Enhancing Data Analysis with ChatGPT: Harnessing the Power of 'Balance' Technology
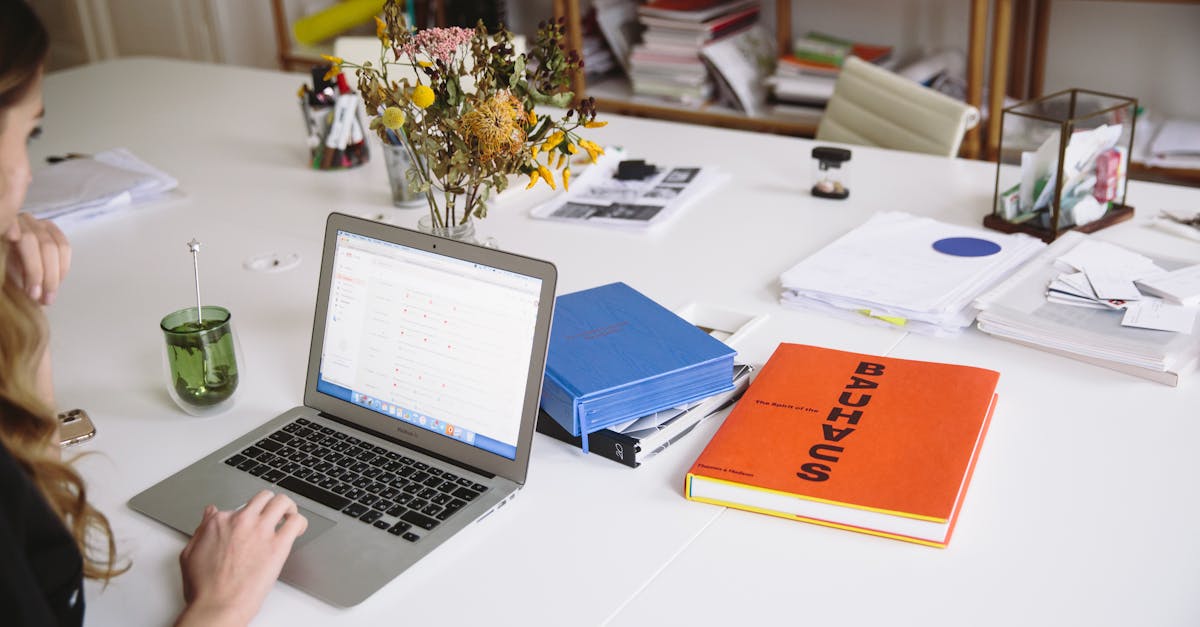
Data analysis has become an integral part of many industries. The ability to extract valuable insights from large data sets can provide businesses with a competitive edge. However, the sheer volume and complexity of data can make analysis a daunting task. This is where the concept of balance comes into play.
Understanding Balance
In the context of data analysis, balance refers to the distribution of resources, such as computing power and memory, between different components of the analysis process. It involves optimizing the allocation of resources to ensure efficient and accurate analysis.
Balance can be achieved at various levels, including hardware, software, and algorithmic levels. At the hardware level, having a well-configured and properly scaled infrastructure can prevent bottlenecks and improve overall performance. At the software level, using appropriate tools and frameworks can enhance efficiency and facilitate smooth data processing. Lastly, at the algorithmic level, employing algorithms that strike the right balance between complexity and accuracy is crucial.
The Role of Balance in Large Data Sets
Large data sets pose unique challenges in terms of analysis. The sheer volume of data can overwhelm traditional analysis methods, making it difficult to draw meaningful conclusions. However, by maintaining balance, data analysts can tackle these challenges effectively.
One way balance can be achieved is through parallel processing. By distributing the workload across multiple machines or processors, the analysis can be executed in a fraction of the time compared to sequential processing. This not only reduces the time required for analysis but also enables quick iterations and experimentation.
Analyzing Patterns and Gaining Insights
With the power of balance, data analysis can effectively uncover patterns and provide valuable insights. By analyzing large data sets, patterns that may go unnoticed with traditional analysis methods can be identified. These patterns can reveal correlations, trends, and anomalies, which can be instrumental in making informed decisions.
In addition to pattern recognition, balance also plays a crucial role in data visualization. Visualization techniques enable analysts to communicate complex data in a more digestible and comprehensible manner. By balancing the use of charts, graphs, and other visual aids, analysis results can be better presented and understood by stakeholders.
Conclusion
The power of balance in data analysis cannot be underestimated. From optimizing resource allocation to enabling efficient parallel processing, balance plays a vital role in ensuring accurate and insightful analysis. By leveraging the right technology and maintaining balance at various levels, businesses can harness the full potential of their data and gain a competitive edge in their respective industries.
Comments:
Thank you all for joining the discussion on my blog post! I'm looking forward to hearing your thoughts on enhancing data analysis with ChatGPT.
I really enjoyed reading your article, Fabio! The concept of using ChatGPT for data analysis is fascinating. I'm curious to know if there are any limitations in its application.
Hi Emily! I'm glad you liked the article. ChatGPT is powerful, but it has limitations. It may generate plausible-sounding responses without being accurate or having access to up-to-date information. So it's important to verify its outputs.
Fabio, I think ChatGPT has amazing potential for assisting with data analysis. But I'm curious about its practical applications. How can it be integrated into existing data analysis workflows?
Hi Liam! You can use the OpenAI API to integrate ChatGPT into your workflows. For instance, you can send prompts to the API and get model-generated responses. This way, you can rely on ChatGPT in real-time while analyzing data.
Fabio, are there any concerns about biased outputs from ChatGPT during data analysis? How can we ensure fairness?
Valid concern, Sophia. Bias is an issue. OpenAI has made efforts to reduce both glaring and subtle biases in ChatGPT. They use a technique called 'instructing the model', which helps to avoid biased responses. But it's a challenge they continue to work on.
Fabio, I'm impressed with the potential of ChatGPT! But is there any way to control the output to make it more specific to the data analysis task at hand?
Absolutely, Olivia! You can provide instructions or specify the format you want the answer in. By using carefully crafted prompts, you can guide the model to generate more relevant and specific responses to your data analysis tasks.
Fabio, are there any risks associated with relying heavily on ChatGPT for data analysis?
Good question, Olivia. One of the risks is over-reliance on ChatGPT without properly verifying its output. There's also the possibility of the model providing inaccurate or misleading responses. So it's important to use it as an assisting tool, not a substitute for human analysis.
Fabio, what kind of computing resources are required to leverage ChatGPT effectively?
Great question, Olivia! ChatGPT inference can be performed on the OpenAI API, so you don't require extensive computing resources locally. You can make API calls based on your usage needs, making it accessible to a wide range of data analysts.
Fabio, how does ChatGPT handle privacy and data confidentiality concerns during data analysis?
Olivia, ChatGPT processes data entered as prompts, but OpenAI retains the data only for 30 days and doesn't use it to improve its models. They have a strong commitment to privacy and the responsible handling of user data.
Fabio, do you have any recommendations for bridging the gap between human analysts and ChatGPT-based data analysis?
Absolutely, Olivia! Proper documentation, clear instructions, and establishing feedback loops between human analysts and ChatGPT outputs can help bridge the gap. Collaboration and ongoing interaction are vital for successful integration of ChatGPT in data analysis workflows.
Fabio, how can data analysts ensure transparency when incorporating ChatGPT into their analysis process?
Transparency is important, Olivia. By documenting the use of ChatGPT in the analysis process, clearly stating its role, outputs, and limitations, analysts can ensure transparency. This helps stakeholders understand the contributions of both the model and the human analyst.
Fabio, what are your thoughts on the potential impact of ChatGPT on the field of data analysis?
Fabio, do you have any recommendations for those who want to start integrating ChatGPT into their data analysis workflows?
Certainly, Sophia! To get started, you can explore the OpenAI API documentation, experiment with sample code, and gradually incorporate ChatGPT into your workflows. It's important to have a clear understanding of its capabilities and limitations for efficient integration.
Fabio, is ChatGPT suitable for analyzing real-time data? Could it handle data streams effectively?
Sophia, ChatGPT is not designed for real-time data analysis due to its sequential processing. It may not handle data streams efficiently. However, you can leverage it for tasks at different stages of the data analysis workflow, such as exploratory analysis or final insights.
Fabio, what are some potential challenges when using ChatGPT for data analysis?
Great question, Sophia. Some challenges include generating accurate and reliable responses, addressing potential bias in outputs, and guiding the model to focus on specific aspects of the analysis. Continuous evaluation and improvement are essential to overcome these challenges.
Fabio, have there been any cases where ChatGPT's responses were significantly different from what domain experts expected in data analysis?
Yes, Sophia. There have been instances where ChatGPT's responses surprised domain experts during data analysis. It shows the model's ability to generate novel insights or identify patterns that might have been overlooked. However, careful verification and collaboration with experts are still crucial to validate these unexpected findings.
Fabio, do you have any advice on how to choose appropriate data analysis tasks to leverage ChatGPT?
Certainly, Sophia! It's important to identify tasks where ChatGPT's generation capabilities can provide value. These can be tasks involving summarization, natural language interactions, code explanations, or answering specific questions about databases. Consider the strengths of ChatGPT and align them with your data analysis needs.
Fabio, have you personally used ChatGPT for any of your own data analysis projects?
Yes, Liam! I have used ChatGPT to assist in analyzing large datasets and extracting meaningful insights. It has proven to be quite effective in handling complex data analysis tasks.
Fabio, what are some potential avenues for future improvements in ChatGPT?
Great question, Liam! OpenAI is actively working on refining the model by reducing biases, improving its behavior, and allowing more user control over outputs. They are also gathering user feedback to understand how it can better address the specific needs of data analysts.
Fabio, can you share any success stories where ChatGPT has significantly improved a data analysis process?
Certainly, Liam! One noteworthy success story is a team that used ChatGPT to streamline their database analysis process. It helped them quickly retrieve relevant database entries based on user queries, saving significant time and effort.
Fabio, how does ChatGPT handle large-scale data analysis tasks? Are there any limitations in terms of data size?
Liam, ChatGPT can handle a range of data analysis tasks, including those involving large datasets. However, there are practical limitations in terms of message length and response time, so it's important to chunk the data and manage the interactions accordingly.
Fabio, thank you for sharing your insights on enhancing data analysis with ChatGPT. It has definitely sparked my interest in exploring this technology further!
Fabio, thank you for sharing your expertise and insights on ChatGPT for data analysis. It has certainly opened up new possibilities in my mind!
That's interesting, Fabio! Do you have any examples of specific data analysis tasks where ChatGPT has shown great results?
Certainly, Emily! ChatGPT has been used for tasks like summarizing articles, answering questions about databases, and even providing code explanations. It's a versatile tool for many data analysis scenarios.
I completely agree, Fabio. ChatGPT should be used as a tool to enhance data analysis, not replace human expertise. Humans can bring critical thinking and domain knowledge that a model may lack.
That's good to know, Fabio! It makes it easier for anyone to leverage the power of ChatGPT in their data analysis projects without worrying about hardware limitations.
That is reassuring, Fabio. Privacy and data security are critical considerations, especially in data analysis projects that deal with sensitive information.
I agree, Fabio. Communication and feedback loops are crucial to ensure effective collaboration between analysts and AI tools in data analysis projects.
Absolutely, Fabio. Transparent communication helps build trust and clarity around the utilization of AI models like ChatGPT in data analysis.
I believe ChatGPT can significantly enhance data analysis by providing quick insights, assisting in complex analyses, and augmenting the capabilities of data analysts. It has great potential for making data analysis more efficient and accessible.
I find the concept of ChatGPT for data analysis quite intriguing. Fabio, would you recommend using it for exploratory analysis or focusing more on the final analysis stages?
Hi Jane! ChatGPT can be effectively utilized in both exploratory and final analysis stages. It can assist in exploring the data, generating preliminary insights, and also providing valuable input for the final analysis. It complements the human analyst's capabilities throughout the entire data analysis process.