Enhancing Data Filtering Efficiency with ChatGPT in Apache Kafka: A Powerful Combination
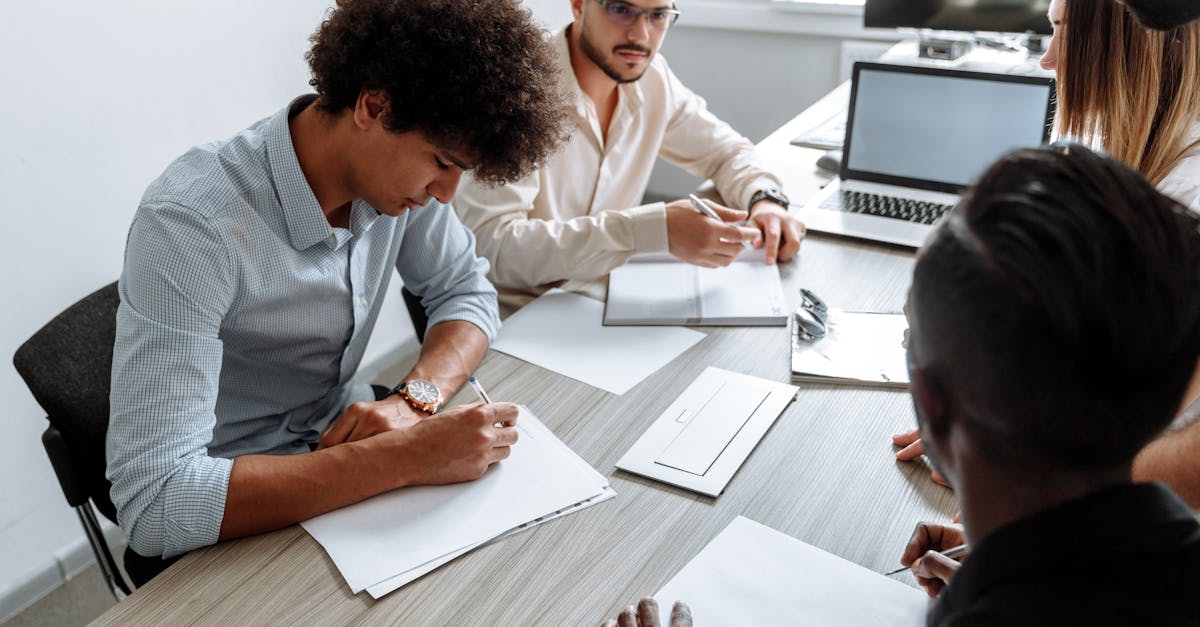
Apache Kafka is a popular distributed streaming platform that is widely used for building real-time data pipelines and streaming applications. It provides a reliable, highly scalable, and fault-tolerant messaging system for handling massive amounts of data. One of the key features of Apache Kafka is its ability to handle data filtering efficiently.
Data Filtering with Apache Kafka
Data filtering is a critical task in data analysis, as it helps to extract relevant information from a large amount of data. Apache Kafka offers a powerful mechanism for data filtering through its Kafka Streams API. The Kafka Streams API allows users to build stream processing applications that can filter, transform, and aggregate data in real-time.
ChatGPT-4, an advanced natural language processing model, can be integrated with Apache Kafka to perform data filtering tasks. ChatGPT-4 is known for its ability to understand and generate human-like text, making it highly useful for analyzing and processing natural language data.
How ChatGPT-4 Can Help in Data Filtering
With the integration of ChatGPT-4 and Apache Kafka, data filtering becomes much more efficient and accurate. ChatGPT-4 can process the data streaming through Apache Kafka and help sort and analyze it based on specific criteria or keywords.
Here are some specific ways ChatGPT-4 can be utilized for data filtering with Apache Kafka:
- Content Filtering: ChatGPT-4 can analyze the content of the incoming data and filter out irrelevant or unwanted information. This can be particularly useful in scenarios where only specific types of data need to be processed or stored.
- Sentiment Analysis: ChatGPT-4 can perform sentiment analysis on textual data, helping to identify positive, negative, or neutral sentiments associated with the messages. This can be helpful in filtering out messages that contain negative or spammy content.
- Keyword Filtering: ChatGPT-4 can be trained to recognize specific keywords or patterns in the data and filter out messages that match those keywords. This can be useful in scenarios where certain information needs to be extracted based on predefined criteria.
- Topic Modeling: By leveraging ChatGPT-4's ability to understand the context and topic of the messages, data filtering can be performed based on the relevance of the messages to a specific topic. This can be helpful in scenarios where only messages related to a particular subject are of interest.
Benefits of Using Apache Kafka and ChatGPT-4 for Data Filtering
The integration of Apache Kafka and ChatGPT-4 offers several advantages for data filtering:
- Real-time Filtering: Apache Kafka allows for processing data in real-time, ensuring that the filtering results are up-to-date and accurate.
- Scalability: Apache Kafka's distributed architecture enables the processing of large amounts of data, making it suitable for filtering massive data streams.
- Natural Language Processing: ChatGPT-4's advanced natural language processing capabilities enhance the accuracy and effectiveness of data filtering, especially for textual data.
- Customizability: ChatGPT-4 can be trained and fine-tuned to adapt to specific filtering requirements, allowing for highly customized data filtering solutions.
- Reduced Manual Effort: By automating the data filtering process with Apache Kafka and ChatGPT-4, manual effort and human errors are minimized, resulting in increased efficiency.
Conclusion
Data filtering is a crucial aspect of data analysis, and Apache Kafka, when combined with ChatGPT-4, offers a powerful solution for efficiently filtering and analyzing real-time data streams. The ability to process data in real-time, coupled with ChatGPT-4's advanced natural language processing capabilities, makes this integration highly valuable for various applications that require accurate and efficient data filtering.
Comments:
Thank you all for your interest and comments on my article. I'm glad to see the discussion starting!
Scott, could you elaborate more on the specific benefits of using ChatGPT in combination with Apache Kafka?
Great article, Scott! I found the combination of ChatGPT and Apache Kafka really interesting. It opens up a lot of possibilities for enhancing data filtering efficiency.
I agree, Rachel. It's exciting to see how AI technologies can be integrated with powerful data processing tools like Kafka.
I'm curious about the performance implications of using ChatGPT with Kafka. Has anyone tried this combination in a real-world scenario?
Scott, what challenges did you face while implementing this combination? Any particular lessons learned?
Jennifer, I encountered some initial challenges regarding the integration and configuring Kafka to work seamlessly with ChatGPT. However, by experimenting and seeking advice from the community, I was able to overcome them successfully.
Emily, thanks for sharing your experience. It's good to know that challenges can be overcome with determination and community support. I'm excited to experiment with this combination.
I've used ChatGPT along with Kafka in a production environment, and I must say the performance was impressive. The setup requires careful consideration, but the results are worth it.
Scott, can you provide some examples of practical applications where this combination can be beneficial?
Mark, one practical application is in the streaming analytics domain. By leveraging ChatGPT to filter and process incoming data in Kafka, organizations can gain real-time insights and make quick decisions based on the analyzed data.
I'm wondering if there are any limitations or potential drawbacks to using ChatGPT and Kafka together?
Laura, one limitation I can think of is the training and fine-tuning requirements for ChatGPT. It requires substantial computing resources and labeled data to achieve optimum performance.
Nathan, thanks for highlighting that point. Managing the training process effectively can be a challenge, especially for organizations with limited resources.
Scott, do you have any recommendations for organizations looking to adopt this combination? Any best practices?
Brian, organizations planning to use this combination should ensure they have a clear understanding of the intended use case and allocate sufficient resources for training and deployment. Collaboration between data scientists, engineers, and domain experts is crucial to achieve optimal results.
What are the potential security considerations when using ChatGPT and Kafka together? Any thoughts?
Sarah, when using ChatGPT and Kafka, it's important to implement secure communication protocols, encryption, and access controls to safeguard sensitive data. Regular security audits are also recommended.
I'm interested to know if ChatGPT can work well with other data processing frameworks apart from Kafka.
Robert, yes, ChatGPT can work well with other data processing frameworks. The key is to choose frameworks that can handle the desired scale, integrate seamlessly, and provide the necessary input/output capabilities for ChatGPT.
Robert, ChatGPT can be integrated with various data processing frameworks, such as Spark or Flink. It depends on the specific use case and requirements.
How does the scalability of ChatGPT and Kafka play out when handling large volumes of data?
Karen, both ChatGPT and Kafka are designed to be scalable. By properly partitioning data across Kafka topics and ensuring efficient resource utilization for ChatGPT, the combination can handle large volumes of data effectively.
Karen, when scaling ChatGPT and Kafka, it's essential to consider horizontal scaling by distributing the workload across multiple instances or nodes. This ensures effective utilization of resources and improves overall performance.
Scott, have you seen any examples of organizations successfully deploying this combination in production?
Roberto, yes, there are organizations successfully using this combination in production. However, due to the sensitivity of their specific use cases, they might not be able to publicly share all the details.
Scott, I understand the confidentiality aspect. It's good to know that the combination has proven value in real-world scenarios.
What are some of the use cases where ChatGPT and Kafka have been particularly effective?
Emily, I've seen ChatGPT and Kafka being used effectively in fraud detection, real-time monitoring of social media streams, and dynamic content filtering based on user feedback.
John, those use cases highlight the versatility of ChatGPT and Kafka. It's exciting to see how they can be applied across different industries.
How would you compare the performance of ChatGPT and Kafka with other similar tools or technologies?
Victoria, the performance comparison depends on the specific requirements and use case. Each tool has its strengths, but the combination of ChatGPT and Kafka can provide a powerful solution for real-time data filtering and analysis.
Scott, are there any specific industry sectors where the combination of ChatGPT and Kafka can bring significant value?
Sophia, the combination of ChatGPT and Kafka can be valuable in sectors like e-commerce for dynamic product recommendation, finance for fraud detection, healthcare for analyzing patient data, and many more. It has broad applicability.
Scott, thank you for the insight. It's fascinating to see how this combination can address various challenges across different industries.
Scott, I can see the potential for significant value across different sectors. Thank you for sharing your insights on this powerful combination!
What are some of the key features or functionalities of ChatGPT that make it a good fit with Kafka?
Alex, ChatGPT's ability to understand and generate human-like text makes it a good fit for processing text data streams in Kafka. It can analyze, filter, and enrich data based on complex criteria.
Laura, could you share some practical examples of dynamic content filtering using ChatGPT and Kafka?
Karen, one example of dynamic content filtering could be in an online news platform. By integrating ChatGPT with Kafka, the system can analyze incoming news articles, filter out irrelevant or low-quality content, and ensure that users receive relevant and high-quality news in real-time.
Laura, that's a great example! It shows how ChatGPT and Kafka can improve the user experience on news platforms by delivering personalized and filtered content.
Laura, I assume the data flow between Kafka and ChatGPT is bidirectional. Can ChatGPT also generate responses based on user input or queries?
Rachel, you're correct. The bidirectional data flow allows ChatGPT to generate responses based on user input or queries. It can engage in interactive conversations, making it highly versatile.
Laura, that's fascinating! The ability of ChatGPT to provide dynamic responses based on user queries adds another layer of value to the combination with Kafka.
Laura, this bidirectional capability showcases the potential of ChatGPT and Kafka for building intelligent chatbots or virtual assistants that can handle a wide range of conversational scenarios.
Can you provide an overview of the implementation process for integrating ChatGPT with Kafka?
Gregory, the implementation process typically involves setting up a Kafka cluster, configuring topics and partitions, integrating with ChatGPT using appropriate APIs, and ensuring proper data serialization and compatibility. It requires coordination between data engineers and AI specialists.
David, thanks for the overview. It sounds like a collaborative effort between different teams is crucial for a successful implementation.
David, I appreciate the detailed explanation. It seems like a comprehensive process that requires careful planning and coordination between different teams.
This combination seems highly promising. I'm excited to see how it evolves further and what new use cases it will be applied to.