Enhancing Fault Diagnosis for Pressure Technology using ChatGPT
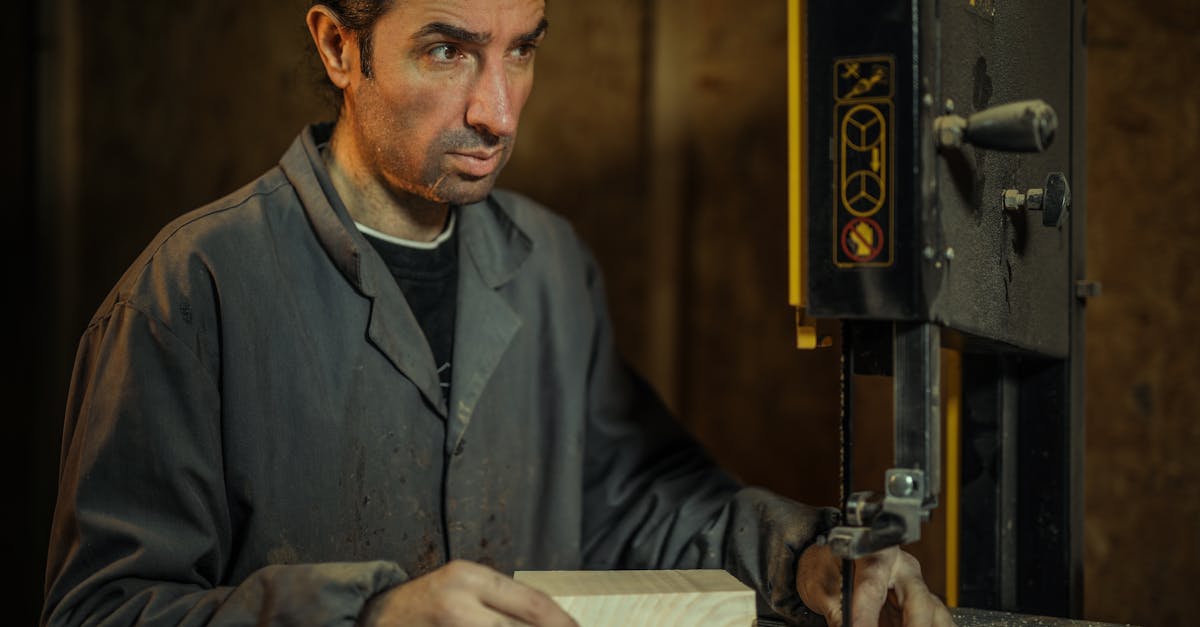
Pressure technologies play a crucial role in various industries, including manufacturing, engineering, and energy. However, like any other complex system, pressure technologies are prone to faults and malfunctions. Identifying and diagnosing these issues quickly and accurately is essential to ensure the smooth functioning of such systems. This is where ChatGPT-4 comes in as a valuable tool in fault diagnosis.
ChatGPT-4, an advanced language model powered by artificial intelligence, can assist in diagnosing faults or malfunctions in pressure technologies by analyzing system behavior, error codes, and user-reported symptoms. Its ability to understand and interpret textual descriptions of issues enables it to provide valuable insights and recommendations for troubleshooting.
How ChatGPT-4 Works
ChatGPT-4 is trained on a diverse range of data, including technical manuals, user forums, and expert knowledge in pressure technologies. This extensive training allows it to understand the intricacies of pressure systems and their potential faults. When given relevant information about a particular issue, ChatGPT-4 uses its knowledge to analyze the problem and provide step-by-step guidance for fault diagnosis.
Users can interact with ChatGPT-4 through a chat interface, where they can describe the symptoms they are experiencing, the error codes displayed by the system, and any other relevant information. ChatGPT-4's natural language processing capabilities enable it to understand and extract key details from the user's input, facilitating accurate fault diagnosis.
Potential Use Cases
ChatGPT-4 can assist in diagnosing a wide range of faults and malfunctions in pressure technologies. Some potential use cases include:
- Pressure Drop: If a pressure system experiences an unexpected drop in pressure, users can describe the circumstances leading to the drop, any abnormal noises, and any other relevant observations. ChatGPT-4 can then analyze this information to identify potential causes, such as leaks, faulty valves, or blockages.
- Overpressure: In situations where a pressure system exceeds safe operating limits, users can provide details such as the pressure levels observed, the duration of the overpressure event, and any associated alarms or warnings. ChatGPT-4 can then analyze this data to pinpoint potential causes, which may include malfunctioning pressure relief valves or sensor inaccuracies.
- Pressure Fluctuation: If a pressure system shows irregular pressure fluctuations, users can describe when and how frequently these fluctuations occur, as well as any accompanying symptoms. ChatGPT-4 can analyze this information to identify potential causes, such as pump issues, pressure regulator problems, or control system malfunctions.
- System Error Codes: Users can provide the specific error codes displayed by the pressure system. ChatGPT-4 can leverage its knowledge base to interpret these error codes and provide guidance on the underlying causes.
Benefits of ChatGPT-4 in Fault Diagnosis
Using ChatGPT-4 for diagnosing faults in pressure technologies offers several benefits:
- Efficiency: ChatGPT-4's ability to process and understand complex textual information allows for efficient fault diagnosis, reducing downtime and minimizing disruptions.
- Accuracy: With its extensive training on pressure technology data, ChatGPT-4 can provide precise recommendations and potential solutions based on the given symptoms, error codes, and system behavior.
- Knowledge Base Expansion: By interacting with users and learning from real-world fault scenarios, ChatGPT-4 can continually improve its fault diagnosis capabilities, expanding its knowledge base and enhancing its potential for accurate diagnoses.
- Accessible Support: ChatGPT-4 can be accessed through various platforms, such as web interfaces or dedicated mobile applications, making it convenient for users to seek assistance anytime and anywhere.
Conclusion
Pressure technologies are critical components in numerous industries, and diagnosing faults or malfunctions promptly is crucial for maintaining operational efficiency. ChatGPT-4, with its advanced language processing capabilities, can assist users in diagnosing pressure system issues by analyzing symptoms, error codes, and system behavior. Its AI-powered knowledge base makes it a valuable tool for fault diagnosis, offering accurate recommendations and troubleshooting guidance. Incorporating ChatGPT-4 into fault diagnosis workflows can lead to more efficient and reliable maintenance of pressure technologies.
Comments:
Thank you all for reading my article on enhancing fault diagnosis for pressure technology using ChatGPT! I'm excited to hear your thoughts and opinions on this topic.
Great article, Hank! I find it fascinating how AI can contribute to fault diagnosis. It definitely has the potential to revolutionize the field.
Thank you, Sarah! Indeed, AI has the capability to offer more accurate and efficient fault diagnosis, saving time and resources.
Interesting read, Hank! Do you think AI will completely replace human expertise in fault diagnosis, or will they work hand in hand?
That's a great question, Michael. While AI can greatly assist in diagnosis, I believe human expertise will still be crucial in complex scenarios that require judgment and domain-specific knowledge.
I think AI will definitely augment human expertise but won't fully replace it. There are certain nuances that only experienced professionals can handle.
Absolutely, Emily. The collaboration between AI and human experts can lead to more accurate diagnoses and better decision-making.
Hank, I'm curious to know more about the performance of ChatGPT in fault diagnosis. Are there any specific cases where it has shown significant improvement?
Great question, Robert! ChatGPT has shown promising results in fault diagnosis, especially in cases with large datasets and patterns that may not be immediately obvious to human experts. It can assist in quickly identifying common faults and potential solutions.
Hank, what measures are taken to ensure that AI doesn't provide inaccurate or misleading diagnoses?
Valid concern, John. AI systems like ChatGPT are trained on extensive datasets to minimize errors, but it's crucial to have a feedback loop with human experts to correct and fine-tune the system over time. Regular verification and validation steps are crucial.
I can see the potential benefits of using AI in fault diagnosis, but what are the potential limitations or challenges we might face?
Great question, Stephen. One of the challenges is the interpretability of AI models. They can sometimes provide accurate diagnoses without explaining the underlying reasons. Ensuring transparency and interpretability is crucial for gaining trust and acceptance in the industry.
Hank, you mentioned utilizing ChatGPT for fault diagnosis, but what about other machine learning models or techniques?
Good point, Jennifer. While ChatGPT can be effective, other machine learning models and techniques like neural networks, decision trees, or support vector machines are also widely used in fault diagnosis. The choice depends on the specific requirements of the application.
Hank, what kind of data sources are usually used to train models like ChatGPT for fault diagnosis?
Excellent question, Mark. Data sources can include historical fault data, sensor readings, maintenance logs, and even expert knowledge encoded into a knowledge base. The more diverse and comprehensive the data, the better the model's performance.
Hank, how accessible are AI-based fault diagnosis systems for industries or businesses with limited resources?
That's an important consideration, Laura. AI-based systems can be costly to develop and implement. However, as AI technology progresses, we can expect more affordable and accessible solutions for businesses of all sizes.
Hank, how does ChatGPT handle real-time fault diagnosis? Is it as effective as offline analysis?
Good question, Daniel. While ChatGPT can handle real-time fault diagnosis, its effectiveness relies on the availability and reliability of live data. Offline analysis can offer more comprehensive insights, but real-time monitoring with ChatGPT can help identify immediate faults or anomalies.
Hank, how does ChatGPT handle situations where hardware failures cause irregular or inconsistent data patterns?
Great question, Olivia. When hardware failures cause inconsistent data patterns, ChatGPT may struggle to provide accurate diagnoses. Robustness to abnormal data patterns is still an ongoing research area, and hybrid approaches are often used where human experts intervene in such situations.
Hank, what are the potential cost savings or benefits that AI-based fault diagnosis systems can provide?
Good point, Matthew. AI-based fault diagnosis systems can lead to significant cost savings by reducing downtime, preventing costly failures, and optimizing maintenance and repair efforts. It can also enhance overall equipment efficiency and prolong its lifespan.
Hank, what are the ethical considerations when using AI for fault diagnosis, especially in critical industries like healthcare or aviation?
Great question, Rachel. Ethical considerations include ensuring the privacy and security of sensitive data, mitigating bias in the models, and maintaining human oversight for critical decisions. Transparency and accountability are essential to incorporating AI in critical industries responsibly.
Hank, what are some of the common challenges faced while implementing AI-based fault diagnosis systems in industries?
Valid question, James. Some common challenges include data accessibility, integration with existing systems, ensuring reliable and continuous data streams, and overcoming resistance to change from personnel. Collaborating with domain experts and a phased implementation strategy can help overcome these challenges.
Hank, what steps can be taken to gain the trust of professionals who might be skeptical about adopting AI for fault diagnosis?
An important question, Sophia. Building trust involves transparently demonstrating the effectiveness and limitations of AI models, including pilot studies or trials, involving experts in the development process, and showcasing the value-add the technology brings to the field.
Hank, what are the key considerations for selecting the appropriate AI model or technique for fault diagnosis?
Good question, Kevin. Key considerations include the complexity of fault patterns, available data, training requirements, interpretability needs, and the specific goals of the fault diagnosis system. It's important to experiment and evaluate different models to find the best fit for the given application.
Hank, do you have any recommendations for industries or businesses wanting to implement AI-based fault diagnosis?
Certainly, Liam. Prioritize data collection, ensure data quality and diversity, collaborate with domain experts, conduct pilot studies for validation, and seek support from experienced AI professionals or consultancies. Starting small and gradually scaling up can also lead to successful implementation.
Hank, are there any regulations or standards that need to be considered when implementing AI in fault diagnosis, especially in safety-critical industries?
Important question, Chloe. Safety-critical industries often have specific regulations and standards. It's essential to consider standards like ISO 26262 for automotive, IEC 61508 for functional safety, or FDA guidelines for healthcare applications. Adhering to such regulations ensures safe and reliable implementation.
Hank, what kind of computing infrastructure would be necessary to deploy AI-based fault diagnosis systems on a large scale?
Great question, Melissa. Large-scale deployment would require robust computing infrastructure like high-performance servers, storage systems, and efficient data processing capabilities. Cloud-based solutions can provide flexibility and scalability, but it's important to design the infrastructure based on the specific requirements of the fault diagnosis system.
Hank, what kind of challenges can arise when integrating AI-based fault diagnosis systems into existing industrial processes?
Good question, Jordan. Challenges can include data integration issues, compatibility with existing infrastructure, training personnel to work with AI systems, managing change within the organization, and addressing concerns related to job displacement. Taking a phased approach, involving stakeholders, and actively addressing these challenges is important for successful integration.
Hank, what potential risks or downsides should be considered when relying heavily on AI for fault diagnosis?
Good question, Emma. Risks include over-reliance on AI leading to complacency in human experts, biases in the training data affecting diagnoses, and potential vulnerabilities to adversarial attacks. Striking the right balance between AI and human expertise, continuous monitoring, and addressing biases are crucial to mitigate these risks.
Hank, apart from the fault diagnosis aspect, can AI be utilized for predictive maintenance in pressure technology as well?
Absolutely, Amy. AI can be immensely beneficial for predictive maintenance in pressure technology. By analyzing historical sensor data, identifying patterns, and detecting early signs of potential faults, AI models can help predict maintenance requirements, reduce unplanned downtime, and optimize maintenance schedules.
Hank, what are the potential benefits of using a chat-based AI system like ChatGPT for fault diagnosis, compared to other types of interfaces?
Good question, Gabriel. Chat-based interfaces like ChatGPT offer a conversational and intuitive way for users to interact with the system. They provide explanations, gather additional context, and allow users to ask follow-up questions. This makes them user-friendly, especially for non-technical personnel, and can help in effectively diagnosing faults.
Hank, what are your thoughts on the future of AI in fault diagnosis? Any exciting developments or trends we can expect to see?
Great question, Jacob. The future of AI in fault diagnosis looks promising. We can expect advancements in interpretability and explainability of AI models, more sophisticated anomaly detection techniques, and increased collaboration between AI and human experts. Additionally, as more industries embrace AI, we may also see industry-specific frameworks and standards being developed.
Thank you all for participating in this discussion! I really appreciate your insightful questions and contributions. It's been a pleasure sharing my thoughts on enhancing fault diagnosis using ChatGPT. Have a great day!