Enhancing Documentation Efficiency: Harnessing the Power of ChatGPT in DFT Technology
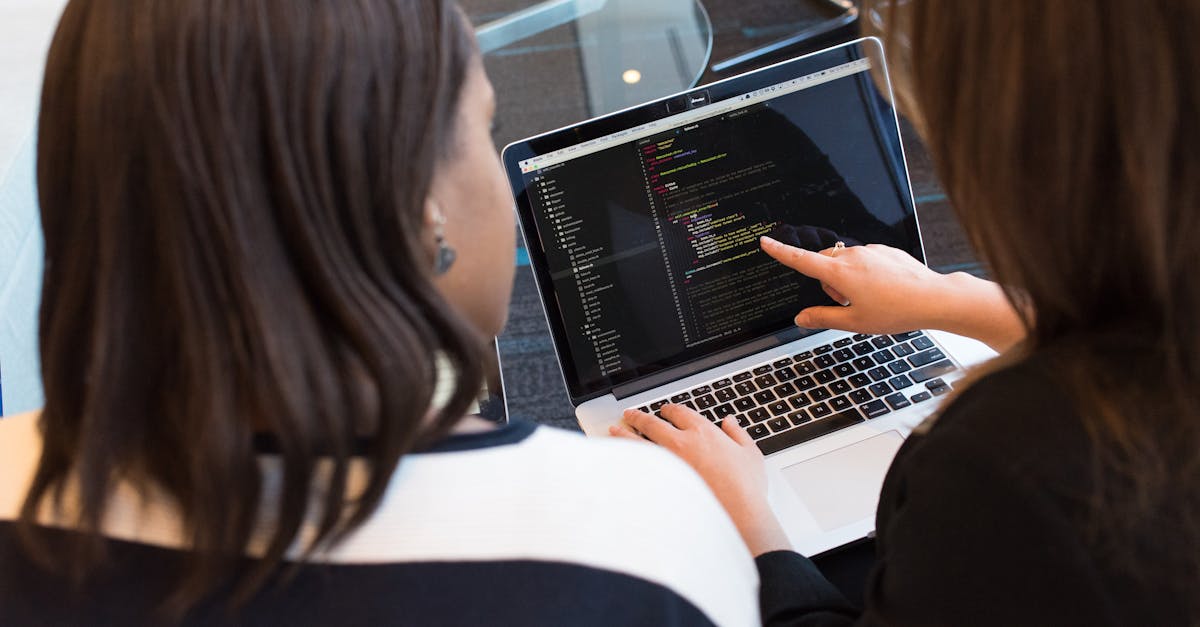
Documentation plays a crucial role in the field of Design for Test (DFT) technology. It ensures that the knowledge and information related to the design, verification, and testing of integrated circuits (ICs) is effectively communicated to engineers, designers, and other stakeholders. However, manual documentation processes can be time-consuming, error-prone, and resource-intensive.
In recent years, the advancement of Natural Language Processing (NLP) and Artificial Intelligence (AI) technologies has led to the development of powerful language models, such as ChatGPT-4. These models can understand and generate human-like text, making them useful for automating various tasks, including DFT documentation processes.
What is DFT?
Design for Test (DFT) is a set of techniques and methodologies used in the design and manufacturing of ICs to ensure their testability and detect potential defects or faults. DFT technology includes various stages, such as test planning, test generation, test application, and test analysis. These processes generate a significant amount of documentation that needs to be maintained and updated regularly.
Challenges in DFT Documentation
Manual documentation in the field of DFT poses several challenges. Firstly, it requires a deep understanding of the domain-specific terminology, concepts, and methodologies. This can be a barrier for engineers who are not well-versed in DFT technology.
Secondly, manual documentation processes are time-consuming and can take away valuable resources from other critical tasks. Engineers spend considerable time researching, organizing, and aligning the content in a structured manner, resulting in a slower documentation cycle.
Lastly, errors in documentation can lead to misinterpretation, misunderstandings, and potential issues during the IC design and manufacturing process. Ensuring accuracy and consistency in documentation can be challenging, especially when different engineers are involved in the process.
Automation with ChatGPT-4
ChatGPT-4, powered by OpenAI, is a state-of-the-art language model that can understand and generate human-like text. It leverages its vast knowledge base and contextual understanding to assist in automating DFT documentation processes.
By utilizing ChatGPT-4, DFT engineers can benefit from the following features:
- Generating structured and coherent documentation: ChatGPT-4 can generate well-structured documentation by understanding input queries and producing informative and accurate responses. Its ability to interpret technical language and knowledge can ensure the generation of high-quality documentation.
- Reducing the documentation cycle: With ChatGPT-4's assistance, engineers can save valuable time by automating repetitive documentation tasks. The model can provide instant responses, suggestions, and examples, reducing the overall time required for creating comprehensive documentation.
- Improving accuracy and consistency: ChatGPT-4 can help maintain the accuracy and consistency of documentation by offering suggestions, identifying potential errors, and providing clarifications. This reduces the likelihood of misinterpretation and misunderstandings among stakeholders.
- Enhancing collaboration and knowledge sharing: ChatGPT-4 can act as a virtual assistant, helping engineers work collaboratively in a shared documentation environment. It can provide real-time suggestions, offer insights, and facilitate knowledge exchange among team members.
Conclusion
Automation through ChatGPT-4 can revolutionize the way DFT documentation processes are conducted. It enables engineers to streamline their workflow, save time, and improve the quality and consistency of documentation. By leveraging the power of AI and NLP technologies, DFT engineers can focus more on critical tasks and achieve greater efficiency in their work.
As DFT technology continues to evolve, the use of intelligent language models like ChatGPT-4 will play a crucial role in simplifying complex documentation processes and enhancing collaboration among engineers in this field.
Comments:
Thank you all for taking the time to read my article on enhancing documentation efficiency with ChatGPT in DFT technology. I'm excited to hear your thoughts and discuss further!
Great article, Gary! I found it interesting how ChatGPT can be applied in DFT. It seems like it has the potential to revolutionize the documentation process.
I agree, Roberta. ChatGPT can greatly improve efficiency and accuracy in documentation. It can save a lot of time and effort.
I have some concerns though. How reliable is ChatGPT in understanding and documenting complex technical information? Has it been extensively tested?
Good question, Jessica. ChatGPT has been trained on diverse data and can handle a wide range of topics, including technical information. However, it is always advisable to review and verify the generated content for accuracy.
I've actually used ChatGPT in my work, and while it performs well in most cases, there are instances where it struggles with technical jargon and context. It's important to have some human intervention in the verification process.
Absolutely, Patrick. Automation tools like ChatGPT can assist in documentation, but human involvement is essential for quality assurance.
Thanks, Patrick, for sharing your practical experience. It's reassuring to know that while ChatGPT can be helpful, it still requires human intervention for accurate technical documentation.
I think ChatGPT has the potential to significantly speed up the documentation process. I can imagine it being a game-changer for organizations dealing with large volumes of documentation.
Indeed, Nathan. ChatGPT can handle repetitive tasks and free up time for professionals to focus on more critical aspects of their work.
Nathan, I agree with you. ChatGPT's potential for speeding up documentation processes is exciting. It could greatly improve overall efficiency and productivity.
I can see the potential benefits of ChatGPT, but there are also concerns regarding data privacy and security. How is the information processed by ChatGPT protected?
Great point, Daniel! When using ChatGPT or any other AI models, it's crucial to ensure data privacy and security. Access controls and encryption methods should be implemented to protect sensitive information.
Gary, are there any limitations to using ChatGPT in DFT technology? Are there specific scenarios where it might not be the most effective solution?
Good question, Emily. While ChatGPT is powerful, it may struggle with highly specialized domains or unique technical challenges that require contextual understanding beyond its training data. In those cases, domain-specific AI models can be advantageous.
That's true, Gary. Having specialized models for complex, niche domains can provide more accurate and context-aware responses.
Emily, another limitation is the potential for bias in AI-generated content. It's important to regularly audit and address any biases that may arise from using ChatGPT for documentation.
You're right, Matthew! Bias detection and mitigation should be a part of the documentation process to ensure fairness and inclusivity.
Gary, I have one final question. Are there any ethical considerations organizations should be aware of when using AI models like ChatGPT in DFT technology?
Excellent question, Daniel! Organizations need to ensure transparency, accountability, and fairness in the use of AI models. They must also address concerns related to biases, data privacy, and potential job displacement caused by automation.
In addition to data protection measures, organizations can also consider using on-premises versions of ChatGPT or implementing secure cloud solutions to further enhance privacy and control.
When it comes to data privacy, it's important to have clear policies in place to govern access, storage, and usage of data. Compliance with relevant regulations and guidelines is crucial.
Exactly, Laura. Data governance and compliance should be prioritized to ensure the responsible and ethical use of AI models like ChatGPT.
I appreciate all the valuable insights and questions shared so far. Is there anything else you'd like to discuss or any additional thoughts on leveraging ChatGPT in DFT technology?
Gary, what about the challenges associated with training ChatGPT specifically for DFT technology? Does it require a significant amount of domain-specific data?
Good point, Lucas. Training ChatGPT for domain-specific applications like DFT technology does require a sufficient amount of relevant data. Access to high-quality, curated data is crucial for achieving optimal performance.
Gary, what steps can organizations take to ensure they have the necessary data for training ChatGPT effectively?
Sophie, organizations can start by identifying their specific data needs and sourcing relevant data from various internal and external sources. Data augmentation techniques can also be employed to increase the training data volume.
Gary, how important is the involvement of subject matter experts in training ChatGPT for domain-specific applications?
Subject matter experts play a critical role in training ChatGPT for domain-specific applications. They can provide expertise, guidance, and assistance in curating and annotating the training data to ensure its quality and relevance.
Gary, overall, I believe the integration of ChatGPT in DFT technology has the potential to revolutionize the way we handle documentation. It's an exciting development!
I share your excitement, Roberta! The advancements in AI technology, like ChatGPT, hold immense potential in transforming documentation workflows for the better.
Involving subject matter experts ensures that the AI model accurately captures the nuances and intricacies of the domain, leading to more reliable and context-aware documentation.
It's crucial to have ethical guidelines and policies in place to guide the responsible and ethical application of AI models, like ChatGPT, in the DFT industry.
Ensuring ongoing monitoring and auditing of AI systems can help identify and address any ethical concerns that may arise in real-world usage.
Thank you all for engaging in this insightful discussion. Your thoughts and questions have been valuable in exploring the potential and challenges of using ChatGPT in DFT technology!
I thoroughly enjoyed reading your article, Gary. It sheds light on the exciting possibilities that ChatGPT offers in the realm of documentation efficiency.
This article has given me a fresh perspective on how advanced AI models like ChatGPT can enhance productivity and streamline the documentation process in the DFT industry.
Gary, your article perfectly illustrates how ChatGPT's capabilities can have a transformative impact on the documentation challenges faced by professionals in the DFT sector.
Thank you, Olivia, Benjamin, and Harry, for your kind words. I'm glad you found the article informative and thought-provoking!