Enhancing Digital Video Processing with ChatGPT: A Promising Application in Machine Vision Technology
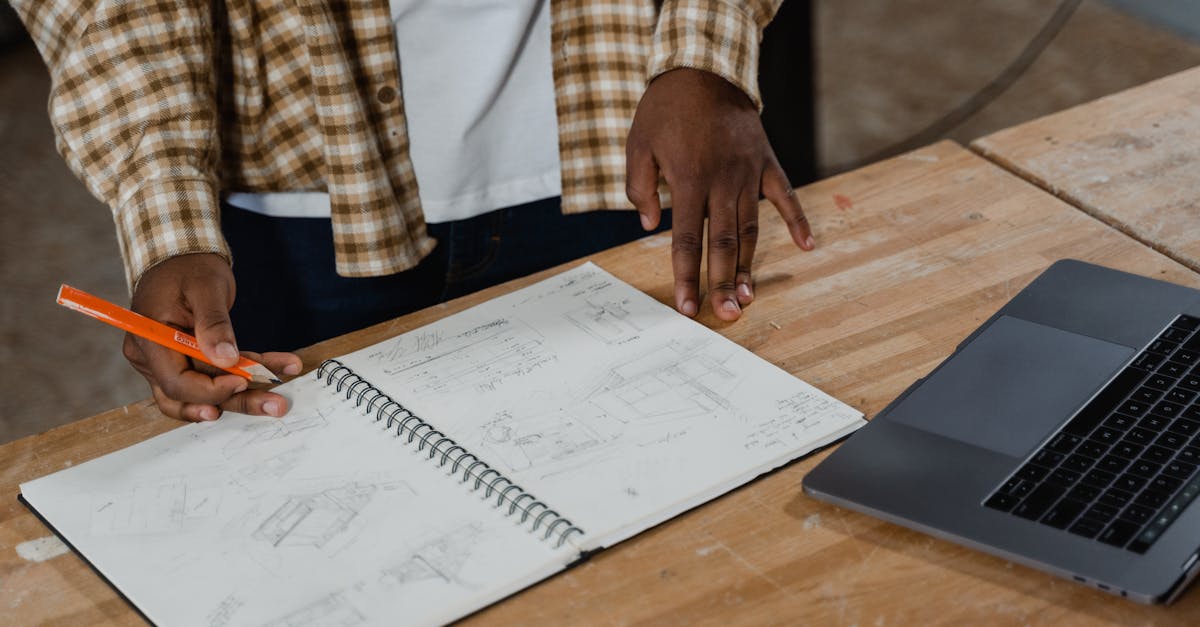
Machine vision, a subfield of artificial intelligence and computer vision, has gained significant attention and advancements in recent years. It involves the development and deployment of algorithms and technologies that enable computer systems to gain visual understanding from digital images or videos.
Understanding Machine Vision
Machine vision technologies are widely used in various industries, including manufacturing, surveillance, medical imaging, and more. These technologies enable computers to perform tasks that typically require human visual perception and interpretation.
One prominent area where machine vision has found practical applications is in digital video processing. With the increasing availability of video data from different sources, extracting meaningful information automatically has become crucial for tasks like activity recognition, anomaly detection, and content analysis.
Role of Machine Vision in Video Frame Labeling and Categorization
ChatGPT-4, a cutting-edge language model developed by OpenAI, can leverage machine vision techniques to assist in the labeling and categorization of elements from video frames. By combining natural language processing capabilities with machine vision, ChatGPT-4 can analyze and understand the visual content within video frames, providing valuable insights and automating labor-intensive processes.
Video frame labeling and categorization involve identifying and classifying objects, actions, or attributes present in individual frames of a video sequence. This process forms the basis for higher-level video analysis tasks, including activity recognition and anomaly detection. Traditionally, these tasks have been performed manually by human annotators, which is time-consuming, expensive, and prone to errors.
Using machine vision techniques, ChatGPT-4 can analyze video frames and identify relevant objects, activities, or patterns. The model can learn from vast amounts of labeled data, enabling it to recognize common objects, understand actions, and identify anomalies automatically. This greatly reduces the manual effort required for video analysis and accelerates the decision-making process.
Advantages and Applications
The integration of machine vision in digital video processing brings several advantages and opens up new possibilities:
- Efficiency: Machine vision algorithms can process video frames at a much higher speed than human annotators, making the analysis process more efficient and scalable.
- Consistency: With the use of predefined models and patterns, machine vision ensures consistent results across different video frames and datasets, minimizing subjective interpretations.
- Accuracy: Leveraging machine learning techniques, machine vision models continuously improve their accuracy as they are trained on larger datasets, leading to better recognition and categorization capabilities.
- Automation: By automating video frame labeling and categorization, machine vision reduces the need for manual intervention, allowing human experts to focus on more complex analysis tasks.
The applications of machine vision in video processing extend across various domains:
- Surveillance: Machine vision enables real-time monitoring and automated detection of suspicious activities or objects in surveillance footage. This assists in enhancing public safety and security.
- Manufacturing and Quality Control: Machine vision systems can assess the quality of products and processes by analyzing video feeds from production lines, ensuring compliance with standards and minimizing defects.
- Healthcare: Machine vision assists medical professionals by enabling automated detection of anomalies in medical imaging and video recordings, aiding in diagnostics and treatment decisions.
- Entertainment and Gaming: Machine vision algorithms can be used to enhance augmented reality and virtual reality experiences by providing real-time analysis of video feeds, creating immersive environments.
Conclusion
The incorporation of machine vision technologies in digital video processing, particularly in tasks like activity recognition and anomaly detection, brings significant advantages in terms of efficiency, accuracy, and automation. ChatGPT-4, with its integration of machine vision, progresses towards providing enhanced video frame labeling and categorization capabilities, empowering various industries to unlock the potential of video data.
Comments:
This article is quite interesting! I hadn't considered the potential of using ChatGPT for digital video processing in machine vision technology before. It could really revolutionize how we analyze and understand visual data.
I agree, Mark! The ability of ChatGPT to generate human-like responses can definitely enhance the way we process digital videos. It opens up exciting possibilities for various applications in the field of machine vision technology.
Thank you, Mark and Anna, for your positive feedback! I'm glad you're excited about the potential of using ChatGPT in this domain. Feel free to share any specific thoughts or questions you have.
In my opinion, one of the major advantages of using ChatGPT for digital video processing is the ability to handle complex contexts and generate meaningful insights. I'm interested to know more about its implementation and the challenges involved.
Definitely, Sara! The context-awareness of ChatGPT can greatly enhance the accuracy and quality of video analysis. It could potentially overcome limitations faced by traditional computer vision algorithms. I also wonder about any potential limitations or biases that need to be addressed.
That's an important point, Sara. Nell, could you provide some insights into the implementation challenges and how ChatGPT addresses them?
As an AI enthusiast, I find the idea of using ChatGPT for digital video processing very intriguing. It has the potential to bring more interpretability and explainability to the field of machine vision. Looking forward to further advancements!
Absolutely, Nathan! The transparency brought by ChatGPT can greatly aid in understanding the decision-making process behind video analysis algorithms. It empowers researchers and practitioners to delve deeper into the results.
Thank you, Nathan and Olivia, for sharing your thoughts! The interpretability aspect is indeed significant when it comes to machine vision technology. It can play a crucial role in building trust and ensuring ethical deployment of AI models.
I wonder if there are any specific domains or industries where ChatGPT's application in video processing has shown promising results. Nell, could you elaborate on that?
Good question, Sophie! It would be interesting to know if ChatGPT has been particularly effective in certain contexts, such as surveillance, autonomous vehicles, or medical imaging.
Certainly, Sophie and Henry! While ChatGPT's application in video processing is still being explored comprehensively, initial experiments have shown promising results in domains like video surveillance for enhanced anomaly detection, autonomous vehicle perception, and even medical imaging analysis for improved diagnostics.
That's fascinating, Nell! It seems like ChatGPT has a wide range of potential applications in diverse industries. The ability to adapt to different contexts makes it a versatile tool for video analysis.
I'm curious to know about the training process of ChatGPT for video processing tasks. How is it trained and what kind of datasets are used?
Great question, Ethan! Training ChatGPT effectively for video processing does require large-scale datasets consisting of videos with corresponding annotations or labels. Labeling such datasets accurately and consistently can be a challenge, and it often involves human experts who carefully annotate the visual data.
Thanks for the insight, Nell! It's crucial to ensure accurate labeling for training data to enable reliable video analysis results. Human experts playing a role in the annotation process is understandable, considering the complexity of video understanding.
I appreciate your explanation, Nell! The importance of carefully annotated datasets for training becomes even more evident when dealing with video data due to its rich and dynamic nature.
That's an interesting question, Ethan! Training ChatGPT for video processing could require a large and diverse dataset. I wonder if there are any particular challenges in curating such datasets.
I'm impressed by the potential impact of ChatGPT in the field of machine vision. However, are there any concerns or risks associated with relying heavily on AI models like ChatGPT for critical tasks?
That's a valid concern, Victoria. While AI models like ChatGPT have incredible potential, we must be cautious about potential biases, lack of generalization, and adversarial attacks that may impact their reliability in critical tasks.
Thank you, Victoria and Ashley, for raising this important point! As with any AI model, ensuring ethical development, rigorous testing, and continuous monitoring are crucial to mitigate risks associated with relying on AI models for critical tasks. Transparency and interpretability can also aid in building trust.
It's good to see that the potential risks are being acknowledged, Nell. In critical domains like healthcare, where lives may be at stake, it becomes even more vital to address biases and ensure the reliability of the AI models.
Absolutely, Daniel. Ensuring fairness, robustness, and accountability should be key priorities when deploying AI models like ChatGPT in critical domains. Collaborative efforts between AI researchers, domain experts, and policymakers can help address these concerns effectively.
I'm curious about the computational requirements when using ChatGPT for video processing. Do you need powerful hardware setups, or is the processing power reasonable?
That's a valid question, Timothy! The computational requirements largely depend on the scale and complexity of the video processing tasks. While powerful hardware setups can expedite processing, advancements in AI hardware accelerators have made it possible to perform video analysis efficiently on a range of systems.
Thank you for the clarification, Nell! It's encouraging to know that ChatGPT can potentially be utilized on various hardware setups, making it more accessible for researchers and practitioners.
Nell mentioned the need for human experts in the annotation process. How do you envision the collaboration between AI models like ChatGPT and human experts in the field of machine vision?
That's an important aspect to consider, Daniel. The collaboration can involve human experts validating and fine-tuning the results generated by AI models. They can also ensure the models are aligned with domain-specific knowledge and requirements to enhance the practicality of the video analysis.
I agree, Sophia. The combination of AI models' capabilities with human expertise can lead to more accurate and reliable video analysis. It's a collaborative approach that maximizes the strengths of both humans and machines.
Could natural language processing techniques be integrated with ChatGPT to enable the extraction of relevant information from videos?
Great question, Sara! Natural language processing techniques can indeed complement ChatGPT in video analysis. By combining the visual understanding capabilities of ChatGPT with linguistic analysis, it's possible to extract and summarize relevant information from videos, improving their interpretability.
Thanks for the response, Nell! It's fascinating to think about the possibilities of combining visual and linguistic analysis to unlock deeper insights from video data.
Absolutely, Sara! The integration of both modalities can provide a more comprehensive understanding of videos and enable applications like video captioning, video summarization, or even generating detailed analytics reports based on the extracted information.
Nell, I'm curious about the limitations of using ChatGPT for video processing. Are there any specific scenarios where its performance might struggle?
Good question, Lucas! While ChatGPT shows promise, it may struggle with real-time video processing due to its computational requirements. Additionally, in highly context-dependent videos with ambiguous or complex scenes, the model's responses might require further manual validation. Ongoing research aims to address these limitations and improve performance.
Nell, what steps are being taken to address potential biases in the generated responses of ChatGPT during video processing?
Thank you for the question, Isabella! Bias mitigation techniques play a crucial role in ensuring fairness and reducing biases in AI models' responses. Efforts are being made to identify and correct biases in training datasets, increase diversity, and provide clear guidelines to human annotators to minimize biased annotations.
That's reassuring, Nell. It's important to strive for unbiased and inclusive video analysis results to ensure equitable outcomes across various demographics and contexts.
I appreciate your response, Nell. It's reassuring to know that steps are being taken to address the concerns associated with deploying AI in critical tasks. Responsible AI development is crucial for the widespread adoption of these technologies.
Indeed, Victoria! Responsible and ethical AI development is essential for building trust and realizing the full potential of AI technologies. Continual improvements and community collaboration are key in advancing the field and addressing the challenges along the way.