Enhancing Disease Detection in Forestry: Leveraging ChatGPT for Advanced Technological Solutions
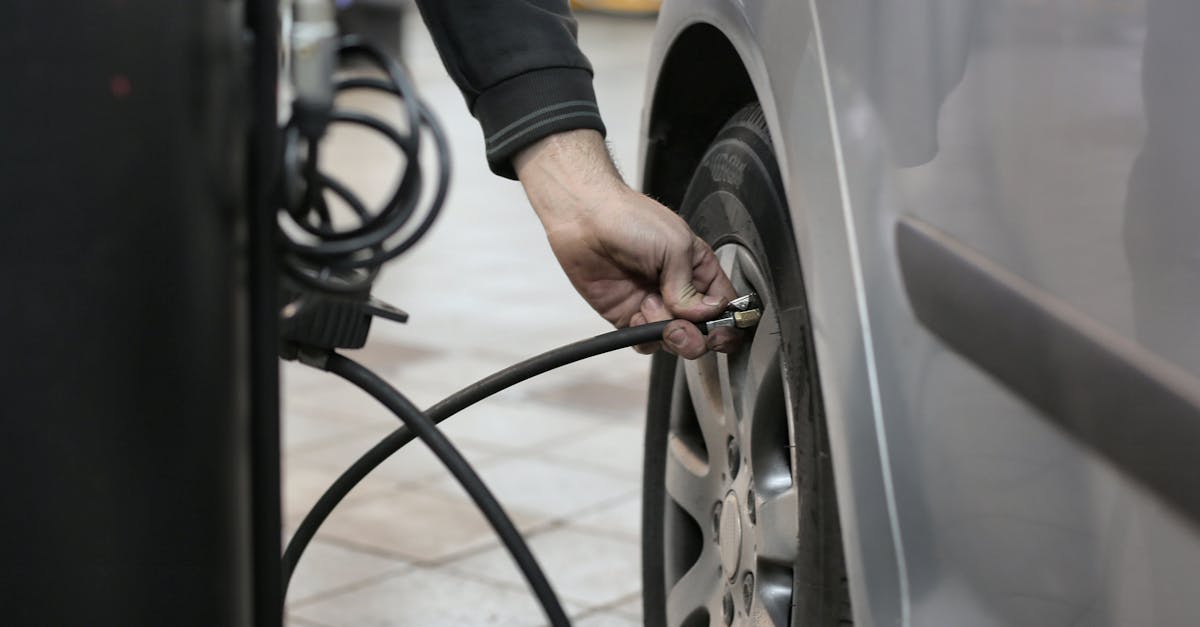
The advancement of technology has greatly benefited various sectors, including the field of forestry. One significant application of technology in this area is disease detection in trees or plants. By utilizing cutting-edge methods and analyzing visual signals or other indicators, it is now possible to identify and prevent the spread of diseases, ultimately preserving the health and vitality of our forests.
Technology
The technology used for disease detection in forestry involves the implementation of computer vision techniques, machine learning algorithms, and various sensors. Computer vision enables the extraction of meaningful information from images or videos of trees and plants. Machine learning algorithms, such as deep learning models, are trained on large datasets to recognize patterns and identify indications of different diseases.
In addition to visual signals, other indicators, including hyperspectral imaging, thermal imaging, and acoustic data, can also be used in disease detection. Hyperspectral imaging captures detailed spectral data to identify specific changes in plant physiology caused by diseases or stress. Thermal imaging detects variations in temperature, which can point to the presence of diseases. Acoustic data analysis focuses on the sounds produced by trees or plants, as certain diseases alter the acoustic properties.
Area: Disease Detection
The specific area of application for this technology is disease detection in forestry. Diseases can severely impact the health and productivity of trees and plants, leading to economic losses and ecological imbalances. Traditional methods of disease detection often rely on human observation, which is subjective, time-consuming, and prone to errors. By deploying high-tech solutions, we can enhance disease detection accuracy, efficiency, and speed.
Usage
The model developed for disease detection in forestry has various practical uses. It can be employed by forest managers, researchers, and government agencies responsible for monitoring and maintaining the health of forests.
One usage scenario involves periodic surveys of forests to identify and diagnose diseases in trees or plants. With the aid of drones or other imaging tools, large areas can be efficiently scanned, and potential disease outbreaks can be quickly detected. By pinpointing affected areas, prompt action can be taken to prevent the spread of diseases, such as targeted treatments or removal of infected trees.
Furthermore, the disease detection model can be integrated into smart forestry systems. These systems utilize various sensors placed throughout the forest to monitor environmental conditions, including temperature, humidity, and light intensity. By combining data from environmental sensors with disease detection algorithms, early warning systems can be developed to alert forest managers of potential disease outbreaks based on predictive models.
Another potential usage is in research and development. By analyzing the data collected from disease detection efforts, researchers can gain insights into the patterns and dynamics of diseases affecting forests. This knowledge can contribute to the development of effective prevention and control strategies, as well as the improvement of overall forest management practices.
Conclusion
The incorporation of technology into disease detection in forestry has revolutionized the way we safeguard the health of our trees and plants. By leveraging computer vision, machine learning, and various sensors, we can accurately and swiftly identify diseases, enabling timely actions to prevent their spread. The technology's usage in forestry extends beyond disease detection, with applications in monitoring, research, and overall forest management. As we continue to advance our technological capabilities, we can ensure the preservation and sustainability of our precious forest resources.
Comments:
This article is really interesting! I never thought about using chatbots for disease detection in forestry. It seems like a great use of technology to help protect our forests.
I agree, Laura. Leveraging ChatGPT for disease detection in forestry can significantly speed up the identification process and ensure earlier intervention to prevent the spread of diseases. It could be a game-changer.
I'm skeptical about relying solely on chatbots for disease detection. Can they really be accurate in identifying various diseases in trees? I think human expertise will still be necessary.
That's a valid concern, Sarah. While it's true that human expertise is irreplaceable, chatbots can be an effective tool to assist experts. They can help analyze data, identify potential issues, and prioritize areas for further inspection. It's a combination of human expertise and technological support.
Thank you for your comments, Laura and Mark. Indeed, chatbots are not meant to replace human expertise but to augment and streamline disease detection processes. They can provide valuable insights and support forest health professionals in making informed decisions.
I'm curious about the accuracy of ChatGPT in this context. Has it been extensively tested and proven effective in detecting diseases in trees?
That's a good question, Chris. It would be great to hear more about the research and testing behind the use of ChatGPT in disease detection for forestry.
Chris and Laura, excellent points. ChatGPT has indeed undergone rigorous testing to ensure its accuracy in detecting diseases in trees. It has shown promising results in initial studies, but further research and refinement are ongoing. Transparency and collaboration among researchers, experts, and the AI community play a crucial role in advancing this technology.
I'm worried that relying on AI for disease detection may lead to a decrease in field work and hands-on experience. It's important to find the right balance between technology and practical knowledge.
I share that concern, Michael. Field work and practical experience are essential in understanding the complexity of forests. Technology can support our efforts, but it should never replace the insights gained from being directly in the field.
Michael and Sarah, you're absolutely right. Technology should always complement field work, not replace it. The aim is to enhance disease detection capabilities and empower experts with tools that can aid their decision-making process without diminishing the importance of firsthand experiences.
This reminds me of how technology now plays a role in various sectors, including agriculture and healthcare. It's exciting to see innovative solutions being incorporated into forestry as well!
I agree, Alice. Embracing technological advancements can have a tremendous positive impact on the future of forestry. It opens up new possibilities and allows us to tackle challenges more effectively.
With the increasing threat of diseases to our forests, it's essential to explore every possible solution. ChatGPT for disease detection seems like a step in the right direction. Kudos to the researchers and professionals working on this!
Thank you for your support and enthusiasm, Laura. Your words resonate with the passion shared by everyone involved in bettering the future of our forests. Together, we can make a difference.
One of the benefits of leveraging AI for disease detection is the ability to process large amounts of data quickly. It can help identify patterns and trends, enabling timely interventions. This efficiency is crucial in forest management.
That's true, David. The vast amount of data in forestry can be overwhelming for human analysis alone. AI can assist in analyzing and extracting valuable insights from that data in a faster and more efficient manner.
David and Sarah, you've highlighted a key advantage of using AI in disease detection - its ability to process and analyze large datasets. This empowers forest health professionals with valuable information to make data-driven decisions in a timely manner.
The collaboration between AI technology and forestry professionals is exciting. It allows us to harness the power of these advanced tools while preserving the expertise and knowledge gained through years of dedicated work.
Well said, Emily! The collaboration between AI and forestry professionals is at the core of developing effective and sustainable solutions. By combining expertise, experience, and technology, we can make significant progress in protecting our forests.
I wonder if there are any ethical considerations in using AI for disease detection in forestry. What are the potential risks and limitations?
Ethical considerations are indeed crucial when working with AI technology. Some considerations include data privacy, bias in algorithms, and ensuring transparency in decision-making processes. We must foster a responsible and accountable AI ecosystem while mitigating risks.
It's important that AI tools like ChatGPT are developed ethically and used responsibly. By being aware of these considerations, we can ensure that technological advancements bring positive impacts without causing harm.
I completely agree, Laura. Ethics should always be a core focus in the development and deployment of AI technologies. It's crucial to strike the right balance between innovation and responsible use.
As a forest health professional, I'm excited about the potential of AI in disease detection. It can provide valuable support in managing and preserving our forests. However, it's essential to remain vigilant and regularly evaluate the accuracy and effectiveness of these technologies.
That's an important point, James. Continuous evaluation and improvement are necessary to ensure that AI tools stay relevant and reliable in disease detection.
Thank you, James and Sarah, for sharing your expertise. Regular evaluation and improvement are indeed crucial to adapt and enhance AI tools for disease detection as new challenges arise in forestry systems.
I'm impressed by the potential of AI in disease detection, but I hope there will be widespread access to such technologies for all forest health professionals, regardless of their resources.
That's a valid concern, Emma. Accessibility is essential for equitable use of AI in disease detection. Efforts should be made to ensure that these technological solutions are available and affordable to all forest health professionals.
Absolutely, Laura and Emma. Accessibility should be a priority, and collaborations are necessary to ensure the adoption and availability of AI tools in disease detection to support all forest health professionals.
I wonder if there are any other potential applications for AI in forestry, beyond disease detection. It seems like there could be more areas where technology can make a difference.
You're right, John. AI has the potential to revolutionize various aspects of forestry, such as forest management, resource allocation, and even predicting forest fire risks. There's still so much to explore!
Indeed, John and Sarah. The application of AI in forestry extends beyond disease detection. Forest management, resource optimization, and predictive analysis for natural hazards are just a few areas where technology can bring significant benefits.
AI tools are undoubtedly powerful, but it's crucial to ensure that they don't replace human jobs. Balancing technological advancements with the preservation of jobs and expertise is essential.
I agree, Michael. It's important to view AI as a tool to enhance human capabilities rather than a replacement. Collaborative efforts between technology and human expertise can lead to better outcomes for both forests and professionals.
Well said, Laura. The optimal integration of AI tools into forestry operations can empower professionals, improve efficiency, and contribute to better forest health management.
AI-driven disease detection can potentially help prevent the spread of diseases across forests and protect biodiversity. It's exciting to think about the positive impact it can have on our ecosystems.
You're absolutely right, Emily. Early and accurate disease detection is crucial for preventing large-scale outbreaks and preserving the health of our forests.
Thank you, Emily and Sarah. Protecting biodiversity and mitigating the impact of diseases on ecosystems are among the key goals for AI-driven disease detection in forestry.
I appreciate this discussion and the insights shared. It shows the potential of AI in forestry while emphasizing the importance of maintaining human expertise and ethical considerations.
Thank you, Oliver. I'm glad this discussion has highlighted the intertwined nature of AI and human expertise, reinforcing the need for responsible and collaborative approaches in advancing disease detection technologies for forestry.