Enhancing FMEA in Technology with ChatGPT: Empowering Risk Assessment and Mitigation
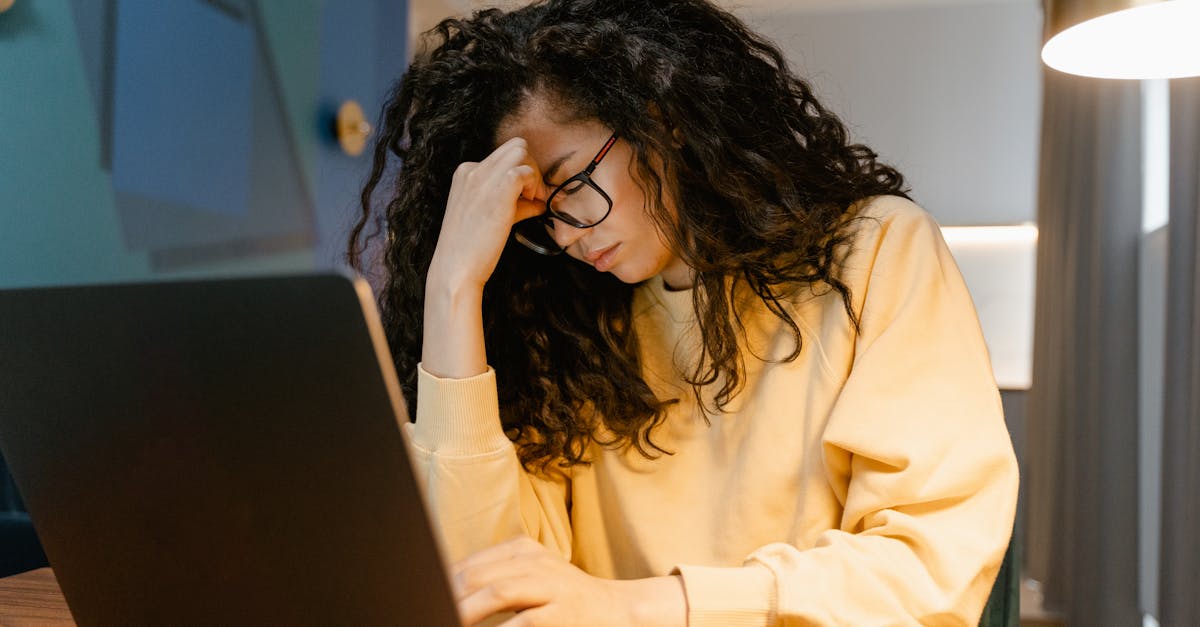
Failure Mode and Effects Analysis (FMEA) is an important technology used in various industries to identify potential failures and their associated risks. In the context of ChatGPT-4, which is an advanced conversational AI model, FMEA can be utilized to analyze past records and proactively identify potential failures in its performance. By employing FMEA techniques, preventive measures can be suggested to enhance the overall reliability and usability of ChatGPT-4.
What is FMEA?
FMEA is a systematic approach used to identify potential failure modes, their causes, and the effects of these failures. It allows organizations to outline possible risks, prioritize them, and develop suitable preventive actions. FMEA is commonly applied during the design and development stages of a product or process to prevent failures or mitigate their impact.
FMEA in Failure Identification for ChatGPT-4
ChatGPT-4, being an advanced conversational AI model, interacts with users and responds to their queries and requests. To ensure a smooth and reliable user experience, it is crucial to identify potential failure modes specific to ChatGPT-4's performance. By using FMEA techniques, ChatGPT-4 can analyze past FMEA records, examining both internal and external factors that can lead to failures.
Some potential failure modes in ChatGPT-4 could include incorrect or misleading responses, inability to comprehend complex queries, or sensitivity to specific triggers that may cause undesirable outputs. By analyzing past FMEA records, ChatGPT-4 can identify these failure modes, their causes, and the potential effects they may have on user interactions. This information is then used to design preventive measures that address these failures proactively.
Usage of FMEA in ChatGPT-4
The usage of FMEA in ChatGPT-4 revolves around utilizing historical records of failure modes and their associated causes. By analyzing these records, patterns and trends can be identified, enabling the proactive identification of potential failures in future interactions. With this information, preventive measures can be implemented, such as fine-tuning the model, updating the underlying algorithms, or introducing new training data specific to identified failure modes.
By regularly applying FMEA to ChatGPT-4, continuous improvements can be made, ensuring a more reliable and efficient conversational AI model. Feedback from users can also be incorporated into the FMEA process, enabling further refinements to prevent known failures and enhance the overall user experience.
Conclusion
The application of FMEA in the context of ChatGPT-4 enables the identification of potential failure modes and the suggestion of preventive measures. By analyzing past FMEA records, ChatGPT-4 can detect failure patterns, their causes, and their effects on user interactions. This information empowers developers and engineers to take proactive actions to enhance the reliability, accuracy, and robustness of ChatGPT-4. As conversational AI models evolve, the usage of FMEA becomes increasingly valuable in ensuring a seamless user experience.
Comments:
Great article, Mike! I've always wondered how AI can be used in risk assessment. Looking forward to reading more about it.
Thanks, Sarah! AI has a lot of potential in risk assessment. It can help identify potential failure modes and suggest mitigation strategies. Feel free to ask any questions you have!
Interesting topic, Mike. I believe incorporating AI in FMEA can greatly enhance its effectiveness. It can analyze vast amounts of data quickly and offer valuable insights.
Definitely, David! AI can process large datasets, identify patterns, and assist in prioritizing risks. It can make the risk assessment process more efficient and accurate.
I've been using FMEA for risk assessment, and it can be quite time-consuming. How can ChatGPT specifically help speed up the process?
Good question, Emily! ChatGPT can provide real-time suggestions during risk assessment by generating potential failure modes and corresponding mitigations based on its training data. It can streamline the brainstorming process and accelerate mitigation planning.
The integration of AI with FMEA sounds promising. Can ChatGPT also help in identifying potential failure modes that were not previously considered?
Absolutely, Tom! ChatGPT can identify failure modes that may have been overlooked by human analysts. It can suggest new insights and help enhance the comprehensiveness of FMEA.
I can see the benefits of using AI, but how do we ensure that the suggested failure modes and mitigations are accurate and reliable?
Valid concern, Julia. While ChatGPT can generate useful suggestions, it's crucial for human analysts to review and validate them. AI is a tool to assist, but human expertise is still necessary for accurate risk assessment.
It's fascinating how AI is advancing across various domains. Incorporating it into FMEA will certainly improve risk analysis and decision-making.
Indeed, Rachel! AI technologies like ChatGPT bring valuable capabilities to risk assessment, allowing us to leverage their strengths for better outcomes.
I wonder if AI can also help in automating the documentation and reporting aspects of FMEA?
That's a great point, Mark! AI can assist in automating report generation by summarizing the identified failure modes, associated risks, and suggested mitigations. It can save time and reduce the manual effort required.
Mike, could you provide some examples of how ChatGPT has been successfully utilized in the technology industry for risk assessment?
Certainly, Sarah! In the technology industry, ChatGPT has been applied to identify failure modes in software systems, recommend security measures to mitigate cyber risks, and analyze potential risks in hardware components. It's a versatile tool for various technology-related risk assessments.
I'm impressed by the potential of AI in risk assessment. Mike, do you think ChatGPT will become a standard tool for FMEA in the future?
It's quite likely, David. As AI technologies continue to evolve and improve, integrating them into FMEA processes could become a standard practice. However, human expertise and validation will always be vital in ensuring accurate risk assessment.
I agree, Mike. AI can enhance FMEA, but human judgment is indispensable to make the final decisions and assess the context-specific impact of identified failure modes.
Exactly, Julia! Human judgment is crucial in considering factors like system dependencies, unique use cases, and practical constraints. ChatGPT can assist, but human analysts bring the necessary context for effective risk assessment.
I appreciate how you emphasize the collaboration between AI and human analysts, Mike. It's essential to leverage the strengths of both for better outcomes.
Absolutely, Tom! The synergy between AI and human analysts can lead to more comprehensive and reliable risk assessments. It's an exciting time for advancements in risk assessment methodologies.
Thanks for the insightful conversation, everyone! I now have a clearer understanding of the potential benefits of using ChatGPT in risk assessment.
You're welcome, Emily! I'm glad I could assist. If you have any more questions in the future, feel free to reach out. Happy risk assessing!
Thank you, Mike! This discussion has been enlightening. Excited to see how AI continues to shape risk assessment practices.
Thank you, Rachel! AI indeed brings exciting possibilities to risk assessment. Stay tuned for more advancements in this field!
Great article, Mike! AI integration with FMEA can be a game-changer, reducing human bias and expanding the scope of risk assessment.
Thanks, John! AI integration can indeed help address biases and provide a more objective analysis. It's an exciting development in the field of risk management.
I've been using FMEA for quality control, but I didn't consider the potential of AI in the process. This article opened my eyes to new possibilities.
That's great to hear, Amy! AI can bring valuable insights to quality control practices. Feel free to explore further and discover how it can enhance your FMEA process.
AI is revolutionizing many industries, and now it's helping in risk assessment. Exciting times we live in!
Indeed, Daniel! AI's impact is far-reaching, and its applications in risk assessment hold great promise. Exciting indeed!
While AI can enhance risk assessment, it's crucial to ensure proper data quality and model understanding for reliable results.
Absolutely, Laura! Data quality and model transparency are crucial for reliable risk assessment. Careful evaluation and validation are essential steps in utilizing AI effectively.
With the rapid advancements in AI, do you think there will be any ethical concerns related to using AI in risk assessment?
Good question, Gregory. Ethical concerns may arise when relying solely on AI-generated insights. Human oversight and accountability are necessary to ensure ethical risk assessment practices.
I can see AI improving risk assessment efficiency, but how accessible is it for smaller companies with limited resources?
Valid concern, Emma. AI adoption can be a challenge for smaller companies, but as technology progresses, more accessible tools and frameworks are being developed. It's an exciting area to watch for future advancements.
AI can undoubtedly enhance risk assessment processes. However, it's important to consider potential security risks associated with AI implementation as well.
Absolutely, Oliver! Security risks should be carefully considered when implementing AI systems. Privacy, data protection, and model vulnerabilities need to be addressed for a robust risk assessment framework.
Mike, could you provide some examples of how ChatGPT has been successfully utilized in the technology industry for risk assessment?
Certainly, Sarah! In the technology industry, ChatGPT has been applied to identify failure modes in software systems, recommend security measures to mitigate cyber risks, and analyze potential risks in hardware components. It's a versatile tool for various technology-related risk assessments.
I'm impressed by the potential of AI in risk assessment. Mike, do you think ChatGPT will become a standard tool for FMEA in the future?
It's quite likely, David. As AI technologies continue to evolve and improve, integrating them into FMEA processes could become a standard practice. However, human expertise and validation will always be vital in ensuring accurate risk assessment.
I agree, Mike. AI can enhance FMEA, but human judgment is indispensable to make the final decisions and assess the context-specific impact of identified failure modes.
Exactly, Julia! Human judgment is crucial in considering factors like system dependencies, unique use cases, and practical constraints. ChatGPT can assist, but human analysts bring the necessary context for effective risk assessment.
I appreciate how you emphasize the collaboration between AI and human analysts, Mike. It's essential to leverage the strengths of both for better outcomes.
Absolutely, Tom! The synergy between AI and human analysts can lead to more comprehensive and reliable risk assessments. It's an exciting time for advancements in risk assessment methodologies.
Thanks for the insightful conversation, everyone! I now have a clearer understanding of the potential benefits of using ChatGPT in risk assessment.
You're welcome, Emily! I'm glad I could assist. If you have any more questions in the future, feel free to reach out. Happy risk assessing!
Thank you, Mike! This discussion has been enlightening. Excited to see how AI continues to shape risk assessment practices.
Thank you, Rachel! AI indeed brings exciting possibilities to risk assessment. Stay tuned for more advancements in this field!
Great article, Mike! AI integration with FMEA can be a game-changer, reducing human bias and expanding the scope of risk assessment.
Thanks, John! AI integration can indeed help address biases and provide a more objective analysis. It's an exciting development in the field of risk management.
I've been using FMEA for quality control, but I didn't consider the potential of AI in the process. This article opened my eyes to new possibilities.
That's great to hear, Amy! AI can bring valuable insights to quality control practices. Feel free to explore further and discover how it can enhance your FMEA process.
AI is revolutionizing many industries, and now it's helping in risk assessment. Exciting times we live in!
Indeed, Daniel! AI's impact is far-reaching, and its applications in risk assessment hold great promise. Exciting indeed!
While AI can enhance risk assessment, it's crucial to ensure proper data quality and model understanding for reliable results.
Absolutely, Laura! Data quality and model transparency are crucial for reliable risk assessment. Careful evaluation and validation are essential steps in utilizing AI effectively.
With the rapid advancements in AI, do you think there will be any ethical concerns related to using AI in risk assessment?
Good question, Gregory. Ethical concerns may arise when relying solely on AI-generated insights. Human oversight and accountability are necessary to ensure ethical risk assessment practices.
I can see AI improving risk assessment efficiency, but how accessible is it for smaller companies with limited resources?
Valid concern, Emma. AI adoption can be a challenge for smaller companies, but as technology progresses, more accessible tools and frameworks are being developed. It's an exciting area to watch for future advancements.
AI can undoubtedly enhance risk assessment processes. However, it's important to consider potential security risks associated with AI implementation as well.
Absolutely, Oliver! Security risks should be carefully considered when implementing AI systems. Privacy, data protection, and model vulnerabilities need to be addressed for a robust risk assessment framework.
Mike, could you provide some examples of how ChatGPT has been successfully utilized in the technology industry for risk assessment?
Certainly, Sarah! In the technology industry, ChatGPT has been applied to identify failure modes in software systems, recommend security measures to mitigate cyber risks, and analyze potential risks in hardware components. It's a versatile tool for various technology-related risk assessments.
I'm impressed by the potential of AI in risk assessment. Mike, do you think ChatGPT will become a standard tool for FMEA in the future?
It's quite likely, David. As AI technologies continue to evolve and improve, integrating them into FMEA processes could become a standard practice. However, human expertise and validation will always be vital in ensuring accurate risk assessment.
I agree, Mike. AI can enhance FMEA, but human judgment is indispensable to make the final decisions and assess the context-specific impact of identified failure modes.
Exactly, Julia! Human judgment is crucial in considering factors like system dependencies, unique use cases, and practical constraints. ChatGPT can assist, but human analysts bring the necessary context for effective risk assessment.
I appreciate how you emphasize the collaboration between AI and human analysts, Mike. It's essential to leverage the strengths of both for better outcomes.
Absolutely, Tom! The synergy between AI and human analysts can lead to more comprehensive and reliable risk assessments. It's an exciting time for advancements in risk assessment methodologies.
Thanks for the insightful conversation, everyone! I now have a clearer understanding of the potential benefits of using ChatGPT in risk assessment.
You're welcome, Emily! I'm glad I could assist. If you have any more questions in the future, feel free to reach out. Happy risk assessing!
Thank you, Mike! This discussion has been enlightening. Excited to see how AI continues to shape risk assessment practices.
Thank you, Rachel! AI indeed brings exciting possibilities to risk assessment. Stay tuned for more advancements in this field!
Great article, Mike! AI integration with FMEA can be a game-changer, reducing human bias and expanding the scope of risk assessment.
Thanks, John! AI integration can indeed help address biases and provide a more objective analysis. It's an exciting development in the field of risk management.
I've been using FMEA for quality control, but I didn't consider the potential of AI in the process. This article opened my eyes to new possibilities.
That's great to hear, Amy! AI can bring valuable insights to quality control practices. Feel free to explore further and discover how it can enhance your FMEA process.
AI is revolutionizing many industries, and now it's helping in risk assessment. Exciting times we live in!
Indeed, Daniel! AI's impact is far-reaching, and its applications in risk assessment hold great promise. Exciting indeed!
While AI can enhance risk assessment, it's crucial to ensure proper data quality and model understanding for reliable results.
Absolutely, Laura! Data quality and model transparency are crucial for reliable risk assessment. Careful evaluation and validation are essential steps in utilizing AI effectively.
With the rapid advancements in AI, do you think there will be any ethical concerns related to using AI in risk assessment?
Good question, Gregory. Ethical concerns may arise when relying solely on AI-generated insights. Human oversight and accountability are necessary to ensure ethical risk assessment practices.
I can see AI improving risk assessment efficiency, but how accessible is it for smaller companies with limited resources?
Valid concern, Emma. AI adoption can be a challenge for smaller companies, but as technology progresses, more accessible tools and frameworks are being developed. It's an exciting area to watch for future advancements.
AI can undoubtedly enhance risk assessment processes. However, it's important to consider potential security risks associated with AI implementation as well.
Absolutely, Oliver! Security risks should be carefully considered when implementing AI systems. Privacy, data protection, and model vulnerabilities need to be addressed for a robust risk assessment framework.
Mike, could you provide some examples of how ChatGPT has been successfully utilized in the technology industry for risk assessment?
Certainly, Sarah! In the technology industry, ChatGPT has been applied to identify failure modes in software systems, recommend security measures to mitigate cyber risks, and analyze potential risks in hardware components. It's a versatile tool for various technology-related risk assessments.
I'm impressed by the potential of AI in risk assessment. Mike, do you think ChatGPT will become a standard tool for FMEA in the future?
It's quite likely, David. As AI technologies continue to evolve and improve, integrating them into FMEA processes could become a standard practice. However, human expertise and validation will always be vital in ensuring accurate risk assessment.
I agree, Mike. AI can enhance FMEA, but human judgment is indispensable to make the final decisions and assess the context-specific impact of identified failure modes.
Exactly, Julia! Human judgment is crucial in considering factors like system dependencies, unique use cases, and practical constraints. ChatGPT can assist, but human analysts bring the necessary context for effective risk assessment.
I appreciate how you emphasize the collaboration between AI and human analysts, Mike. It's essential to leverage the strengths of both for better outcomes.
Absolutely, Tom! The synergy between AI and human analysts can lead to more comprehensive and reliable risk assessments. It's an exciting time for advancements in risk assessment methodologies.
Thank you for reading my article on enhancing FMEA in technology with ChatGPT! I'm excited to hear your thoughts and opinions.
Great article, Mike! FMEA is such an important aspect of risk assessment in technology. Integrating ChatGPT seems like a smart way to improve the process.
I'm not familiar with ChatGPT, but after reading your article, it seems like it could be a game-changer for risk assessment in technology. Can you explain a bit more about how it works?
Sure, Maria! ChatGPT is a language model developed by OpenAI. It uses deep learning techniques to generate human-like text based on the input it receives. In the context of FMEA, ChatGPT can assist users in identifying potential risks and suggesting mitigation strategies.
I'm a big believer in the power of AI, but when it comes to risk assessment in technology, I feel like human expertise is essential. How can ChatGPT replicate that level of expertise?
Valid point, John. While ChatGPT may not replicate human expertise entirely, it can complement it by providing suggestions and insights based on its training data. It can be a helpful tool to assist experts in identifying potential risks that may have been overlooked.
I see the potential value of ChatGPT in risk assessment, but what about its limitations? Can it provide accurate and reliable recommendations, especially in complex technological systems?
That's a valid concern, Emily. ChatGPT's recommendations should be considered as suggestions rather than absolute truths. It's important to validate and verify the recommendations using real-world expertise and knowledge. ChatGPT can help in generating ideas, but the final decision should always be made by human experts.
As an engineer, I can see the potential of using ChatGPT in FMEA. It could help speed up the process, especially when dealing with large-scale systems. However, I'm also worried about potential biases in the training data that could affect the recommendations. How is that addressed?
Great question, Sarah. Addressing biases is crucial. OpenAI makes efforts to reduce both glaring and subtle biases in ChatGPT. They continuously improve the model and actively seek feedback from users to minimize any negative impacts. Bias is indeed an important aspect to consider when applying such AI models to risk assessment.
I appreciate the potential of ChatGPT in enhancing FMEA, but I'm concerned about the ethical implications. How can we ensure that the use of AI in risk assessment doesn't compromise privacy or result in unintended consequences?
Ethics is a vital consideration, David. When implementing ChatGPT or any AI system, it's important to ensure data privacy, establish clear guidelines for usage, and have appropriate risk mitigation processes in place. Transparency and accountability are key in safeguarding against unintended consequences.
I can see the benefits of using ChatGPT for risk assessment, but what about the potential for malicious use? How can we prevent bad actors from exploiting the system?
You raise a valid concern, Jennifer. Implementing safeguards like user authentication, access controls, and monitoring can help prevent misuse. Additionally, continually updating and improving the model's training data can help make it more robust against malicious intents.
I'm curious about the scalability of ChatGPT in FMEA. Can it handle large-scale systems with complex interdependencies effectively?
Scalability is an important aspect, Alex. ChatGPT can be trained on large amounts of relevant data, which helps it understand complex interdependencies and improve its recommendations. However, there may still be limitations in certain highly specialized domains. Continuous model development and domain-specific fine-tuning can help address scalability concerns.
I'm concerned about the potential impact on employment in risk assessment roles. Could the integration of ChatGPT in FMEA lead to job losses?
That's a valid concern, Linda. While ChatGPT can increase efficiency and productivity, it's important to remember that it is meant to augment human expertise, not replace it entirely. Human judgment, domain knowledge, and critical thinking skills will continue to play a significant role in risk assessment. It's more of a collaboration between humans and AI rather than a complete replacement of human roles.
ChatGPT sounds promising, but do you foresee any challenges in implementing it in real-world organizations, especially regarding integration with existing risk assessment processes?
Integration can indeed be a challenge, Chris. Organizations need to assess their existing processes and workflows to identify the most suitable points for ChatGPT integration. It may require training and onboarding users, addressing any technical hurdles, and ensuring compatibility with existing tools and systems. However, with proper planning and implementation, the benefits can outweigh the challenges.
I agree, Mike. The key is to view ChatGPT as a valuable tool that can enhance risk assessment rather than as a replacement. Human judgment and expertise should remain at the forefront while leveraging the capabilities of AI.
Thank you for explaining, Mike. I have a better understanding of how ChatGPT can benefit FMEA in technology. It's definitely an exciting development!
I appreciate your insights, Mike. It's important to strike the right balance between AI and human expertise when it comes to risk assessment.
Valid point about using ChatGPT's recommendations as suggestions rather than absolute truths. Human experts should always have the final say in decision-making.
I'm glad to hear that efforts are made to address biases in ChatGPT. It's important to strive for fairness and avoid perpetuating biases in risk assessment.
Transparency, security, and ethical considerations are definitely crucial when implementing AI systems for risk assessment. Thanks for highlighting that, Mike.
Preventing malicious use is a valid concern. It's essential to implement robust security measures to protect against misuse of AI systems.
It's reassuring to know that ChatGPT can handle large-scale systems effectively. Scalability is key when it comes to risk assessment in complex technological environments.
I agree, Mike. AI should be seen as a tool to assist and enhance risk assessment, not as a threat to job roles. Human judgment remains irreplaceable.
Proper planning and integration are important for successful implementation. Organizations should take the necessary steps to ensure a smooth adoption of ChatGPT in their risk assessment processes.
Absolutely, Mike. The collaboration between human expertise and AI capabilities is where the true potential lies.
Indeed, the advancements in AI, like ChatGPT, are incredibly exciting. They can revolutionize risk assessment in technology.
Finding the right balance between human judgment and AI assistance is key to effective risk assessment. It's an evolving field.
I'm glad the article addressed the importance of validation and verification of ChatGPT's recommendations. It's crucial to rely on real-world expertise in decision-making.
Addressing biases in AI models like ChatGPT is a continuous effort. Collaboration between AI developers and domain experts can help achieve more accurate and fair risk assessments.
Ethical considerations cannot be overlooked when implementing AI systems for risk assessment. Transparency and accountability are critical for responsible use.
Safeguards against malicious use are vital in the adoption of AI-assisted risk assessment. Users should be properly authenticated, and access controls should be in place.
Having a scalable AI system like ChatGPT is a huge advantage when analyzing large-scale technological systems. It can save valuable time and resources.
It's reassuring to know that human roles in risk assessment won't be replaced entirely by AI. Collaboration between humans and AI can lead to more effective outcomes.
Integration challenges are common when adopting new technologies. With careful planning and implementation, ChatGPT can be successfully integrated into existing risk assessment processes.
Indeed, Robert. Human judgment, expertise, and critical thinking still have an irreplaceable role in risk assessment. AI can enhance and assist but not replace domain expertise.
The potential of AI, like ChatGPT, to augment our capabilities in risk assessment is truly exciting. It opens up new possibilities for improving safety and reliability in technology.
AI technologies should be embraced as tools that enable experts to make more informed decisions, not as complete replacements for human judgment.
Validation and verification are crucial aspects of any AI system used in risk assessment. The final decision should always rely on human expertise and domain knowledge.
Ensuring fairness and avoiding biases in AI models is an ongoing process. Continuous improvement and user feedback are essential components in that journey.
Preventing malicious use of AI systems is a shared responsibility. Industry standards and regulations play a vital role in addressing this concern.
Scalability is a key factor in the effectiveness and efficiency of risk assessment. AI systems like ChatGPT can handle the complexities of large-scale technological systems effectively.
AI should be seen as a collaborative tool that complements human expertise. It can help improve the risk assessment process rather than replacing human roles.
Integration challenges are common, but with proper planning and implementation, ChatGPT can integrate seamlessly into existing risk assessment processes, enhancing their capabilities.
Absolutely, Emily. The synergy between human judgment and AI assistance can result in more effective and reliable risk assessments.