Enhancing Data Analysis in Multi-Unit Technology with ChatGPT
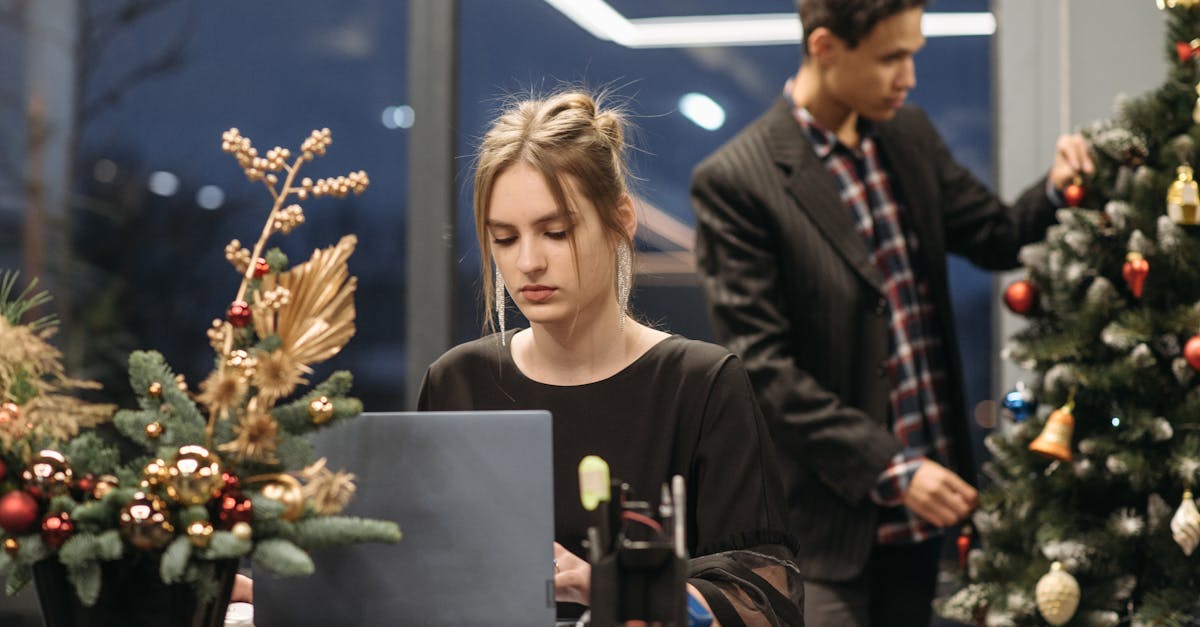
With the advancements in artificial intelligence, ChatGPT-4 has emerged as an incredibly powerful tool for assisting in data analysis. This multi-unit technology has revolutionized the way data is interpreted, visualized, and trends are predicted.
Interpreting Complex Data
Data analysis often involves dealing with complex datasets that can be difficult to comprehend and extract meaningful insights from. ChatGPT-4 is designed to assist analysts in interpreting such complex data by providing relevant explanations and context.
By leveraging its powerful natural language processing capabilities, ChatGPT-4 can understand the intricacies of the data and provide explanations in a user-friendly manner. It can help analysts uncover patterns, relationships, and correlations within the data that might otherwise go unnoticed. This makes data analysis faster, more accurate, and less prone to human biases.
Creating Visual Representations
Visualizations play a crucial role in data analysis, as they provide a quick and intuitive way to understand the patterns and trends present in the data. ChatGPT-4 excels in creating visual representations that effectively convey complex information.
Using its advanced analytical capabilities, ChatGPT-4 can automatically generate a wide range of visualizations, including charts, graphs, and even interactive dashboards. These visual representations aid in identifying key insights, outliers, and trends within the data. Analysts can customize these visualizations according to their requirements and present them in reports or presentations.
Predicting Trends
One of the most valuable aspects of data analysis is the ability to predict future trends based on historical data. ChatGPT-4 is equipped with powerful predictive modeling capabilities, enabling analysts to forecast trends accurately.
By analyzing historical patterns, ChatGPT-4 can identify hidden trends and patterns that might not be evident to human analysts. It can provide insights into emerging trends, market shifts, or customer behavior, helping organizations make informed business decisions.
Conclusion
ChatGPT-4 has transformed the field of data analysis by revolutionizing the way complex data is interpreted, visualized, and trends are predicted. Its ability to understand and explain complex data, create visual representations, and predict trends makes it an invaluable tool for analysts.
By leveraging ChatGPT-4, data analysts can unlock deeper insights from their datasets, make data-driven decisions, and gain a competitive edge in today's data-driven world.
Comments:
Thank you all for taking the time to read my article on enhancing data analysis with ChatGPT! I'm excited to hear your thoughts and answer any questions you may have.
Great article, Paula-Kaye! I really enjoyed reading it. The idea of leveraging ChatGPT for multi-unit technology is fascinating. How do you envision it being used in real-world scenarios?
Thank you, Emily! In real-world scenarios, ChatGPT can be used to analyze and interpret data from multiple technology units simultaneously. For example, it can be applied to analyze data collected from multiple IoT devices or analyze data from a network of connected sensors. It can help identify patterns, anomalies, or derive meaningful insights from massive volumes of data.
Well-written article, Paula-Kaye! ChatGPT seems like a promising approach to enhancing data analysis. However, what are some of the challenges or limitations you foresee when implementing this in a multi-unit technology setup?
Thank you, Daniel! While ChatGPT offers exciting possibilities, there are some challenges to consider. One challenge is ensuring the accuracy and reliability of the analysis when dealing with large datasets. Training ChatGPT on diverse data can help address this, but there may still be cases where it produces inaccurate or biased results. Another challenge is the potential impact of data privacy and security concerns, especially when dealing with sensitive data from multiple units.
Excellent article, Paula-Kaye! I am curious about the computational requirements for implementing ChatGPT in multi-unit technology. Would it require a significant amount of computational resources?
Thank you, Isabella! Implementing ChatGPT in a multi-unit technology setup does require a considerable amount of computational resources. Training ChatGPT on large datasets and processing data from multiple units can be computationally intensive. However, advancements in hardware and distributed processing techniques can help mitigate these challenges to some extent.
Impressive article, Paula-Kaye! ChatGPT has great potential in multi-unit technology. Are there any specific industries or domains where you see the most immediate value in applying this approach?
Thank you, Alexandra! ChatGPT can be valuable in various industries and domains. Some immediate applications could be in smart cities, industrial automation, healthcare monitoring systems, or energy management systems. It can assist in data analysis and provide valuable insights for optimizing operations, identifying anomalies, predicting failures, and improving overall efficiency.
Well done on the article, Paula-Kaye! I'm intrigued by the potential impact of ChatGPT in multi-unit technology, but is there a risk of overreliance on automated data analysis? How do we ensure the human context is not overlooked?
Thank you, Oliver! Overreliance on automated data analysis is indeed a concern. While ChatGPT can assist in processing and analyzing vast amounts of data, it's crucial to maintain a balance and involve human expertise in interpreting the results. Human context, domain knowledge, and critical thinking should not be overlooked as they play a vital role in decision-making and understanding the larger context.
Interesting article, Paula-Kaye! Do you think ChatGPT can handle real-time data analysis in multi-unit technology, or is it more suited for offline analysis?
Thank you, Sophia! ChatGPT can be used for both real-time and offline data analysis in multi-unit technology. In real-time scenarios, it can process and analyze data as it is generated, providing immediate insights and responses. For offline analysis, it can handle large volumes of historical data, allowing thorough analysis and exploration of trends and patterns.
Brilliant article, Paula-Kaye! How does ChatGPT handle outliers or anomalies in multi-unit data? Can it detect and alert about unusual patterns or data points?
Thank you, Hannah! ChatGPT can be trained to detect outliers or anomalies in multi-unit data. By learning patterns from diverse and representative datasets during training, it can identify unusual patterns or data points that deviate from the norm. This can help in detecting anomalies or alerting about potential issues that may require attention in the multi-unit technology setup.
Insightful article, Paula-Kaye! I'm curious about the scalability aspect. How well does ChatGPT scale when analyzing data from a large number of units?
Thank you, Nathan! The scalability of ChatGPT depends on several factors, including the hardware setup and the size of the dataset. With powerful computational resources and distributed processing techniques, it can scale to handle data from a large number of units. However, it's important to consider the computational demands and resource allocation to ensure efficient analysis of the data.
Well-articulated article, Paula-Kaye! Could ChatGPT's analysis capabilities in multi-unit technology be improved by incorporating user feedback or domain-specific knowledge during the training phase?
Thank you, Liam! User feedback and domain-specific knowledge can definitely enhance ChatGPT's analysis capabilities. Models like ChatGPT can benefit from continuous feedback loops, where user feedback is used to improve the accuracy and relevance of the analysis. Incorporating domain-specific knowledge can also help in better understanding and contextualizing the data, leading to more meaningful insights.
Fantastic article, Paula-Kaye! How does ChatGPT handle the integration of data from different types of units or devices with varying data formats?
Thank you, Elizabeth! ChatGPT can handle the integration of data from different types of units or devices with varying data formats. By preprocessing and transforming the data into a common format, ChatGPT can effectively analyze and interpret the integrated data. However, data preprocessing and standardization are essential to ensure accurate analysis and to handle any format inconsistencies.
Informative article, Paula-Kaye! What are some potential risks or ethical considerations associated with using ChatGPT for data analysis in a multi-unit technology environment?
Thank you, Henry! There are indeed ethical considerations when using ChatGPT for data analysis in multi-unit technology. One risk is the potential for the algorithm to amplify or perpetuate biases present in the training data. Ensuring diverse and representative training data can mitigate this risk. Additionally, the responsible use of data and addressing privacy concerns are vital to maintain trust and avoid misuse of data in sensitive multi-unit technology environments.
Well-explained article, Paula-Kaye! How does ChatGPT handle real-world challenges such as missing data or incomplete observations in multi-unit technology?
Thank you, Abigail! ChatGPT can handle missing data or incomplete observations in multi-unit technology, up to a certain extent. By training on datasets with missing data and employing imputation techniques, it can learn to make reasonable predictions or fill in the missing information. However, the accuracy of the analysis may depend on the extent of missing data and the nature of the missingness.
Engaging article, Paula-Kaye! Are there any privacy concerns when processing and analyzing data from multiple units using ChatGPT? How can these concerns be addressed?
Thank you, Victoria! Privacy concerns are indeed significant when processing and analyzing data from multiple units with ChatGPT. Anonymization of the data, adopting strong encryption practices, and ensuring strict access controls can help mitigate privacy risks. Additionally, policies and guidelines must be in place to govern data usage, storage, and sharing to maintain compliance with privacy regulations and protect sensitive information.
Interesting insights, Paula-Kaye! Could you elaborate on the potential roles of human-machine collaboration in multi-unit technology? How can ChatGPT and human analysts complement each other?
Thank you, Jack! Human-machine collaboration plays a crucial role in multi-unit technology. ChatGPT can assist in automating data analysis tasks, processing large volumes of data, and identifying patterns or anomalies. Human analysts, on the other hand, bring domain expertise, critical thinking, and context to the analysis. By working together, human analysts can validate the findings, interpret the results in the larger context, and make informed decisions based on both machine-generated insights and human insights.
Well-presented article, Paula-Kaye! Can ChatGPT be tailored or customized to specific domain requirements in multi-unit technology, or does it have a more generic approach?
Thank you, Grace! ChatGPT can be tailored and customized to specific domain requirements in multi-unit technology. Fine-tuning the model on domain-specific datasets can improve its performance and relevance within that particular domain. By training on relevant data and incorporating domain-specific language, it can provide more accurate and meaningful insights tailored to the specific needs of multi-unit technology setups.
Excellent article, Paula-Kaye! I'm curious about the training process of ChatGPT for multi-unit technology. How does it deal with the trade-off between model complexity and training time?
Thank you, Sophie! The training process of ChatGPT involves finding the right balance between model complexity and training time. Increasing the model complexity can potentially improve performance but comes with increased computational requirements and longer training times. Various strategies like pretraining on large datasets and fine-tuning on domain-specific datasets can help strike the right balance, allowing for effective analysis without excessive training times.
Informative article, Paula-Kaye! Do you see any challenges in building trust and adoption of ChatGPT-based data analysis in multi-unit technology environments?
Thank you, Michael! Building trust and adoption of ChatGPT-based data analysis in multi-unit technology environments can face challenges. One significant challenge is the explainability of the analysis results. The black-box nature of models like ChatGPT can make it difficult for users to understand how the analysis is performed. Incorporating explainable AI techniques and providing transparency in the analysis process can help build trust and encourage adoption among users and stakeholders.
Well-argued article, Paula-Kaye! How can ChatGPT handle multilingual data in multi-unit technology? Can it analyze data from units that provide information in different languages?
Thank you, Scarlett! ChatGPT can indeed handle multilingual data in multi-unit technology. By training on diverse datasets that include multiple languages, it can learn to analyze and process data in different languages. This enables it to handle data from units that provide information in various languages. However, it's important to ensure language-specific preprocessing and training to achieve accurate and reliable analysis results.
Fascinating article, Paula-Kaye! What are the key considerations when selecting or preparing the training data for ChatGPT in a multi-unit technology environment?
Thank you, Blake! When selecting or preparing the training data for ChatGPT in a multi-unit technology environment, several key considerations should be kept in mind. The training data should be diverse, representative, and relevant to the specific domain or application. It's important to include data from different units, data formats, and various scenarios to ensure the model generalizes well. Additionally, data pre-processing and cleaning play a crucial role in removing noise, inconsistencies, or biases that can impact the quality of analysis.
Great job on the article, Paula-Kaye! Can ChatGPT handle online learning or adaptation in the context of multi-unit technology?
Thank you, Lucy! ChatGPT currently does not support online learning or adaptation out of the box. However, with suitable modifications and approaches, it is possible to enable online learning in the context of multi-unit technology. This would allow the model to adapt and learn from new incoming data in real-time, improving its performance and applicability in dynamic multi-unit environments.
Insightful article, Paula-Kaye! Considering the large volume of data typically involved in multi-unit technology, how can ChatGPT handle the challenges of data storage and processing?
Thank you, David! Handling the challenges of data storage and processing in multi-unit technology requires robust infrastructure and distributed processing techniques. ChatGPT can be deployed in distributed computing environments or cloud platforms that provide scalable storage and processing capabilities. Using technologies like data lakes, distributed file systems, or parallel computing, it can handle large volumes of data efficiently, enabling analysis at scale.
Excellent article, Paula-Kaye! How does ChatGPT handle time-series data in the context of multi-unit technology?
Thank you, Ruby! ChatGPT can handle time-series data in the context of multi-unit technology. By capturing temporal patterns and dependencies during training, it can analyze and interpret time-series data from multiple units. This can be valuable in detecting trends, forecasting future values, or identifying anomalies over time, providing valuable insights for decision-making and optimization in multi-unit technology environments.
Great read, Paula-Kaye! How can enhanced data analysis with ChatGPT in multi-unit technology contribute to advancement in other fields, such as predictive maintenance or resource optimization?
Thank you, Oscar! Enhanced data analysis with ChatGPT in multi-unit technology can have significant contributions to other fields. For example, in predictive maintenance, it can analyze data from multiple units to identify patterns indicative of potential failures, enabling timely maintenance actions. In resource optimization, it can help analyze data from various units to identify inefficiencies or areas for improvement, leading to optimized allocation of resources. ChatGPT's capability to process and interpret large-scale data can drive advancements in these and many other domains.
Well-articulated article, Paula-Kaye! In the context of multi-unit technology, can ChatGPT handle unstructured or textual data effectively?
Thank you, Emily! ChatGPT is effective in handling unstructured or textual data in the context of multi-unit technology. With its language processing capabilities, it can analyze and interpret textual data from various sources, such as sensor logs, diagnostic reports, or maintenance records. By learning from a wide range of textual data during training, ChatGPT can extract valuable insights and information from unstructured data, contributing to comprehensive data analysis in multi-unit technology environments.
Engrossing article, Paula-Kaye! How can the integration of ChatGPT in multi-unit technology impact decision-making processes?
Thank you, Mason! The integration of ChatGPT in multi-unit technology can significantly impact decision-making processes. By providing automated data analysis and insights, it enables faster and more objective decision-making. Real-time analysis can help identify emerging patterns or issues, allowing proactive decision-making. Additionally, the availability of comprehensive analysis results can support informed decision-making, optimize resource allocation, and improve overall operational efficiency in multi-unit technology setups.