Enhancing Fault Prediction in Ocean Freight Technology with ChatGPT
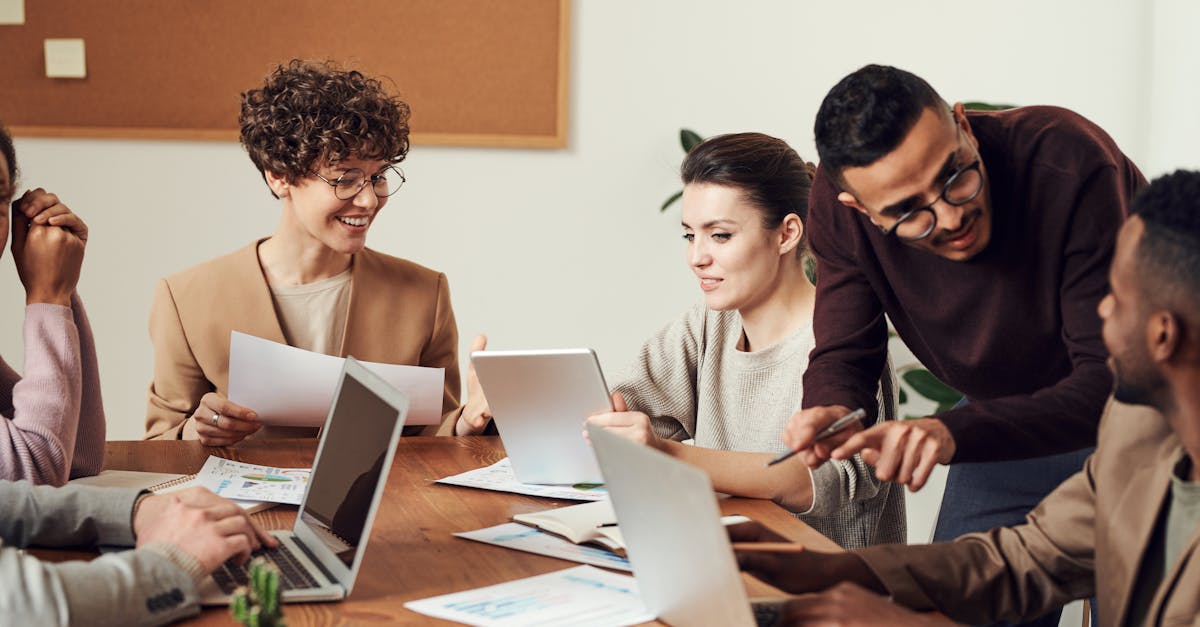
In the realm of ocean freight, efficiency and reliability are crucial factors to ensure smooth operations. Any unexpected equipment faults can cause significant delays and financial losses. However, with the advancements in technology, specifically the usage of ChatGPT, the prediction of faults and maintenance needs for ocean freight equipment has become more accurate and efficient than ever before.
ChatGPT, an AI-powered language model, can use vast amounts of data collected from various sources to analyze and predict potential faults and maintenance requirements for ocean freight equipment. By utilizing natural language processing and machine learning algorithms, ChatGPT can detect patterns and anomalies that may indicate an upcoming fault or maintenance need.
The predictive capabilities of ChatGPT, combined with its ability to process large volumes of data, are revolutionary in the world of ocean freight. By continuously monitoring equipment conditions, analyzing historical data, and comparing it with real-time sensor readings, ChatGPT can identify subtle indications of faults or upcoming maintenance needs.
By predicting faults and maintenance needs in advance, ChatGPT enables shipping companies to take proactive measures to avoid unexpected downtime and disruptions. This not only minimizes financial losses but also helps maintain a high level of customer satisfaction by ensuring timely deliveries.
The application of ChatGPT in fault prediction for ocean freight has several benefits. Firstly, it allows shipping companies to allocate resources efficiently by prioritizing maintenance tasks based on predicted fault severity. This helps optimize the utilization of manpower and reduces unnecessary costs associated with reactive emergency repairs.
Secondly, the proactive approach to fault prediction and maintenance planning ensures a safer working environment for the crew and the cargo. By addressing potential issues before they escalate, the risk of equipment breakdowns and accidents can be significantly reduced.
Furthermore, ChatGPT's ability to continuously learn and improve its predictive capabilities over time further enhances its accuracy and reliability. As it processes more data and gains experience, its fault prediction models become more refined, allowing for even more precise identification of potential equipment failures.
In conclusion, the integration of ChatGPT into the field of ocean freight fault prediction brings immense value to the industry. By leveraging its data analysis and machine learning capabilities, ChatGPT can help shipping companies prevent downtime, improve operational efficiency, and enhance overall customer satisfaction. With the continuous advancement in AI technology, the future of fault prediction in ocean freight looks promising, ensuring a smoother and more reliable shipping experience for all parties involved.
Comments:
Thank you all for taking the time to read my article on enhancing fault prediction in ocean freight technology with ChatGPT! I would love to hear your thoughts and feedback.
Great article, Lisa! I found the idea of using ChatGPT for fault prediction quite intriguing. It opens up new possibilities in the field. I wonder how accurate the predictions can be.
I agree, Mark. ChatGPT's ability to process and understand vast amounts of data could lead to more accurate predictions. However, it would be interesting to know if it has any limitations. Lisa, what are your thoughts on this?
Lisa, your article was a fascinating read. I can definitely see the potential benefits of using ChatGPT for fault prediction. It could help streamline operations and minimize disruptions. Have you considered any practical use cases for this technology?
Thank you, Michael! Certainly, there are multiple practical use cases for this technology. One example is predicting equipment failures in advance, allowing proactive maintenance to prevent delays and costly repairs. It could also help identify trends and patterns in faults to drive system improvements.
Lisa, I must say, the concept of integrating AI to improve fault prediction in ocean freight technology is quite innovative. However, I'm curious about the potential risks associated with relying heavily on AI algorithms. Any thoughts on this?
Absolutely, Emily. While AI algorithms like ChatGPT can greatly enhance fault prediction, there are risks involved. Factors like biased data, model limitations, and algorithmic errors can impact the accuracy. It's crucial to continuously evaluate and validate the results to minimize potential risks and ensure reliable predictions.
I'm impressed by the potential ChatGPT offers, Lisa. By analyzing data and predicting faults, it can improve efficiency and reduce downtime. However, do you think the implementation of such technology would require a significant upfront investment?
Thank you, Sophie. Implementing ChatGPT for fault prediction does require initial investment, including training the model on relevant data and adapting it to the specific domain. However, the long-term benefits, such as minimizing disruptions and optimizing operations, can outweigh the initial costs in many cases.
Lisa, your article shed light on an exciting application of ChatGPT. The ability to accurately predict faults in ocean freight technology can save time, money, and resources. How scalable do you think this technology is?
Thank you, Caroline. ChatGPT has shown promising scalability. As the model is trained on more data and fine-tuned for specific domains, its fault prediction capabilities can be extended to larger systems, making it viable for widespread use in the ocean freight industry, even for complex and high-volume operations.
This article offers valuable insights, Lisa. Fault prediction is crucial in ensuring operational efficiency. I wonder if ChatGPT can build on historical data and assist in deriving preventive measures to minimize faults and their impacts. Would you recommend such an approach?
Thank you, Robert. Absolutely, ChatGPT can leverage historical data to identify trends and patterns, assisting in the formulation of preventive measures. By understanding the common causes of faults and analyzing past incidents, the system can provide actionable recommendations to minimize the occurrence of future faults and their associated impacts.
Lisa, your article was thought-provoking. However, I'm curious to know if ChatGPT can handle rare or complex faults that may not have sufficient data available for training.
Good point, Oliver. ChatGPT's performance in handling rare or complex faults heavily relies on the availability and quality of training data. In cases where there might be limited data, alternative approaches like transfer learning or anomaly detection techniques can be utilized to supplement the model's understanding. Continuous feedback loops with human experts also help improve predictions for rare and evolving faults.
Lisa, I found your article fascinating. How long does it typically take to implement a system like ChatGPT for fault prediction in ocean freight technology?
I'm glad you found it interesting, Grace. The implementation timeline for integrating ChatGPT for fault prediction can vary depending on factors such as the size of the system, the availability and quality of training data, and the complexity of the integration process. It can take several weeks to months to train and deploy the model, but this investment can lead to long-term benefits in terms of improved fault prediction and system reliability.
Lisa, fantastic article highlighting the potential of ChatGPT for fault prediction. However, do you think there could be any ethical considerations when using AI in this context?
Thank you, Daniel. Ethical considerations are indeed essential when using AI in fault prediction. It's crucial to ensure fairness, avoid discrimination, and address any potential biases in the data and models. Transparency in how predictions are made and involving human oversight and review processes can help mitigate ethical concerns. Additionally, proper data privacy and security measures must be in place to safeguard sensitive information.
Lisa, your article presents an innovative approach. What steps can be taken to validate the accuracy and reliability of ChatGPT's fault predictions?
Thank you, Michelle. Validating the accuracy and reliability of ChatGPT's fault predictions is crucial. It can involve comparing the model's predictions with actual faults and tracking the outcomes over time. Continuous monitoring and feedback loops with domain experts allow refining the model's performance. Various evaluation metrics, like precision, recall, and F1 score, can also be used to assess the model's effectiveness in fault prediction.
Lisa, the potential of ChatGPT for fault prediction in ocean freight technology is impressive. However, have there been any real-world implementations or success stories you can share?
Absolutely, Jason. Real-world implementations of ChatGPT for fault prediction are emerging. For example, in a shipping company, it was used to predict maintenance needs of machinery on cargo ships, reducing downtime and improving operational efficiency. Another success story is from a port where ChatGPT was applied to predict faults in container handling systems, allowing proactive repairs to minimize disruptions. These implementations demonstrate the practical value of the technology.
Lisa, your article is eye-opening. Is ChatGPT already being used in the ocean freight industry, or is it still in the experimental stage?
Thank you, Sarah. While ChatGPT is currently being explored and tested in the ocean freight industry, widespread adoption is still in its early stages. As more organizations recognize the benefits of AI-powered fault prediction, we expect to see increased implementation in the coming years, leading to a more efficient and reliable industry.
Lisa, your article is very insightful. Can ChatGPT be customized to suit specific needs and adapt to unique operational environments?
Absolutely, Ethan. One of the advantages of ChatGPT is its flexibility and customizability. The model can be fine-tuned on specific data from the operational environment, allowing it to adapt and provide fault predictions tailored to the unique needs of the ocean freight industry. This customization ensures better accuracy and relevance in predicting faults for each specific context.
Congratulations on an informative article, Lisa. The integration of AI like ChatGPT into ocean freight technology holds great promise. However, do you anticipate any challenges with data availability or data quality for training such models?
Thank you, Sophia. Data availability and quality can indeed pose challenges when training AI models like ChatGPT. It's crucial to have access to diverse and representative data to ensure the model's performance across different fault scenarios. Data cleaning and preprocessing techniques, along with expert domain knowledge, can help address issues related to missing or noisy data, ensuring the accuracy and reliability of the predictions.
Lisa, your article provides valuable insights into the future of fault prediction in ocean freight technology. Going forward, how do you see AI technologies like ChatGPT evolving in this domain?
Thank you, Jacob. AI technologies like ChatGPT have a promising future in fault prediction for the ocean freight industry. We can expect further advancements in natural language processing models, enabling even better understanding of fault-related data. Increased collaboration between AI systems and domain experts will lead to more accurate predictions, improved fault prevention, and increased operational efficiency. These technologies will continue to evolve and become integral to the future of the industry.
Lisa, your article provides a fresh perspective on fault prediction. However, I'm curious about the training process. Could you elaborate on how ChatGPT is trained for such tasks?
Certainly, Natalie. Training ChatGPT involves initially pretraining the model on a large corpus of publicly available text from the internet to learn grammar, facts, and reasoning abilities. Then, it undergoes domain-specific fine-tuning using task-specific data, i.e., in this case, fault-related data from ocean freight technology. The model is trained to predict the next word in a sentence, which helps it learn the underlying patterns and relationships necessary for fault prediction. This process ensures that ChatGPT becomes proficient in understanding and generating relevant responses within the given domain.
Lisa, this is an exciting article. How do you foresee the integration of ChatGPT for fault prediction impacting the competitiveness of companies in the ocean freight industry?
Thank you, Ava. The integration of ChatGPT for fault prediction can significantly impact the competitiveness of companies in the ocean freight industry. By minimizing disruptions, reducing downtime, and optimizing operations, companies utilizing this technology can gain a competitive edge. The ability to proactively address faults and maintain reliable service can enhance customer satisfaction, improve efficiency, and attract more business opportunities. Hence, early adopters of AI-powered fault prediction stand to gain a significant advantage in the market.
Lisa, your article paints a promising picture for the future of fault prediction using AI. However, I'm curious about the potential impact on employment in the industry. Could AI technologies like ChatGPT replace human expertise entirely?
Great question, Lucas. AI technologies like ChatGPT are meant to complement and assist human expertise rather than replacing it entirely. While these technologies can automate certain tasks and enhance fault prediction capabilities, they still rely on human involvement and decision-making for critical aspects. Human experts play a vital role in interpreting predictions, validating results, and making informed decisions based on AI insights. The integration of AI in fault prediction is about augmenting human capabilities, improving efficiency, and empowering experts, rather than replacing them.
Lisa, your article has brought up some important points regarding fault prediction. I'm curious about the computational resources required to implement a system like ChatGPT. Can it be feasibly integrated into existing infrastructure?
Thank you, Harper. Implementing ChatGPT for fault prediction does require computational resources, especially during the training phase. However, once the model is trained and deployed, the ongoing resource requirements for prediction tasks are relatively lower. Depending on the existing infrastructure and scalability requirements, the necessary computational resources can be provisioned to accommodate the deployment of ChatGPT. The integration can be customized to suit the existing hardware and software environment, making it feasible for practical implementation.
Lisa, your article highlights the potential behind ChatGPT for fault prediction. I wonder if there are any other AI models or techniques that can be combined with ChatGPT to further improve prediction accuracy?
Thank you, Ryan. ChatGPT can certainly benefit from combining other AI models or techniques. For instance, anomaly detection algorithms can be used in conjunction with ChatGPT to identify and handle rare or unusual faults that might not have sufficient training data. Additionally, ensemble methods that merge predictions from multiple models can enhance the overall accuracy of fault prediction. Leveraging a combination of various AI techniques allows us to leverage their respective strengths and address potential limitations, leading to more accurate and reliable predictions.
Lisa, your article is informative. However, I'm curious about the interpretability of ChatGPT's predictions. Can it provide insights into why a fault is predicted or suggest potential underlying causes?
Good point, Isabella. The interpretability of ChatGPT's predictions is an important aspect. While ChatGPT can generate fault predictions, its inherent design as a language model makes it challenging to provide detailed insights into why a fault is predicted or suggest specific underlying causes. However, by combining ChatGPT's predictions with explainable AI techniques or using approaches like counterfactual reasoning, we can make progress in generating more interpretable outputs. Enhancing the interpretability of AI models is an active area of research that can further boost the value of fault predictions.
Lisa, your article brings up fascinating possibilities for the ocean freight industry. Could ChatGPT be extended beyond fault prediction to other areas of operations, such as optimizing routes or reducing inefficiencies?
Absolutely, David. ChatGPT's potential extends beyond fault prediction. It can be applied to various areas of operations in the ocean freight industry. For example, it can optimize routes by considering weather patterns, traffic, and vessel capacities. It can help reduce inefficiencies in cargo handling and storage, contributing to improved resource allocation. With appropriate adaptation and training, ChatGPT can provide valuable insights and assistance across multiple aspects of the industry, enhancing overall efficiency and performance.
Lisa, your article is eye-opening. However, I'm curious if there are any regulatory or legal considerations to keep in mind when implementing ChatGPT for fault prediction in the ocean freight industry.
Good question, Emma. When implementing ChatGPT for fault prediction or any AI technology, it's important to consider regulatory and legal aspects. Depending on the jurisdiction, data privacy, security, and compliance regulations must be followed. Transparency in the use of AI and accountability for the system's decisions are also crucial. Additionally, collaboration with legal experts can help ensure the implementation aligns with applicable laws and regulations governing the ocean freight industry. Adhering to ethical principles and industry standards is key for responsible and successful integration of AI technologies.
Lisa, your article presents an exciting future for fault prediction. However, could the performance of ChatGPT be impacted by external factors like environmental changes or technological advancements?
Great point, Caleb. External factors like environmental changes or technological advancements can certainly impact the performance of ChatGPT for fault prediction. For instance, if there are sudden shifts in operating conditions or substantial changes in the technology being used, the model's predictions might require recalibration or fine-tuning to stay accurate. Continuous monitoring and adaptation to evolving circumstances are crucial to ensure the reliability and effectiveness of the model. As the industry progresses, staying attuned to such external factors becomes vital in maintaining fault prediction performance.
Lisa, your article provides great insights into fault prediction. Are there any potential limitations or challenges in implementing ChatGPT that you think should be considered?
Thank you, Victoria. Implementing ChatGPT for fault prediction comes with a few considerations. Firstly, obtaining high-quality, labeled fault-related data for training is crucial to ensure accurate predictions. Secondly, challenges related to model interpretability and explaining the reasoning behind predictions need to be addressed. Additionally, ongoing model maintenance and adaptation to changing scenarios should be planned for to maintain the predictive performance. Addressing these limitations and challenges with proper strategies and collaboration can lead to successful implementation and harnessing the full potential of ChatGPT for fault prediction.