Enhancing Data Analysis in ATM Networks Technology with ChatGPT
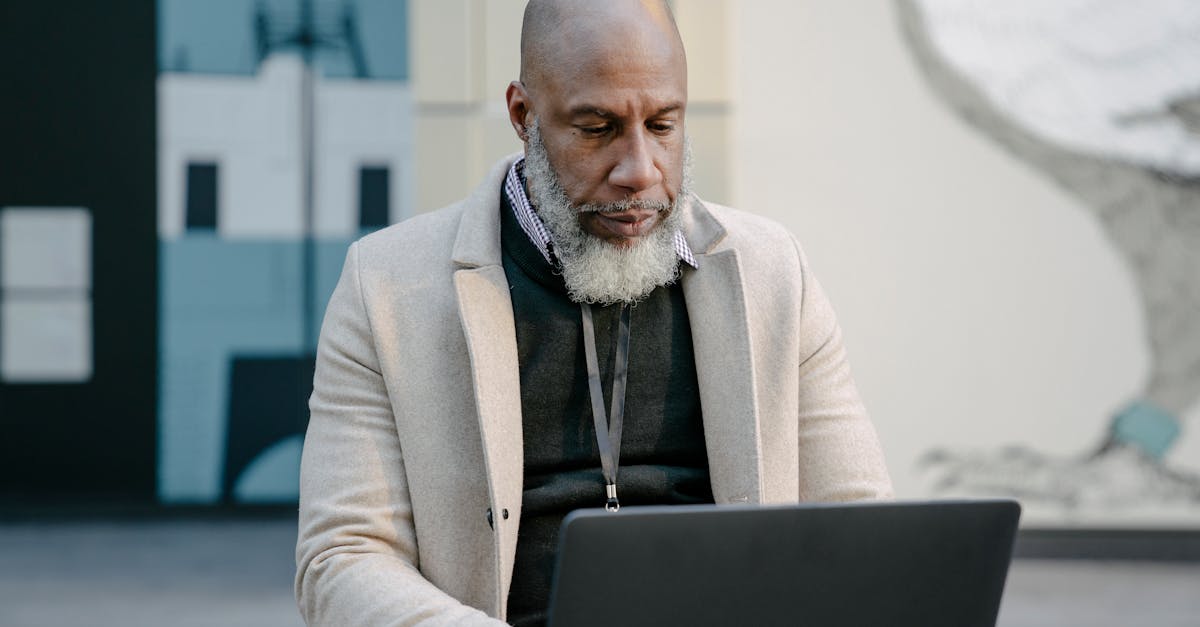
ATM networks, short for Asynchronous Transfer Mode networks, have become a fundamental part of the modern financial industry. These networks enable users to perform various banking transactions, such as cash withdrawals, fund transfers, balance inquiries, and more, conveniently and securely. However, what many people may not realize is the vast amount of data generated by ATM networks on a daily basis.
The data generated by ATM networks holds immense potential in providing valuable insights for both banking institutions and financial analysts. With the right tools and techniques, this data can be analyzed to gain a deeper understanding of customer behavior, improve banking services, detect fraudulent activities, and optimize ATM network performance.
Data analysis plays a crucial role in extracting meaningful information from the massive volumes of ATM network data. By leveraging data analysis techniques such as statistical analysis, data mining, and machine learning, analysts can identify trends, patterns, and anomalies in the data that can be used to drive business decisions.
One of the primary areas where data analysis can be applied to ATM network data is customer behavior analysis. By analyzing transaction records, financial institutions can gain insights into customer preferences, spending patterns, and even potential upselling opportunities. These insights can then be utilized to personalize banking services, develop targeted marketing campaigns, and improve customer satisfaction.
Another significant area of data analysis in the context of ATM networks is fraud detection. By monitoring transaction data in real-time and applying advanced analytics algorithms, potential fraudulent activities can be identified and prevented. Suspicious transactions, such as multiple withdrawals from different ATMs within a short time frame, can trigger alerts for further investigation, ultimately safeguarding the financial institution and its customers.
Furthermore, data analysis can also be employed to optimize the performance of ATM networks. By analyzing network traffic data, utilization rates, and response times, banking institutions can identify bottlenecks, predict peak usage periods, and enhance network efficiency. Additionally, data analysis can help identify underperforming ATM locations and determine the need for maintenance or relocation, saving costs and improving overall service quality.
In conclusion, the vast amount of data generated by ATM networks can be a goldmine of invaluable insights. Through the application of data analysis techniques, banking institutions and financial analysts can harness the power of this data to enhance customer experience, detect fraudulent activities, and optimize network performance. As the financial industry continues to evolve, leveraging the potential of ATM network data analysis will serve as a catalyst for innovation and improved financial services.
Comments:
Thank you all for taking the time to read my article on enhancing data analysis in ATM Networks Technology with ChatGPT. I'm excited to hear your thoughts and engage in a discussion!
Great article, Julia! I really enjoyed how you explained the potential of ChatGPT in improving data analysis in ATM networks. The use of AI in this field is fascinating.
Thank you, David! I agree, the application of AI in analyzing ATM network data can bring significant advancements in efficiency and security.
I agree, Julia. Privacy and security are crucial aspects when it comes to utilizing AI in sensitive areas such as ATM networks. Ensuring data protection should be a top priority.
Absolutely, David. Protecting customer data and maintaining privacy and security are essential considerations when implementing AI technologies in ATM networks or any other sensitive domain.
It's exciting to think about the possibilities of AI in ATM network data analysis. Great job, Julia!
Thank you, David! The possibilities AI brings to ATM network data analysis are indeed exciting, and I appreciate your positive feedback!
I found your article very informative, Julia. It's interesting to see how ChatGPT can contribute to enhancing data analysis in ATM networks. Do you think there are any potential challenges in implementing this technology?
Thank you, Sophie! Yes, implementing ChatGPT in ATM networks does come with challenges. One primary concern is ensuring the privacy and security of customer data while using AI algorithms for analysis.
Julia, do you think the implementation of ChatGPT in ATM networks would require hardware upgrades or can existing infrastructure supports it?
Great question, Sophie! ChatGPT implementation generally doesn't require significant hardware upgrades. It can be integrated into existing infrastructure, but optimizing the network's capacity may be necessary for handling increased data analysis load.
I agree, Julia. Privacy and security are always concerns in implementing AI technologies, especially in sensitive areas like ATM networks. Proper measures must be in place.
Exactly, Sophie. Prioritizing privacy and security measures is crucial to ensure trust and confidence in utilizing AI technologies, especially in the banking sector.
Excellent article, Julia! I believe integrating ChatGPT into ATM networks can revolutionize customer service as well. It can provide real-time support and answer customer queries efficiently.
Thank you, Raymond! You're right, ChatGPT can indeed enhance customer service in ATM networks by offering personalized and prompt assistance.
Julia, I believe integrating ChatGPT into ATM networks can also help customers with complex transactions and provide personalized financial advice. Do you think that's feasible?
Indeed, Raymond! ChatGPT's ability to understand and respond to complex queries makes it feasible to assist customers with various transaction-related inquiries and provide personalized financial advice.
I had no idea that ChatGPT could be so useful in the world of ATM networks. Your article opened my eyes to its potential. Well done, Julia!
Thank you, Sarah! I'm glad the article shed light on the wider applications of ChatGPT in the context of ATM networks.
Julia, what level of accuracy can we expect from ChatGPT in analyzing ATM network data? Are there any limitations to its accuracy?
Good question, Sarah! ChatGPT's accuracy in analyzing ATM network data can be high, but it's important to consider potential limitations due to the nature of the dataset, training methods, and the technology's inherent biases.
Julia, you did a great job explaining the technical aspects in a clear and concise manner. It's fascinating to see how AI can optimize data analysis in ATM networks.
Thank you, Michael! Making technical concepts understandable for a wider audience is always one of my goals. I appreciate your feedback!
Julia, do you think ChatGPT can also help in detecting and preventing fraudulent activities in ATM networks?
Absolutely, Michael! ChatGPT can contribute to fraud detection and prevention in ATM networks by analyzing patterns, identifying suspicious activities, and alerting the necessary authorities.
I'm impressed by the potential benefits that integrating ChatGPT into ATM networks can bring. Increased efficiency, improved security, and better customer service sound promising!
Indeed, Lily! The benefits of using ChatGPT in ATM networks are numerous and can significantly enhance the overall experience for both customers and network operators.
Julia, how do you envision the future of ATM networks, considering the advancements in AI and data analysis?
Excellent question, Lily! With AI and data analysis advancements, I envision a future where ATM networks offer seamless, personalized experiences, enhanced security measures, and real-time support for customers.
Julia, I appreciate your comprehensive exploration of ChatGPT's role in data analysis for ATM networks. It's exciting to see AI making strides in the financial sector.
Thank you, Oliver! AI and machine learning are undoubtedly transforming the financial sector, and their potential in ATM networks is promising.
Julia, do you think there will be any ethical considerations to address when integrating ChatGPT into ATM networks, especially regarding customer data privacy?
Absolutely, Oliver! Ethics plays a crucial role in AI implementation, especially in sensitive areas like banking. Ensuring customer data privacy, transparency, and accountability should be of utmost importance.
As an ATM network operator, I'm curious about the potential implementation challenges. Julia, could you provide some insights on overcoming these obstacles?
Certainly, Maria! Overcoming implementation challenges requires careful planning, collaboration with AI experts, and thorough testing to ensure privacy, security, and accuracy in the analysis.
Thank you, Julia! I agree that careful planning and collaboration are key to overcoming implementation challenges and ensuring the successful integration of ChatGPT in ATM networks.
You're welcome, Maria! I'm glad you found the insights helpful. Collaboration and careful planning can indeed go a long way in successfully implementing AI technologies in ATM networks.
The use of ChatGPT for data analysis in ATM networks seems promising. Julia, do you think there might be any limitations or drawbacks to its implementation?
Good question, Daniel! While ChatGPT has great potential, there might be limitations in handling certain types of complex queries or dealing with rare scenarios. It's important to consider these factors when implementing the technology.
Thank you for highlighting the potential limitations, Julia. Considering biases is crucial to avoid unintended consequences in the analysis results.
Exactly, Daniel. Being aware of biases and taking steps to mitigate them is important to ensure fair and accurate analysis results in the context of ATM networks.
Thank you for addressing the limitations, Julia. It's vital to assess the technology's limitations to set realistic expectations and utilize it effectively.
Julia, your article sparked my interest in the potential of ChatGPT for ATM networks. Are there any specific use cases where ChatGPT has already been successfully implemented?
Thank you, Emily! ChatGPT has been successfully implemented in various customer service chatbots, virtual assistants, and knowledge bases to provide quick and accurate responses in different industries.
Julia, it's fascinating to see how ChatGPT's success in other industries can now be leveraged in the ATM network domain. Exciting times ahead!
Indeed, Emily! The advancements made in AI applications across different industries can be leveraged to enhance ATM networks and provide a more efficient and personalized experience.
Julia, I appreciate how you highlighted the implications of using AI in ATM networks and the importance of maintaining a balance between automation and human involvement.
Thank you, Jack! Finding the right balance between AI and human involvement is crucial to ensure the best results and maintain the human touch in customer interactions.
Julia, I think your article opens up a new realm of possibilities in the field of ATM networks. AI integration has great potential in improving efficiency and customer satisfaction.
Thank you, Jack! I believe AI integration, when done thoughtfully, can indeed bring significant improvements to ATM networks, benefiting both operators and customers.