Enhancing Fault Diagnosis in ATM Networks: Leveraging ChatGPT's Intelligence
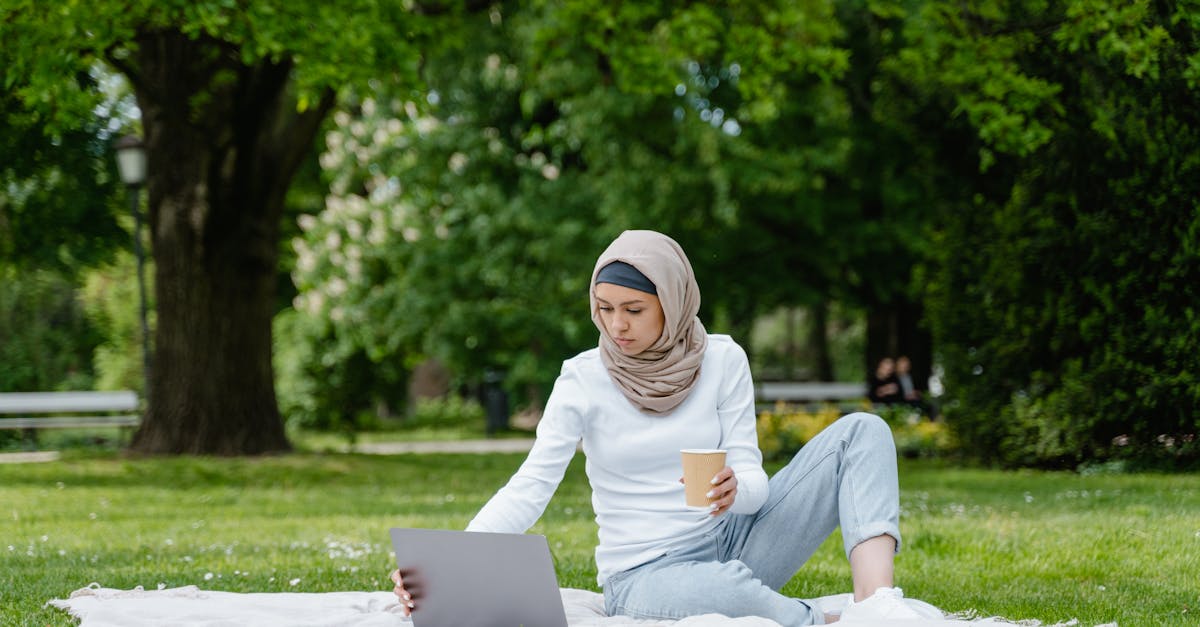
With the increasing reliance on ATM networks in various industries, it becomes imperative to ensure their uninterrupted functioning. Fault diagnosis plays a crucial role in identifying potential problems in ATM hardware or software before they impact the service delivery. Predictive modeling is a powerful technology that can aid in this process and enhance the efficiency of ATM network management.
Understanding ATM Networks
ATM, or Asynchronous Transfer Mode, is a communication protocol widely used in telecommunications to transmit digital data. ATM networks provide high-speed and reliable data transmission for a variety of services, including voice, video, and data. The networks consist of ATM switches, which route data packets through the network based on ATM cells.
The Importance of Fault Diagnosis
Fault diagnosis is an essential aspect of maintaining the smooth functioning of ATM networks. It involves identifying and analyzing potential issues that can lead to disruptions in service delivery. Timely detection of faults allows operators to take proactive measures, minimizing downtime and maximizing network performance and availability.
The Role of Predictive Modeling in Fault Diagnosis
Predictive modeling utilizes advanced algorithms and statistical techniques to analyze data and make predictions about future events or behaviors. In the context of ATM networks, predictive modeling can be used to identify potential problems by analyzing historical data related to network performance, hardware and software configurations, and environmental factors.
By analyzing this data, predictive models can identify patterns, correlations, and anomalies that may indicate the presence of a fault or a potential issue. This information can then be used to prioritize maintenance activities and allocate resources efficiently, ensuring that critical faults are addressed promptly.
Benefits of Predictive Modeling for Fault Diagnosis in ATM Networks
Predictive modeling offers several advantages when applied to fault diagnosis in ATM networks:
- Early Detection: By utilizing historical data and identifying patterns, predictive modeling can often detect potential faults before they actually occur. This allows operators to address the issues proactively, minimizing service disruptions.
- Optimized Resource Allocation: By prioritizing maintenance activities based on predicted faults, predictive modeling helps allocate resources effectively. It ensures that critical areas of the network receive timely attention, optimizing the use of manpower and minimizing downtime.
- Improved Decision Making: Predictive models provide actionable insights that enable informed decision making. By understanding potential problems in advance, operators can plan and budget for necessary repairs or upgrades, reducing reactive interventions and associated costs.
- Increased Network Performance: By identifying and addressing potential problems proactively, predictive modeling enhances overall network performance and minimizes service disruptions. This results in higher customer satisfaction and increased operational efficiency.
Conclusion
Fault diagnosis plays a vital role in maintaining the reliability and performance of ATM networks. By utilizing predictive modeling, operators can identify potential issues before they impact the network. This technology enhances decision making, optimizes resource allocation, and improves network performance. Implementing predictive modeling for fault diagnosis in ATM networks is a proactive approach that ensures smooth operations and satisfied customers.
Comments:
This article on enhancing fault diagnosis in ATM networks using ChatGPT's intelligence is fascinating. It's impressive to see how AI is being utilized in complex systems.
I agree, Sarah. AI has become an integral part of various industries, and its potential in fault diagnosis can greatly improve network reliability.
Mark, while AI can improve efficiency, it's important to have humans as a backup in case something goes wrong or the system encounters an unfamiliar situation.
Indeed, AI-driven fault diagnosis can significantly reduce downtime and maintenance costs in ATM networks. It's a step towards more efficient and reliable systems.
Emma, you mentioned reduced maintenance costs. Could AI-driven fault diagnosis potentially lead to job losses in the field of network maintenance and troubleshooting?
John, automation can indeed lead to changes in job roles, but it may also create new opportunities for professionals to focus on higher-value tasks that require human judgment.
While AI has its merits, we must also consider the limitations and potential risks associated with relying on automated systems for critical network operations.
Carlos, I understand your concern. AI technologies should be implemented with caution to ensure they complement human expertise rather than replace it entirely.
I fully agree with you, Karen. AI can assist experts, but it shouldn't replace their decision-making abilities and years of experience completely.
Samuel, I believe that integrating AI into fault diagnosis can augment human decision-making and lead to faster and more accurate problem resolution.
Emma, I agree that AI can bring agility and accuracy to fault diagnosis, potentially reducing downtime and enhancing customer experience.
Thank you all for your comments and valuable insights! I appreciate your perspectives on the article.
I wonder what specific techniques ChatGPT employs to enhance fault diagnosis in ATM networks.
That's a great question, Michael. I'm curious to know more about the underlying mechanisms of ChatGPT's intelligence in this context.
I'm guessing ChatGPT leverages natural language processing to understand and analyze network fault data, Michael.
That makes sense, Sophie. Natural language processing could be very useful in interpreting network logs and error messages.
I've read that ChatGPT utilizes transformer models, which excel at handling sequential data like logs and messages in fault diagnosis.
Absolutely, Sarah. Human supervision and intervention are crucial to avoid catastrophic consequences if the AI system fails or provides incorrect diagnoses.
To add to that, Ryan, regular system audits and rigorous testing can help identify any shortcomings or biases in the AI-driven fault diagnosis process.
David, you make a valid point. The jobs landscape may change, but there will always be a need for skilled professionals to manage and maintain complex network infrastructures.
Laura, you're right. AI should be seen as a tool that enhances human capabilities rather than a replacement for skilled professionals.
David, I couldn't agree more. Continuous monitoring of the AI system's performance and addressing any biases that may arise are essential.
Sophie, NLP combined with transformer models seems like an ideal combination in this context. It can understand logs and communicate diagnostic insights effectively.
Ryan, I think a collaborative approach combining AI and human expertise would be the most effective in fault diagnosis.
Lisa, a collaborative approach would ensure the best of both worlds—human expertise and AI's analytical power—working hand in hand.
John, a human-AI collaboration can make fault diagnosis more efficient, especially in situations where time is of the essence.
Emma, the combination of human expertise and AI's ability to process data at scale can indeed lead to faster problem resolution, benefiting both service providers and end-users.
Emma, agreed. Time is critical, especially in high-stakes situations, where AI-assisted fault diagnosis can help reduce detection and resolution times.
Sarah, transformer models are indeed powerful for dealing with sequential data. Their ability to capture dependencies can help in fault diagnosis where events are often interconnected.
Mark, the ability of transformer models to capture complex relationships is undoubtedly beneficial in diagnosing issues that may span multiple network components.
Absolutely, continuous evaluation and improvement are necessary to mitigate any potential biases and ensure the system performs effectively in diverse scenarios.
Sophie, continuous evaluation and improvement will be vital to ensure AI systems adapt to changing network architectures and evolving faults.
Sophie, I completely agree. Ethical considerations should also be incorporated into the design and deployment of AI-driven fault diagnosis systems.
Lisa, ethical considerations are crucial. Transparency and accountability in AI systems can help build trust and ensure responsible deployment.
Integrating AI into fault diagnosis should be seen as a complement rather than a replacement. It can analyze vast amounts of data quickly, aiding human experts in decision-making.
Daniel, striking the right balance between AI and human expertise is crucial for successful fault diagnosis without compromising safety or reliability.
Karen, you summed it up well. Collaboration between AI systems and human professionals can lead to more robust and effective fault diagnosis.
Laura, I believe the combination also has the potential to improve the overall reliability of ATM networks by identifying patterns and addressing them proactively.
Samuel, exactly! AI can offer insights that might not be immediately apparent to human operators, leading to more proactive fault mitigation strategies.
Emma, I think the combination can also help identify and address potential vulnerabilities in the network before they lead to actual failures.
Samuel, proactive identification of vulnerabilities is crucial in maintaining network resilience and delivering a reliable service to customers.
Laura, collaboration between humans and AI in fault diagnosis aligns with the vision of augmented intelligence—enabling humans and machines to work together effectively.
Daniel, exactly! Combining human intelligence with AI technologies can lead to more effective fault diagnosis, benefiting both service providers and end-users.
NLP and transformer models can enhance fault diagnosis, but we should also be cautious about potential biases in training data that might affect diagnostic accuracy.
Michael, addressing biases in training data is essential to ensure fair and accurate fault diagnosis, especially when AI systems are making critical decisions.
David, agreed. Bias mitigation and robust validation processes are vital to ensure AI-driven fault diagnosis doesn't perpetuate discriminatory or harmful actions.
Sophie, ensuring transparency in the decision-making process of AI systems can help address concerns about accountability and potential biases.
Sophie, transparency is key to building trust in AI-driven fault diagnosis and garnering support from stakeholders.
Agreed, John. Human oversight adds an extra layer of dependability and helps prevent any unintended consequences of relying solely on AI.
Sophie, Lisa, and John, transparency, accountability, and human oversight are critical in ensuring that AI-driven fault diagnosis benefits us without compromising our safety or trust.
AI brings exciting possibilities, but we should also plan for contingencies and have experts available to intervene when AI alone isn't sufficient.