Enhancing Data Analysis in Design for Manufacturing Technology with ChatGPT
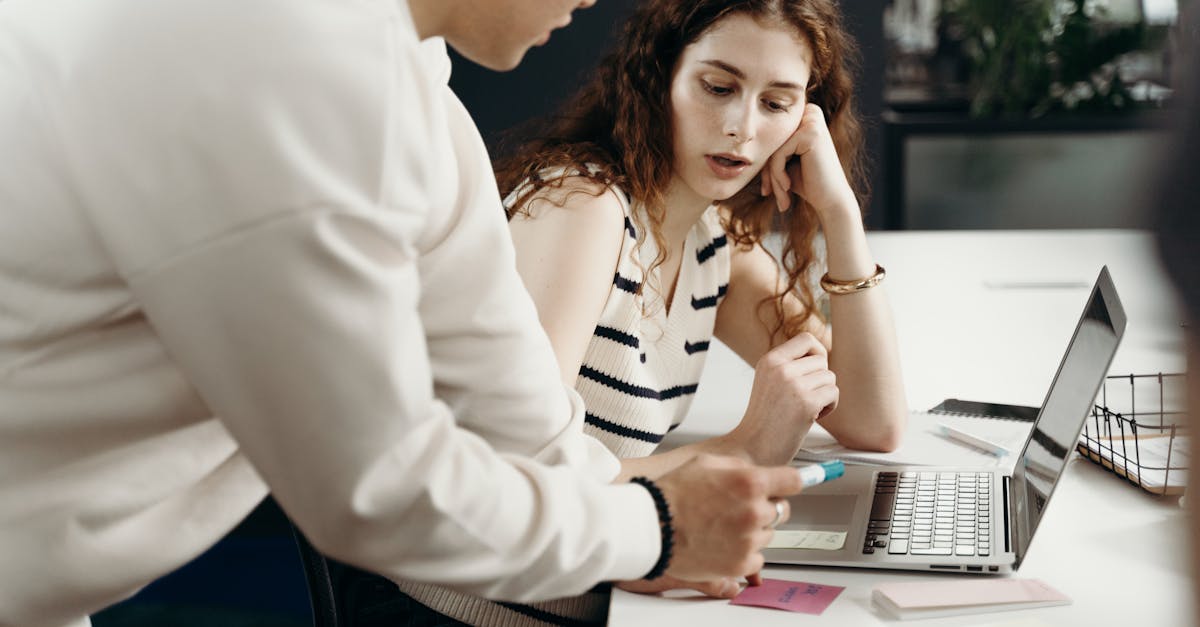
In today's fast-paced manufacturing industry, companies are constantly striving for increased efficiency and productivity in their design and production processes. Design for Manufacturing (DFM) is a technology that focuses on optimizing designs for easier and more cost-effective manufacturing.
One key aspect of DFM is the usage of data analysis techniques to gain valuable insights into the design process. With the advancements in artificial intelligence (AI) technology, large sets of manufacturing data can now be processed and analyzed in a much faster and more accurate manner.
How does it work?
The AI can take in vast amounts of manufacturing data, including design drawings, specifications, production records, and performance data, and analyze them using data analysis algorithms. These algorithms can identify patterns, correlations, and anomalies within the data, revealing potential areas for improvement in design efficiency.
The AI system can perform various data analysis techniques, such as statistical analysis, predictive modeling, and optimization algorithms. By analyzing the data, it can identify bottlenecks in the production process, suggest modifications to the design to reduce manufacturing complexities, and optimize the use of resources.
Benefits of DFM and Data Analysis
The combination of DFM and data analysis has numerous advantages for manufacturers:
- Enhanced Design Efficiency: By leveraging data analysis, manufacturers can identify design features that may lead to production challenges or delays. This enables them to modify the design early in the process, optimizing it for easier and more efficient manufacturing.
- Reduced Manufacturing Costs: Data analysis algorithms can identify cost-saving opportunities, such as the elimination of unnecessary components or the optimization of manufacturing processes. This can lead to significant cost reductions and increased competitiveness in the market.
- Improved Product Quality: By analyzing performance data and identifying correlations, manufacturers can make design adjustments that enhance product quality and reliability.
- Faster Time-to-Market: With AI-powered data analysis, manufacturers can quickly analyze large amounts of data, gaining insights that can accelerate the design and production process, reducing time-to-market for new products.
The Future of Design for Manufacturing
The integration of AI technology and data analysis techniques in DFM is still in its early stages, but its potential impact on the manufacturing industry is immense. As AI algorithms become more intelligent and sophisticated, manufacturers will be able to leverage the power of big data to optimize their designs and processes to levels never before possible.
Furthermore, the combination of DFM and data analysis has the potential to revolutionize the entire product development lifecycle. With the ability to continuously collect and analyze manufacturing data, manufacturers can create iterative feedback loops, improving designs and processes in real-time and adapting to changing market demands.
Conclusion
The use of AI technology and data analysis techniques in Design for Manufacturing opens up new possibilities for manufacturers to optimize their designs, improve manufacturing efficiency, and reduce costs. By leveraging the power of data, manufacturers can stay competitive in today's rapidly evolving market and deliver products of the highest quality.
Comments:
Thank you all for joining the discussion on my blog article 'Enhancing Data Analysis in Design for Manufacturing Technology with ChatGPT'. I am excited to hear your thoughts and answer any questions you may have!
Great article, Sam! I found the concept of using ChatGPT in manufacturing technology fascinating. It seems like it can greatly enhance data analysis and improve efficiency. Do you think there are any limitations or challenges to its implementation?
Thank you for your feedback, Adam! While ChatGPT has shown promising results, one limitation could be the quality and volume of the data it has been trained on. It might struggle with industry-specific jargon and context. Additionally, ensuring the model's biases don't interfere with decision making is an important consideration.
I'm curious about the potential impact of ChatGPT on collaboration between different teams involved in manufacturing. Could you elaborate on that, Sam?
That's a great question, Melissa! ChatGPT can facilitate collaboration by acting as a virtual assistant that assists different team members with data analysis tasks. It can help bridge the gap between teams who may have varied expertise and knowledge levels regarding data analysis techniques.
I wonder about the security of using ChatGPT in a manufacturing environment. How can we ensure that sensitive data is protected?
Excellent point, Robert! Security is crucial when implementing any technology. It's important to carefully manage access controls and encrypt sensitive data when using ChatGPT. Additionally, regular security audits and updates to the system's protocols can help mitigate risks.
I can see the benefit of using ChatGPT for data analysis, but what about the interpretability of its results? How can we trust the decisions it makes?
Interpretability is indeed important, Sarah. A potential approach is to introduce transparency into the system by designing explanations or providing context along with the generated results. This way, users can understand the reasoning behind the decisions made by ChatGPT and build trust in its outputs.
I'm curious about the training process of ChatGPT. How much training data is typically required to achieve good results in a manufacturing context?
Great question, Emily! The training process of ChatGPT requires a significant amount of data to achieve good performance. In a manufacturing context, a large dataset that covers various scenarios, challenges, and solutions is ideal. This helps to ensure that the model can generalize well and provide meaningful insights.
I love the idea of leveraging AI for data analysis in manufacturing. Do you think ChatGPT can replace human analysts altogether or is it better seen as a complementary tool?
Fantastic question, David! ChatGPT should be seen as a complementary tool rather than a replacement for human analysts. It can automate certain aspects of data analysis and provide insights, but human expertise is still valuable for critical thinking, domain knowledge, and contextual understanding that AI may lack.
Considering the constant advancements in AI, how do you see the role of ChatGPT evolving in the future, Sam?
Thanks for bringing that up, Lisa! In the future, I believe ChatGPT and similar AI technologies will become even more powerful, leveraging larger datasets and improved training techniques. They will continue to support decision-making processes, facilitate collaboration, and provide valuable insights in manufacturing and other industries.
I'm concerned about potential biases in the training data. How can we ensure that ChatGPT doesn't reinforce any preexisting biases and maintains fairness?
Valid concern, Kevin. Bias mitigation is crucial. It starts with carefully curating diverse and representative training data while being mindful of potential biases. Additionally, ongoing evaluation and monitoring of ChatGPT's outputs can help identify and address any biases that may arise.
Following up on Emily's question, how long does it take to train a ChatGPT model for manufacturing applications?
The training time for ChatGPT can vary significantly based on the size of the dataset, computational resources available, and the desired level of performance. It can range from several days to even weeks, especially for larger models with more parameters.
I see immense potential in using ChatGPT for improving data analysis in design for manufacturing. How would you recommend organizations get started with implementing this technology?
Great to hear your enthusiasm, James! Implementing ChatGPT involves defining objectives, selecting the right training data, fine-tuning the model, and establishing a feedback loop for continuous improvement. Organizations can start with small pilot projects to understand the technology's potential before scaling it across their manufacturing processes.
Is ChatGPT compatible with different types of manufacturing technologies, or is it more suitable for specific ones?
ChatGPT is versatile and can be used with different types of manufacturing technologies. It can be applied to diverse areas such as process optimization, quality control, predictive maintenance, and supply chain management. Its adaptability makes it a valuable tool across various manufacturing domains.
Sam, what kind of accuracy or error rate can we expect from ChatGPT in manufacturing applications?
Achieving a specific accuracy or error rate is challenging to predict, Adam. It depends on various factors such as the quality of the training data, the complexity of the manufacturing processes involved, and the level of fine-tuning performed. It's best to establish appropriate evaluation metrics and benchmarks specific to the application at hand.
I'm concerned about potential job displacement with the introduction of AI like ChatGPT. What are your thoughts on this, Sam?
Valid concern, Melissa. While AI may automate certain tasks, it also creates new opportunities. Instead of job displacement, we should focus on upskilling the workforce to work alongside AI technologies. People can transition to higher-value tasks that require creativity, critical thinking, and decision-making, ensuring a positive impact on job roles in the long run.
I would like to know if there are any current real-world examples of ChatGPT's successful implementation in the manufacturing sector?
Certainly, Robert! There are already several real-world examples where ChatGPT has proven its value in manufacturing. For instance, it has been used to optimize production processes, predict failure risks, and assist in quality control inspections. These applications showcase the potential of using ChatGPT to enhance data analysis in manufacturing.
Regarding the training data, what strategies can be employed to ensure it is representative and covers diverse scenarios?
Great question, Emily! To ensure representative training data, organizations can gather data from various sources, involve experts from different teams, and consider real-life scenarios encountered in manufacturing. It's important to cover different challenges, variations, and edge cases so that ChatGPT can handle a wide range of situations.
Can ChatGPT handle unstructured data from sources like images and sensor readings, or is it primarily focused on text-based data?
ChatGPT primarily excels in text-based data analysis, Kevin. However, it can also be coupled with other AI models specialized in image analysis or sensor data interpretation. By combining different models, organizations can leverage ChatGPT's strengths alongside the capabilities of other models to analyze a broader range of data types.
Are there any ethical considerations that organizations should keep in mind when using ChatGPT for data analysis in manufacturing?
Absolutely, Sarah. Ethical considerations are vital. Organizations should ensure that the data used for training is gathered and used responsibly, respecting privacy regulations and avoiding biases. Transparent communication about the system's capabilities and limitations is also essential to ensure ethical use.
How challenging it is for non-technical personnel to interact with ChatGPT for data analysis? Do they need extensive technical knowledge?
ChatGPT can be designed to be user-friendly and accessible to non-technical personnel, David. While some technical knowledge can be helpful, the goal is to make the interaction intuitive and provide clear instructions or interfaces. With a well-designed user experience, non-technical personnel should be able to leverage ChatGPT effectively.
What potential limitations or risks should organizations consider before implementing ChatGPT for data analysis in the manufacturing sector?
Good question, Lisa! Organizations should be aware of potential outputs that may not align with expectations due to biases or data limitations. Security considerations like data protection and privacy should also be addressed. Additionally, there should be a plan to continuously monitor and evaluate ChatGPT's performance and address any limitations or risks that arise.
What kind of computational resources are typically needed to implement ChatGPT for manufacturing data analysis?
The computational resources required for ChatGPT can vary depending on the model size, training data, and the hardware available. It can range from running the model on a single GPU to leveraging large-scale compute clusters with specialized AI accelerators. The aim is to have a setup that provides a balance between resource usage and performance.
Could you provide some examples of the types of insights or recommendations ChatGPT can generate in a manufacturing context?
Certainly, Mary! ChatGPT can generate insights such as process optimization recommendations based on historical data, early detection of quality issues, suggestions for maintenance schedules, or even provide recommendations for supply chain optimization. The specific insights depend on the data available and the objectives of the analysis.
What are the key factors organizations should consider before deciding to implement ChatGPT in their manufacturing operations?
Adam, organizations should first evaluate their data availability and quality. Sufficient data is crucial for training ChatGPT effectively. They should also assess their existing data analysis processes and identify areas where ChatGPT can provide value. Additionally, considering the feasibility and potential return on investment is essential.
Sam, how do you see the implementation of ChatGPT impacting the overall competitiveness of manufacturers?
Melissa, the implementation of ChatGPT can enhance the competitiveness of manufacturers by improving operational efficiency, enabling better decision-making, and reducing time-to-market for new products. By leveraging AI technologies like ChatGPT, manufacturers can gain a competitive edge by leveraging data-driven insights to optimize their processes and improve overall performance.
In terms of scalability, what kind of challenges might organizations face when implementing ChatGPT for data analysis in manufacturing? Are there any limitations?
Scalability can be a challenge, Robert. As the volume of data and complexity of manufacturing processes increase, ensuring efficient processing of requests and maintaining response times becomes crucial. Adequate computational resources, efficient architecture design, and constant monitoring are essential to handle scalability challenges and ensure smooth operations.
What are the potential cost implications of implementing ChatGPT for data analysis in manufacturing? Is it affordable for organizations of different sizes?
The cost implications can vary depending on factors like the scale of implementation, computational resources required, and the level of customization needed. While there may be initial investment costs, organizations can assess the potential return on investment based on expected benefits. The affordability for organizations of different sizes will depend on their specific circumstances and priorities.