lead to more robust predictive modeling outcomes.
Fantastic article, Maureen! How can organizations ensure the ethical use of ChatGPT in predictive modeling to avoid biases or unintended consequences?
Thank you, Harper! Organizations can ensure ethical use of ChatGPT in predictive modeling by implementing responsible AI practices. This includes proactively addressing biases in data, regularly evaluating and monitoring model performance, and maintaining transparency in the decision-making process. Open collaboration, diverse perspectives, and robust ethical frameworks can help prevent unintended consequences and promote fair and responsible predictive modeling with ChatGPT.
Great insights, Maureen! Could you share any future advancements or developments you anticipate in the field of data acquisition with ChatGPT?
Thanks, Zoe! In the future, we can expect advancements in ChatGPT's ability to handle more complex and diverse data types seamlessly. There may also be improvements in fine-tuning mechanisms to adapt the model to specific domains more efficiently. Further research into privacy-preserving techniques and better interpretability methods are also anticipated, enhancing the trust and usability of ChatGPT in data acquisition and predictive modeling.
Excellent article, Maureen! With ChatGPT's rising popularity, are there any emerging challenges that researchers and practitioners should be aware of?
Thank you, Nathan! As ChatGPT gains popularity, researchers and practitioners should be cognizant of potential ethical concerns, model biases, and limitations. It's important to consider the social impact of AI technologies like ChatGPT and ensure that they are used responsibly. Ongoing research and dialogue are necessary to address emerging challenges and promote the responsible development and deployment of ChatGPT in predictive modeling.
Maureen, your article was very enlightening! Could ChatGPT be used alongside traditional statistical models to improve predictive modeling outcomes?
Thanks, Anna! Absolutely, ChatGPT can be used alongside traditional statistical models to complement the predictive modeling process. By combining the strengths of both approaches, organizations can leverage the contextual understanding and language generation capabilities of ChatGPT while benefiting from the interpretability and explanatory power of traditional statistical models.
Maureen, your article was a great read! Are there any potential risks involved in relying heavily on ChatGPT for predictive modeling?
Thank you, Lucas! Relying heavily on ChatGPT for predictive modeling can introduce risks, such as the model generating incorrect or misleading predictions due to limited understanding or biases in the training data. It's important to maintain human oversight and critically evaluate the model's outputs. Proper validation, monitoring, and continuous improvement processes must be followed to mitigate any potential risks and ensure the reliability of predictive modeling using ChatGPT.
Thank you all for your engaging comments and questions! I appreciate your participation and enthusiasm. If you have any further inquiries or want to discuss anything related to the article, please don't hesitate to ask.