Enhancing Logistics Optimization with ChatGPT: A Groundbreaking Approach using Mathematical Programming Technology
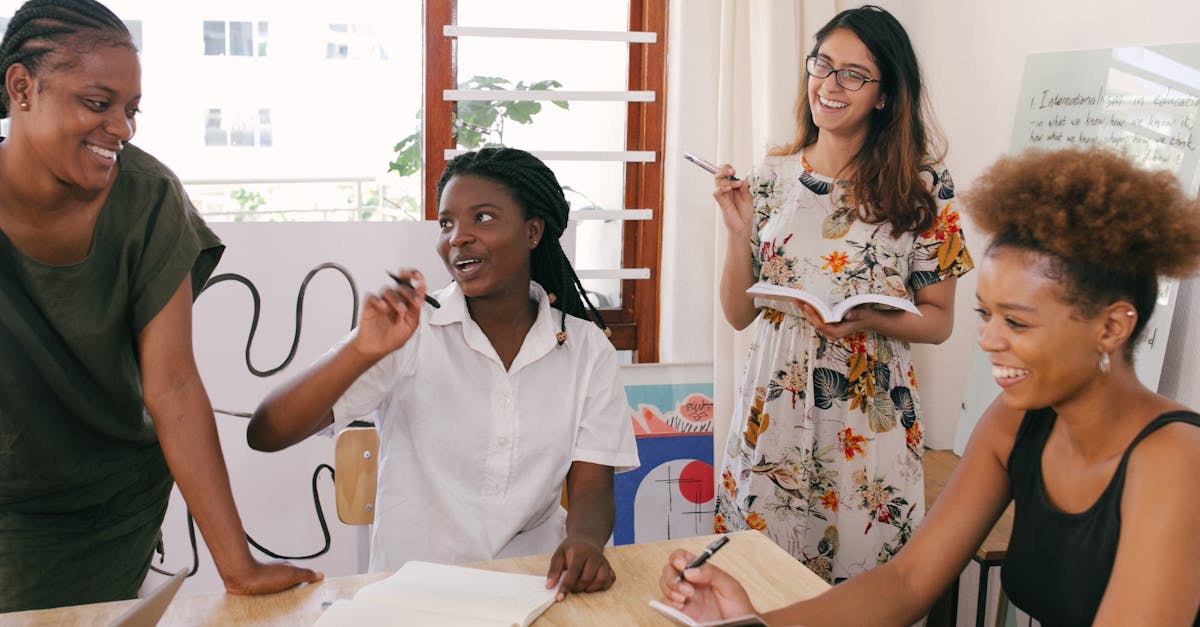
In the world of logistics, efficiency plays a crucial role in ensuring the smooth operation of supply chain networks. As businesses strive to streamline their operations and minimize costs, the application of mathematical programming techniques has become essential. One such tool that shows great promise in this field is ChatGPT-4.
What is Mathematical Programming?
Mathematical programming, also known as mathematical optimization, is a branch of operations research that uses mathematical models to find the best possible solution to a given problem. It involves the use of mathematical techniques, algorithms, and data analysis to optimize complex systems and make informed decisions.
The Role of Mathematical Programming in Logistics Optimization
Logistics optimization is all about finding the most efficient way to transport goods from one location to another while minimizing costs and maximizing resource utilization. Mathematical programming techniques provide a powerful framework for tackling these challenges.
ChatGPT-4, an advanced language model developed by OpenAI, can be effectively utilized in logistics optimization through its mathematical programming capabilities. By leveraging its natural language understanding and generation capabilities, ChatGPT-4 can assist logistics professionals in various mathematical aspects of their work.
Cost Minimization
One crucial factor in logistics optimization is cost minimization. ChatGPT-4 can help in this area by analyzing the relevant cost variables, such as transportation costs, storage costs, and inventory carrying costs. It can then suggest optimal strategies to minimize these costs without compromising the quality and efficiency of the logistics operation.
Route Optimization
Another important aspect of logistics optimization is finding the best routes for transporting goods. ChatGPT-4 can assist in this area by using mathematical programming techniques to analyze various factors, such as distance, traffic patterns, and delivery time windows. It can then generate optimized routes that minimize transportation time and costs while maximizing delivery efficiency.
Furthermore, ChatGPT-4 can help logistics professionals consider constraints and variables that influence route optimization, such as vehicle capacity, driver availability, and customer preferences. By taking these factors into account, it can generate tailored solutions that meet specific requirements and lead to improved logistics operations.
The Future of Logistics Optimization with ChatGPT-4
The integration of ChatGPT-4 into logistics operations has the potential to revolutionize the field of logistics optimization. By combining the power of mathematical programming with advanced natural language generation capabilities, ChatGPT-4 can assist logistics professionals in making data-driven decisions at a whole new level.
With the ability to understand complex logistics problems and generate optimized solutions, ChatGPT-4 opens up new possibilities for cost reduction, improved delivery times, and enhanced customer satisfaction. By harnessing its potential, businesses can gain a competitive edge in an increasingly fast-paced and demanding logistics landscape.
Conclusion
Mathematical programming, when combined with AI-powered language models like ChatGPT-4, gives logistics professionals a powerful tool for optimizing their operations. From cost minimization to route optimization, ChatGPT-4 can provide valuable insights and generate tailored solutions that improve efficiency and drive business success in the logistics industry. As technology continues to evolve, the potential for further advancements in logistics optimization is vast, and ChatGPT-4 is at the forefront of this exciting progress.
Comments:
Thank you all for joining the discussion on my blog article! I'm excited to discuss the groundbreaking approach of using ChatGPT for enhancing logistics optimization with mathematical programming technology. Let's dive into the conversation!
Great article, Claire! This approach seems promising in terms of improving logistics optimization. Can you share some real-world examples where ChatGPT has been successfully used in this area?
Brian, I can share an example from my experience. We implemented ChatGPT in a warehouse management system, where it helped optimize order picking routes. It reduced travel time by 15% and significantly improved efficiency.
Brian, another successful implementation of ChatGPT in logistics is in demand forecasting. By analyzing historical data and consumer interactions, ChatGPT can provide accurate demand predictions, which helps optimize inventory management and avoid stockouts.
Thanks for the clarification, Brian. It's encouraging to see successful applications across different aspects of logistics optimization. The ability to optimize resources, reduce costs, and enhance decision-making is incredibly valuable.
Sophia, another potential drawback is the interpretability of results. While ChatGPT offers robust optimization, the decision-making process may lack transparency due to the underlying neural network's complexity. It's crucial to balance the benefits with the need for interpretability, especially in regulated domains.
Thanks, Andrew and Oliver, for sharing those examples. It's impressive to see the positive impact of ChatGPT in streamlining various logistics operations. The versatility of this approach opens up exciting possibilities!
Hi Claire, thanks for sharing your insights. I'm curious about the limitations of using ChatGPT in logistics optimization. Are there any challenges or drawbacks we should be aware of?
I agree with Sophia. Although ChatGPT shows promise, it's important to consider potential biases and ethical concerns. How can we ensure fair decision-making and avoid perpetuating any biases in logistics optimization?
That's an excellent point, Emily. Fairness and unbiased decision-making are crucial. It's important to regularly evaluate datasets for biases, followed by appropriate mitigation strategies. Careful monitoring, diverse perspectives, and audits can help ensure the optimization process remains fair and ethical.
Claire, what strategies can organizations use to validate the optimization results generated by ChatGPT during the logistics optimization process?
Emily, organizations can employ various strategies to validate optimization results. Some common approaches include comparing the model output against historical data, conducting sensitivity analyses, performing A/B testing, and continuously monitoring and fine-tuning the model's performance. The process should be iterative, utilizing feedback loops and incorporating expert knowledge to ensure the generated results align with the practical feasibility and objectives of the logistics optimization problem.
Validating the optimization results is essential, Emily. By conducting regular reviews and validation processes, organizations can ensure the outputs generated by ChatGPT align with the expected outcomes and practical constraints. The involvement of domain experts plays a crucial role in identifying any discrepancies or issues.
I think ethical considerations are essential, Emily. We must ensure transparency in the optimization process while explicitly addressing biases at various stages. Regular audits and clear guidelines can help avoid perpetuating biases and ensure a fair and inclusive approach.
Michael, you're absolutely right. Transparency is key. Auditing the decision-making process and ensuring diverse stakeholder involvement can help spot biases and develop approaches that prioritize fairness and inclusivity.
Absolutely, Sophia. Accurate demand forecasting leads to better inventory management, reduced costs, and improved customer satisfaction due to fewer stockouts or overstock situations.
Oliver, accurate demand forecasting directly impacts inventory levels and procurement decisions. By utilizing ChatGPT's capabilities, logistics operations can avoid understocking, overstocking, and improve cost-efficiency. It's an exciting use case!
Valid concern, Sophia. Companies using ChatGPT should have robust moderation systems in place to review and filter suggestions before implementing them. Human reviewers can ensure intervention when necessary and refine the model to minimize any potential issues.
Hello, Claire! I'm curious about the timeframe to implement ChatGPT for logistics optimization. How long does it typically take from inception to deployment?
Hi Claudia! The implementation timeframe can vary depending on factors like the complexity of the logistics processes, available data, and the level of integration required. Generally, it can take several weeks to a few months from inception to deployment. Each use case requires careful planning and considerations.
Thanks for the prompt response, Claire! It's helpful to have an understanding of the typical timeframe involved. Planning and resource allocation should be considered thoroughly to ensure smooth implementation.
You're welcome, Claudia! Indeed, careful planning and allocation of resources contribute to a successful implementation. Starting with smaller-scale pilots and gradually expanding the application's scope allows organizations to navigate challenges effectively.
Claire, how does the computational complexity of incorporating mathematical programming technology with ChatGPT compare to traditional optimization methods?
Julia, the computational complexity of integrating mathematical programming technology with ChatGPT is generally higher compared to traditional optimization methods. The neural network calculations, coupled with optimization algorithms, require more substantial computational resources. However, advancements in hardware and optimization techniques have made the integration feasible and efficient for various real-world applications.
Thank you for the explanation, Claire. It's interesting to see the benefits of combining the power of neural networks with mathematical programming for more effective logistics optimization.
Claire, given the dependence on data quality, what steps should organizations take to ensure accurate data collection and cleaning for optimal ChatGPT performance?
Sophia, organizations should focus on data collection from reliable sources and ensure it represents the relevant real-world scenarios. Data cleaning processes should be applied to remove anomalies, errors, and inconsistencies. Validating the data against ground truth or established industry standards is also critical.
Sophia, accurate demand forecasting also enables effective production planning, reducing lead times, and minimizing waste in manufacturing processes. The potential cost savings and efficiency gains are significant.
Oliver, I completely agree. The entire supply chain can benefit from accurate demand forecasting, resulting in reduced lead times, less waste, and increased overall operational efficiency.
Absolutely, Sophia! Effective demand forecasting reduces the risk of lost sales due to stockouts or excess inventory, ultimately improving customer satisfaction and profitability.
Sophia, accurate demand forecasting is also essential in maintaining optimal production levels, reducing wastage, and ensuring resource allocation aligns with customer needs. It enables effective supply chain management as well.
I appreciate your response, Michael. Transparency and accountability are crucial to building trust in the optimization process. Companies must not only focus on the benefits but also address potential risks and ethical implications.
Transparency alone may not be enough, Emily. Continuous monitoring and evaluation by an independent body can help identify and rectify any biases or unintended consequences. This external review process adds an additional layer of checks and balances.
Besides transparency, Michael, actively involving impacted stakeholders in the optimization design and decision-making process enhances the chances of detecting biases and ensuring fairness. Collaboration and diverse perspectives are essential.
Transparency and collaboration, David, are key pillars. By involving stakeholders representing diverse backgrounds and ensuring an inclusive approach, we can develop optimization models that align with societal values and avoid undue harm.
Hi Brian, thank you! Yes, there have been successful implementations of ChatGPT in logistics. One example is its application in intelligent fleet management systems. ChatGPT can assist in decision-making, route planning, and resource allocation, improving overall efficiency. Hi Sophia, great question! While ChatGPT offers significant advantages, one limitation is its dependence on data quality. Inaccurate or incomplete data can lead to suboptimal outcomes. Additionally, the model's lack of true understanding may result in occasional inappropriate suggestions. Proper validation and integration are crucial to overcome these challenges.
Claire, I find the idea of combining mathematical programming technology with ChatGPT fascinating. However, could you elaborate on the computational requirements of this approach? Are there any concerns regarding scalability?
Daniel, the computational requirements depend on various factors like model size, data volume, and complexity of optimization. While scalability is a consideration, advancements in hardware and parallel processing techniques enable efficient calculations. However, it's essential to evaluate the computational infrastructure and model performance for each use case.
Claire, considering the occasional inappropriate suggestions you mentioned, what measures can be taken to prevent any potential issues or ensure intervention when necessary?
Good question, Sophia. It's crucial to incorporate human-in-the-loop mechanisms during optimization. Implementing human oversight, monitoring system outputs, and providing clear guidelines for intervention can help address any potential issues and avoid undesirable outcomes.
Daniel, regarding scalability, advancements in cloud computing and distributed architecture have proven to handle the computational requirements of ChatGPT-based applications effectively. Scalability challenges can be mitigated through robust infrastructure planning and resource allocation.
Daniel, considering the complexity of integrating mathematical programming and ChatGPT, what factors should organizations consider while selecting optimization algorithms to ensure efficient performance?
Sophia, organizations should consider factors like problem structure, problem size, available input data, and specific constraints when selecting optimization algorithms. It's crucial to balance computational efficiency, accuracy, and scalability while considering the specific requirements of the logistics optimization problem at hand.
Thank you for the insights, Daniel. Organizations need to assess the specific requirements and constraints to ensure the selection of optimization algorithms that align with their logistics optimization goals effectively.
Indeed, Sophia. Accurate demand forecasting plays a key role in successful supply chain management. It impacts procurement, production planning, and inventory optimization, leading to improved customer satisfaction and cost efficiency.
Oliver, precise demand forecasting strengthens the resilience and adaptability of the entire supply chain. Manufacturers can adjust production levels, suppliers can optimize inventory, and retailers can meet customer demands effectively. It's a win-win situation!
Sophia and Oliver, accurate demand forecasting eliminates the guesswork involved in supply chain management. It empowers organizations to make data-driven decisions and provides a competitive edge by reducing costs and enhancing customer satisfaction.
Daniel, could you elaborate on the benefits of using mathematical programming technology alongside ChatGPT? How does it enhance the optimization process compared to using either approach alone?
Claudia, combining mathematical programming technology with ChatGPT offers several advantages. Mathematical programming provides a solid framework for optimization, allowing precise modeling of logistics problems. The power of ChatGPT adds flexibility and adaptability to accommodate the dynamic nature of real-world scenarios. Together, they enhance the decision-making process, improve resource allocation, and identify optimal solutions that may not be apparent using either approach alone.
Well said, Daniel! Mathematical programming enhances the reliability and accuracy of optimization, while ChatGPT brings in contextual understanding and generates valuable insights. This combined approach leverages the strengths of both technologies, resulting in improved logistics optimization and increased operational efficiency.