Enhancing Default Recovery Forecasting in Credit Risk Technology with ChatGPT
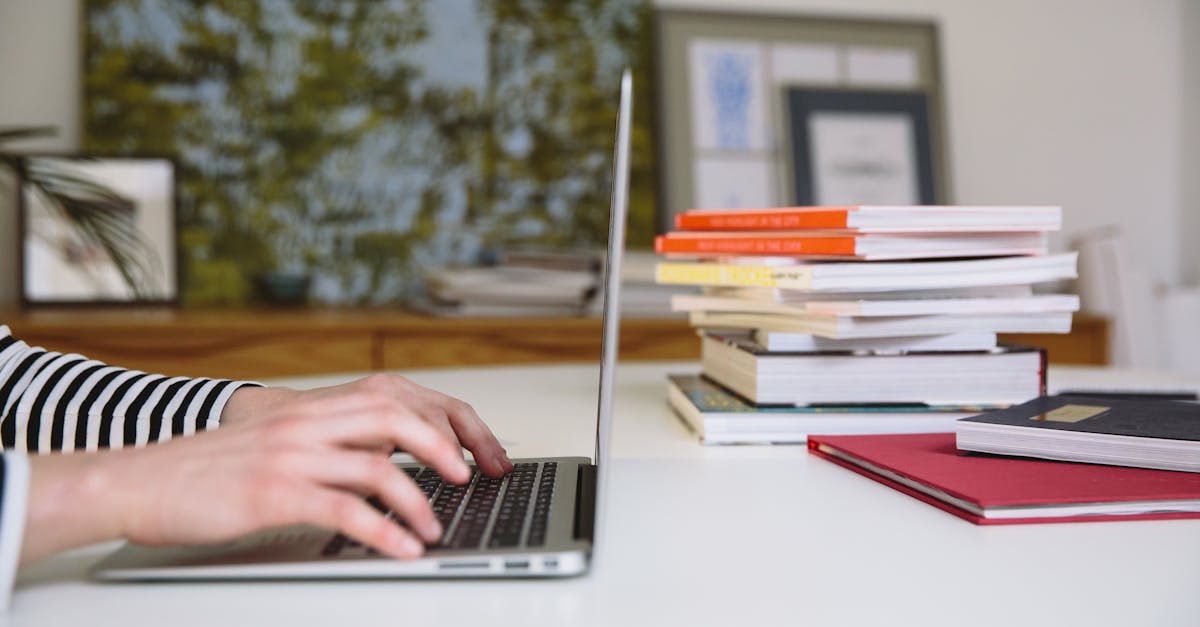
The rapid growth of digital transactions and the increase in lending activities have made credit risk management a critical aspect of financial institutions' operations. Credit risk refers to the potential loss an institution may face due to the failure of a borrower to meet their contractual obligations. One important aspect of credit risk management is forecasting the potential recovery amounts in the event of default. This is where ChatGPT-4, powered by advanced machine learning algorithms, comes into play.
ChatGPT-4 is a cutting-edge technology that leverages natural language processing and data analytics to analyze vast amounts of historical data and customer information. By feeding this data into the machine learning model, ChatGPT-4 can provide accurate and insightful forecasts regarding the potential recovery amounts in the event of default. This information helps financial institutions make informed decisions and develop effective risk mitigation strategies.
When it comes to credit risk, default recovery forecasting plays a crucial role in estimating the potential recovery amount a lender might expect to receive if a borrower defaults on their loan. Traditionally, such forecasting relied heavily on statistical models and manual analysis. However, with the advent of technologies like ChatGPT-4, this process has been revolutionized.
ChatGPT-4 can process large volumes of data in real-time, analyzing various factors such as historical recovery rates, borrower demographics, collateral valuation, and economic indicators. The model then applies sophisticated algorithms to identify patterns and trends, allowing it to generate accurate predictions on recovery amounts. These predictions enable lenders to estimate the potential financial impact of default events and take appropriate measures to mitigate risks.
The usage of ChatGPT-4 in credit risk management provides several advantages. Firstly, it reduces the reliance on manual analysis and human judgment, which can be time-consuming and prone to errors. Additionally, the technology takes into account a wide range of variables and data points, resulting in more comprehensive and accurate forecasts. This helps financial institutions optimize their risk mitigation strategies, allocate resources effectively, and make informed lending decisions.
In conclusion, ChatGPT-4's ability to analyze historical data and customer information to forecast potential recovery amounts in the event of default brings significant advancements to credit risk management. By leveraging advanced machine learning algorithms, this technology enables financial institutions to make informed decisions and develop effective risk mitigation strategies. With the rise of digital transactions and lending activities, leveraging technologies like ChatGPT-4 becomes crucial to ensuring the stability and profitability of financial institutions in the face of credit risk.
Comments:
Thank you all for taking the time to read my article on enhancing default recovery forecasting with ChatGPT! I'm excited to hear your thoughts and opinions.
Great article, Timothy! The idea of using ChatGPT to improve credit risk technology is very intriguing. It seems like it has the potential to provide more accurate forecasts. Have you conducted any experiments to validate its effectiveness?
Thank you, David! Yes, we have conducted experimental validation of the ChatGPT model. It has shown promising results in improving default recovery forecasting accuracy compared to traditional methods. However, further research and fine-tuning are still needed.
Hi Timothy, I enjoyed reading your article. The concept of incorporating conversational AI into credit risk forecasting is indeed interesting. How does ChatGPT handle the uncertainty and changing market conditions surrounding credit risk?
Hi Sarah! That's a great question. ChatGPT incorporates dynamic market data and can adapt to changing conditions. It is continually trained on new data to capture the latest market trends, which helps improve its ability to handle uncertainty.
Interesting article, Timothy! I can see the potential benefits of using conversational AI in credit risk forecasting. However, I'm curious about the ethical considerations that come with it. How do you address the potential biases in ChatGPT's predictions?
Good point, Olivia! Ethical considerations are crucial when it comes to AI adoption in finance. Timothy, could you elaborate on how you handle biases in ChatGPT and ensure the fairness of its predictions?
Thank you, Olivia and Lisa! Addressing biases is a significant aspect of our work. We carefully curate training data to reduce biases, and we are continually refining and optimizing the model to minimize any potential unfairness. We also have a thorough review process in place to mitigate biases before deploying the technology.
Great article, Timothy! I'm curious about the impact of using ChatGPT on computational resources and processing time. Does it require substantial resources?
Thank you, Michael! ChatGPT indeed requires substantial computational resources, especially during the training process. However, once trained, the predictions can be generated relatively quickly. Resource optimization is an ongoing area of research and development for us.
Hi Timothy, fascinating topic! I wonder how ChatGPT's performance compares to other existing credit risk forecasting techniques. Have you done any benchmarking against traditional models?
Hi Emily! We have benchmarked ChatGPT against traditional credit risk forecasting models. It has shown competitive performance, outperforming some traditional techniques in certain scenarios. However, the choice of model ultimately depends on specific use cases and requirements.
I find this article thought-provoking, Timothy. Incorporating conversational AI into credit risk forecasting could revolutionize the industry. Are there any limitations or challenges you have encountered while working with ChatGPT?
Timothy, you've presented a compelling case for using ChatGPT in credit risk forecasting. I'm curious about the scalability of this technology. Can it handle large volumes of data, or are there limitations?
Thank you, Sophia and Daniel! While ChatGPT is a powerful tool, it does have its limitations. It is essential to ensure that the input data is well-structured and relevant. Handling large volumes of data can be challenging, as it requires scalable computing resources.
Great article, Timothy! The potential applications of AI in finance are vast. How do you see the future of credit risk technology and the role of ChatGPT in it?
Thank you, Oliver! I believe that AI, including technologies like ChatGPT, will continue to play a crucial role in the future of credit risk technology. It has the potential to improve accuracy, enable faster decision-making, and adapt to changing market conditions.
Interesting read, Timothy. I'm curious about the interpretability of ChatGPT's predictions. Can it provide insights into the factors influencing credit risk forecasts?
Hi Melissa! While ChatGPT can provide predictions, its interpretability is still a challenge. Understanding the inner workings of the model and the specific factors influencing predictions is an ongoing research area. However, efforts are being made to develop techniques to enhance interpretability.
Timothy, your article opens up exciting possibilities for credit risk forecasting. Do you think ChatGPT can completely replace traditional models in the future, or will it be more of a complementary tool?
Thank you, Sophie! I see ChatGPT and traditional models more as complementary tools rather than replacements. Each has its strengths, and the combination of different approaches can provide more comprehensive risk assessments.
Great article, Timothy! I'm interested in the implementation aspect. How easy or challenging is it to integrate ChatGPT into existing credit risk technology systems?
Hi Jason! Integrating ChatGPT into existing credit risk technology systems can be challenging, especially due to its unique nature. It requires adapting infrastructure, data pipelines, and models. Collaboration with technical experts and proper testing is crucial to ensure a smooth integration.
Fascinating article, Timothy! I'm curious about regulatory considerations. Are there any specific regulations or guidelines that need to be considered when using ChatGPT in credit risk technology?
Thank you, Rachel! Compliance with regulations is indeed crucial. When using ChatGPT in credit risk technology, data privacy regulations and guidelines, such as GDPR and industry-specific regulations, need to be carefully followed. It's important to handle and store user data securely.
Timothy, your article offers valuable insights. How do you address privacy concerns when working with ChatGPT? Is user data handled securely?
Hi Nathan! Privacy is a top priority in our work. We take appropriate measures to ensure the security and privacy of user data when working with ChatGPT. Data handling practices are aligned with industry best practices and regulatory requirements.
Interesting article, Timothy! I wonder if there have been any instances where ChatGPT's predictions differed significantly from traditional models. How do you handle conflicting results?
Thank you, Liam! In some cases, ChatGPT's predictions have exhibited significant differences from traditional models, highlighting its unique approach. When faced with conflicting results, thorough analysis and evaluation are necessary to understand the underlying reasons and make informed decisions.
Timothy, your article sheds light on an exciting application of AI. Can ChatGPT be used for risk assessment in other industries beyond credit risk?
Hi Sophia! Absolutely, ChatGPT can have applications beyond credit risk. It can be utilized for risk assessment in various industries, such as insurance, healthcare, and cybersecurity. Its language processing capabilities make it versatile for analyzing and predicting risks.
Great article, Timothy! How user-friendly is ChatGPT for non-technical users? Can someone without a technical background leverage its capabilities?
Thank you, Elena! ChatGPT is designed to be user-friendly for non-technical users. While some technical knowledge is beneficial, efforts have been made to simplify the interface and make it accessible to a wider range of users.
Timothy, your article presents an exciting advancement in credit risk technology. Are there any specific challenges or limitations you faced during the development and implementation of ChatGPT?
Hi Oliver! Developing and implementing ChatGPT did present various challenges. Fine-tuning the model, handling diverse data sources, and ensuring reliability were among the key challenges. Additionally, addressing ethical considerations and regulatory requirements required extensive research and collaboration.
Timothy, your article highlights the potential of ChatGPT in credit risk forecasting. Are there any prerequisites or specific data requirements for implementing this technology?
Thank you, Emily! Implementing ChatGPT does require relevant data. The availability of historical credit risk data, market data, and other relevant variables is essential for training the model. Clean, representative, and well-structured data enhances the accuracy and reliability of the results.
Great read, Timothy! Can ChatGPT be trained using proprietary data from financial institutions, or is it limited to publicly available datasets?
Hi Daniel! ChatGPT can be trained using both publicly available datasets and proprietary data, including financial institutions' specific data. Utilizing proprietary data allows the model to learn from the institution's unique credit risk patterns, potentially improving its forecasting capabilities.
Fascinating article, Timothy! I wonder if ChatGPT can account for black swan events or extreme market conditions that are not captured in historical data. Is there any way to incorporate such events into the forecasting process?
Thank you, Emma! Accounting for black swan events is indeed a challenge. While historical data may not directly capture such extreme events, ChatGPT's ability to process textual data and external information can provide some insights. However, incorporating black swan events into the forecasting process remains an active area of research.
Timothy, your article brings up an interesting topic. Can ChatGPT consider qualitative and unstructured data in credit risk forecasting, or is it limited to quantitative inputs?
Hi Sophie! ChatGPT can consider qualitative and unstructured data, such as textual information, news articles, or expert opinions, alongside quantitative inputs. This flexibility allows for a more comprehensive view of credit risk factors and can improve forecasting accuracy.
Great article, Timothy! How does ChatGPT handle outliers or anomalies in the data? Does it account for potential data quality issues?
Thank you, Grace! ChatGPT considers outliers and anomalies in the data to an extent. However, extensive preprocessing and data cleaning are necessary to ensure that potential data quality issues are addressed. Detecting and handling outliers is an ongoing area of improvement for the model.
Timothy, your article explores an innovative approach. How do you validate and monitor the performance of ChatGPT in real-world credit risk scenarios?
Hi Daniel! Validating and monitoring ChatGPT's performance in real-world credit risk scenarios is crucial. We conduct thorough backtesting, comparing model predictions with actual outcomes. Ongoing monitoring and iteration help refine the model's performance and address any detected issues.
Interesting article, Timothy! How widely adopted is ChatGPT in the credit risk industry? Do you see increasing adoption in the coming years?
Thank you, Sophie! ChatGPT is still in the early stages of adoption in the credit risk industry. While the interest and potential are high, wider adoption will depend on factors like further research, regulatory considerations, and successful real-world implementations.
Timothy, your article sparks curiosity. What steps are taken to ensure the reliability and robustness of ChatGPT's predictions?
Hi Isabella! Reliability and robustness are paramount. Proper model validation, extensive testing, and ongoing monitoring are crucial to ensure the predictions are as accurate and reliable as possible. The model's performance is also continuously enhanced through feedback loops and iteration.
Thank you all for the engaging discussion and valuable insights! Your comments and questions have been thought-provoking, and I appreciate your interest in the application of ChatGPT in credit risk forecasting. Feel free to reach out if you have any further queries or observations.
Thank you, Timothy, for sharing your expertise and addressing our questions. This article has certainly expanded my knowledge on the potential of AI in credit risk technology. Keep up the great work!