Enhancing Data Modeling in Teradata Data Warehouse with ChatGPT: A Smarter Approach to Data Management
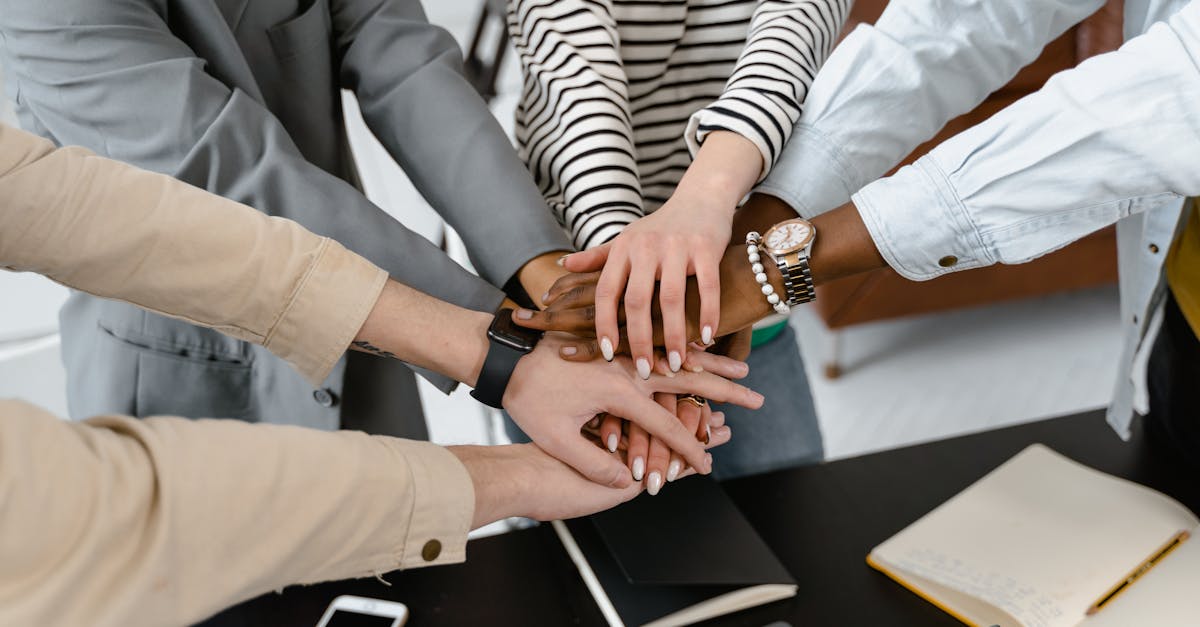
Data modeling plays a crucial role in designing efficient and effective data warehouses. It involves creating logical and physical data models that facilitate the organization, storage, and retrieval of data. With the advancements in artificial intelligence and natural language processing, chatgpt-4 emerges as a promising technology to aid in the development of these data models and schemas, enabling a more efficient data modeling process.
The Power of Teradata Data Warehouse
Teradata Data Warehouse is a powerful and highly scalable analytical database platform that allows organizations to consolidate their diverse data sources into one unified view. It provides robust data warehousing capabilities, including data integration, data storage, and advanced analytics, offering a comprehensive solution for managing large volumes of structured and unstructured data.
The Significance of Data Modeling in Teradata
Data modeling is crucial in the context of a Teradata Data Warehouse as it helps design the structure, relationships, and constraints of the data. It allows organizations to define data schemas, optimize performance, and ensure data integrity. Effective data modeling ensures that the data warehouse is designed in such a way that it meets the current and future analytic and reporting needs of the organization.
The Efficiency Challenge in Data Modeling
Data modeling is a complex process that requires meticulous attention to detail and a deep understanding of the organization's data requirements. Traditionally, data modeling involves multiple stakeholders, including business analysts, data architects, and subject matter experts, coming together to define the data models and schemas. This collaborative approach often leads to iterations, lengthy meetings, and significant effort, causing delays in the data modeling process.
chatgpt-4: Revolutionizing Data Modeling
With the introduction of chatgpt-4, the data modeling process in Teradata Data Warehouse can be greatly enhanced. Powered by state-of-the-art language models and machine learning algorithms, chatgpt-4 possesses the capability to understand and generate human-like text, making it an ideal virtual assistant for data modeling tasks.
Utilizing chatgpt-4, stakeholders involved in the data modeling process can communicate their requirements to the system using plain language. The system can then interpret the inputs, analyze the data context, and generate data models and schemas accordingly. This eliminates the need for extensive manual work and speeds up the data modeling cycle.
Benefits of Using chatgpt-4 in Data Modeling
- Improved Efficiency: chatgpt-4 automates various aspects of data modeling, reducing manual effort and minimizing the time required to develop data models.
- Enhanced Collaboration: chatgpt-4 acts as a virtual assistant, supporting collaboration among stakeholders by providing suggestions, insights, and guiding them through the data modeling process.
- Reduced Errors: By leveraging the power of chatgpt-4, organizations can minimize human errors in data modeling, ensuring the accuracy and integrity of the data models and schemas.
- Increased Scalability: As chatgpt-4 can handle a large volume of data modeling requests simultaneously, it allows organizations to scale their data modeling efforts efficiently.
Conclusion
The advent of chatgpt-4 in the data modeling field brings numerous benefits to the Teradata Data Warehouse ecosystem. Organizations can leverage this advanced technology to streamline and expedite their data modeling processes, resulting in improved efficiency, enhanced collaboration, reduced errors, and increased scalability. By incorporating chatgpt-4 into their data modeling workflows, organizations can take a significant step towards building robust and optimized data warehouses that meet their analytical and reporting needs.
Comments:
Great article, Jay! I really enjoyed reading about how ChatGPT can enhance data modeling.
I agree, Oliver! ChatGPT's ability to understand natural language queries can definitely streamline data management.
Thank you, Oliver! I appreciate your positive feedback.
Oliver and Rachel, I completely agree! Natural language interfaces can revolutionize data management.
Rachel and Chloe, natural language understanding can simplify data modeling for non-technical users.
This is fascinating! I never thought about using AI like ChatGPT for data management.
Emily, it's amazing how AI technologies like ChatGPT continue to evolve and find applications in various fields.
Jacob, I'm glad you find the advancements in AI and ChatGPT fascinating.
Jay, your article is a testament to the ongoing advancements in AI-driven data management.
Jay, AI's ability to automate data management tasks promotes efficiency and mitigates human error.
I'm intrigued by the potential of ChatGPT in the data warehouse. Can't wait to see more applications.
Michael, I'm also curious to see how ChatGPT can be further leveraged in data warehouse scenarios.
Sophie, there are indeed exciting possibilities ChatGPT can bring to the data warehouse.
Jay, I'm curious about the challenges and limitations that might arise in using ChatGPT for data warehousing.
Sophie, the combination of human expertise and ChatGPT's suggestions shows great promise.
Jay, incorporating ethics and bias considerations into AI systems like ChatGPT will be crucial in data management.
Jay, discussing AI ethics in the data management landscape is vital to avoid biases and ensure fairness.
Data modeling can definitely benefit from smarter approaches like ChatGPT.
Absolutely, Sophia! An intelligent system like ChatGPT can significantly improve data modeling accuracy.
Emma, ChatGPT's intelligent suggestions can indeed minimize errors in data modeling.
The article highlights the possibilities of enhancing data management. Impressive!
I'm excited to see how ChatGPT can improve the efficiency of data modeling in Teradata.
Laura, I'm confident that ChatGPT will indeed contribute to better data modeling practices.
Natalie, ChatGPT holds promise for improving data modeling practices significantly.
Jay, with ChatGPT, we can aim for more accurate and efficient data modeling outcomes.
Jay, I'm curious about how ChatGPT handles outliers and anomalies in data modeling.
Laura, I share in your excitement about the potential of ChatGPT in data modeling.
Jay, I appreciate your effort to highlight the potential of ChatGPT for data modeling.
Jay, do you have any insights on integrating ChatGPT in existing data modeling workflows?
This article is a great introduction to the potential benefits of using ChatGPT in data management.
Benjamin, the integration of AI technologies like ChatGPT with data management systems has immense potential.
Daniel, integrating AI solutions like ChatGPT can bring valuable insights to data management.
ChatGPT seems like it could revolutionize the way we approach data modeling.
Agreed, Olivia! ChatGPT can offer a fresh perspective on data modeling and decision-making.
Olivia, the inclusion of ChatGPT in data modeling workflows can lead to better decision-making.
Olivia, the interpretability of ChatGPT's decision-making process could greatly benefit data modeling teams.
Interesting article! I'd love to hear more about practical use cases for ChatGPT in data warehouses.
Henry, real-world examples of ChatGPT in data warehouses would definitely enrich our understanding.
Gabriel, real-world use cases would definitely demonstrate the potential value of ChatGPT better.
Gabriel, examples showing how ChatGPT has improved existing data modeling processes would be insightful.
ChatGPT's capabilities make it an exciting tool for enhancing data modeling.
Adam, I'm thrilled to witness the impact of ChatGPT on data modeling practices.
David, it's great to see your enthusiasm for the positive impact of ChatGPT.
Jay, your article provided a great overview of the benefits of using ChatGPT in data modeling.
Jay, I'm excited about the potential of ChatGPT to automate repetitive tasks in data modeling.
Jay, could ChatGPT's language model be fine-tuned specifically for data modeling tasks?
Jay, I wonder how ChatGPT handles complex data relationships and dependencies in a data warehouse.
Jay, it would be interesting to explore how ChatGPT can align with existing data modeling methodologies.
Jay, how can ChatGPT assist in identifying and resolving data quality issues in a warehouse?
The article showcases how AI advancements can bring more efficiency to data management processes.