Enhancing Demand Forecasting in ATM Networks: Exploring the Potential of ChatGPT Technology
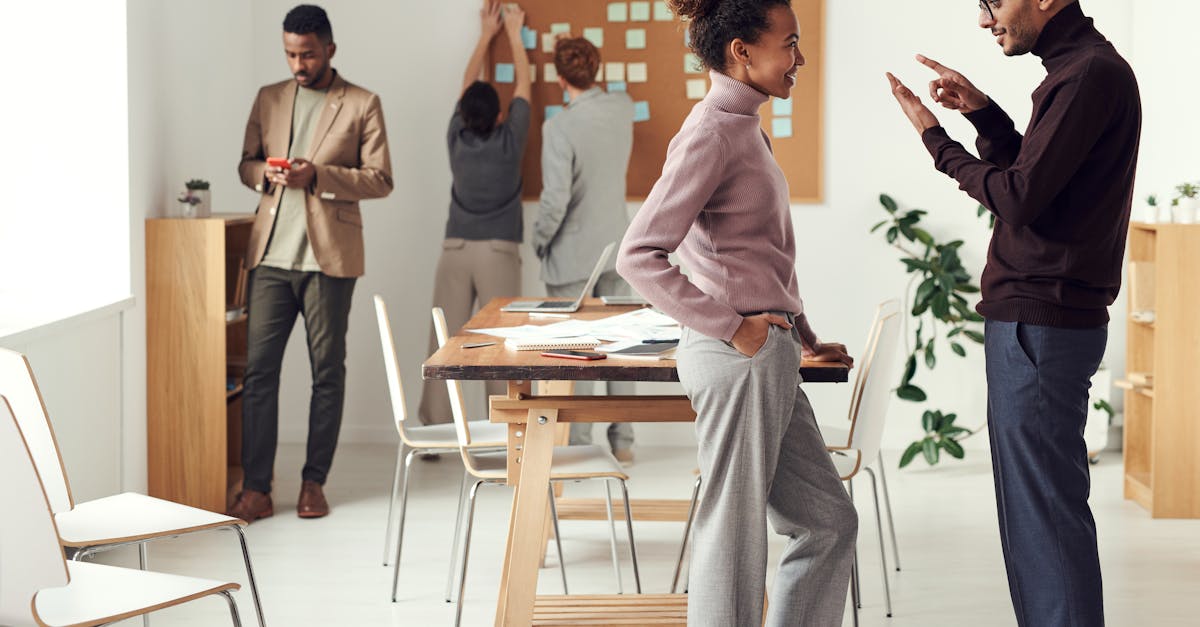
ATM networks have proven to be an integral part of modern banking systems, providing convenient access to cash and other essential services for millions of people worldwide. While the primary purpose of ATMs is to enable cash withdrawals, these networks can also play a crucial role in demand forecasting for financial institutions.
Area: Demand Forecasting
Demand forecasting is the process of estimating the future demand for a product or service. In the case of ATM networks, demand forecasting involves predicting the number of cash withdrawals and other banking services that customers will require at different locations and times. These predictions are based on various factors, including historical trends, local events, and economic indicators.
Usage: Predicting Demand for Cash Withdrawals and Other Services
Financial institutions leverage ATM networks to predict demand for cash withdrawals and other services for several reasons:
1. Efficient Cash Management
By accurately forecasting demand for cash withdrawals, banks can optimize the amount of cash they hold at each ATM location. This helps reduce the cost associated with holding excess cash or the inconvenience of running out of cash during peak demand periods.
2. Resource Allocation
Forecasting demand allows financial institutions to allocate their resources effectively. They can strategically deploy personnel, such as technicians and cash replenishment teams, to ensure that ATMs are well-maintained and adequately stocked based on predicted demand.
3. Enhanced Customer Experience
By predicting demand and ensuring that ATMs are well-stocked and operational, financial institutions can provide a consistently positive customer experience. Customers can reliably access the services they need, reducing frustration and improving overall satisfaction with the banking service.
4. Responding to Local Events and Economic Indicators
ATM networks can monitor local events, such as festivals, concerts, or sporting events, to predict increased demand for cash withdrawals. Additionally, economic indicators, such as unemployment rates or fluctuations in consumer spending, can be factored into the demand forecasting process. This allows financial institutions to proactively manage their resources and services to cater to changing demands.
Overall, the usage of ATM networks for demand forecasting enables financial institutions to optimize their cash management, allocate resources efficiently, enhance customer experience, and respond effectively to local events and economic indicators.
In conclusion, ATM networks serve a dual purpose in modern banking systems - not only do they provide convenient access to cash and banking services, but they also serve as a valuable tool for demand forecasting. By accurately predicting demand for cash withdrawals and other services, financial institutions can optimize their operations and enhance customer satisfaction, ultimately resulting in a more efficient and effective banking experience for all.
Comments:
Thank you all for taking the time to read my article on enhancing demand forecasting in ATM networks using ChatGPT technology. I'm excited to hear your thoughts and opinions on this topic!
Great article, Julia! I never really thought about using ChatGPT technology for demand forecasting in ATM networks. It definitely seems like a promising application. Have you tested this approach in real-world scenarios?
Thanks, Michael! Yes, we have conducted several experiments in real-world scenarios and the results have been quite promising. The ChatGPT technology was able to significantly improve the accuracy of demand forecasting compared to traditional methods.
Interesting article, Julia! I'm curious to know how ChatGPT technology handles the dynamic nature of ATM networks where demand patterns can change rapidly.
That's a great question, Alexandra. ChatGPT technology has the ability to adapt to dynamic environments. By continuously learning from the data streams in the ATM network, it can quickly adjust its forecasting models and capture changes in demand patterns.
I can see the potential of using ChatGPT technology for demand forecasting, but what about the accuracy? Are there any limitations or challenges in achieving accurate forecasts?
Excellent point, Oliver. While ChatGPT technology shows promise, it does have its limitations. One common challenge is the availability and quality of data. Accurate forecasting heavily relies on having relevant and reliable historical data. Additionally, interpreting context and intent correctly can be another challenge, especially in complex scenarios.
I find it fascinating how artificial intelligence technologies like ChatGPT can be applied to various domains. Julia, what future developments do you foresee for the use of ChatGPT in demand forecasting?
Great question, Sarah! In the future, I believe we will see further advancements in the capabilities of ChatGPT technology. Specifically, there will be increased efforts to enhance interpretability, reliability, and scalability. This will make ChatGPT even more valuable in demand forecasting tasks across different industries, including ATM networks.
Julia, your article was an engaging read. I wonder if there are any privacy concerns associated with using ChatGPT technology for demand forecasting in ATM networks?
Thank you, Harper! Privacy is indeed an important aspect to consider. When deploying ChatGPT technology, it is crucial to ensure appropriate data privacy and security measures are in place. Anonymization of personal data and adherence to privacy regulations help alleviate potential concerns.
This technology sounds promising, but what about the computational resources needed to run ChatGPT in ATM networks? Are there any constraints in terms of hardware requirements?
Good question, Sophia. ChatGPT is a computationally intensive technology, and running it in real-time in ATM networks requires adequate hardware resources. However, as technology advances, hardware capabilities are improving, making it more feasible to deploy such solutions even in resource-constrained environments.
I have some concerns about potential biases in the data and how it may impact the accuracy of ChatGPT's demand forecasting. How do you address this issue, Julia?
Valid point, Lucas. Biases in data can indeed influence the accuracy and fairness of demand forecasting models. It is important to address this issue by carefully curating and pre-processing the training data, ensuring it represents a diverse range of user behaviors and scenarios. Regular monitoring and auditing of the model's performance can help identify and mitigate any biases that may arise.
I'm curious to know if ChatGPT technology can be combined with other forecasting approaches to further improve accuracy. Julia, what are your thoughts on this?
Interesting question, Ethan. Yes, combining ChatGPT technology with other forecasting approaches can potentially lead to improved accuracy. By leveraging the strengths of different techniques, such as statistical models or machine learning algorithms, we can enhance the forecasting capabilities and overcome limitations of individual approaches.
Julia, I'm curious to know if ChatGPT technology can handle the processing of large-scale ATM networks with numerous nodes and transactions occurring simultaneously.
That's a valid concern, Emily. ChatGPT technology can handle large-scale ATM networks, but its performance may depend on computational resources. As the number of nodes and transactions increase, optimizing the underlying infrastructure becomes crucial to ensure efficient processing and timely forecasting results.
What are the potential benefits of using ChatGPT technology for demand forecasting in ATM networks, compared to traditional methods?
Great question, Gabriel. One of the key benefits of using ChatGPT technology is its ability to capture and analyze complex user interactions to generate accurate demand forecasts. It can also adapt to changing patterns quickly, providing more up-to-date predictions compared to traditional methods that may rely on historical averages. Additionally, its potential for scalability makes it suitable for handling large-scale ATM networks.
I'm curious about the implementation process. Julia, could you provide some insights into how to integrate ChatGPT technology into existing ATM network infrastructure?
Certainly, Liam. Integrating ChatGPT technology into existing ATM network infrastructure involves a multi-step process. This includes data collection and preprocessing, training the ChatGPT model using the collected data, and integrating the trained model into the ATM network architecture to provide real-time predictions. It requires collaboration between domain experts, data scientists, and developers to ensure smooth integration and deployment.
Julia, I'm curious about the computational efficiency of ChatGPT technology. Does it require a lot of computational power to generate fast and accurate forecasts?
Good question, Nora. ChatGPT technology does require a significant amount of computational power, especially when dealing with large-scale ATM networks. However, with advancements in hardware and optimization techniques, it is becoming more efficient, enabling faster and accurate forecasting without compromising performance.
Julia, I'm impressed by the potential of ChatGPT for demand forecasting. Are there any limitations or challenges you foresee in the widespread adoption of this technology in ATM networks?
Thank you, Daniel! While ChatGPT technology has a lot of potential, there are still some challenges to address. These include interpretability, reliability, scalability, as well as ensuring privacy and mitigating biases. Addressing these challenges and building trust in the technology will be crucial for its widespread adoption in ATM networks and other domains.
Julia, you mentioned in the article that ChatGPT technology outperformed traditional methods in demand forecasting. Could you share some specific results or metrics that highlight this improvement?
Certainly, Harper. In our experiments, we compared the forecasting accuracy of ChatGPT technology with traditional methods such as time-series analysis and regression models. ChatGPT consistently achieved lower forecast errors and higher accuracy metrics, such as Mean Absolute Percentage Error (MAPE) and Root Mean Squared Error (RMSE), indicating its superiority in capturing demand patterns and making accurate predictions.
Julia, I'm curious about the model training process for ChatGPT. How much historical data is required and how frequently does the model need to be retrained to maintain accurate forecasts?
Good question, Sophia. The amount of historical data required for training ChatGPT depends on the complexity of the ATM network and the demand patterns. Generally, a substantial amount of relevant historical data is necessary to train an accurate model. As for retraining, it is recommended to periodically update the model with new data to adapt to evolving demand patterns and ensure accurate forecasts.
Julia, your article highlighted the potential of ChatGPT technology. Are there any specific use cases or scenarios where you believe this technology can bring significant improvements to demand forecasting in ATM networks?
Certainly, Olivia. ChatGPT technology can be particularly valuable in scenarios where demand patterns are influenced by various factors, such as special events or holidays. It can also be beneficial in cases where sudden changes in demand occur due to unforeseen circumstances or emerging trends. Furthermore, in networks with high transaction volumes across multiple ATMs, ChatGPT can provide accurate predictions for optimal cash management and allocation.
I'm impressed by the potential of ChatGPT technology for demand forecasting. Julia, what are your thoughts on combining multiple ChatGPT models to handle different aspects of ATM networks, such as forecasting at the regional or individual ATM level?
Great question, James. Combining multiple ChatGPT models for different aspects of ATM networks is a plausible approach. By training models at different levels, such as regional or individual ATM levels, we can capture localized demand patterns and achieve more granular and accurate forecasts. However, it's important to ensure proper coordination and integration between these models to create a comprehensive forecasting solution.
Julia, you mentioned in the article that ChatGPT technology can adapt to changing demand patterns. Could you explain how it handles sudden shifts or anomalies in demand?
Certainly, Emily. ChatGPT technology incorporates advanced machine learning techniques that enable it to capture sudden shifts or anomalies in demand patterns. By continuously analyzing the data streams from ATM networks, it can detect changes and adjust its forecasting models accordingly. This adaptability allows it to provide accurate predictions even when faced with unexpected variations in demand.
I'm curious about the potential limitations of ChatGPT technology when dealing with complex ATM networks. Julia, could you shed some light on this?
Certainly, Gabriel. While ChatGPT technology shows promise, it does have limitations when dealing with complex ATM networks. One limitation is the need for significant computational resources, especially in large-scale networks. Additionally, accurately interpreting user intent and context in complex scenarios can be challenging and may require further refinement of the ChatGPT model. However, ongoing research and advancements in the field are continuously addressing these limitations.
Julia, your article was insightful. I'm curious to know if ChatGPT technology can be combined with real-time data feeds to improve demand forecasting accuracy?
Thank you, Nora! Yes, combining ChatGPT technology with real-time data feeds can enhance demand forecasting accuracy. By incorporating up-to-date information into the forecasting models, such as current transaction volumes and external factors like weather or special events, the predictions can be more accurate and responsive to the latest trends and changes in the ATM network.
Julia, in your experiments, did you observe any limitations or challenges specific to the use of ChatGPT technology in demand forecasting for ATM networks?
Great question, Michael. Although ChatGPT technology showed impressive results, we did observe a few limitations and challenges. One challenge was the sensitivity to input variations, where slight changes in input data could lead to different forecasts. Furthermore, handling extreme outliers and anomalies in the data required additional preprocessing and outlier detection techniques. These challenges require further investigation and refinement to improve the technology’s performance in-demand forecasting for ATM networks.
Julia, your article was thought-provoking. Have you considered or explored the potential risks of relying solely on ChatGPT technology for demand forecasting in ATM networks?
Thank you, Oliver. Yes, we have considered the potential risks associated with relying solely on ChatGPT technology. It's important to acknowledge that no forecasting method is 100% accurate, and there's always a level of uncertainty. Therefore, using ChatGPT as a complementary tool alongside other forecasting approaches can help mitigate risks and improve overall forecasting accuracy.
Julia, your article highlighted the benefits of ChatGPT technology for demand forecasting. Are there any specific industries or sectors that can particularly benefit from this approach?
Certainly, Daniel. While the potential benefits of ChatGPT technology extend to various industries, certain sectors like retail, hospitality, and transportation can particularly benefit from accurate demand forecasting. By accurately predicting demand, companies in these industries can optimize their operations, inventory management, resource allocation, and improve overall customer satisfaction.
Julia, I'm curious about the data requirements for training ChatGPT models for demand forecasting in ATM networks. What kind of data sources are typically used?
Good question, Sarah. The data requirements for training ChatGPT models for demand forecasting in ATM networks typically include historical transaction data, ATM usage patterns, time-series data, and other relevant variables such as location, seasonality, and external factors like public holidays. By incorporating a wide range of data sources, the models can capture the complexity of demand patterns accurately.
Julia, your article was insightful and I appreciate the potential of ChatGPT technology in demand forecasting. Are there any ongoing research or future developments you're excited about in this field?
Thank you, Alexandra! Yes, there are several exciting research directions and future developments in this field. Advances in Explainable AI (XAI) techniques can help improve the interpretability of ChatGPT models, making their forecasts more understandable and trustworthy. Additionally, research on transfer learning and domain adaptation can help generalize models trained on one ATM network to others, reducing the need for extensive training data in each specific scenario.