Enhancing Education with ChatGPT: Exploring the Power of Predictive Analytics Technology
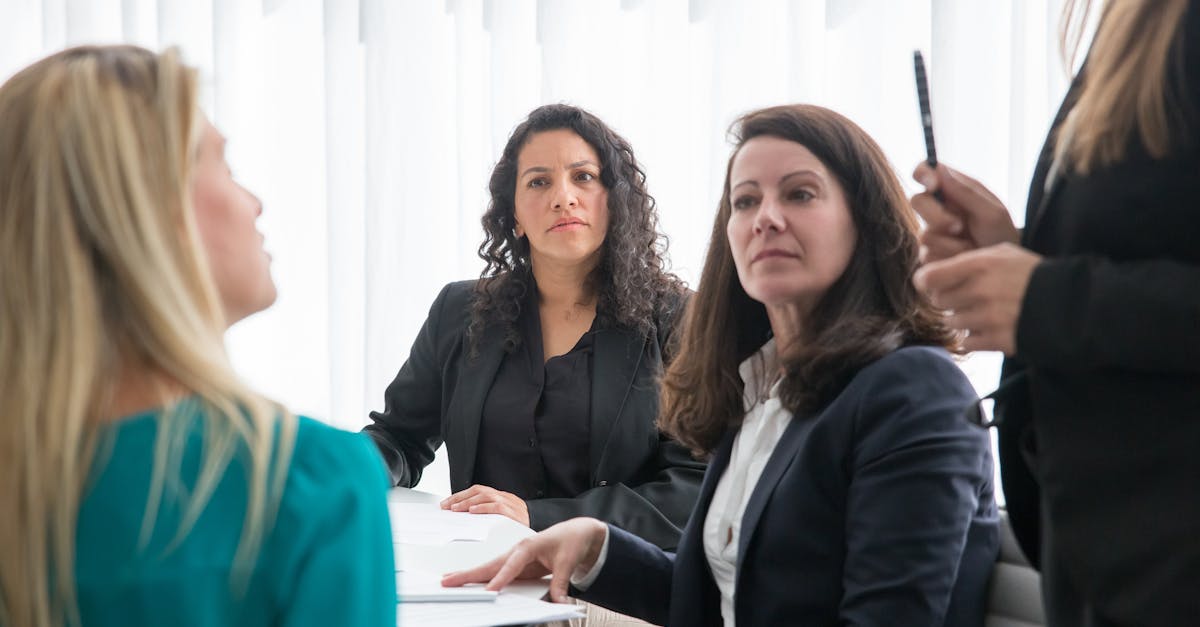
Predictive analytics, a technology that leverages data, statistical algorithms, and machine learning techniques, is making significant strides in the education sector. By analyzing patterns and trends from vast amounts of student data, predictive analytics can provide valuable insights into student performance, helping educators devise better and personalized learning plans.
How Predictive Analytics Works in Education
Using predictive analytics in education involves collecting and analyzing various types of data, such as student demographics, academic records, attendance, and engagement levels. These data points are combined and processed using advanced algorithms to identify trends, correlations, and hidden patterns.
Based on the analyzed data, predictive analytics models can then anticipate a student's future performance, including their likelihood of success or failure in particular subjects or courses. This information helps educators identify students who may require additional support or intervention to improve their academic outcomes.
Benefits of Using Predictive Analytics in Education
Predictive analytics offers several benefits in the field of education:
- Early Intervention: By identifying at-risk students early on, predictive analytics enables educators to intervene and provide targeted support before students fall behind.
- Personalized Learning: Predictive analytics can help educators develop personalized learning plans tailored to each student's strengths, weaknesses, and learning style. This individualized approach enhances student engagement and improves overall academic performance.
- Resource Allocation: With predictive analytics, educational institutions can allocate their resources more effectively. By identifying common problem areas and student needs, institutions can optimize the allocation of teaching staff, materials, and funding.
- Evidence-Based Decision Making: Predictive analytics enables educators to make data-driven decisions based on objective insights. By relying on evidence rather than intuition, educational institutions can design and implement interventions with higher success rates.
Challenges of Implementing Predictive Analytics in Education
Although predictive analytics has great potential in education, its implementation is not without challenges:
- Data Quality: Having access to accurate and reliable data is crucial for predictive analytics models to yield meaningful insights. Ensuring data quality and consistency across different systems and platforms can be a significant hurdle.
- Privacy and Ethical Concerns: Collecting and analyzing sensitive student data raises privacy and ethical concerns. Protecting student privacy and ensuring the appropriate use of data should be a priority when implementing predictive analytics in education.
- Teacher Training: Educators need to be trained in interpreting and utilizing the insights provided by predictive analytics effectively. This requires ongoing professional development and support to ensure successful adoption and implementation.
Conclusion
Predictive analytics holds immense potential in the education sector. By leveraging student data and advanced algorithms, educators can gain insights into student performance and tailor their teaching strategies to maximize learning outcomes. While there are challenges in implementing predictive analytics, addressing these hurdles can lead to improved educational practices and better support for students.
Comments:
This article provides valuable insights into the potential of predictive analytics technology in education. I believe it has the power to revolutionize the way we approach teaching and learning.
I completely agree, Brian. Predictive analytics can help identify at-risk students, enabling early intervention and targeted support. It has the potential to greatly improve academic outcomes.
While I see the benefits, I am concerned about student privacy. How can we ensure that the data collected is used ethically and responsibly?
That's a valid concern, Michael. Stringent data protection measures and clear consent policies are crucial in maintaining student privacy. Institutions need to prioritize ethical use of data.
Michael, you raise an important point. Educational institutions must establish robust privacy policies and ensure transparency in data collection and usage. Protecting student privacy should be a top priority.
I can see how predictive analytics can improve personalized learning experiences. By analyzing student data, educators can tailor instruction to meet individual needs. It's an exciting development.
Absolutely, Daniel. Predictive analytics can also assist in curriculum planning, identifying areas where students might struggle and adapting teaching techniques accordingly. It promotes targeted instruction.
I agree with you, Olivia. It helps teachers become more proactive by identifying potential challenges ahead of time. This can lead to more effective teaching strategies and student success.
Could predictive analytics potentially replace traditional assessments in the future? It seems like this technology has the potential to provide more accurate insights into student progress.
Robert, I believe predictive analytics can supplement traditional assessments, but it should not completely replace them. Both methods have unique advantages and should be used together to gain a comprehensive understanding of student progress.
While it can provide valuable insights, I don't think it should replace traditional assessments entirely. A balanced approach that utilizes both predictive analytics and traditional assessments is likely the way forward.
I wonder if there are any potential biases in the predictive analytics algorithms. We need to ensure fairness and equal opportunities for all students.
Karen, you bring up a crucial concern. It is essential to regularly assess and mitigate any biases present in predictive analytics algorithms. Fairness and equality must be integral to their development and implementation.
While predictive analytics can indeed enhance instruction, we must not solely rely on data. The human connection between teachers and students should never be neglected.
Very true, Michael. While analytics can provide valuable insights, the role of teachers in building relationships, providing emotional support, and fostering a positive learning environment cannot be replaced.
I'd like to know more about the implementation challenges of predictive analytics in education. Are there any specific hurdles institutions might face?
The availability and quality of data can be a significant challenge, David. Institutions need to ensure data accuracy, accessibility, and compatibility across different systems for effective implementation.
Additionally, there might be resistance to change among educators who are not familiar with analytics tools. Professional development and training programs can help address this hurdle.
I agree, Brian. Educators need to be equipped with the necessary knowledge and skills to effectively leverage predictive analytics tools. Ongoing support and training are crucial for successful implementation.
I'm curious about the potential cost implications of implementing predictive analytics in education. Would it require significant financial resources?
Cost is definitely a consideration, Laura. Implementing predictive analytics tools might require initial investments in infrastructure, data management systems, and staff training. However, the long-term benefits might outweigh the costs.
It's important to assess the return on investment and consider the potential cost savings from improved student outcomes, Laura. The financial impact should be evaluated alongside the educational benefits.
Are there any examples of successful implementations of predictive analytics in education that we can learn from?
One example is Georgia State University. They used predictive analytics to identify students at risk of dropping out and successfully intervened, improving graduation rates and reducing achievement gaps.
Another example is the University of California, Berkeley. They implemented analytics tools to personalize instruction and improve student engagement, resulting in higher course completion rates.
Both examples demonstrate the promising potential of predictive analytics in education. By leveraging data effectively, institutions can make informed decisions to support student success.
I have concerns about the potential over-reliance on technology in education. We shouldn't neglect the importance of hands-on, experiential learning.
I agree, Karen. While technology can enhance learning, it should be used as a tool to support and complement hands-on experiences, not as a replacement for active engagement in the learning process.
Balancing technology and real-world experiences is vital for holistic education. We shouldn't lose sight of the value of practical, hands-on learning opportunities.
How can predictive analytics address the needs of diverse learners? Is it adaptable enough to cater to individual learning styles and preferences?
Robert, that's an important consideration. Predictive analytics can help identify individual learning needs and tailor instruction accordingly, making it adaptable to cater to diverse learners.
Additionally, predictive analytics can assist in identifying barriers faced by specific student groups, enabling institutions to develop targeted interventions and support mechanisms to address their needs.
I can see the potential benefits of predictive analytics, but what about potential pitfalls? Are there any risks associated with its implementation?
One risk is the possibility of reinforcing existing biases if the algorithms are not carefully designed and monitored. We need to be mindful of potential unintended consequences.
Another risk is the over-reliance on data without considering the context. Educators should use predictive analytics as a tool alongside their expertise, rather than blindly following the recommendations.
How can educators ensure student engagement and motivation when utilizing predictive analytics? Are there any strategies to keep students actively involved in the learning process?
Incorporating gamification and interactive elements can help maintain student engagement, Laura. By making learning enjoyable and rewarding, educators can enhance motivation and participation.
Additionally, providing timely feedback and using the insights gained from predictive analytics to offer personalized learning experiences can keep students actively involved and interested in their own progress.
What steps can educational institutions take to ensure the successful implementation of predictive analytics? Any key considerations?
Clear communication and stakeholder involvement are vital, Karen. Instituting a comprehensive implementation plan with the buy-in and support of all relevant parties can significantly increase the chances of success.
Equity should also be a priority when implementing predictive analytics, Karen. Institutions should consider the needs of underprivileged students and ensure that all learners have equal access to the benefits.
Furthermore, starting with small pilot projects to test and refine predictive analytics tools can help mitigate risks and identify potential challenges early on, before full-scale implementation.
Thank you all for your valuable contributions and thoughtful discussions. Your insights and questions highlight the importance of ethical implementation, privacy, data accuracy, and student engagement in leveraging predictive analytics effectively in education.