Enhancing Hardware Support Efficiency: Leveraging ChatGPT for Predictive Maintenance
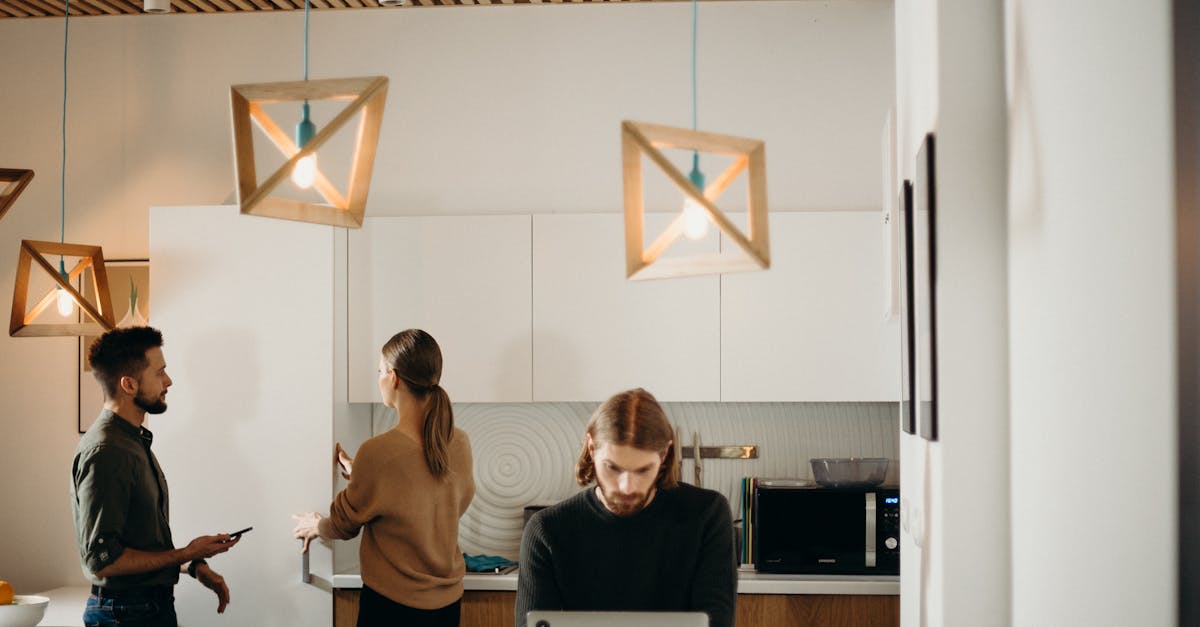
In recent years, advancements in technology have allowed us to harness the power of predictive maintenance to minimize downtime and maximize efficiency. Predictive maintenance uses data and analytics to predict potential hardware malfunctions before they occur, allowing for proactive solutions to be implemented. One crucial aspect of predictive maintenance is hardware support, which plays a vital role in ensuring smooth operations and preventing costly disruptions.
Technology
The technology used in hardware support for predictive maintenance relies on various sensors and monitoring devices to collect data about equipment performance. This data is then analyzed using advanced algorithms and machine learning techniques to identify patterns and anomalies that indicate potential hardware malfunctions.
Hardware support technology encompasses a wide range of devices and systems, including vibration sensors, temperature sensors, pressure sensors, and many others. These devices are strategically placed on equipment, capturing real-time data that can be fed into predictive maintenance software.
Area: Predictive Maintenance
Predictive maintenance is a practice that aims to predict equipment failure or maintenance needs before they happen. By analyzing data gathered from sensors and other sources, predictive maintenance systems can detect early warning signs of impending hardware malfunctions. This allows maintenance teams to intervene and address issues with targeted and proactive solutions, minimizing downtime and reducing repair costs.
Predictive maintenance can be applied to a wide range of industries, including manufacturing, transportation, healthcare, and energy. By implementing predictive maintenance strategies, organizations can significantly improve their overall operational efficiency while reducing the risks associated with unexpected equipment failures.
Usage: Predicts Potential Hardware Malfunctions Facilitating Proactive Solutions
The primary purpose of hardware support in predictive maintenance is to detect potential hardware malfunctions early on, enabling proactive solutions to be implemented. By continuously monitoring equipment health and performance, hardware support technologies can identify deviations from normal operating conditions that may indicate impending failures.
For example, if a temperature sensor detects a sudden rise in temperature beyond a defined threshold, it could indicate an overheating issue. Prompt notifications can be sent to the maintenance team, who can then take corrective actions before a complete breakdown occurs. By addressing the issue proactively, organizations can avoid costly downtime and potential safety hazards.
Hardware support in predictive maintenance also enables remote monitoring, allowing maintenance teams to access real-time data from anywhere. This remote monitoring capability offers flexibility and convenience, especially for organizations with multiple locations or remote assets. It enables maintenance personnel to prioritize and schedule repairs, ultimately reducing unplanned downtime and optimizing maintenance efforts.
Conclusion
Hardware support is an essential component of predictive maintenance systems. By leveraging a variety of sensors and monitoring devices, hardware support technologies enable organizations to predict potential hardware malfunctions and take proactive measures. Early detection and intervention can significantly reduce downtime, increase equipment lifespan, and ultimately improve operational efficiency. The implementation of hardware support for predictive maintenance is an investment that pays off in terms of improved productivity, reduced costs, and increased customer satisfaction.
Comments:
This article presents an interesting approach to improving hardware support efficiency. Leveraging ChatGPT for predictive maintenance seems like a promising idea. I'm curious to learn more about the potential benefits and challenges of implementing such a solution.
I completely agree, Jack. Predictive maintenance can definitely help in minimizing downtime and optimizing resources. I wonder if there are any specific industries or types of hardware where this approach has been successfully applied already?
Thank you, Jack and Emily, for your interest in the topic. Predictive maintenance using ChatGPT has shown potential benefits across various sectors, including manufacturing, energy, and transportation. By monitoring real-time sensor data and using machine learning models, it is possible to predict potential hardware failures and schedule proactive maintenance. However, challenges such as data accuracy and model training should be carefully addressed.
I'm skeptical about relying solely on ChatGPT for predictive maintenance. While it can assist in identifying potential issues, human expertise and domain knowledge should still be essential in making informed decisions. It would be interesting to hear more about how ChatGPT integrates with the existing maintenance workflows.
Great point, Robert. Machines might not interpret data in the same way humans do. ChatGPT could be an excellent aid, but human judgment and expertise need to work hand in hand with technology. The integration of ChatGPT within maintenance teams and workflows is a crucial aspect to consider.
You raise a valid concern, Robert. While ChatGPT can provide valuable insights, it should be seen as a tool to support human decision-making rather than a standalone solution. Effective integration involves defining clear roles for both machine learning models and human experts, ensuring their collaboration to achieve better maintenance outcomes.
I find the idea fascinating, but I worry about the associated costs of implementing predictive maintenance using ChatGPT. Are there any estimates on the initial investment required, ongoing maintenance expenses, and the expected return on investment?
Indeed, Sophia, the costs associated with implementing predictive maintenance using ChatGPT should be carefully evaluated. Initial investments can vary depending on factors such as the scale, complexity, and existing infrastructure of the system. Conducting a cost-benefit analysis and assessing the potential savings and operational improvements would enable organizations to make informed decisions.
Sophia, that's an important consideration. Implementing predictive maintenance can require significant upfront investment in infrastructure, data collection, and model development. However, the potential benefits, such as reduced operational costs, increased equipment lifespan, and minimized unplanned downtime, can result in substantial long-term returns on investment.
I wonder if ChatGPT can handle the diversity of hardware systems and models out there. Not all devices and equipment are the same, and they may generate data in different formats. It would be interesting to know how adaptable and scalable the ChatGPT approach is in real-world scenarios.
That's a great question, Lily. The adaptability of ChatGPT to different hardware systems and data formats is crucial. Juliette, could you elaborate on how the model can handle these challenges and whether any customization is required to fit specific industrial contexts?
I think it's important to understand that while ChatGPT can provide valuable insights, it might not address all hardware-specific nuances out of the box. Depending on the domain, some customization and fine-tuning could be necessary to adapt the model to a specific industry context.
The potential of predictive maintenance using ChatGPT is exciting, but what about the security implications? Considering that real-time sensor data is involved, how can we ensure that the predictive models are not vulnerable to exploitation or manipulation?
I second that concern, Adam. Maintaining the integrity and security of the data and models is crucial. Juliette, it would be helpful to hear about any measures in place to tackle potential security vulnerabilities associated with predictive maintenance.
Security is indeed a paramount consideration, Adam. Data encryption, access controls, and secure data handling practices should be put in place to mitigate risks. Robust model validation and integrity checks along with regular updates and monitoring can help maintain secure and reliable predictive maintenance systems.
I'm fascinated by the potential of ChatGPT in the field of predictive maintenance. However, I'm curious about the limitations and potential drawbacks of using this approach. Juliette, could you shed some light on any challenges that organizations might face while implementing predictive maintenance with ChatGPT?
I'm glad you brought up that question, Sophie. While implementing predictive maintenance with ChatGPT can offer several benefits, some challenges include the need for sufficient high-quality training data, continuous model optimization, and interpretability of model outputs. These are a few areas that require careful consideration and ongoing refinement.
I agree, Jack. Interpreting ChatGPT's predictions and understanding the reasoning behind its responses can be challenging. In certain situations, a lack of interpretability might restrict professionals' trust in the model. Organizations should work on making the decision-making process transparent and explainable to ensure confidence in the technology.
Transparency is indeed crucial, Olivia. Professionals need to understand how ChatGPT arrives at its recommendations to make informed decisions. Additionally, continuous monitoring and auditing of the models' performance can help ensure that any biases or limitations are timely identified and addressed.
Absolutely, Robert. Bias mitigation and fairness considerations should be an integral part of the model development process. Transparency and accountability in AI systems can help minimize inadvertent biases and promote trust among users and stakeholders.
Thanks for addressing my question, Jack. I can see how the challenges you mentioned need careful attention to ensure the effectiveness of predictive maintenance using ChatGPT. It's essential to continuously learn from real-world deployments and iterate on the approaches to maximize the benefits and reliability.
Data quality is indeed crucial, Jack. Garbage in, garbage out. Ensuring high-quality training data is vital for accurate and reliable predictions. It requires thorough data collection protocols and data cleaning processes to eliminate noisy, incomplete, or biased data that could adversely affect the predictions.
You're absolutely right, Robert. Data quality is the foundation for accurate predictions. Organizations must implement robust data validation processes and ensure ongoing data hygiene practices to maintain the effectiveness of predictive maintenance systems.
Data quality is indeed crucial, Robert. Garbage data leads to unreliable predictions and potentially inaccurate maintenance decisions. Investing in a robust data management strategy and carefully curating high-quality data will pave the way for more accurate and effective predictive maintenance outcomes.
How do you see the adoption of ChatGPT for predictive maintenance evolving in the near future? Do you think it will become a mainstream practice across various industries?
That's an excellent question, Jonathan. As the technology matures, we can expect wider adoption of ChatGPT for predictive maintenance. However, successful implementation will rely on addressing the challenges I mentioned earlier, acquiring high-quality data, promoting collaboration between humans and models, and building trust in the technology across industries. Continued research and development will undoubtedly shape the future of this field.
Absolutely, ensuring data integrity and protection should be a priority. Regular security audits and ongoing collaboration between data science and cybersecurity teams can help identify and mitigate vulnerabilities proactively.
I believe that with advancements in data collection, processing power, and machine learning, the adoption of ChatGPT for predictive maintenance has the potential to become more mainstream. As it proves its value and reliability, organizations can use it to gain a competitive edge and improve their overall operational efficiency.
This article opens up exciting possibilities for the future of maintenance practices. The integration of AI technologies like ChatGPT in predictive maintenance could significantly transform how organizations manage their assets and improve their overall operational efficiency.
I share your enthusiasm, Alex. Leveraging AI-driven predictive maintenance can create a paradigm shift in how organizations approach maintenance, moving from reactive to proactive strategies. By addressing issues before they escalate, companies can reduce costs and optimize their resource allocation.
Absolutely, Emily. Proactive maintenance can lead to reduced downtime, increased productivity, and improved customer satisfaction. Leveraging AI technologies like ChatGPT offers a promising avenue to achieve these goals.
Adaptability is key, Lily. ChatGPT should be able to handle diverse data formats and hardware systems. The scalability and generalization capabilities of the model need to be thoroughly evaluated to ensure effectiveness across different industrial contexts.
Indeed, Emily. It's essential to validate ChatGPT's performance on various hardware systems and ensure that the model remains adaptable as new technologies and data formats emerge.
Emily, I agree with your point about the potential industry-specific challenges. Different sectors may have varying hardware requirements and diverse data sources. Customization becomes essential in these cases to ensure accurate and reliable predictive maintenance outcomes.
The adoption of ChatGPT for predictive maintenance will likely increase significantly in the near future. We are witnessing a growing demand for data-driven decision-making and optimization. As organizations recognize the potential benefits and overcome the challenges, they will embrace this technology to gain a competitive edge and drive efficiency.
Securing data and models is paramount in any predictive maintenance solution. Regular security assessments, encryption, and access controls are essential to safeguard sensitive information from potential cyber threats.
I appreciate all the insights shared here. The potential benefits of leveraging ChatGPT for predictive maintenance seem substantial. As organizations strive for operational excellence, it's exciting to see how AI can play a crucial role in optimizing hardware support efficiency.
I'm glad this discussion touched upon the security aspect, as it is often an overlooked concern in the pursuit of technological advancements. It's crucial to have robust security measures in place to protect sensitive data from potential breaches.
The potential of predictive maintenance using ChatGPT is undoubtedly exciting. With the right implementation and adaptation, organizations can revolutionize their maintenance practices and move towards more efficient and proactive strategies.
I agree, Jonathan. The ability to predict and prevent equipment failures can lead to substantial cost savings and a significant improvement in overall operational efficiency. Organizations that embrace this approach early on can stay ahead in their respective industries.
Precisely, Sophie. The advantages that predictive maintenance using ChatGPT brings, such as reducing unplanned downtime, optimizing maintenance schedules, and streamlining resource allocation, can drive organizations towards improved productivity and profitability.
I appreciate your response, Juliette. Addressing the potential security vulnerabilities is crucial for the widespread adoption of predictive maintenance technologies. Ensuring robust cybersecurity measures and regular risk assessments will help instill confidence in the reliability and integrity of the systems.
I completely agree, Sophie. Iterative learning and continuous improvement should be an integral part of deploying predictive maintenance systems. By capturing insights from real-world data, organizations can refine their models and enhance their performance over time.
This technology has the potential to be a game-changer for industries reliant on critical hardware systems. The ability to proactively maintain equipment and prevent costly breakdowns can have a significant positive impact on both productivity and customer satisfaction.
Indeed, Sophie. Embracing AI technologies like ChatGPT for predictive maintenance can optimize uptime, resource utilization, and overall equipment effectiveness. It enables organizations to shift from traditional, reactive maintenance to more proactive and strategic approaches.
You're absolutely right, Jonathan. The shift towards proactive and strategic maintenance practices can unlock substantial gains for organizations. By leveraging AI and ChatGPT, companies can harness insights from their data and make smarter decisions to optimize system performance and reduce failures.
Predictive maintenance using ChatGPT has immense potential, indeed. Beyond the technical aspects, organizations should also prioritize change management and cultivating a culture of embracing new technologies. Smooth implementation and adoption are critical to realizing the full benefits.
Absolutely, Jack. Embracing technological advancements like ChatGPT opens doors to innovation and competitive advantages. Organizations should proactively invest in upskilling and change management processes to ensure a smooth transition to predictive maintenance practices.
I appreciate the insights shared here. The discussions highlight the potential of predictive maintenance using ChatGPT and also emphasize the challenges that organizations need to address. It's exciting to witness the convergence of AI technologies and industrial practices to optimize efficiency and reduce operational risks.
Well said, Jonathan. The future of maintenance lies in leveraging AI and advanced data analytics to drive smarter decision-making and ensure optimal performance of critical assets. It's an exciting time for industries looking to unlock new potentials and drive innovation.