Enhancing Endorsement Technology with ChatGPT's Sentiment Analysis
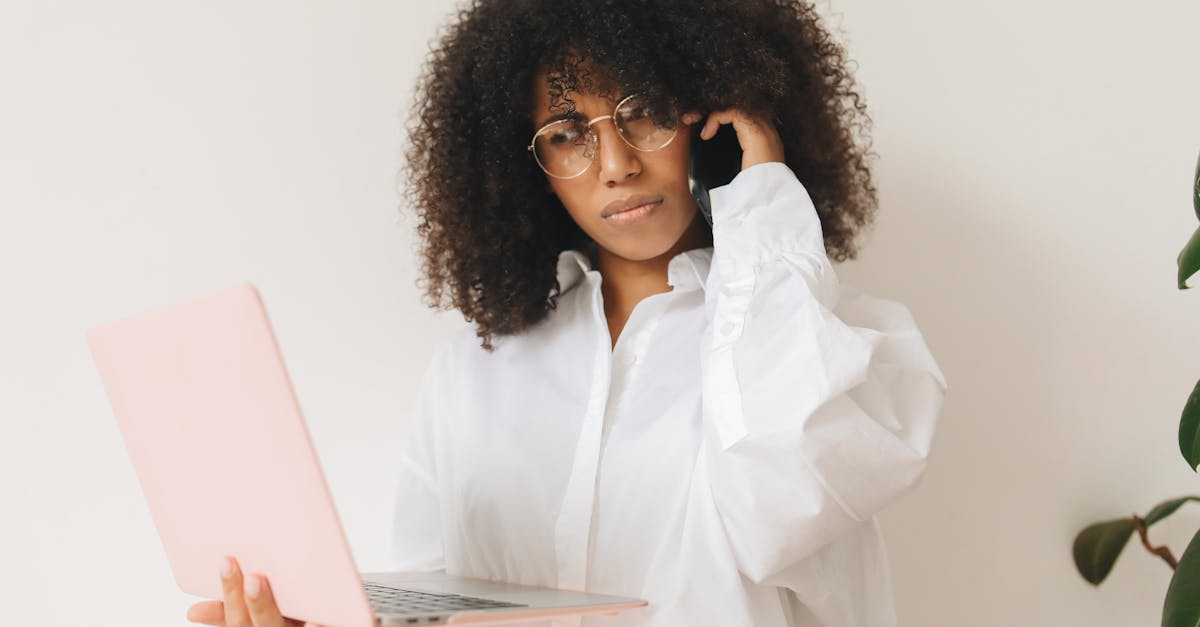
The advent of sophisticated Artificial Intelligence (AI) technologies has enabled many drastic changes in the field of sentiment analysis. Among these technologies, end-user endorsements play a vital role in shaping user perspectives and market trends. This article will delve into the technology of endorsements and its area of application in sentiment analysis, with a specific emphasis on its usage in the renowned AI model, ChatGPT-4.
A Brief on Endorsements
Endorsements represent affirmations or approvals of a product or service's benefit, typically provided by a renown or influencer. In the digital sphere, these endorsements can be both overt (like testimonial-based advertisements) and covert (such as subtly placed products in a widely viewed online video). It has been studied and established that these endorsements can affect purchasing decisions and overall customer sentiment towards a product or brand. Given the impact, it becomes crucial for brands to analyze these sentiments to strategize their marketing moves effectively.
Sentiment Analysis: A Comprehensive Understanding
Sentiment Analysis, often referred to as 'opinion mining,' is a field that uses NLP (Natural Language Processing), text analysis, and computational linguistics to identify and extract personal information from source materials. It helps to discern if the endorsement's impact is positive, negative, or neutral. It is indicative of customers' deeper emotions, opinions, and attitudes towards a product or brand.
The Role of ChatGPT-4 in Sentiment Analysis
Powered by OpenAI, ChatGPT-4 is an AI language model based on the transformer architecture that can generate human-like text based on a given input. It leverages the power of machine learning algorithms to understand, interpret, and respond in a human-like manner. It's the latest version of ChatGPT and has demonstrated remarkable progress in understanding context and language nuances.
ChatGPT-4's application in the realm of sentiment analysis is manifold. Firstly, it can analyze textual data from multiple sources like social media comments, blog posts, product reviews, and chat transcripts to assess customer sentiments. It can help marketers uncover hidden patterns and sentiments that would likely be missed by manual analysis. Its deep learning capabilities allow it to identify even the subtlest cues of customer satisfaction, displeasure, or neutral feelings.
Secondly, ChatGPT-4, with its enhanced conversational capabilities, could interact directly with customers. AI chatbots based on ChatGPT-4 could be used as a tool for gathering endorsements or feedback and analyzing customer sentiment in real-time. Engaging with customer queries, understanding their problems, providing solutions, and gauging their responsiveness - all these functions can be coordinated seamlessly by ChatGPT-4.
Conclusion
In the era where data is available profoundly, technology like endorsements for sentiment analysis using high-performing AI models like ChatGPT-4 can be game-changers. They not only help in assessing customers' reaction towards a product or brand endorsement but also in shaping strategies that directly reflect the brands' understanding of their customers. Their significance is only set to rise in the coming times as AI continues to penetrate multiple business domains.
Comments:
Thank you all for joining the discussion on my article, 'Enhancing Endorsement Technology with ChatGPT's Sentiment Analysis'. I'm excited to hear your thoughts!
Great article, Joseph! The application of ChatGPT's sentiment analysis in endorsement technology seems promising. It could revolutionize the way endorsements are measured and understood.
I agree with Sarah. The potential benefits of using ChatGPT's sentiment analysis are huge, especially in enhancing the accuracy and credibility of endorsements.
While I see the potential, I'm concerned about the reliability of sentiment analysis. It heavily relies on context and can sometimes misinterpret emotions. What steps have been taken to address this issue?
James, you raise an important concern. While sentiment analysis can never be 100% perfect, continuous improvement through refining the models and incorporating user feedback can help minimize inaccuracies. It's an evolving field, and refinements will address the challenges you mentioned.
James, that's a valid point. Sentiment analysis can indeed have limitations. Joseph, I would be interested to hear your thoughts on how you plan to mitigate such concerns.
James and Olivia, you both make valid points. Addressing the limitations of sentiment analysis is crucial, and it's an ongoing effort. Feedback from users and refining the models based on real-world data will play a significant role in ensuring accuracy and reducing misinterpretations.
I can see how sentiment analysis can be valuable for endorsements, but what about the privacy concerns? Analyzing sentiments requires analyzing personal data, doesn't it?
Emily, that's a good concern to raise. However, sentiment analysis does not necessarily require analyzing personal data. It can work based on the text itself without delving into identifiable information. Privacy safeguarding approaches can be implemented, ensuring the ethical use of the technology.
Emily, Sarah is correct. When implementing sentiment analysis, any personal data should be handled responsibly following privacy guidelines. The focus is on analyzing the sentiment of the content rather than profiling individuals.
This article presents an interesting use case for ChatGPT! By incorporating sentiment analysis, endorsements can become much more reliable. Looking forward to seeing this technology in action.
Absolutely, Lisa. Adding sentiment analysis to endorsement technology can provide a more nuanced understanding of consumers' reactions. It can help businesses better understand and leverage the impact of endorsements on their products or services.
This article overlooks the potential biases in sentiment analysis. Different cultures and contexts may interpret sentiments differently. How can we ensure the analysis remains objective and neutral in such cases?
Excellent point, Sophia! Biases in sentiment analysis are a concern. To mitigate this, diverse training data representative of various cultures and contexts should be incorporated. Regular evaluation and updates to the sentiment models will be necessary to ensure objectivity and neutrality.
Sophia and Joshua, I appreciate your insights. Bias mitigation in sentiment analysis is crucial. Joseph, could you share any plans you have for addressing this issue?
Oliver, I agree with Joseph's response. Continuously auditing the models, seeking external feedback, and collaborating with diverse communities should help in building more inclusive and unbiased sentiment analysis models.
Sophia and Joshua, you raise a valid concern. Addressing biases is a top priority to ensure the objectivity and fairness of sentiment analysis. Incorporating diverse training data and refining the models to handle various cultural contexts is part of the plan to mitigate biases.
I'm impressed by the potential of sentiment analysis in endorsements. It could save a lot of manual effort in collecting and measuring sentiments. Looking forward to seeing it adopted widely!
Indeed, David. The automation and efficiency of sentiment analysis can prove to be a game-changer in endorsements. It will enable businesses to make data-driven decisions and enhance the overall endorsement experience.
I must say, sentiment analysis in endorsements has the potential to impact marketing strategies significantly. It could help businesses identify trends, capture customer sentiment, and tailor their approaches accordingly.
Joseph, I really enjoyed your article. Could you elaborate on any specific industries or sectors where you think the application of sentiment analysis in endorsements would be particularly beneficial?
Olivia, sentiment analysis can be valuable across various industries. Some specific examples include retail, hospitality, technology, and even entertainment. Endorsements are present in almost every sector, and sentiment analysis can enhance the impact and understanding of those endorsements.
Joseph, I agree with you. In sectors like e-commerce, understanding customer sentiment through endorsement analysis can help businesses tailor their product recommendations, personalize the shopping experience, and ultimately increase customer satisfaction.
Oliver, well-said! The potential for personalized recommendations by incorporating sentiment analysis is immense. It can create a win-win situation for both businesses and customers.
While sentiment analysis is valuable, it shouldn't replace thorough human analysis. There might still be subjective nuances that automated sentiment analysis might miss. Humans can provide deeper insights.
I agree, Emma. Automated sentiment analysis should supplement human analysis rather than replace it completely. A combination of both approaches could lead to more comprehensive insights.
True, Emma and Harper. Human analysis brings subjective insights and interpretations that automated sentiment analysis might lack. A balanced approach, leveraging the strengths of both, would be ideal.
The use of sentiment analysis in endorsements certainly has tremendous potential. However, its effectiveness might vary according to the platform, language, or medium of the endorsements. Joseph, how do you plan to address these variations?
Sophia, that's an essential consideration. Different platforms or languages can introduce variations in sentiment analysis results. Joseph, I'm curious to know how robust the sentiment analysis model is in handling such variations.
James, Joseph's response aligns with best practices. Incorporating diverse datasets that represent different scenarios will ensure the sentiment analysis model can handle variations and provide reliable insights across platforms and languages.
Sophia and James, you bring up an important aspect. To address variations, the sentiment analysis model will be trained and fine-tuned with diverse datasets covering various platforms, languages, and mediums. This approach should help increase the effectiveness and adaptability of the technology.
A thought-provoking article, Joseph. Sentiment analysis can definitely enhance the endorsement technology landscape. It might even pave the way for new possibilities and innovations in this field.
Couldn't agree more, John. Advancements in sentiment analysis have the potential to drive significant progress in the endorsement technology domain. The future looks exciting!
Joseph, great work on the article. Sentiment analysis has come a long way, and its integration with endorsement technology can pave the way for more sophisticated and data-driven strategies in various industries.
I'm impressed by the potential of sentiment analysis in improving the reliability of endorsements. Joseph, what challenges do you foresee in adopting this technology widely?
Oliver, while sentiment analysis holds immense promise, widespread adoption may face challenges such as initial costs, infrastructure requirements, and resistance to change. However, as the technology proves its value, these barriers are likely to diminish over time.
Oliver, Joseph nailed it. Embracing any new technology often faces resistance and overcoming it requires showcasing its benefits and ROI. As sentiment analysis continues to demonstrate its value, I believe wider adoption will follow.
Joseph, I thoroughly enjoyed reading your article. It's fascinating to see the potential of sentiment analysis in transforming endorsements. Best of luck with further advancements in this field!
Joseph, thank you for shedding light on the potential of sentiment analysis in endorsements. It's an exciting area where technology can enhance our understanding of consumer sentiments and preferences.
Thank you, Emily and Sophia, for your kind words. I'm glad you found the article interesting. It's indeed an exciting field with tremendous possibilities. Your feedback and engagement mean a lot.