Enhancing Efficiency and Accuracy in Manual Review for FPLC Technology with ChatGPT
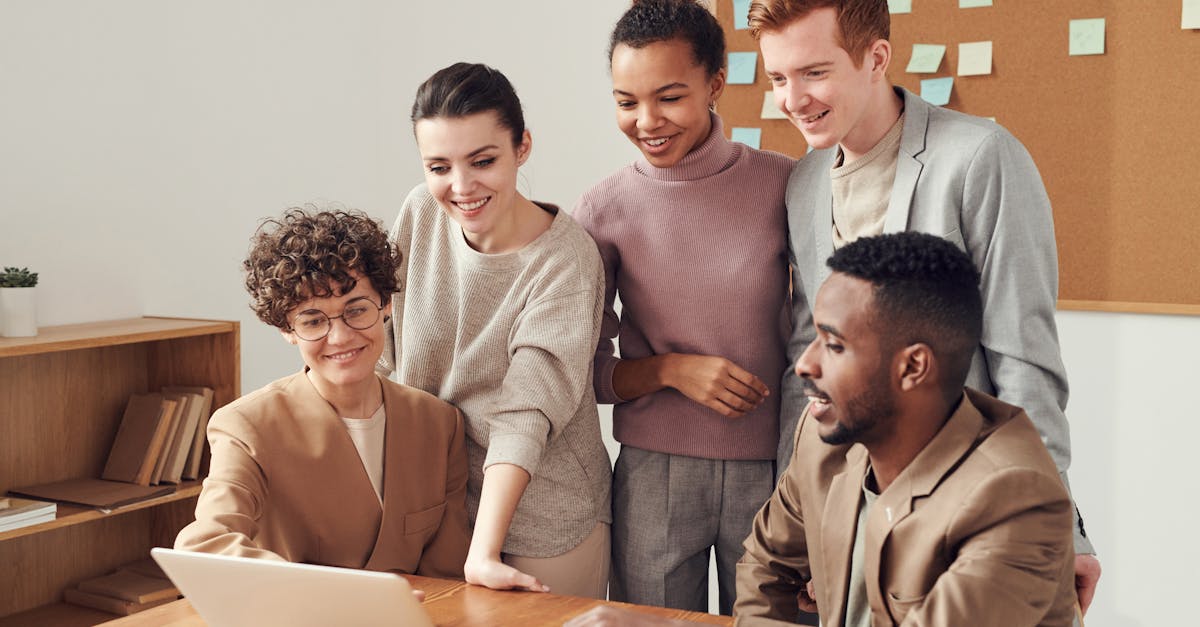
Introduction
The advancement of technology has revolutionized various aspects of our lives, and the field of laboratory instruments is no exception. The introduction of the Fast Protein Liquid Chromatography (FPLC) system has greatly enhanced the purification and analysis of proteins. However, to ensure accurate and efficient operation, it is crucial to carefully review the user manuals, product guides, and other technical documentation related to FPLC systems.
Challenges in Manual Review
Manual review of documentation can be a time-consuming and error-prone task. Human reviewers need to carefully read through every detail of the documentation to ensure accuracy and relevance. Mistakes during the review process can lead to improper use of the FPLC system, potentially affecting experimental results and laboratory productivity.
The Need for Automation
To overcome the challenges faced in manual review, automation technology can be employed. Automatic review systems for FPLC documentation can not only save time but also improve the accuracy and consistency of the review process. These systems utilize advanced algorithms to analyze the content, identify potential issues or errors, and provide suggestions for improvement.
Usage of Automated Review Systems for FPLC Manuals
Automated review systems designed specifically for FPLC systems can greatly enhance the efficiency and effectiveness of the manual review process. These systems can automatically analyze user manuals, product guides, and other technical documentation to ensure they meet industry standards, provide accurate instructions, and address all necessary aspects related to FPLC system usage.
By leveraging natural language processing and machine learning technologies, these systems can identify grammatical errors, inconsistencies, and missing information in the documentation. Additionally, they can provide recommendations for improving the clarity, conciseness, and overall quality of the content. This ensures that users can easily understand and follow the instructions, minimizing the risk of potential mistakes or accidents during FPLC system operation.
Benefits of Automated Review Systems
Implementing automated review systems for FPLC documentation offers several notable benefits:
- Time-saving: Manual review can be a time-consuming task, especially for complex technical documentation. Automated systems streamline the process, significantly reducing the time required for review.
- Enhanced accuracy: Machine algorithms are highly accurate in detecting errors and inconsistencies. By eliminating human error, the chances of launching inaccurate or incomplete documentation are greatly minimized.
- Consistency: Automated systems ensure consistent review standards are applied throughout the documentation. Human reviewers might have different interpretations or overlook specific details, whereas automated systems follow predefined rules consistently.
- Improved quality: Automated review systems provide valuable feedback and suggestions to enhance the overall quality of the documentation. This ensures that end-users have comprehensive and easy-to-understand instructions for operating the FPLC system.
Conclusion
Automated review systems offer a practical solution for ensuring accurate and comprehensive documentation for FPLC systems. By leveraging technology, manual review processes can be automated, resulting in significant time savings, improved accuracy, and better quality documentation. Adopting automated review systems can ultimately enhance the overall usage and efficiency of FPLC systems in the laboratory.
Comments:
Thank you all for reading my article on enhancing efficiency and accuracy in manual review for FPLC technology with ChatGPT. I'm excited to discuss this topic with you.
Kyle, are there any benchmarks or studies comparing ChatGPT's accuracy with other manual review methods? I'd like to know if the technology is more reliable.
Michael, there have been benchmark studies that show ChatGPT's accuracy and reliability comparable to human reviewers. It has shown promising results in identifying errors and providing consistent judgments.
Kyle, in your article, you mentioned that ChatGPT can learn from user feedback. How does this feedback loop work, and how does it enhance accuracy?
Michael, the feedback loop allows users to provide feedback on ChatGPT's suggestions or judgments. This feedback is used to continuously train and improve the underlying model, enhancing its accuracy and aligning it with specific user requirements.
Thanks for explaining, Kyle. The feedback loop plays a vital role in continuously improving and optimizing the system.
That's impressive, Kyle. Continuous learning and improvement through user feedback make ChatGPT a valuable tool for manual review.
Great article, Kyle! I've recently started using ChatGPT in my lab, and it has definitely made the manual review process much faster.
Emma, how has ChatGPT improved the speed of manual review in your lab? Are there any specific tasks where it has been most effective?
Liam, ChatGPT has been most effective in reviewing large datasets and identifying potential errors or anomalies. It quickly highlights areas that require closer inspection, allowing us to focus our manual review efforts more efficiently.
I'm curious, Kyle, how does ChatGPT improve the accuracy of manual review? Is it better than other methods?
The idea of using an AI-powered chatbot for FPLC manual review sounds interesting. Are there any limitations or challenges in its implementation?
Hi Kyle, I enjoyed reading your article. Could you explain how ChatGPT integrates with FPLC technology?
The speed improvement is mainly because ChatGPT can handle a large volume of data much faster than a human reviewer. It doesn't get tired or lose focus, so it maintains consistent performance throughout the review process.
Thanks for the clarification, Emma. It's fascinating how AI can assist in such complex tasks. Do you think ChatGPT has the potential to revolutionize manual review processes beyond FPLC?
Liam, absolutely! ChatGPT showcases the potential of AI in improving the efficiency and accuracy of manual review processes across various scientific and technical fields. It's a stepping stone towards more automation and optimization.
Emma, have you encountered any challenges or limitations while using ChatGPT for manual review?
Sara, one challenge is that ChatGPT's responses may not always be fully understandable or reliable. We need to carefully review its suggestions and validate them against our domain expertise.
However, it's important to note that ChatGPT is not meant to replace human reviewers entirely. It serves as a valuable tool to assist and expedite the manual review process.
Kyle, what are some of the challenges when implementing ChatGPT for FPLC manual review? Are there any limitations we should be aware of?
Samantha, one challenge is ensuring that ChatGPT understands the specific context and requirements of FPLC technology. It may require some fine-tuning of the model and dataset to optimize its performance.
Thanks for sharing, Kyle. It's important to be aware of the limitations and invest effort in optimizing the system for accurate results.
Another limitation is that ChatGPT’s responses are dependent on the input it receives. In cases where the input data is incomplete or ambiguous, it may not provide accurate or conclusive suggestions for manual review.
Kyle, how do users interact with ChatGPT during the manual review process? Do they input data manually, or does ChatGPT automatically analyze the FPLC data?
Sophia, users interact with ChatGPT through a user-friendly interface where they can input FPLC data or upload files for analysis. ChatGPT analyzes the data, identifies potential issues, and provides suggestions or clarifications to the user.
Kyle, can ChatGPT be easily integrated with existing FPLC systems, or does it require significant modifications to the infrastructure?
Oliver, integrating ChatGPT with existing FPLC systems usually requires some modifications to the infrastructure. The extent of modifications depends on the specific system and requirements, but it's typically manageable with proper technical guidance.
Thanks for the insight, Kyle. Considering the potential benefits, it seems worthwhile to invest in the necessary modifications for integration.
Got it, Kyle. Taking privacy, security, and compatibility into account is crucial when adopting new AI technologies.
That's fascinating, Kyle! It seems like a user-friendly interface and seamless interaction are key to successful implementation. Thanks for your response.
Furthermore, the quality of suggestions may vary depending on the complexity of the FPLC data and the training of the underlying model. It's crucial to continuously improve and fine-tune the system.
Thank you, Emma! It's exciting to think about the possibilities of AI-assisted manual reviews. I believe it will bring significant benefits to various fields in the future.
I agree, Liam! AI has already proven to be a game-changer in several areas, and its integration with manual reviews will undoubtedly enhance accuracy and efficiency.
Absolutely, Liam! AI integration should be seen as a complementary approach rather than a replacement to human expertise. It's all about leveraging the best of both worlds!
Well said, Sara! Collaboration between AI and human experts will lead to remarkable advancements in various scientific and technical fields.
Agreed, Sara! AI systems should be viewed as decision-support tools that enhance our capabilities, not replace them.
Absolutely, Emma! It's all about striking the right balance between automation and human decision-making for optimal outcomes.
Emma, it's important to validate AI suggestions against domain expertise. Human intervention will always be necessary to ensure reliable results.
Absolutely, Sara! Human expertise is irreplaceable, and machines are here to assist us in making better decisions, not replace our judgment.
In some cases, third-party platforms or APIs can facilitate the integration process. It's important to consider factors like data privacy, security, and compatibility during the implementation.
As the model receives more feedback and learns from the user's expertise, it becomes more reliable and consistent in providing meaningful suggestions during the manual review process.
Kyle, how long does it usually take for ChatGPT to learn and adapt to a particular lab's requirements?
Joanna, the learning and adaptation period for ChatGPT can vary depending on the complexity of the lab's requirements, available data, and the level of fine-tuning performed. It typically ranges from a few weeks to a couple of months.
Thanks, Kyle! I guess the learning period requires patience and collaboration between the lab and AI experts.
During this period, it's important to provide the model with diverse and representative data to ensure it comprehensively understands the nuances of the specific lab's manual review process.
I can imagine that fine-tuning the model to match FPLC requirements might be challenging. It requires domain expertise to achieve the desired accuracy, right?
Samantha, you're absolutely right. Achieving the desired accuracy and performance requires domain expertise to fine-tune the model adequately. Collaboration between data scientists, domain experts, and lab personnel is crucial in optimizing the system.
Agreed, Kyle. Being aware of limitations and ensuring optimization will help us harness the potential of ChatGPT for FPLC manual review.
Collaboration among different teams will be key in maximizing the system's performance. Thanks for the clarification, Kyle.
I understand. Providing diverse and representative data will ensure that the model captures the intricacies of our lab's requirements.